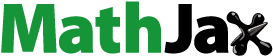
ABSTRACT
Paediatric cancer survivors often suffer from cognitive long-term difficulties. Consequently, strengthening cognition is of major clinical relevance. This study investigated cerebral changes in relation to cognition in non-brain tumour paediatric cancer survivors after working memory or physical training compared to a control group. Thirty-four children (≥one-year post-treatment) either underwent eight weeks of working memory training (n = 10), physical training (n = 11), or a waiting period (n = 13). Cognition and MRI, including arterial spin labelling and diffusion tensor imaging, were assessed at three time points (baseline, post-training, and three-month follow-up). Results show lower cerebral blood flow immediately after working memory training (z = −2.073, p = .038) and higher structural connectivity at the three-month follow-up (z = −2.240, p = .025). No cerebral changes occurred after physical training. Short-term changes in cerebral blood flow correlated with short-term changes in cognitive flexibility (r = −.667, p = .049), while long-term changes in structural connectivity correlated with long-term changes in working memory (r = .786, p = .021). Despite the caution given when interpreting data from small samples, this study suggests a link between working memory training and neurophysiological changes. Further research is needed to validate these findings.
Introduction
Due to early diagnosis and improved treatment approaches, long-term survival rates for paediatric cancer survivors are 80% (Xu et al., Citation2016). However, this promising improvement comes with an increased number of paediatric cancer survivors suffering from adverse long-term effects, such as cognitive difficulties (Krull et al., Citation2018). Cognitive long-term sequelae are associated with impaired daily functioning and quality of life (Kunin-Batson et al., Citation2014). In a previous cross-sectional study, our young Swiss paediatric cancer survivors likewise revealed long-term cognitive difficulties (Siegwart et al., Citation2020). In addition, paediatric cancer survivors reveal significant structural and functional brain alterations (Kesler et al., Citation2021). Even non-brain tumour paediatric cancer survivors present structural and functional brain alterations associated with cognitive performance (Kesler et al., Citation2014; Siegwart et al., Citation2021; Spitzhüttl et al., Citation2020; van der Plas et al., Citation2017). Brain alterations presumably emerge as a consequence of cancer itself and the cytotoxic cancer treatments (Kesler et al., Citation2016). Treatment-related factors such as cranial radiotherapy (Spiegler et al., Citation2004) and high-dose chemotherapy (Sleurs et al., Citation2019) specifically contribute to these changes. For instance, leukaemia patients receiving central nervous system (CNS)-directed treatment face a higher risk for cognitive difficulties (Williams & Cole, Citation2021). However, it is important to note that even cancer treatment that is not directed at the CNS can cause neural damage (Ahles & Saykin, Citation2007; Sleurs et al., Citation2016).
Cancer and its treatment during childhood and adolescence disrupt brain maturation processes such as angiogenesis, synaptic pruning, and myelination (Gogtay et al., Citation2004; Ikonomidou, Citation2018). Because brain maturation is associated with cognitive development, cerebral alterations due to cancer and its treatment can impede the developmental trajectory of cognitive functions (Hearps et al., Citation2017). Evidence-based and effective interventions are needed to counteract long-term cognitive sequelae in children and adolescents. Cognitive and physical training programmes have been applied to foster cognitive functioning in various children and adolescents with atypical development. Cogmed (Klingberg et al., Citation2005) is a cognitive training programme often used in clinical practice. The training focuses on strengthening the working memory capacity. It is based on the assumption that through intensive and repeated training, working memory but ideally also untrained cognitive functions can be improved (near- and far-transfer effect; Sala & Gobet, Citation2020; Shipstead et al., Citation2012). In paediatric survivors of brain tumour and leukaemia, studies have found beneficial effects of Cogmed on cognitive functions (Conklin et al., Citation2015, Citation2017; Hardy et al., Citation2013). A recent meta-analysis including typically developing children and an active control group reported a significant effect of working memory training on cognitive skills directly related to the working memory training (near transfer g = 0.47), whereas little to no evidence was found regarding far-transfer effects (g = 0.09; Sala & Gobet, Citation2020). These results are in line with the conclusions of other meta-analyses on working memory training in the healthy population (Aksayli et al., Citation2019; Melby-Lervåg et al., Citation2016; Sala et al., Citation2019). Consequently, working memory training is repeatedly being criticized because of its specificity and lack of generalizability (Kassai et al., Citation2019; Melby-Lervåg & Hulme, Citation2013).
Physical training programmes are another promising strategy to support cognitive development (Pesce et al., Citation2021). Studies have shown beneficial effects of physical exercise on a range of cognitive functions in healthy children and adolescents (Singh et al., Citation2019). Cognitively challenging physical exercises are reported to particularly improve cognitive functions such as executive functions (Pesce, Citation2012; Tomporowski & Pesce, Citation2019). Exergaming (i.e., active video gaming) can be designed as physical training that fosters cognitive functions (Benzing & Schmidt, Citation2018). Therefore, it is increasingly used to improve cognitive functions in clinical-related settings (Stanmore et al., Citation2017). A very recent systematic review meta-analysis on the effect of physical training on cognitive functions included 1277 individuals across 18 randomized controlled trials (RCT), all of whom were affected by paediatric cancer (Bernal et al., Citation2023). The authors present moderate evidence that, compared with control, physical activity and exercise improved objective cognitive performance measures (five RCTs; Hedges’ g = 0.40) and patient-reported measures of cognitive function (13 RCTs; Hedges’ g = 0.26). In our previous study (Brainfit study; Benzing et al., Citation2018), we investigated the effectiveness of the Cogmed and Exergame training in 69 paediatric cancer survivors and revealed an improvement of the trained visual working memory after Cogmed training (near transfer effect), but no training effects on cognitive functions after the Exergame training (Benzing et al., Citation2020).
The literature suggests that cognitive and physical interventions might result in structural and functional changes in the brain (Buschkuehl et al., Citation2012; Hötting & Röder, Citation2013), which may transfer to behavioural changes. Cerebral blood flow (CBF) is considered a crucial physiological marker for healthy brain development and an important contributor to neurorehabilitation (Liu et al., Citation2018; Wang & Licht, Citation2006). The development of CBF is known to undergo a decrease during late childhood and early adolescence in healthy children (Biagi et al., Citation2007; Liu et al., Citation2018). However, it likely follows atypical pathways in non-brain tumour paediatric cancer survivors (Schuerch et al., Citation2023). CBF was closely associated with long-term cognitive and motor functions in non-brain tumour paediatric cancer survivors, with higher CBF relating to lower cognitive and motor functions as presented in one of our previous cross-sectional studies (Schuerch et al., Citation2023). Studies in healthy adults suggest that CBF increases emerge after short and high-demanding cognitive trainings (as additional executive control is needed to perform the task) whereas CBF decreases occur after longer trainings when participants are able to perform the task effortlessly (Buschkuehl et al., Citation2014; Schneiders et al., Citation2012). The heightened activity during cognitive training may trigger changes in local blood flow to meet the metabolic demands of active neurons, a phenomenon known as neurovascular coupling (Bogorad et al., Citation2019). Furthermore, cognitive training could potentially impact blood vessel structure and function, enhancing CBF in brain regions associated with the trained cognitive functions (Bogorad et al., Citation2019). In addition, cognitive training might stimulate the release of neurotransmitters and neurotrophic factors. These substances can influence blood vessel dilation and promote angiogenesis, which could contribute to changes in cerebral blood flow (Bogorad et al., Citation2019; Toda et al., Citation2009). To summarize, changes in CBF might be one of the underlying cerebral mechanisms of cognitive improvements after working memory training.
Not only working memory training but also physical training can entail changes in CBF. The literature from healthy adults suggests that physical exercise might lead to improved cognitive functions through changes in vascularization and CBF as indirectly measured with functional magnetic resonance imaging (Hötting & Röder, Citation2013). Furthermore, a study assessing healthy brain aging found a positive relationship between physical activity and CBF (Boraxbekk et al., Citation2016). Chaddock-Heyman et al. (Citation2016) proposed that the results found in adults might also apply to children during cerebral development. However, in patients with breast cancer, physical fitness was associated with CBF, but physical training did not alter CBF (Koevoets et al., Citation2023).
In addition to CBF, white matter is a crucial component of cognitive and physical training effects in the brain. White matter comprises neuronal fibres with varying extents of myelination that enable signal transfer across brain regions and is therefore important for intact brain functioning (Buyanova & Arsalidou, Citation2021). Prior studies have shown increased structural connectivity after two months of working memory training in young healthy adults (Caeyenberghs et al., Citation2016; Takeuchi et al., Citation2010). However, when examining the effect of working memory training in extremely preterm-born children, Kelly et al. (Citation2020, Citation2021) found similar changes in white matter microstructure when comparing working memory training to an active control group. Hence, these two studies showed a common structural connectivity increment in training and control subjects, which yielded evidence against a specific effect of working memory training with respect to white matter metrics. In a systematic review of exercise trials in children with brain tumour, Sharma et al. (Citation2021) propose that exercise has a positive effect on volumetric or diffusion-based neuroimaging outcomes, as well as motor performance and cardiorespiratory fitness. Particularly, Riggs et al. (Citation2017) revealed an increase in structural connectivity after three months of exercise training in paediatric brain tumour survivors.
Based on the above findings we conclude that still little is known about training-related cerebral changes in non-brain tumour paediatric cancer survivors following cognitive or physical training. Therefore, the present study aims to investigate the effects of working memory and physical training on CBF and structural connectivity in non-brain tumour paediatric cancer survivors. By doing so, we hope to gain a better understanding of the underlying mechanisms of training effects. Given the limited and partly inconsistent evidence about training-related cerebral changes in paediatric cancer survivors, we took an exploratory approach refraining from formulating directional hypotheses regarding the cerebral changes following working memory or physical training. We hypothesize that (a) an 8-week computerized working memory or physical training will lead to short- and long-term changes in CBF and structural connectivity in non-brain tumour paediatric cancer survivors and (b) training-related changes in CBF and structural connectivity are related to cognitive changes.
Methods
Design and procedure
This longitudinal randomized controlled study reports on a subsample of the Brainfit study, a clinical trial aiming to evaluate cognitive, motor, and neural characteristics of paediatric cancer survivors and the behavioural and neural effectiveness of working memory and physical training immediately after the training and at three-month follow-up (for more details see Benzing et al., Citation2018, Citation2020). The present analyses focused on the physiological correlates before and after working memory or physical training, namely CBF and structural connectivity. The Brainfit study was approved by the local ethics committee (KEK) of Bern and Zurich, Switzerland (KEK BE 196/15; KEK ZH 2015–0397; ICTRP NCT02749877) and was conducted between January 2017 and December 2018 at the Children’s University Hospital, Inselspital, Bern. In accordance with the Code of Ethics of the World Medical Association (Declaration of Helsinki), legal guardians gave written informed consent and all participants provided assent to participate. At baseline, immediately after the training or waiting period, and at three-month follow-up, cognitive performance was assessed and magnetic resonance imaging (MRI) was conducted (). After baseline assessment, patients were randomized into either one of the intervention groups or the waiting control group using SecuTrial by R.E. adopting the minimization algorithm with age, sex, nonverbal IQ, study centre, and CNS involvement of the cancer diagnosis as stratification factors. Blinding was implemented for investigators conducting the assessments, but due to the nature of supervised intervention studies, double-blinding was not feasible. Furthermore, patients remained blinded to the specific study hypotheses. For more information about the main study, including enrolment and allocation, see Benzing et al. (Citation2018, Citation2020) and Figure S1.
Figure 1. Design of the Brainfit study.
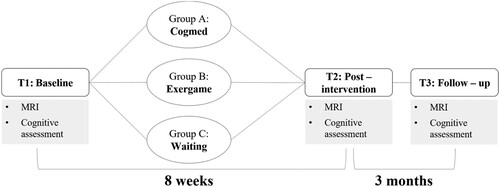
Paediatric cancer survivors
Paediatric cancer survivors registered in the Swiss Childhood Cancer Registry were recruited from the Children’s University Hospital Bern or Zurich, Switzerland. Inclusion criteria were: (a) diagnosed within the past ten years with cancer (e.g., brain tumour, spinal cord, leukaemia), (b) cancer treatment terminated at least 12 months prior to participation (to assess the long-term effects of paediatric cancer), and (c) age between 7 and 16 years at the time of the first assessment. Further, only patients with additional chemotherapy or radiation therapy were included when cancer treatment comprised surgery. Exclusion criteria were: (a) inability to cooperate with study procedures, (b) any unstable health status, (c) substance abuse or noncompliance, and (d) metal items in the body and pregnancy (MRI exclusion criteria). The present study included a subsample of non-brain tumour paediatric cancer survivors (brain and spinal cord were not directly affected by cancer itself) who had undergone MRI in the framework of the Brainfit study (n = 34). Detailed sample description as well as varying sample sizes for the time point comparisons (T1–T2 and T1–T3) are shown in , S2, and S3.
Intervention
Following randomization, the interventions were set up at the participants’ homes. The first training session was carried out under the supervision of specifically trained research assistants. The participants received weekly phone calls from trained coaches throughout the training period. The participants’ parents or legal guardians provided supervision of the training sessions. Group A and B (see ) underwent an eight-week training programme with an individually adjusted difficulty level, with the goal to train three times per week for 45 min. Group A trained the storage and processing of verbal and visuospatial components taxing working memory capacity with a computerized working memory training (Cogmed RM®; Klingberg et al., Citation2005). Group B received a physical training (XBOX Kinects, Microsoft, Redmond, WA). The so-called Exergame (Shape UP, Ubisoft, Montreal) included coordination exercises and dance-like activities that have been shown to be cognitively and physically challenging. Group C served as a waiting control group and was provided with one of the two training programmes after completing the follow-up study (please see Benzing et al. (Citation2020) for further details on the interventions). Groups A and B filled out training diaries throughout the training period, reporting how many training sessions they performed.
Assessments
Assessments took place at the University Hospital in Bern and were conducted by psychologists, postgraduate students, and study assistants under supervision. After each assessment, participants were reimbursed for their travel expenses and compensated with a gift voucher valued at 30 Swiss francs. Age and sex were obtained from questionnaires. Weight and height were measured with a scale and a tape rule. The BMI (kg/m2) was calculated based on weight and height. Socioeconomic status was measured using the German version of the Family Affluence Scale II (FAS; Boudreau & Poulin, Citation2009). Sum scores range from zero to nine, with higher scores marking higher socioeconomic status. Handedness was classified according to the writing hand observed during the neuropsychological assessment. Cancer type, age at diagnosis, type of treatment, and treatment duration were extracted from the Swiss Childhood Cancer Registry and checked using clinical records in case of missing data.
Cognitive assessment
Nonverbal IQ was assessed using the Test of Nonverbal Intelligence, fourth edition (TONI-4; Brown et al., Citation2010; Ritter et al., Citation2011). Executive functions were assessed based on the model of Anderson (Citation2002): Visuospatial working memory was assessed using the Block Recall subtest of the Working Memory Test Battery for Children (WMTB-C; Gathercole et al., Citation2004; Pickering & Gathercole, Citation2001). Inhibition was measured with the third condition of the color-word interference subtest (Stroop task) of the Delis-Kaplan Executive Function System (D-KEFS; Delis et al., Citation2001). Cognitive flexibility was measured with the fourth condition of the color-word interference subtest (Switching task) of the D-KEFS (Delis et al., Citation2001). Planning was assessed using the Rover subtest of the K-ABC II (Melchers & Preuß, Citation1991). Processing speed was evaluated with the processing speed index generated from the subtests coding and symbol search of the German version of the Wechsler Intelligence Scale for Children, fourth edition (WISC-IV; Wechsler, Citation2003).
Neuroimaging
Image acquisition
The neuroimaging data was obtained at the Institute of Diagnostic and Interventional Neuroradiology at the University Hospital of Bern and the MR images were acquired using a 3T whole-body MRI system (Magnetom Prisma, Siemens Medical Systems, Erlangen, Germany) equipped with a 64-channel head coil. A neuroradiologist evaluated all scans.
Structural imaging: T1-weighted structural imaging was conducted using a 3D T1 magnetization-prepared rapid gradient echo (MPRAGE) sequence with the subsequent parameters: total acquisition time, TA = 4:33 min; repetition time, TR = 1950 ms; echo time, TE = 2.19 ms; slices per slap = 176; field of view, FoV = 256 mm × 256 mm; matrix dimension = 256 × 256; isovoxel resolution = 1 mm3.
Arterial spin labelling: For the acquisition of the CBF, we adopted a pulsed arterial spin labelling sequence with a FAIR (slice selective and nonslice selective inversion pulse) labelling scheme combined with a QUIPSSII (saturation scheme) module for bolus cut-off, as depicted in Wang et al. (Citation2003). The subsequent parameters were applied: total acquisition time, TA = 4:59 min, repetition time, TR = 4600 ms, echo time TE = 16.18 ms, bolus duration = 800 ms, inversion time, TI = 1500 ms, field of view, FoV = 192 mm × 192 mm, flip angle = 180°, voxel size = 1.5 × 1.5 × 3.0 mm. Additionally, a M0 image (TA = 2.16 min) for quantification purpose of CBF was recorded with TR = 8000 ms and inversion time TI = 7000 ms. All other parameters were unchanged.
Diffusion tensor imaging: To assess measures of white matter structural connectivity, a spin-echo echo-planar 2D diffusion-weighted imaging (DWI) protocol was run (TA = 1:37 min, TR = 3200 ms, TE = 72 ms, isotropic voxel size = 2.2 mm3, FoV = 220 mm × 220 mm, 100 × 100 matrix, slice number = 54, pixel bandwidth = 2084 Hz/pixel). Diffusion-sensitizing gradients were employed along N = 12 non-collinear directions at b-values of b = 1300 s/mm2 (two averages, separately stored) and one b = image (one average) was acquired per slice.
Preprocessing and analyses
Arterial spin labelling: For the preprocessing, SPM12 and MATLAB (Version R2019b; the MathWorks Inc., Natick, MA, USA) were used. Initially, the structural and functional images were manually reoriented, setting the origin at the anterior commissure. Afterward, the structural images underwent segmentation into gray matter, white matter, and cerebrospinal fluid. Using the gray and white matter images, the structural images were then skull-stripped. To address motion artefacts, the raw pASL images were realigned. Subsequently, CBF quantification was carried out using an in-house MATLAB script. The ASL technique allows for CBF estimation and a calibrated CBF measure was obtained by solving a one-compartment-model (Buxton, Citation2005; Wang et al., Citation2003) with the following equation:
The variables are as follows: blood/tissue water partition coefficient λ = 0.9 g/ml, ΔM represents the difference signal (control − label), T1 = 1650 ms – the standard value recommended for arterial blood at 3T, labelling efficiency α = 0.85, M0 refers to the equilibrium brain tissue magnetization, and the time constants TI and TI1 (=bolus duration) are previously described. To compute whole-brain CBF values, a threshold of 150 was set for the raw ASL images to exclude non-brain contributions. Subsequently, the resulting CBF maps were co-registered to the skull-stripped structural images and normalized to the MNI standard space. Gaussian smoothing (6 × 6 × 6 mm³ Full-Width at Half-Maximum) was applied to reduce inter-individual anatomical differences. To account for the partial volume effect of brain perfusion, each CBF map was masked with the individual normalized segmented gray matter structural image using a threshold of 0.7 to create the gray matter mask. Region of interest (ROI) analyses were performed using explicit masks of the working memory and motor network. The working memory ROI was extracted from a mask based on visuospatial working memory-related activation of our healthy control group during task-based fMRI, as described in Siegwart et al. (Citation2021), where a similar patient subsample to this study was included. The motor ROI was adopted from Sharma and Cohen (Citation2012), as it includes non-primary motor areas (Steiner et al., Citation2021; extracted with the WFU Pickatlas; Version 3.0.5b; https://www.fmri.wfubmc.edu/downloads). This motor ROI has been previously applied in our studies involving paediatric samples (Schuerch et al., Citation2023; Steiner et al., Citation2021).
Diffusion tensor imaging: DTIprep (https://www.nitrc.org/projects/dtiprep/) was used for preprocessing and quality check. A DTI diffusion scheme was employed, capturing data from 12 diffusion sampling directions at a b-value of 1300 s/mm2. We acknowledge that a DTI sequence with a greater number of diffusion directions could yield more comprehensive information. However, due to time restrictions, we applied a short DTI sequence with only 12 diffusion directions. This compromise ensured participant comfort and compliance, thereby enhancing the quality of our study. The acquired images had an in-plane resolution and slice thickness of 2.2 mm. To ensure the accuracy of the b-table, an automatic quality control routine (Schilling et al., Citation2019) with additional visual inspection was performed. The diffusion data underwent reconstruction in the MNI space utilizing q-space diffeomorphic reconstruction (Yeh & Tseng, Citation2011), which generated the spin distribution function (Yeh et al., Citation2010). A diffusion sampling length ratio of 1.25 was applied, resulting in an output resolution of 2 mm isotropic. Restricted diffusion was quantified using restricted diffusion imaging (Yeh et al., Citation2017). For fibre tracking, a deterministic algorithm was employed (Yeh et al., Citation2013). Whole-brain seeding was implemented, with randomly selected thresholds for anisotropy (from a range) and angular threshold (ranging from 15 to 90 degrees). The step size was randomly selected within a range of 0.5–1.5 voxels. To enhance the smoothness of fibre trajectories, the propagation direction was averaged with a randomly selected percentage (ranging from 0% to 95%). Tracts with lengths shorter than 30 mm or longer than 400 mm were discarded. In total, 1'000,'000 seeds were placed. The brain parcellation method known as HCP-MMP was utilized, and the connectivity matrix was calculated using the ncount (connection normalized by the median length) of the connecting tracts. Subsequently, the connectivity matrix and graph theoretical analysis were performed using DSI Studio (http://dsi-studio.labsolver.org). For statistical analyses, we used the median value of the connectivity matrix ranging between zero and one and the number of tracts per thousand [k].
Statistical analyses
The SPSS software (Version 28.0.1.1; IBM Corp., Armonk, NY, USA) was used for statistical analysis. For data visualization, ggplot 2 package in R (Version 3.3.5; Wickham, Citation2016) was used. The significance level was set to p < .05 (two-sided). Cohen’s r was calculated as effect size with r = .10 small effect, r = .30 medium effect, and r = .50 large effect (Cohen, Citation1988). Given the small sample size, non-parametric tests were applied for all analyses. As our analyses were of exploratory nature, they were not corrected for multiple testing (Althouse, Citation2016). Group differences in demographics, medical, and training data were examined using either Kruskal–Wallis H, Mann–Whitney U, or Pearson-Chi2 tests. To analyse possible training-related changes in CBF and structural connectivity, Wilcoxon-signed-rank tests were used for all three groups separately. To assess associations between cerebral changes and changes in outcome measures, Spearman’s rank correlational analyses were calculated. We based our post-hoc correlational analyses on the significant changes (from the Wilcoxon-signed-rank Tests) in CBF and structural connectivity. Raw scores were used for the cognitive variables. Using raw scores has the advantage of providing a more precise evaluation of individual performance and greater variance within the group. To construct a composite score of processing speed, raw scores of the respective subtests were transformed into z-scores using the mean and standard deviation of our patient sample. Change scores (relative deltas, Δ) were calculated by subtracting the value of the baseline assessment (T1) either from the value immediately after the training (T2) or from the follow-up (T3) and dividing it by the value of the baseline assessment ((T2 − T1)/T1 or (T3 − T1)/T1). Relative deltas were created for CBF, structural connectivity, and cognitive performance. We refrained from conducting linear mixed models because we did not fulfil the necessary assumptions. To cancel out learning effects, we compared the relative deltas of the Cogmed group and the Exergame group with the waiting control group. We defined the comparison between the baseline assessment (T1) and the assessment immediately after the training period (T2) as short-term effects. The comparison between the baseline assessment (T1) and the three-month follow-up assessment (T3) was defined as long-term effects. Due to the small and clinically heterogeneous training groups, the relationship between training-related cerebral changes and cancer treatment, cancer type, and other clinical measures was only analysed descriptively.
Results
Demographics, medical characteristics, and training data
The three groups were comparable regarding demographics, medical characteristics, and training sessions (see ). According to the training diaries, only 50% of the Cogmed group and only 54.5% of the Exergame group reached the prescribed amount of at least 20 training sessions. Details on cancer type and cancer treatment are presented in Table S1.
Table 1. Demographics, medical characteristics, and training data at baseline (T1).
Changes in cognitive performance
Cognitive performance did not differ significantly at baseline (T1) between the three groups. Due to small sample sizes, we compared the relative cognitive change (relative deltas) of the Cogmed group and the Exergame group with the waiting control group. After the Cogmed training, short- and long-term changes in visuospatial working memory were significantly more pronounced than in the waiting control group (short-term: U = 27, p = .018; long-term: U = 9, p = .001). After the Exergame training, short- and long-term cognitive changes did not differ significantly from those observed in the waiting control group (see Table S4).
Changes in cerebral blood flow and structural connectivity
CBF and structural connectivity did not differ significantly between the three groups at baseline (T1; ps > .05).
Short-term effects (T1 versus T2)
Immediately after the Cogmed training, CBF within the working memory ROI was significantly reduced when compared to baseline (z = −2.073, p = .038, see and Table S2). At the individual level, there was a decrease in CBF within the working memory ROI in eight of nine patients. In the Cogmed group, global CBF and CBF within the motor ROI did not differ between T1 and T2. After the Exergame training and waiting control period, CBF did not change significantly between T1 and T2.
Figure 2. Short- and long-term effects depicted as a series of boxplots (with interquartile range and minimum and maximum value) in (a) CBF within the working memory ROI and (b) structural connectivity (number of tracts [k]) presented for the three groups. The lines connect the mean values. Depending on comparison (T1–T2, T1–T3), sample sizes for cerebral blood flow analyses differed. Waiting control group: n = 8 to 10; Cogmed group: n = 7 to 9; Exergame group: n = 7 to 10; see Table S2. Depending on comparison (T1–T2, T1–T3), sample sizes for structural connectivity analyses differed. Waiting control group: n = 10 to 13; Cogmed group: n = 8 to 10; Exergame group: n = 7 to 10; see Table S3. *p < 0.05.
![Figure 2. Short- and long-term effects depicted as a series of boxplots (with interquartile range and minimum and maximum value) in (a) CBF within the working memory ROI and (b) structural connectivity (number of tracts [k]) presented for the three groups. The lines connect the mean values. Depending on comparison (T1–T2, T1–T3), sample sizes for cerebral blood flow analyses differed. Waiting control group: n = 8 to 10; Cogmed group: n = 7 to 9; Exergame group: n = 7 to 10; see Table S2. Depending on comparison (T1–T2, T1–T3), sample sizes for structural connectivity analyses differed. Waiting control group: n = 10 to 13; Cogmed group: n = 8 to 10; Exergame group: n = 7 to 10; see Table S3. *p < 0.05.](/cms/asset/0a8d0944-d46f-4865-b0c7-d65be7b0640e/pnrh_a_2356294_f0002_ob.jpg)
Structural connectivity did not change between T1 and T2, neither in the training groups nor the waiting control group, even when excluding the outliers (see Table S3).
Long-term effects (T1 versus T3)
At three-month follow-up (T3), no significant changes in CBF were found in any of the three groups compared to the baseline assessment (see Table S2).
After the Cogmed training, structural connectivity significantly increased at the three-month follow-up when compared to baseline in regard to the number of tracts (z = −2.386, p = .017; see and Table S3) and in regard to the median of the connectivity matrix (z = −2.240, p = .025; see Table S3). At the individual level, there was an increase in structural connectivity in seven of eight patients (for the number of tracts [k] as well as the median of the connectivity matrix). After the physical training and in the waiting control group, structural connectivity remained stable between T1 and T2, even when excluding the outliers (see Table S3).
Association between cerebral changes and cognitive changes
Post-hoc correlational analyses were based on the results of significant training-related cerebral changes. Hence, we only report correlations in the Cogmed group, as only in this group significant cerebral changes occurred. The short-term changes in CBF within the working memory ROI correlated significantly with the short-term changes in cognitive flexibility (r = −.667, p = .049; see ). This indicates that a decrease in CBF within the working memory ROI was related to an increase in cognitive flexibility. In addition, a significant association between the long-term changes in the median of the connectivity matrix and long-term changes in visuospatial working memory was found, which implies that stronger connectivity was related to better visuospatial working memory (r = .786, p = .021; see ). No other significant associations between training-related cerebral and cognitive changes occurred (see Table S5). The number of completed training sessions was unrelated to cerebral changes.
Figure 3. (a) Correlation between short-term changes in CBF within the working memory ROI and short-term changes in cognitive flexibility (n = 9) and (b) Correlation between long-term changes in structural connectivity and long-term changes in visuospatial working memory (n = 8). The solid line represents the linear regression fit. The shaded area around the line indicates the 95% confidence interval.

Discussion
Summary of study results
In this longitudinal study, we examined the effects of working memory and physical training on CBF and structural connectivity and the associations with cognitive functions in non-brain tumour paediatric cancer survivors. First, our exploratory analyses showed that working memory training entailed a short-term decrease in CBF and a long-term increase in structural connectivity. No cerebral changes were found after physical training. Second, we found an association between cerebral changes and changes in visuospatial working memory and cognitive flexibility.
Effect of cognitive training and physical training on cognition
A comprehensive meta-analysis suggests that working memory training can improve the trained functions in healthy children and adolescents, whereas transfer effects to untrained cognitive domains remain scarce (g = 0.09; Sala & Gobet, Citation2020). Our small non-brain tumour survivor sample shows a near transfer effect with improvements in visuospatial working memory performance after the working memory training that goes beyond the improvements seen in the waiting control group. Our results are consistent with data from our larger sample of paediatric cancer survivors (n = 69), including the present participants. In the previous behavioural study, working memory training entailed significant improvements in the trained visuospatial working memory in the short- and long-term, whereas no significant cognitive improvement was found after the physical training (Benzing et al., Citation2020). While there are many studies on the effect of physical activity on different health outcomes (see Cheung et al. (Citation2021) for a systematic review), a very recent review and meta-analysis summarizes the benefit of physical training on cognitive functions in paediatric cancer survivors (Bernal et al., Citation2023). The authors conclude that overall, physical activity and exercise have only moderate effects on cognitive performance (Bernal et al., Citation2023). However, individual studies still demonstrate significant improvements after physical activity training on attention, but no treatment effects on working memory, processing speed, verbal fluency, or memory (Sabel et al., Citation2017). Still, physical training in paediatric cancer survivors have shown to entail significant benefits on motor functions (see Rapti et al. (Citation2023) for a review), self-efficacy (Li et al., Citation2013; Ruble et al., Citation2016), increases in quality of life, decreases in fatigue (Wurz & Brunet, Citation2016; Yeh et al., Citation2011), and improvements in mood (Huang et al., Citation2014). Furthermore, in a previous study including part of the present sample, motor activities and executive functions were closely interrelated in paediatric cancer survivors and lead to the assumption that training motor functions would entail a benefit on cognitive performance (Benzing et al., Citation2022). Hence, we conclude that in our study, the physical training might not have been cognitively stimulating enough or might have suffered from insufficient intensity or low frequency to imply a cognitive change in our paediatric cancer survivor sample. Furthermore, it is important to acknowledge that the present study did not exclusively include paediatric cancer survivors with cognitive deficits. The average cognitive performance of the present sample was within the normative range. In contrast, a study conducted by Conklin et al. (Citation2015), which focused solely on paediatric cancer survivors with cognitive deficits, revealed training effects including far transfer onto untrained cognitive functions following working memory training.
Effect of cognitive training and physical training on cerebral perfusion
There is currently no clear pattern of results on specific neural mechanisms underlying working memory training, neither with respect to changes in neural activation patterns, resting state connectivity, brain structure, nor the dopaminergic system (Buschkuehl et al., Citation2012). Concerning CBF, one study suggested increased perfusion that correlated with task proficiency after seven days of working memory training in healthy young adults (Buschkuehl et al., Citation2014). These results presumably reflect an improved neural readiness or the recruitment of additional functional resources after a short cognitive training. However, the authors suggest that longer-lasting working memory training likely entails a decrease in CBF coming along with more cognitive efficacy and hence, less neural effort during task performance (Buschkuehl et al., Citation2014). Consistent with this notion, the present study found a decrease in CBF after working memory training in the survivor sample. To perform a task effectively, paediatric cancer survivors might need more brain capacity, but in the course of training, this over-recruitment of brain capacity may reduce, suggesting a decrease in cerebral activation coming along with increased cerebral efficiency (Kesler et al., Citation2021; Robinson et al., Citation2010). Likewise, our previous cross-sectional study associated lower CBF with better cognitive and motor performance (Schuerch et al., Citation2023). It is important to note that in the current study, the changes in CBF after working memory training were only short-term, similar to the transient effects of working memory training described in the meta-analytic review of Melby-Lervåg and Hulme (Citation2013).
Regarding the effects of physical training on CBF, the literature is still inconclusive. Our physical training did not facilitate changes in CBF. Nevertheless, since aerobic exercise can promote neuronal plasticity, it is unsurprising that it also promotes cerebrovascular plasticity (Barnes, Citation2015). Furthermore, the potential benefits of aerobic exercise on brain functions are suggested to stem from alterations in CBF, resulting in an improved oxygen supply of the brain (Hötting & Röder, Citation2013). Several studies in mouse models have shown exercise-induced increases in microvascular density in the motor cortex and striatum (Nishijima et al., Citation2016). Robust models to study cerebrovascular plasticity in humans are still continually advancing and need to be applied in health and disease. In breast cancer survivors, moderate to large (but nonsignificant) effects on cognition and CBF were found following high-intensity physical training (Northey et al., Citation2019). Furthermore, in patients with breast cancer, changes in CBF were associated with changes in physical fitness, but physical training did not influence CBF (Koevoets et al., Citation2023).
Effect of cognitive training and physical training on structural connectivity
Our present finding of a long-term increase in structural connectivity after working memory training is in line with the literature. In healthy young adults, an increase in structural connectivity occurred shortly after working memory training (Caeyenberghs et al., Citation2016) and at a two-month follow-up (Takeuchi et al., Citation2010). Similar results are presented in school-aged survivors of neonatal critical illness. Immediately after working memory training, children exhibited increased structural connectivity (Schiller et al., Citation2019). However, Kelly et al. (Citation2020, Citation2021) were unable to replicate these findings in a group of extremely preterm-born children two weeks after working memory training and indicated that the working memory training group revealed a similar increase in structural connectivity as the active control group.
Physical training might similarly affect structural connectivity. For example, Scholz et al. (Citation2009) conducted a six weeks of juggling training with healthy adults and found an increase in structural connectivity immediately after the training and at one-month follow-up. In paediatric brain tumour survivors, Riggs et al. (Citation2017) found an increase in structural connectivity immediately after three months of exercise training and at a six-month follow-up. However, as for CBF, we did not find any changes in structural connectivity after physical training.
Relationship between cerebral and cognitive changes
After the working memory training, we found associations between cerebral and cognitive changes. In specific, short-term changes in CBF were negatively associated with short-term changes in cognitive flexibility. In addition, long-term changes in structural connectivity were positively associated with long-term changes in visuospatial working memory. Likewise, prior studies have shown associations between cerebral changes and cognitive improvement. For example, Schiller et al. (Citation2019) revealed that children (survivors of neonatal critical illness) who cognitively improved after the working memory training had significantly higher structural connectivity than children without improvement. In addition, changes in CBF have been associated with task proficiency in healthy adults after cognitive training (Buschkuehl et al., Citation2014). Taken together, our results further strengthen the proposed relationship between cognitive and cerebral changes after cognitive training.
Summary of cerebral changes
It seems difficult to detect a clear pattern between cerebral and cognitive changes after either cognitive or physical training. In general, training-related cerebral changes are thought to reflect complex, highly dynamic neuroplastic processes that often underlie cognitive changes. In detail, increases in neural activation at the beginning of the training phase are suggested to be followed by decreases in neural activation during the consolidation of the training task (Buschkuehl et al., Citation2012). We hypothesized changes in cerebral perfusion and structural connectivity after cognitive as well as physical training. The present study’s findings align with our previous results revealing specific working memory improvements merely after working memory training (Benzing et al., Citation2020). Despite the lack of cognitive improvements after physical training in our previous behavioural study, it would have been possible that physical training nevertheless entailed cerebral changes. A notion assumes that cerebral changes might function as a precursor for cognitive changes (Kelly et al., Citation2020). Still, our understanding of whether and how cognitive and physical training can contribute to substantial cerebral changes remains limited (Buschkuehl et al., Citation2012). Owing to the high specificity of the working memory training, cerebral changes might be more likely to occur than after the broader and less targeted physical training. Given that previous studies reported cerebral changes following physical training (Hötting & Röder, Citation2013; MacIntosh et al., Citation2017; Riggs et al., Citation2017), again, one needs to keep in mind that the lack of such changes in our study might be due to the insufficient intensity and frequency of training sessions. In addition, one could argue that the applied physical training was not specific enough and needs higher cognitive demands to enhance cognitive processes (Pesce, Citation2012; Tomporowski & Pesce, Citation2019). Furthermore, the time to start with a training regime (during or after cancer) might have an impact on the extent of cerebral changes. As we applied the training with a mean of around four years after cancer and its treatment, we only observed effects in a chronic period, where cerebral and cognitive development after cancer and its treatment have already progressed.
Limitations
The most prominent limitation of the present study is the small sample size, which forced us to use descriptive measures and basic statistical tests, without being able to include possible influencing factors (such as time since treatment or age at diagnosis). Our results might not have enough statistical power to detect the training effects of the training interventions. Furthermore, it is important to note that our analyses were of exploratory nature and therefore, we did not correct for multiple testing. Moreover, the present study solely provided a basic categorization of cancer types and treatment modalities. Future studies should include more detailed treatment information to examine differential impacts of cancer-related risk factors particularly focusing on whether cancer treatment was CNS-directed (e.g., intrathecal chemotherapy, cranial radiotherapy). In addition, our participants completed a low number of training sessions, with considerable variance in both training groups. Lastly, our DTI sequence only included 12 directions and with a TA = 1:37 min, which limits the explanatory power of the results. Thus, our results should be interpreted as exploratory findings.
Future perspectives
Working memory training specifically improves working memory performance and might not be universally suitable to improve cognitive functioning in paediatric cancer survivors generally. Hence, it is crucial to comprehensively assess cognitive functioning to identify individuals with working memory difficulties and further specifically tailor working memory training for these individuals. Additionally, future training studies should integrate the individual preferences and motivational factors of participants (Katz et al., Citation2021). To further improve patient adherence and sustainability in real-word situations, e-health approaches, including regular reminders, social exchange, and an individualized feedback system might be beneficial. Moreover, a combination of cognitive and physical training (rather than isolated, specific trainings of cognitive and motor performance) offers an interesting approach since cognitive and motor functions are tightly interrelated and mutually develop during childhood (Benzing et al., Citation2022; Van Waelvelde et al., Citation2020). The combination of cognitive and physical trainings might also address the before mentioned issue of working memory trainings being too specific and physical trainings not targeting cognitive functions enough. Future training studies could additionally increase training effects and generalization by incorporating social interactions through group settings (Riggs et al., Citation2017; Schmidt et al., Citation2015), social media, or by including neurostimulation such as transcranial direct current stimulation which likely supports neural changes during training (Quinn et al., Citation2020).
Conclusion
To conclude, a short-term decrease in CBF and a long-term increase in structural connectivity occurred in non-brain tumour paediatric cancer survivors after working memory training whereas no cerebral changes emerged after the physical training. Further, associations between cerebral and cognitive changes were presented. Despite the small sample size, the present findings offer a first valuable insight into the training-related neuroplasticity in non-brain tumour paediatric cancer survivors years after cancer and its treatment.
Schuerch_Supplement_NeuropsychRehab_updated.docx
Download MS Word (77.6 KB)Acknowledgments
We thank the participating children, their parents, and the team of the Swiss Childhood Cancer Registry for their support. KS: Formal analysis, Investigation, Methodology, Software, Validation, Visualization, Roles/Writing – original draft, Writing – review and editing; MG: Formal analysis, Investigation, Methodology, Software, Supervision, Validation, Roles/Writing – original draft, Writing – review and editing; VB: Conceptualization, Data curation, Project administration, Supervision, Writing – review and editing; VS: Data curation, Project administration, Supervision, Writing – review and editing; AF: Formal analysis, Methodology, Software, Writing – review and editing; NS: Supervision, Validation, Writing – review and editing; CK: Methodology, Software, Validation, Writing – review and editing; JR: Resources, Writing – review and editing; RE: Conceptualization, Data curation, Funding acquisition, Investigation, Methodology, Project administration, Resources, Supervision, Roles/Writing – original draft; Writing – review and editing.
Disclosure statement
The authors report no potential conflict of interest. JR is meanwhile an employee of Novartis Pharma, Basel.
Data availability statement
The data that support the findings of this study are available from the corresponding author, [RE], upon reasonable request.
Additional information
Funding
References
- Ahles, T. A., & Saykin, A. J. (2007). Candidate mechanisms for chemotherapy-induced cognitive changes. Nature Reviews Cancer, 7(3), 192–201. https://doi.org/10.1038/nrc2073
- Aksayli, N. D., Sala, G., & Gobet, F. (2019). The cognitive and academic benefits of Cogmed: A meta-analysis. Educational Research Review, 27(February), 229–243. https://doi.org/10.1016/j.edurev.2019.04.003
- Althouse, A. D. (2016). Adjust for multiple comparisons? It’s not that simple. The Annals of Thoracic Surgery, 101(5), 1644–1645. doi:10.1016/j.athoracsur.2015.11.024
- Anderson, P. (2002). Assessment and development of executive function (EF) during childhood. Child Neuropsychology, 8(2), 71–82. https://doi.org/10.1076/chin.8.2.71.8724
- Barnes, J. N. (2015). Exercise, cognitive function, and aging. Advances in Physiology Education, 39(1), 55–62. https://doi.org/10.1152/advan.00101.2014
- Benzing, V., Eggenberger, N., Spitzhüttl, J., Siegwart, V., Pastore-Wapp, M., Kiefer, C., Slavova, N., Grotzer, M., Heinks, T., Schmidt, M., Conzelmann, A., Steinlin, M., Everts, R., & Leibundgut, K. (2018). The Brainfit study: Efficacy of cognitive training and exergaming in pediatric cancer survivors – A randomized controlled trial. BMC Cancer, 18(1), 18. https://doi.org/10.1186/s12885-017-3933-x
- Benzing, V., & Schmidt, M. (2018). Exergaming for children and adolescents: Strengths, weaknesses, opportunities and threats. Journal of Clinical Medicine, 7(11), 422. https://doi.org/10.3390/jcm7110422
- Benzing, V., Siegwart, V., Anzeneder, S., Spitzhüttl, J., Grotzer, M., Roebers, C. M., Steinlin, M., Leibundgut, K., Everts, R., & Schmidt, M. (2022). The mediational role of executive functions for the relationship between motor ability and academic performance in pediatric cancer survivors. Psychology of Sport and Exercise, 60(February), 102160. https://doi.org/10.1016/j.psychsport.2022.102160
- Benzing, V., Spitzhüttl, J., Siegwart, V., Schmid, J., Grotzer, M., Heinks, T., Roebers, C. M., Steinlin, M., Leibundgut, K., Schmidt, M., & Everts, R. (2020). Effects of cognitive training and exergaming in pediatric cancer survivors—A randomized clinical trial. Medicine & Science in Sports & Exercise, 52(11), 2293–2302. https://doi.org/10.1249/MSS.0000000000002386
- Bernal, J. D. K., Recchia, F., Yu, D. J., Fong, D. Y., Wong, S. H. S., Chung, P. K., Chan, D. K. C., Capio, C. M., Yu, C. C. W., Wong, S. W. S., Sit, C. H. P., Chen, Y. J., Thompson, W. R., & Siu, P. M. (2023). Physical activity and exercise for cancer-related cognitive impairment among individuals affected by childhood cancer: A systematic review and meta-analysis. The Lancet Child & Adolescent Health, 7(1), 47–58. https://doi.org/10.1016/S2352-4642(22)00286-3
- Biagi, L., Abbruzzese, A., Bianchi, M. C., Alsop, D. C., Del Guerra, A., & Tosetti, M. (2007). Age dependence of cerebral perfusion assessed by magnetic resonance continuous arterial spin labeling. Journal of Magnetic Resonance Imaging, 25(4), 696–702. https://doi.org/10.1002/jmri.20839
- Bogorad, M. I., DeStefano, J. G., Linville, R. M., Wong, A. D., & Searson, P. C. (2019). Cerebrovascular plasticity: Processes that lead to changes in the architecture of brain microvessels. Journal of Cerebral Blood Flow & Metabolism, 39(8), 1413–1432. https://doi.org/10.1177/0271678X19855875
- Boraxbekk, C. J., Salami, A., Wåhlin, A., & Nyberg, L. (2016). Physical activity over a decade modifies age-related decline in perfusion, gray matter volume, and functional connectivity of the posterior default-mode network—A multimodal approach. NeuroImage, 131, 133–141. https://doi.org/10.1016/j.neuroimage.2015.12.010
- Boudreau, B., & Poulin, C. (2009). An examination of the validity of the family affluence scale II (FAS II) in a general adolescent population of Canada. Social Indicators Research, 94(1), 29–42. https://doi.org/10.1007/s11205-008-9334-4
- Brown, L., Sherbenou, R. J., & Johnsen, S. K. (2010). TONI-4, test of nonverbal intelligence. Pro-ed.
- Buschkuehl, M., Hernandez-Garcia, L., Jaeggi, S. M., Bernard, J. A., & Jonides, J. (2014). Neural effects of short-term training on working memory. Cognitive, Affective, & Behavioral Neuroscience, 14(1), 147–160. https://doi.org/10.3758/s13415-013-0244-9
- Buschkuehl, M., Jaeggi, S. M., & Jonides, J. (2012). Neuronal effects following working memory training. Developmental Cognitive Neuroscience, 2(SUPPL. 1), S167–S179. https://doi.org/10.1016/j.dcn.2011.10.001
- Buxton, R. B. (2005). Quantifying CBF with arterial spin labeling. Journal of Magnetic Resonance Imaging, 22(6), 723–726. https://doi.org/10.1002/jmri.20462
- Buyanova, I. S., & Arsalidou, M. (2021). Cerebral white matter myelination and relations to age, gender, and cognition: A selective review. Frontiers in Human Neuroscience, 15(July), 1–22. https://doi.org/10.3389/fnhum.2021.662031
- Caeyenberghs, K., Metzler-Baddeley, C., Foley, S., & Jones, D. K. (2016). Dynamics of the human structural connectome underlying working memory training. The Journal of Neuroscience, 36(14), 4056–4066. https://doi.org/10.1523/JNEUROSCI.1973-15.2016
- Chaddock-Heyman, L., Erickson, K. I., Chappell, M. A., Johnson, C. L., Kienzler, C., Knecht, A., Drollette, E. S., Raine, L. B., Scudder, M. R., Kao, S. C., Hillman, C. H., & Kramer, A. F. (2016). Aerobic fitness is associated with greater hippocampal cerebral blood flow in children. Developmental Cognitive Neuroscience, 20, 52–58. https://doi.org/10.1016/j.dcn.2016.07.001
- Cheung, A. T., Li, W. H. C., Ho, L. L. K., Ho, K. Y., Chan, G. C. F., & Chung, J. O. K. (2021). Physical activity for pediatric cancer survivors: A systematic review of randomized controlled trials. Journal of Cancer Survivorship, 15(6), 876–889. https://doi.org/10.1007/s11764-020-00981-w
- Cohen, J. (1988). Statistical power analysis for behavioral sciences. Routledge Academic.
- Conklin, H. M., Ashford, J. M., Clark, K. N., Martin-Elbahesh, K., Hardy, K. K., Merchant, T. E., Ogg, R. J., Jeha, S., Huang, L., & Zhang, H. (2017). Long-term efficacy of computerized cognitive training among survivors of childhood cancer: A single-blind randomized controlled trial. Journal of Pediatric Psychology, 42(2), 220–231. https://doi.org/10.1093/jpepsy/jsw057
- Conklin, H. M., Ogg, R. J., Ashford, J. M., Scoggins, M. A., Zou, P., Clark, K. N., Martin-Elbahesh, K., Hardy, K. K., Merchant, T. E., Jeha, S., Huang, L., & Zhang, H. (2015). Computerized cognitive training for amelioration of cognitive late effects among childhood cancer survivors: A randomized controlled trial. Journal of Clinical Oncology, 33(33), 3894–3902. https://doi.org/10.1200/JCO.2015.61.6672
- Delis, D. C., Kaplan, E., & Kramer, J. H. (2001). Delis-Kaplan executive function system. Pearson Psychological Corporation.
- Gathercole, S. E., Pickering, S. J., Ambridge, B., & Wearing, H. (2004). The structure of working memory from 4 to 15 years of age. Developmental Psychology, 40(2), 177–190. https://doi.org/10.1037/0012-1649.40.2.177
- Gogtay, N., Giedd, J. N., Lusk, L., Hayashi, K. M., Greenstein, D., Vaituzis, A. C., Nugent, T. F., Herman, D. H., Clasen, L. S., Toga, A. W., Rapoport, J. L., & Thompson, P. M. (2004). Dynamic mapping of human cortical development during childhood through early adulthood. Proceedings of the National Academy of Sciences, 101(21), 8174–8179. https://doi.org/10.1073/pnas.0402680101
- Hardy, K. K., Willard, V. W., Allen, T. M., & Bonner, M. J. (2013). Working memory training in survivors of pediatric cancer: A randomized pilot study. Psycho-Oncology, 22(8), 1856–1865. https://doi.org/10.1002/pon.3222
- Hearps, S., Seal, M., Anderson, V., McCarthy, M., Connellan, M., Downie, P., & De Luca, C. (2017). The relationship between cognitive and neuroimaging outcomes in children treated for acute lymphoblastic leukemia with chemotherapy only: A systematic review. Pediatric Blood & Cancer, 64(2), 225–233. https://doi.org/10.1002/pbc.26188
- Hötting, K., & Röder, B. (2013). Beneficial effects of physical exercise on neuroplasticity and cognition. Neuroscience & Biobehavioral Reviews, 37(9), 2243–2257. https://doi.org/10.1016/j.neubiorev.2013.04.005
- Huang, J. S., Dillon, L., Terrones, L., Schubert, L., Roberts, W., Finklestein, J., Swartz, M. C., Norman, G. J., & Patrick, K. (2014). Fit4Life: A weight loss intervention for children who have survived childhood leukemia. Pediatric Blood & Cancer, 61(5), 894–900. https://doi.org/10.1002/pbc.24937
- Ikonomidou, C. (2018). Chemotherapy and the pediatric brain. Molecular and Cellular Pediatrics, 5(1), 8. https://doi.org/10.1186/s40348-018-0087-0.
- Kassai, R., Futo, J., Demetrovics, Z., & Takacs, Z. K. (2019). A meta-analysis of the experimental evidence on the near- and far-transfer effects among children’s executive function skills. Psychological Bulletin, 145(2), 165. doi:10.1037/bul0000180
- Katz, B., Jones, M. R., Shah, P., Buschkuehl, M., & Jaeggi, S. M. (2021). Individual differences in cognitive training research. In T. Strobach & J. Karbach (Eds.), Cognitive training: An overview of features and applications (pp. 107–123). Springer. https://doi.org/10.1007/978-3-030-39292-5_8
- Kelly, C. E., Harding, R., Lee, K. J., Pascoe, L., Josev, E. K., Spencer-Smith, M. M., Adamson, C., Beare, R., Nosarti, C., Roberts, G., Doyle, L. W., Seal, M. L., Thompson, D. K., & Anderson, P. J. (2021). Investigating the brain structural connectome following working memory training in children born extremely preterm or extremely low birth weight. Journal of Neuroscience Research, 99(10), 2340–2350. https://doi.org/10.1002/jnr.24818
- Kelly, C. E., Thompson, D. K., Chen, J., Josev, E. K., Pascoe, L., Spencer-Smith, M. M., Adamson, C., Nosarti, C., Gathercole, S., Roberts, G., Lee, K. J., Doyle, L. W., Seal, M. L., & Anderson, P. J. (2020). Working memory training and brain structure and function in extremely preterm or extremely low birth weight children. Human Brain Mapping, 41(3), 684–696. https://doi.org/10.1002/hbm.24832
- Kesler, S. R., Gugel, M., Huston-Warren, E., & Watson, C. (2016). Atypical structural connectome organization and cognitive impairment in young survivors of acute lymphoblastic leukemia. Brain Connectivity, 6(4), 273–282. https://doi.org/10.1089/brain.2015.0409
- Kesler, S. R., Gugel, M., Pritchard-Berman, M., Lee, C., Kutner, E., Hosseini, S. M. H., Dahl, G., & Lacayo, N. (2014). Altered resting state functional connectivity in young survivors of acute lymphoblastic leukemia. Pediatric Blood & Cancer, 61(7), 1295–1299. doi:10.1002/pbc.25022
- Kesler, S. R., Sleurs, C., McDonald, B. C., Deprez, S., van der Plas, E., & Nieman, B. J. (2021). Brain imaging in pediatric cancer survivors: Correlates of cognitive impairment. Journal of Clinical Oncology, 39(16), 1775–1785. https://doi.org/10.1200/JCO.20.02315
- Klingberg, T., Fernell, E., Olesen, P. J., Johnson, M., Gustafsson, P., Dahlström, K., Gillberg, C. G., Forssberg, H., & Westerberg, H. (2005). Computerized training of working memory in children with ADHD – A randomized, controlled trial. Journal of the American Academy of Child & Adolescent Psychiatry, 44(2), 177–186. https://doi.org/10.1097/00004583-200502000-00010
- Koevoets, E. W., Petr, J., Monninkhof, E. M., Geerlings, M. I., Witlox, L., van der Wall, E., Stuiver, M. M., Sonke, G. S., Velthuis, M. J., Jobsen, J. J., van der Palen, J., Mutsaerts, H. J. M. M., de Ruiter, M. B., May, A. M., & Schagen, S. B. (2023). Effect of physical exercise on MRI-assessed brain perfusion in chemotherapy-treated breast cancer patients: A randomized controlled trial. Journal of Magnetic Resonance Imaging 59, 1667–1680. https://doi.org/10.1002/jmri.28967
- Krull, K. R., Hardy, K. K., Kahalley, L. S., Schuitema, I., & Kesler, S. R. (2018). Neurocognitive outcomes and interventions in long-term survivors of childhood cancer. Journal of Clinical Oncology, 36(21), 2181–2189. https://doi.org/10.1200/JCO.2017.76.4696
- Kunin-Batson, A., Kadan-Lottick, N., & Neglia, J. P. (2014). The contribution of neurocognitive functioning to quality of life after childhood acute lymphoblastic leukemia. Psycho-Oncology, 23(6), 692–699. https://doi.org/10.1002/pon.3470
- Li, H. C. W., Chung, O. K. J., Ho, K. Y., Chiu, S. Y., & Lopez, V. (2013). Effectiveness of an integrated adventure-based training and health education program in promoting regular physical activity among childhood cancer survivors. Psycho-Oncology, 22(11), 2601–2610. https://doi.org/10.1002/pon.3326
- Liu, F., Duan, Y., Peterson, B. S., Asllani, I., Zelaya, F., Lythgoe, D., & Kangarlu, A. (2018). Resting state cerebral blood flow with arterial spin labeling MRI in developing human brains. European Journal of Paediatric Neurology, 22(4), 642–651. https://doi.org/10.1016/j.ejpn.2018.03.003
- MacIntosh, B. J., Shirzadi, Z., Scavone, A., Metcalfe, A. W., Islam, A. H., Korczak, D., & Goldstein, B. I. (2017). Increased cerebral blood flow among adolescents with bipolar disorder at rest is reduced following acute aerobic exercise. Journal of Affective Disorders, 208(October 2016), 205–213. https://doi.org/10.1016/j.jad.2016.08.060
- Melby-Lervåg, M., & Hulme, C. (2013). Is working memory training effective? A meta-analytic review. Developmental Psychology, 49(2), 270–291. https://doi.org/10.1037/a0028228
- Melby-Lervåg, M., Redick, T. S., & Hulme, C. (2016). Working memory training does not improve performance on measures of intelligence or other measures of “far transfer”: Evidence from a meta-analytic review. Perspectives on Psychological Science, 11(4), 512–534. https://doi.org/10.1177/1745691616635612
- Melchers, P., & Preuß, U. (1991). Kaufman assessment battery for children (German version). Swets and Zeilinger.
- Nishijima, T., Torres-Aleman, I., & Soya, H. (2016). Exercise and cerebrovascular plasticity. In Progress in Brain Research (pp. 243–268). Elsevier. https://doi.org/10.1016/bs.pbr.2016.03.010
- Northey, J. M., Pumpa, K. L., Quinlan, C., Ikin, A., Toohey, K., Smee, D. J., & Rattray, B. (2019). Cognition in breast cancer survivors: A pilot study of interval and continuous exercise. Journal of Science and Medicine in Sport, 22(5), 580–585. https://doi.org/10.1016/j.jsams.2018.11.026
- Pesce, C. (2012). Shifting the focus from quantitative to qualitative exercise characteristics in exercise and cognition research. Journal of Sport and Exercise Psychology, 34(6), 766–786. https://doi.org/10.1123/jsep.34.6.766
- Pesce, C., Vazou, S., Benzing, V., Álvarez-Bueno, C., Anzeneder, S., Mavilidi, M. F., Leone, L., & Schmidt, M. (2021). Effects of chronic physical activity on cognition across the lifespan: A systematic meta-review of randomized controlled trials and realist synthesis of contextualized mechanisms. International Review of Sport and Exercise Psychology, 16(1), 722–760. https://doi.org/10.1080/1750984X.2021.1929404
- Pickering, S. J., & Gathercole, S. E. (2001). The working memory test battery for children. SanAntonio (CA): Psychological Corporation.
- Quinn, D. K., Upston, J., Jones, T., Brandt, E., Story-Remer, J., Fratzke, V., Wilson, J. K., Rieger, R., Hunter, M. A., Gill, D., Richardson, J. D., Campbell, R., Clark, V. P., Yeo, R. A., Shuttleworth, C. W., & Mayer, A. R. (2020). Cerebral perfusion effects of cognitive training and transcranial direct current stimulation in mild-moderate TBI. Frontiers in Neurology, 11(October), 1–11. https://doi.org/10.3389/fneur.2020.545174
- Rapti, C., Dinas, P. C., Chryssanthopoulos, C., Mila, A., & Philippou, A. (2023). Effects of exercise and physical activity levels on childhood cancer: An umbrella review. Healthcare, 11(6), 1–31. https://doi.org/10.3390/healthcare11060820
- Riggs, L., Piscione, J., Laughlin, S., Cunningham, T., Timmons, B. W., Courneya, K. S., Bartels, U., Skocic, J., De Medeiros, C., Liu, F., Persadie, N., Scheinemann, K., Scantlebury, N., Szulc, K. U., Bouffet, E., & Mabbott, D. J. (2017). Exercise training for neural recovery in a restricted sample of pediatric brain tumor survivors: A controlled clinical trial with crossover of training versus no training. Neuro-Oncology, 19(3), 440–450. https://doi.org/10.1093/neuonc/now177
- Ritter, N., Kilinc, E., Navruz, B., & Bae, Y. (2011). Test review: L. Brown, R. J. Sherbenou, & S. K. Johnsen Test of nonverbal intelligence-4 (TONI-4). Austin, TX: PRO-ED, 2010. Journal of Psychoeducational Assessment, 29(5), 484–488. https://doi.org/10.1177/0734282911400400
- Robinson, K. E., Livesay, K. L., Campbell, L. K., Scaduto, M., Cannistraci, C. J., Anderson, A. W., Whitlock, J. A., & Compas, B. E. (2010). Working memory in survivors of childhood acute lymphocytic leukemia: Functional neuroimaging analyses. Pediatric Blood & Cancer, 54(4), 585–590. doi:10.1002/pbc.22362
- Ruble, K., Scarvalone, S., Gallicchio, L., Davis, C., & Wells, D. (2016). Group physical activity intervention for childhood cancer survivors: A pilot study. Journal of Physical Activity and Health, 13(3), 352–359. doi:10.1123/jpah.2015-0050
- Sabel, M., Sjölund, A., Broeren, J., Arvidsson, D., Saury, J. M., Gillenstrand, J., Emanuelson, I., Blomgren, K., & Lannering, B. (2017). Effects of physically active video gaming on cognition and activities of daily living in childhood brain tumor survivors: A randomized pilot study. Neuro-Oncology Practice, 4(2), 98–110. https://doi.org/10.1093/nop/npw020
- Sala, G., Deniz Aksayli, N., Semir Tatlidil, K., Tatsumi, T., Gondo, Y., & Gobet, F. (2019). Near and far transfer in cognitive training: A second-order meta-analysis. Collabra: Psychology, 5(1), 1–22. https://doi.org/10.1525/collabra.203
- Sala, G., & Gobet, F. (2020). Working memory training in typically developing children: A multilevel meta-analysis. Psychonomic Bulletin & Review, 27(3), 423–434. https://doi.org/10.3758/s13423-019-01681-y
- Schiller, R. M., IJsselstijn, H., Madderom, M. J., van Rosmalen, J., van Heijst, A. F. J., Smits, M., Verhulst, F., Tibboel, D., & White, T. (2019). Training-induced white matter microstructure changes in survivors of neonatal critical illness: A randomized controlled trial. Developmental Cognitive Neuroscience, 38(July 2018), 100678. https://doi.org/10.1016/j.dcn.2019.100678
- Schilling, K. G., Yeh, F.-C., Nath, V., Hansen, C., Williams, O., Resnick, S., Anderson, A. W., & Landman, B. A. (2019). A fiber coherence index for quality control of B-table orientation in diffusion MRI scans. Magnetic Resonance Imaging, 58, 82–89. doi:10.1016/j.mri.2019.01.018
- Schmidt, M., Jäger, K., Egger, F., Roebers, C. M., & Conzelmann, A. (2015). Cognitively engaging chronic physical activity, but not aerobic exercise, affects executive functions in primary school children: A group-randomized controlled trial. Journal of Sport and Exercise Psychology, 37(6), 575–591. https://doi.org/10.1123/jsep.2015-0069
- Schneiders, J. A., Opitz, B., Tang, H., Deng, Y., Xie, C., Li, H., & Mecklinger, A. (2012). The impact of auditory working memory training on the fronto-parietal working memory network. Frontiers in Human Neuroscience, 6(JUNE 2012), 1–14. https://doi.org/10.3389/fnhum.2012.00173
- Scholz, J., Klein, M. C., Behrens, T. E. J., & Johansen-Berg, H. (2009). Training induces changes in white-matter architecture. Nature Neuroscience, 12(11), 1370–1371. https://doi.org/10.1038/nn.2412
- Schuerch, K., Grieder, M., Benzing, V., Siegwart, V., Federspiel, A., Slavova, N., Kiefer, C., & Roessler, J. (2023). How is cerebral perfusion associated with functional outcome in pediatric cancer survivors? Developmental Neuropsychology, 48(4), 186–202. https://doi.org/10.1080/87565641.2023.2215360
- Sharma, B., Allison, D., Tucker, P., Mabbott, D., & Timmons, B. W. (2021). Exercise trials in pediatric brain tumor: A systematic review of randomized studies. Journal of Pediatric Hematology/Oncology, 43(2), 59–67. https://doi.org/10.1097/MPH.0000000000001844
- Sharma, N., & Cohen, L. G. (2012). Recovery of motor function after stroke. Developmental Psychobiology, 54(3), 254–262. https://doi.org/10.1002/dev.20508
- Shipstead, Z., Redick, T. S., & Engle, R. W. (2012). Is working memory training effective? Psychological Bulletin, 138(4), 628–654. https://doi.org/10.1037/a0027473
- Siegwart, V., Benzing, V., Spitzhuettl, J., Schmidt, M., Grotzer, M., Steinlin, M., Leibundgut, K., Roebers, C., & Everts, R. (2020). Cognition, psychosocial functioning, and health-related quality of life among childhood cancer survivors. Neuropsychological Rehabilitation, 922–945. https://doi.org/10.1080/09602011.2020.1844243
- Siegwart, V., Steiner, L., Pastore-Wapp, M., Benzing, V., Spitzhuttl, J., Schmidt, M., Kiefer, C., Slavova, N., Grotzer, M., Roebers, C., Steinlin, M., Leibundgut, K., & Everts, R. (2021). The working memory network and its association with working memory performance in survivors of non-CNS childhood cancer. Developmental Neuropsychology, 46(3), 249–264. https://doi.org/10.1080/87565641.2021.1922410
- Singh, A. S., Saliasi, E., Van Den Berg, V., Uijtdewilligen, L., De Groot, R. H. M., Jolles, J., Andersen, L. B., Bailey, R., Chang, Y. K., Diamond, A., Ericsson, I., Etnier, J. L., Fedewa, A. L., Hillman, C. H., McMorris, T., Pesce, C., Pühse, U., Tomporowski, P. D., & Chinapaw, M. J. M. (2019). Effects of physical activity interventions on cognitive and academic performance in children and adolescents: A novel combination of a systematic review and recommendations from an expert panel. British Journal of Sports Medicine, 53(10), 640–647. https://doi.org/10.1136/bjsports-2017-098136
- Sleurs, C., Deprez, S., Emsell, L., Lemiere, J., & Uyttebroeck, A. (2016). Chemotherapy-induced neurotoxicity in pediatric solid non-CNS tumor patients: An update on current state of research and recommended future directions. Critical Reviews in Oncology/Hematology, 103, 37–48. https://doi.org/10.1016/j.critrevonc.2016.05.001
- Sleurs, C., Lemiere, J., Radwan, A., Verly, M., Elens, I., Renard, M., Jacobs, S., Sunaert, S., Deprez, S., & Uyttebroeck, A. (2019). Long-term leukoencephalopathy and neurocognitive functioning in childhood sarcoma patients treated with high-dose intravenous chemotherapy. Pediatric Blood & Cancer, 66(10), 1–12. https://doi.org/10.1002/pbc.27893
- Spiegler, B. J., Bouffet, E., Greenberg, M. L., Rutka, J. T., & Mabbott, D. J. (2004). Change in neurocognitive functioning after treatment with cranial radiation in childhood. Journal of Clinical Oncology, 22(4), 706–713. https://doi.org/10.1200/JCO.2004.05.186
- Spitzhüttl, J. S., Kronbichler, M., Kronbichler, L., Benzing, V., Siegwart, V., Pastore-Wapp, M., Kiefer, C., Slavova, N., Grotzer, M., Roebers, C. M., Steinlin, M., Leibundgut, K., & Everts, R. (2020). Impact of non-CNS childhood cancer on resting-state connectivity and its association with cognition. Brain and Behavior, 11(1), 1–16. https://doi.org/10.1002/brb3.1931
- Stanmore, E., Stubbs, B., Vancampfort, D., de Bruin, E. D., & Firth, J. (2017). The effect of active video games on cognitive functioning in clinical and non-clinical populations: A meta-analysis of randomized controlled trials. Neuroscience & Biobehavioral Reviews, 78(March), 34–43. https://doi.org/10.1016/j.neubiorev.2017.04.011
- Steiner, L., Homan, S., Everts, R., Federspiel, A., Kamal, S., Rodriguez, J. A. D., Kornfeld, S., Slavova, N., Wiest, R., & Kaelin-Lang, A. (2021). Functional connectivity and upper limb function in patients after pediatric arterial ischemic stroke with contralateral corticospinal tract wiring. Scientific Reports, 11(1), 1–11. doi:10.1038/s41598-020-79139-8
- Takeuchi, H., Sekiguchi, A., Taki, Y., Yokoyama, S., Yomogida, Y., Komuro, N., Yamanouchi, T., Suzuki, S., & Kawashima, R. (2010). Training of working memory impacts structural connectivity. The Journal of Neuroscience, 30(9), 3297–3303. https://doi.org/10.1523/JNEUROSCI.4611-09.2010
- Toda, N., Ayajiki, K., & Okamura, T. (2009). Cerebral blood flow regulation by nitric oxide: Recent advances. Pharmacological Reviews, 61(1), 62–97. https://doi.org/10.1124/pr.108.000547
- Tomporowski, P. D., & Pesce, C. (2019). Exercise, sports, and performance arts benefit cognition via a common process. Psychological Bulletin, 145(9), 929. doi:10.1037/bul0000200
- van der Plas, E., Schachar, R. J., Hitzler, J., Crosbie, J., Guger, S. L., Spiegler, B. J., Ito, S., & Nieman, B. J. (2017). Brain structure, working memory and response inhibition in childhood leukemia survivors. Brain and Behavior, 7(2), 1–16. https://doi.org/10.1002/brb3.621
- Van Waelvelde, H., Vanden Wyngaert, K., Mariën, T., Baeyens, D., & Calders, P. (2020). The relation between children’s aerobic fitness and executive functions: A systematic review. Infant and Child Development, 29(3), e2163. https://doi.org/10.1002/icd.2163
- Wang, J., & Licht, D. J. (2006). Pediatric perfusion MR imaging using arterial spin labeling. Neuroimaging Clinics of North America, 16(1), 149–167. https://doi.org/10.1016/j.nic.2005.10.002
- Wang, J., Licht, D. J., Jahng, G. H., Liu, C. S., Rubin, J. T., Haselgrove, J., Zimmerman, R. A., & Detre, J. A. (2003). Pediatric perfusion imaging using pulsed arterial spin labeling. Journal of Magnetic Resonance Imaging, 18(4), 404–413. https://doi.org/10.1002/jmri.10372
- Wechsler, D. (2003). WISC-4: Wechsler intelligence scale for children: Technical and interpretive manual. Pearson.
- Wickham, H. (2016). Data analysis. In Ggplot2 (pp. 189–201). Springer.
- Williams, A. L. M., & Cole, P. D. (2021). Biomarkers of cognitive impairment in pediatric cancer survivors. Journal of Clinical Oncology, 39(16), 1766–1774. https://doi.org/10.1200/JCO.20.02436
- Wurz, A., & Brunet, J. (2016). The effects of physical activity on health and quality of life in adolescent cancer survivors: A systematic review. JMIR Cancer, 2(1), e6. https://doi.org/10.2196/cancer.5431
- Xu, J., Kochanek, K. D., Murphy, S. L., & Tejada-Vera, B. (2016). Deaths: Final data for 2014. In National Center for Health Statistics (U.S.). Division of Vital Statistics (Ed.), National vital statistics reports (Vol. 65, Issue 4). https://stacks.cdc.gov/view/cdc/40133
- Yeh, C. H., Man Wai, J. P., Lin, U. S., & Chiang, Y. C. (2011). A pilot study to examine the feasibility and effects of a home-based aerobic program on reducing fatigue in children with acute lymphoblastic leukemia. Cancer Nursing, 34(1), 3–12. https://doi.org/10.1097/NCC.0b013e3181e4553c
- Yeh, F., Liu, L., Hitchens, T. K., & Wu, Y. L. (2017). Mapping immune cell infiltration using restricted diffusion MRI. Magnetic Resonance in Medicine, 77(2), 603–612. doi:10.1002/mrm.26143
- Yeh, F.-C., & Tseng, W.-Y. I. (2011). NTU-90: A high angular resolution brain atlas constructed by q-space diffeomorphic reconstruction. Neuroimage, 58(1), 91–99. doi:10.1016/j.neuroimage.2011.06.021
- Yeh, F.-C., Verstynen, T. D., Wang, Y., Fernández-Miranda, J. C., & Tseng, W.-Y. I. (2013). Deterministic diffusion fiber tracking improved by quantitative anisotropy. PLoS One, 8(11), e80713. doi:10.1371/journal.pone.0080713
- Yeh, F.-C., Wedeen, V. J., & Tseng, W.-Y. I. (2010). Generalized q-sampling imaging. IEEE Transactions on Medical Imaging, 29(9), 1626–1635. doi:10.1109/TMI.2010.2045126