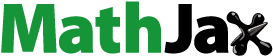
ABSTRACT
Many young people are potentially at risk of noise-induced hearing loss due to unsafe use of personal listening devices. The aim of this cross-sectional study was to examine the association of sociodemographic factors and risk behavior with unsafe use of personal listening devices in adolescents to identify a target group for prevention. A smartphone application was developed to objectively measure music listening habits among 314 adolescents with a mean age of 13 years and 7 months (SD ±5 months). Listening habits were characterized as safe or unsafe based on the weekly noise dose. Data on sociodemographic factors and traditional health risk behaviors were obtained by questionnaires. Within the study group, 10.5% of the participants exceeded the 50%, and 4.8% the 100% recommended weekly noise dose. Adolescents with a lower socioeconomic status were more likely to engage in unsafe listening habits as compared to adolescents with a higher socioeconomic status. Additionally, risk behavior was associated with higher odds of having unsafe listening habits as compared to no risk behavior. Age, sex and educational levels were not significantly associated with unsafe listening habits. The findings of the present study indicate that interventions to promote safe listening habits should target adolescents with a lower socioeconomic status and higher risk behavior. Future research is needed to investigate how these adolescents can be motivated to adopt safe listening habits.
Introduction
Today, up to 95% of the population in developed countries owns a smartphone, often with an integrated music player (Anderson and Jiang Citation2018). This development has raised concerns over its effect on hearing, especially among young people, who are more likely to engage in unhealthy listening behavior compared to other age groups (Fligor et al. Citation2014; Gilliver et al. Citation2017; Feder et al. Citation2019). Continued exposure to excessive noise is known to induce metabolic and mechanical changes causing cell death and physical loss of integrity of hair cells, which results in irreversible hearing loss (Le et al. Citation2017). As hearing loss is considered a substantial contributor to the global burden of disease, prevention is of great importance (Haile et al. Citation2021).
As not every young person who uses a personal listening device is at risk of hearing damage, it should be decided on to whom preventive interventions should be directed. Identifying determinants of unsafe listening habits may help to define target groups for prevention. Specific sociodemographic factors have been found to influence listening habits such as age, sex, educational level and socioeconomic status (Vogel et al. Citation2007; Portnuff Citation2016). For example, males are reported to be more likely to engage in unsafe listening compared to females (Torre Iii Citation2008; Dreher et al. Citation2018; Basu et al. Citation2019). With regard to the educational level, a lower educational level is significantly associated with unsafe listening habits among adolescents (Vogel et al. Citation2009; Dreher et al. Citation2018). An association between socioeconomic status and adolescents’ attitude and behavior towards loud sounds has previously been described by Widen and Erlandsson (Widén and Erlandsson Citation2004). The authors showed that adolescents with a higher socioeconomic status are more concerned about the risk of hearing loss due to excessive noise and use hearing protection more often compared to their peers with a lower socioeconomic status.
In addition to these sociodemographic factors, several studies reported a correlation between self-reported unsafe listening habits and more traditional risk behaviors including smoking, alcohol consumption, cannabis use and unprotected sexual intercourse (Bohlin and Erlandsson Citation2007; Vogel et al. Citation2012; Parsons et al. Citation2019). Adolescence is a period of transition between childhood and adulthood, and compared to other life periods characterized by many physical and psychological changes, among others an increase in risk behavior (Steinberg Citation2008). This includes adolescent behavior towards noise exposure but also other health risk behaviors, which may explain the correlation found.
At present, the measurement of music listening habits remains a challenge for researchers, with many of the available methodologies limited by a lack of validity, such as the use of self-reports (Kaplan-Neeman et al. Citation2017; Paping et al. Citation2021). Self-reports are convenient and low costs, but sensitive to recall bias. Moreover, self-reports measuring listening habits at a single point in time do not take into account that listening habits are variable. In search of ways to objectively monitor noise exposure from smartphones, we developed a smartphone application that was able to collect data on listening habits in a large population-based cohort of adolescents. The aim of the present study was to examine the association of sociodemographic factors and risk behavior with objectively measured unsafe music listening habits among adolescents using personal listening devices to identify target groups for prevention. We hypothesized that both sociodemographic factors and risk behavior have an impact on listening habits, and could help in identifying high-risk groups.
Methods
Study design and population
sectional study was embedded in the Generation R study, a population-based prospective birth cohort study in Rotterdam, the Netherlands. The design and population have been described previously (Kooijman et al. Citation2016). The study was approved by the Medical Ethical Committee at Erasmus Medical Center. Written informed consent was obtained from all participants and their parents. Adolescents aged 12–17 years who visited the research center at the Erasmus Medical Center between May 2017 and September 2019 were invited to participate in this sub-study. To be eligible to participate, adolescents needed to have a smartphone. The first version of the smartphone application was designed for smartphones running the Android operation only. The updated version, available from April 2019 onwards, was able to run on iOS smartphones as well. Of the 3,237 adolescents who visited the research center, 978 (30.2%) had a smartphone and agreed to participate in this sub study. Of these participants, 484 (49.5%) installed and started the application. We excluded participants with a OnePlus smartphone as listening levels were incorrectly converted (n = 2), participants whose listening habits were monitored less than 7 days as this monitoring was considered too short (n = 65), or more than 40 days as participants automatically received an overview of their listening habits at the end of the monitoring period that may have influenced their listening habits thereafter (n=4). Last, participants who did not listen to music or a video with headphones at least once while the application was active were excluded as we cannot rule out that the application was set to be inactive (n = 99) ().
Smartphone application
The application was overseen by the departments of Communication, Security, Legal Affairs and the Data Protection of the Erasmus Medical Center. Several rounds of technical development and field testing were conducted to confirm the accuracy and reliability of the application before the application was implemented within the Generation R Study. The application was specifically aimed to record listening habits, that is, the frequency, duration and listening level. The application was available for free through Google Play and Apple store. Minimal storage capacity was required, and the power consumption was low. Participants were able to use their own ear- or headphones. A unique username and password were required to login, provided during participants’ visit at the research center. The application ran constantly in the background to collect data on listening habits. The specific methodology on how listening habits were recorded has been described previously (Paping et al. Citation2021). Although the assessment duration was intended to be 35 days, participants could delete the application at any time. The data recorded by the application were transferred to a secured online server every time the smartphone was connected to Wi-Fi.
Weekly noise dose calculations
Consistent with previous studies, weekly noise doses were calculated on the basis of occupational safety standards. The National Institute for Occupational Safety and Health (NIOSH) defines a time-weighted average of 85 dBA for an 8-hour period as the maximum recommended noise dose per day (National Institute for Occupational Safety and Health Citation1998). For every 3 dB increase in noise level, the allowable exposure time is reduced by half. Exposures from individual activities in a given day are added together to calculate the daily noise dose. The maximum recommended weekly noise dose is attained when the daily noise dose is exceeded 5 out of 7 days.
The data collected by the application were used to calculate participants’ average weekly noise dose in several stages. First, we converted the listening levels into estimated output levels in dBA using the regression equation of Williams et al. (Williams et al. Citation2010), presented by:
Hereafter, daily noise doses were calculated, using the following formula:
D= daily noise dose (in percentages)
Cn= total time of exposure at a specified noise level (in minutes)
Tn= exposure time at which noise for this specified noise level becomes hazardous (in minutes)
The exposure time at which noise for a specified noise level becomes hazardous was calculated by:
Tn = exposure time at which noise for this level becomes hazardous (in minutes)
L= exposure level (in dBA)
Last, the weekly noise doses were calculated by multiplying the denominators of the equation of the daily noise dose by 5, and summing in this equation over a week. As some of the listeners in the present study did not listen for an inter number of weeks, the average weekly noise dose was calculated by summing over the entire participation period and dividing by the number of weeks in which they participated.
Unsafe listening habits
Two definitions of unsafe listening habits were used, namely an average weekly noise dose >50%, assuming that adolescents are exposed also to other sources of recreational noise exposure, and an average weekly noise dose >100%.
Covariates
Sociodemographic factors
Data on sociodemographic characteristics (age, sex, educational level and socioeconomic status) were obtained through parental questionnaires at the age of 13 years. The educational level of the participant was defined as lower (primary education only or preparatory secondary vocational education), intermediate (senior general secondary education), or higher (university preparatory education) (Statistics Netherlands Citation2021). Household income was used as marker for socioeconomic status, and was classified as below the national average (<€2800), or equal to or above the national average (≥€2800).
Risk behavior
Participants were asked to complete a computerized questionnaire on risk behavior during their visit to the research center. The risk-taking questionnaire included questions on any substance use, specifically tobacco, alcohol, and cannabis, as well as sexual activity. Smoking on average more than one cigarette a month, an episode of being drunk, drinking more than 3 glasses of alcohol on one occasion, smoking cannabis and having unsafe sexual intercourse in the past year were considered risk behaviors. Participants were categorized in two groups: not engaging in risk behavior and engaging in any of the risk behavior. To reduce the risk of social desirability bias, participants completed the questionnaire in a private setting and were able to skip questions if they did not want to share this sensitive information.
Statistical analyses
Descriptive statistics were used to evaluate the characteristics of the study population and listening habits. Continuous data were described as mean (standard deviation (SD)) when normally distributed, or median (interquartile range (IQR)) when not normally distributed. Between-group differences were analyzed using χ2 statistics and independent t-tests. To examine whether non-response was selective, we compared the baseline characteristics and listening habits of adolescents included and excluded from the analyses. Multivariable logistic regression models were used to examine the association of the sociodemographic factor age, sex, educational level, socioeconomic status and risk behavior with unsafe listening habits. The correlation between the variables was assessed, to ensure multicollinearity was not present. Odds ratios (ORs) with 95% confidence intervals (CIs) were calculated. Missing data in covariates were imputed using the multiple imputation method using chained equations to select the most likely value for a missing response. Ten imputed datasets were generated. As no major differences in the magnitude or direction of the effect estimates were observed between analyses with imputed missing data and complete cases only, we only present the pooled results based on imputed datasets. Two-tailed tests were used for all analyses with statistical significance accepted at p < .05. Statistical analyses were performed using SPSS version 24.0 for Windows software (SPSS Inc).
Results
A total of 314 participants with a mean age of 13 years and 7 months (SD ±5 months) were included, of whom 51.6% were males. gives an overview of the characteristics of the total study population.
Table 1. Characteristics of the included study population.
Non-response analyses
Non-response analyses showed that, as compared to adolescents excluded from the analyses (N = 2,923), those included were on average younger (13 years and 7 months vs. 13 years and 8 months, t= −3.1, p = .002), had a higher net household income (61.8 vs. 56.4%, χ2 = 8.1, p = .017), and attended a higher educational level (51.0 vs. 44.2%, χ2 = 10.4, p = .015) (Table S1). However, the proportion of missing data on household income and educational level was higher among the excluded participants (household income: 22.6% vs. 15.6%; educational level: 19.4% vs. 12.4%)
In a sensitivity analysis, we compared the self-reported listening habits of participants included and excluded in the analysis. There was no significant difference in self-reported frequency of listening, listening time and listening level (Table S2).
Listening habits measured by the smartphone application
Listening habits were monitored over a median of 33 days (IQR, 22.0–35.0). A total of 33 (10.5%) participants had a daily noise dose >50%, and 15 (4.8%) >100%. The demographics of the participants engaging in unsafe listening habits are presented in Table S3.
Factors associated with unsafe listening habits
presents the results of the multivariable logistic regression analyses. Household income and risk behavior were significantly associated with unsafe listening habits. Specifically, adolescents with a household income below the national average were more likely to engage in unsafe listening habits compared to adolescents with a household income equal to or above the national average (noise dose >50%: OR 2.6, 95% CI 1.1; 6.1 and noise dose >100%: OR 5.0, 95% CI 1.3; 18.9). Moreover, engaging in risk behavior increased the odds of having unsafe listening habits (noise dose >50%: OR 3.6, 95% CI 1.6; 8.3 and noise dose >100%: OR 3.7 (1.1; 12.0)). Participants’ age, sex and educational level were not significantly associated with unsafe listening habits.
Table 2. Multivariable logistic regression for the association of sociodemographic factors and risk behavior with unsafe listening habits (n = 314)..
Discussion
As noise-induced hearing loss is expected to inflict a widespread lifelong and disability in modern societies, prevention is of great importance. In the present study, 4.8% of the adolescents using personal listening devices were found to exceed the weekly noise dose when using a personal listening device. Socioeconomic status and risk behavior were significant association with unsafe listening habits. The current study expands upon previous literature by including objective data of listening habits.
The majority of adolescents using personal listening devices in the present study exhibited listening habits that could be considered as safe. Prior research using self-reports to measure listening habits reported a large variation in number of people exceeding recommended exposure limits, which to some extent may be due to differences in study population and definitions applied as described in the systematic review of Jiang et al. (Jiang et al. Citation2016). An important limitation of self-reports is that they do not account for individual variations in listening habits. To our knowledge, to date, only two studies have reported the weekly noise dose based on objective measurements of listening habits (Portnuff et al. Citation2013; Kaplan-Neeman et al. Citation2017). In the study of Portnuff et al., an external dosimeter was used to measure listening habits (Portnuff et al. Citation2013). In total, 16.7% of the 24 participants aged 19–29 years were reported to exceed the recommended weekly noise dose, which is higher than the 4.8% found in our study. This can possibly be explained by the fact that participants were required to report at least 10 hours of personal listening device use a week in the study of Portnuff et al. In the paper of Kaplan-Neeman et al., 2.7% of the 40 participants aged 18–32 years were found to exceed the recommended weekly noise dose (Kaplan-Neeman et al. Citation2017). Although a smartphone application was used to measure listening habits, participants received a loaner smartphone instead of using their own, which may have influenced the results. It is important to keep in mind that in present study adolescents who did not use their personal listening device at least once during the monitoring period were excluded. Therefore, it is likely that the proportion of adolescents engaging in unsafe listening habits is lower in the general population.
For the purpose of targeted prevention, it is important to identify which adolescents exhibit unsafe listening habits. In line with the study of Widen and Erlandsson, we observed that adolescents with a lower socioeconomic status were more likely to engage in unsafe listening habits as compared to adolescents with a higher socioeconomic status (Widén and Erlandsson Citation2004). This can possibly be explained by differences in knowledge and risk perception. Besides being aware of the negative consequences of excessive noise on hearing, perceived individual susceptibility is of importance (Diviani et al. Citation2019). Several studies have found that students who engage in unsafe listening habits experience low personal vulnerability to noise-induced hearing loss (Vogel et al. Citation2008; Rawool and Colligon-Wayne Citation2008). Another explanation is that adolescents with a lower socioeconomic status report less parental involvement, which has been recognized as an important factor in reducing health risk behaviors (Hindelang et al. Citation2001; DiClemente et al. Citation2001).
In the present study, risk behavior was significantly associated with higher odds of having unsafe listening habits. It is known that music, like substance use, can improve mood and distract people from unpleasant feelings or thoughts (Kemper and Danhauer Citation2005). Interestingly, several studies have demonstrated that the areas of the brain activated by music are similar to those that respond to other euphoria-inducing stimuli such as drugs and sex (Blood and Zatorre Citation2001; Menon and Levitin Citation2005; Bourdaghs and Silverman Citation2020). Traditionally, prevention programs have focussed on a single risk behavior. However, as risk behaviors share a common set of risk and protective factors, it should be possible to design a prevention program targeting multiple-health risk behavior simultaneously (Bailey Citation2009; Jackson et al. Citation2012). By identifying common pathways to risk behavior, multiple health problems might be mitigated.
Over the past decade, many campaigns have been developed to address the potential risk of exposure to loud music, attempting to motivate young people to adopt safe listening behaviors. However, the effectivity of these campaigns is being questioned (Weichbold and Zorowka Citation2007). Widely addressed campaigns might go unnoticed by the target population. The findings of the present study indicate that the largest public health gains are likely to be achieved through prevention strategies targeted at adolescents with a lower socioeconomic status and those engaging in risk behavior. Future research is needed to investigate how these adolescents can be motivated to adopt safe listening habits.
Strength and limitations
A strength of the present study is the objective measurement of listening habits using a smartphone application. The application was easy to use and required minimal storage capacity and battery. In contrast to previous studies measuring listening habits objectively, the participants in this study had the advantage that they did not need to carry an external or foreign device with them, which potentially reduces the validity of the data (Portnuff et al. Citation2013; Kaplan-Neeman et al. Citation2017). Furthermore, listening habits were monitored for a longer period compared to, which is of value since listening habits may vary over time. A limitation is that the application could only be installed on a single smartphone. If participants used multiple personal listening devices, the weekly noise dose is an underestimation of their true value. To calculate participants’ weekly noise dose, the listening levels registered by the smartphone were converted into estimated output levels using the regression equation of Williams et al., published in 2010 (Williams et al. Citation2010). According to the literature, output levels vary depending on the type of headphones used and style of music (Breinbauer et al. Citation2012; Kim and Han Citation2018; Shim et al. Citation2018). We did not take these factors into account, which could lead to an under- or overestimation of participants' true daily noise dose. Although we expect this possible bias to be non-differential, in an ideal situation we would have measured the output levels produced in the ears during listening for every participant individually.
To date, there is no universally accepted standard for safe listening. In line with previous research, the weekly noise dose was calculated on the basis of occupational safety standards. A weekly noise dose >100% was considered as unsafe. Moreover, a lower cutoff was chosen as we believe that other sources of recreational noise exposure should be taken into account when calculating the weekly noise dose. Analyses with both definitions yielded different results for the number of adolescents exhibiting unsafe listening habits as expected but did not change the association of risk behavior with unsafe listening habits. As the number of participants exceeding the 100% weekly noise dose was relatively low, these results should be interpreted with caution. Although we reached the target sample size in the present study, the participation rate was lower than expected. There was a selection towards a relatively Western and highly educated population, which may affect the generalizability of our findings. A lack of consent and not having a smartphone were the main reasons for exclusion. The lower response rate due to no consent may be caused by the limited time at the research center allocated to inform about the application.
Conclusions
The majority of adolescents using personal listening devices in the present study exhibited listening habits that could be considered as safe. Preventive interventions should target adolescents with a lower socioeconomic status and higher risk behavior, as these groups were found to be more likely to engage in unsafe listening habits. Future research is needed to investigate how these groups of adolescents can be motivated to adopt safe listening habits. Smartphone applications can potentially be used as platform to deliver customized and targeted messages encouraging safe listening habits.
Supplemental Material
Download MS Word (23.3 KB)Acknowledgement
The Generation R Study is conducted by the Erasmus Medical Center in close collaboration with Faculty of Social Sciences of the Erasmus University Rotterdam, the Municipal Health Service Rotterdam area, Rotterdam, and the Stichting Trombosedienst & Artsenlaboratorium Rijnmond (STAR-MDC), Rotterdam. We gratefully acknowledge the contribution of children and parents, general practitioners, hospitals, midwives and pharmacies in Rotterdam. We would like to thank Jasper Weeteling and Innovattic, the developers of the smartphone applications, and Teun van Immerzeel for his contribution to the data analyses.
Disclosure statement
No potential conflict of interest was reported by the author(s).
Supplementary material
Supplemental data for this article can be accessed here.
Additional information
Funding
References
- Anderson M, Jiang J. 2018. Teens, social media & technology 2018. Pew Res Cent. 31(2018):1673–1689.
- Bailey JA. 2009. Addressing common risk and protective factors can prevent a wide range of adolescent risk behaviors. J Adolesc Health. 45(2):107–108. doi:10.1016/j.jadohealth.2009.05.007.
- Basu S, Garg S, Singh MM, Kohli C. 2019. Knowledge and practices related to the use of personal audio devices and associated health risks among medical students in Delhi. J Educ Health Promot. 8. doi:10.4103/jehp.jehp_308_18.
- Blood AJ, Zatorre RJ. 2001. Intensely pleasurable responses to music correlate with activity in brain regions implicated in reward and emotion. Proc Natl Acad Sci. 98(20):11818–11823. doi:10.1073/pnas.191355898.
- Bohlin MC, Erlandsson SI. 2007. Risk behaviour and noise exposure among adolescents. Noise Health. 9(36):55. doi:10.4103/1463-1741.36981.
- Bourdaghs S, Silverman MJ. 2020. A neurological rationale for music therapy to address social connectivity among individuals with substance use disorders. Arts Psychother. 70:101681. doi:10.1016/j.aip.2020.101681.
- Breinbauer HA, Anabalón JL, Gutierrez D, Cárcamo R, Olivares C, Caro J. 2012. Output capabilities of personal music players and assessment of preferred listening levels of test subjects: outlining recommendations for preventing music‐induced hearing loss. The Laryngoscope. 122(11):2549–2556. doi:10.1002/lary.23596.
- DiClemente RJ, Wingood GM, Crosby R, Sionean C, Cobb BK, Harrington K, Davies S, Hook EW, Oh MK. 2001. Parental monitoring: association with adolescents’ risk behaviors. Pediatrics. 107(6):1363–1368. doi:10.1542/peds.107.6.1363.
- Dreher A, Weilnhammer V, Gerstner D, Hendrowarsito L, Twardella D, Reiter C, Perez-Alvarez C, Steffens T, Herr C, Heinze S, et al. 2018. Longitudinal analysis of leisure noise exposure among adolescents with special focus on portable listening devices: the OHRKAN cohort study. Int J Audiol. 57(12):889–897. doi:10.1080/14992027.2018.1510187
- Feder K, Marro L, McNamee J, Michaud D. 2019. Prevalence of loud leisure noise activities among a representative sample of Canadians aged 6–79 years. J Acoust Soc Am. 146(5):3934–3946. doi:10.1121/1.5132949.
- Fligor BJ, Levey S, Levey T. 2014. Cultural and demographic factors influencing noise exposure estimates from use of portable listening devices in an urban environment. J Speech, Lang, Hear Res. 57(4):1535–1547. doi:10.1044/2014_JSLHR-H-12-0420.
- Gilliver M, Nguyen J, and Beach EF Barr C, editors. 2017. Personal Listening Devices in Australia: Patterns of Use and Levels of Risk. Semin Hear. 38(4):282–297. doi:10.1055/s-0037-1606324.
- Haile LM, Kamenov K, Briant PS, Orji AU, Steinmetz JD, Abdoli A, Abdollahi M, Abu-Gharbieh E, Afshin A, Ahmed H, et al. 2021. Hearing loss prevalence and years lived with disability, 1990–2019: findings from the global burden of disease study 2019. The Lancet. 397(10278):996–1009. doi:10.1016/S0140-6736(21)00516-X
- Hindelang RL, Dwyer WO, Leeming FC. 2001. Adolescent risk-taking behavior: A review of the role of parental involvement. Curr Probl Pediatr. 31(3):67–83. doi:10.1016/S1538-5442(01)70035-1.
- Jackson CA, Henderson M, Frank JW, Haw SJ. 2012. An overview of prevention of multiple risk behaviour in adolescence and young adulthood. J Public Health (Bangkok). 34(suppl_1):i31–i40. doi:10.1093/pubmed/fdr113.
- Jiang W, Zhao F, Guderley N, Manchaiah V. 2016. Daily music exposure dose and hearing problems using personal listening devices in adolescents and young adults: A systematic review. Int J Audiol. 55(4):197–205. doi:10.3109/14992027.2015.1122237.
- Kaplan-Neeman R, Muchnik C, Amir N. 2017. Listening to music with personal listening devices: monitoring the noise dose using a smartphone application. Int J Audiol. 56(6):400–407. doi:10.1080/14992027.2017.1297541.
- Kemper KJ, Danhauer SC. 2005. Music as therapy. South Med J. 98(3):282–288. doi:10.1097/01.SMJ.0000154773.11986.39.
- Kim G, Han W. 2018. Sound pressure levels generated at risk volume steps of portable listening devices: types of smartphone and genres of music. BMC Public Health. 18(1):481. doi:10.1186/s12889-018-5399-4.
- Kooijman MN, Kruithof CJ, van Duijn CM, Duijts L, Franco OH, van Ijzendoorn MH, de Jongste JC, Klaver CCW, van der Lugt A, Mackenbach JP, et al. 2016. The Generation R study: design and cohort update 2017. Eur J Epidemiol. 31(12):1243–1264. doi:10.1007/s10654-016-0224-9
- Le TN, Straatman LV, Lea J, Westerberg B. 2017. Current insights in noise-induced hearing loss: a literature review of the underlying mechanism, pathophysiology, asymmetry, and management options. J Otolaryngol - Head Neck Surg. 46(1):41. doi:10.1186/s40463-017-0219-x.
- Menon V, Levitin DJ. 2005. The rewards of music listening: response and physiological connectivity of the mesolimbic system. Neuroimage. 28(1):175–184. doi:10.1016/j.neuroimage.2005.05.053.
- National Institute for Occupational Safety and Health. 1998. Occupational noise exposure revised criteria 1998. Cincinnati: U.S. department of health and human services. Centers for Disease Control and Prevention.
- Paping DE, Vroegop JL, Koenraads SP, le Clercq CM, Goedegebure A, de Jong RJB, et al. 2021. A smartphone application to objectively monitor music listening habits in adolescents. J Otolaryngol - Head Neck Surg. 50(1):1–10.
- Parsons J, Reed MB, Torre Iii P. 2019. Headphones and other risk factors for hearing in young adults. Noise Health. 21(100):116.
- Portnuff CDF. 2016. Reducing the risk of music-induced hearing loss from overuse of portable listening devices: understanding the problems and establishing strategies for improving awareness in adolescents. Adolesc Health Med Ther. 7:27. doi:10.2147/AHMT.S74103.
- Portnuff CDF, Fligor BJ, Arehart KH. 2013. Self-Report and long-term field measures of MP3 player use: how accurate is self-report? Int J Audiol. 52(sup1):S33–S40. doi:10.3109/14992027.2012.745649.
- Rawool VW, Colligon-Wayne LA. 2008. Auditory lifestyles and beliefs related to hearing loss among college students in the USA. Noise Health. 10(38):1. doi:10.4103/1463-1741.39002.
- Shim H, Lee S, Koo M, Kim J. 2018. Analysis of output levels of an MP3 player: effects of earphone type, music genre, and listening duration. J Audiol Otol. 22(3):140.
- Statistics Netherlands. Standard classification of education 2021 (Standaard Onderwijsindeling 2021). Voorburg/Heerlen.
- Steinberg L. 2008. A social neuroscience perspective on adolescent risk-taking. Dev Rev. 28(1):78–106. doi:10.1016/j.dr.2007.08.002.
- Torre Iii P. 2008. Young adults’ use and output level settings of personal music systems. Ear Hear. 29(5):791–799. doi:10.1097/AUD.0b013e31817e7409.
- Vogel I, Brug J, Hosli EJ, CPB Van Der Ploeg, Raat H, van der Ploeg CPB. 2008. MP3 players and hearing loss: adolescents’ perceptions of loud music and hearing conservation. J Pediatr. 152(3):400–4. e1. doi:10.1016/j.jpeds.2007.07.009.
- Vogel I, Brug J, van der Ploeg CP, Raat H. 2007. Young people’s exposure to loud music: a summary of the literature. Am J Prev Med. 33(2):124–133. doi:10.1016/j.amepre.2007.03.016.
- Vogel I, van de Looij-Jansen PM, Mieloo CL, Burdorf A, de Waart F. 2012. Risky music-listening behaviors and associated health-risk behaviors. Pediatrics. 129(6):1097–1103. doi:10.1542/peds.2011-1948.
- Vogel I, Verschuure H, van der Ploeg CP, Brug J, Raat H. 2009. Adolescents and MP3 players: too many risks, too few precautions. Pediatrics. 123(6):e953–8. doi:10.1542/peds.2008-3179.
- Weichbold V, Zorowka P. 2007. Can a hearing education campaign for adolescents change their music listening behavior? Int J Audiol. 46(3):128–133. doi:10.1080/14992020601126849.
- Widén SEO, Erlandsson SI. 2004. The influence of socioeconomic status on adolescent attitude to social noise and hearing protection. Noise Health. 7(25):59.
- Williams W, Purnell J, Parnell J, Samuels S, Tsitsos C, Arcondoulis EJG, et al. 2010. The statistical distribution of expected noise level output from commonly available personal stereo players. Acoust Aust. 38(3):119–122.