Abstract
Energy benchmarking for buildings has become increasingly important in government policy and industry practice for energy efficiency. The questions of how energy benchmarking is currently conducted, and how it might be improved using rapidly growing quantities of self-reported data, are examined. A case study of commercial office buildings in New York City demonstrates how the rapid growth in self-reported data presents both new opportunities and challenges for energy benchmarking for buildings. A critique is presented for the scoring methodology and data sources for Energy Star, one of the largest and most successful benchmarking certification schemes. Findings from recent studies are examined to illustrate how this certification currently works in the marketplace. Self-reported building energy data are rapidly growing in Portfolio Manager (the user interface to Energy Star) due to mandatory energy benchmarking laws, and can be used to improve Energy Star's current scoring methods. These self-reported data are tested and improved for analysis by applying theories and methods of data quality developed in computer science, statistics and data management. These new data constitute a critical building block for the development of energy efficiency policies, and will affect how government, consultants, and owners measure and compare building energy use.
Introduction
How is benchmarking currently used to measure the energy performance of existing buildings, and how might it be improved, in particular using rapidly growing quantities of self-reported data? The continuing development of computers, networks and mobile devices is changing how data and information shape society (Gleick, Citation2011; Halpern, Citation2013). In energy benchmarking specifically, self-reported data, analysis and government policies are beginning to interact in new and interesting ways. While self-reported data are perhaps not yet ‘big’, this paper argues that building energy-use analysis in the near future will require insight into similarly new, rapidly growing data sources, much as existing data now shape current understanding of building energy use. Other data sources are likely to emerge with new technologies and policies, such as sub-metering, intelligent building controls, and other forms of pervasive computing and sensing.
This paper also seeks to expand the fundamental understanding of what benchmarking is and what it can do. Benchmarking in general is closely allied to the concepts of efficiency and productivity analysis, and the idea of energy benchmarking for buildings has been around for more than 30 years. It was recognized long ago that information asymmetries exist between all parties – building owners, tenants, investors, consultants and policy-makers. This is a fundamental obstacle to investment in energy efficiency, because parties who do not have reliable information about future operating savings are unlikely to pay upfront for additional improvements (Blumstein, Krieg, Schipper, & York, Citation1980). Benchmarking can potentially reduce these asymmetries and the high, associated transaction costs.
Benchmarking is often grouped with other certification schemes such as building ratings and eco-labels (Perez-Lombard, Ortiz, González, & Maestre, Citation2009). All these schemes are growing rapidly worldwide, as a result of either voluntary industry efforts or mandatory policies such as the European Performance Buildings Directive (EPBD) (Andaloro, Salomone, Ioppolo, & Andaloro, Citation2010). One key distinction is that benchmarking usually implies comparing the energy performance of similar buildings, hence they are sometimes called ‘operational’ ratings; while other ratings and certification schemes such as Leadership in Energy and Environmental Design (LEED) or energy codes can be met by including features at the design stage, hence they are sometimes called ‘asset’ ratings. While it is possible to compare buildings with modelled performance, as in ‘parametric’ or ‘simulated’ benchmarking, this paper specifically focuses on benchmarking systems that measure and compare the actual energy use of existing buildings.
The actual energy consumption of existing buildings has become recognized as important for a number of reasons. First, buildings consume approximately 40% of primary energy worldwide (Perez-Lombard, Ortiz, & Pout, Citation2008). Second, interest in Europe and the United States has gradually shifted from the building of new settlements to the management of existing building stock, which will dominate energy use for the foreseeable future (Kohler, Steadman, & Hassler, Citation2009; Nelson, Citation2004). A compelling example is New York City (NYC), which estimates that existing buildings consume 55% of the energy and produce 75% of the greenhouse gas (GHG) emissions in the city, and that 80% of the present building stock will still exist in 2050 (City of New York, Citation2010). Third, considerable evidence has emerged that building energy consumption is extremely heterogeneous, even in similar buildings, and that this heterogeneity often depends on occupant behaviour, operations and management (Lutzenheiser, Citation1993). Measuring actual energy use is a necessary first step in order to capture potential efficiencies in operations. Fourth, studies have shown that it can be more cost-effective to change operations by retro-commissioning rather than changing equipment by retrofitting (Mills, Citation2011). As a result, energy benchmarking has become an important instrument of policy, and a major focus of energy efficiency policy in the United States, particularly at the city and state levels. Over the past four years, nine major cities and two states (described below in ) have passed benchmarking laws that require the gathering of building energy performance data by owners and disclosure to prospective buyers and tenants, sometimes via public websites. Similarly, the further development of the EPBD is likely to lead to the introduction of new operational rating systems throughout Europe, though this will vary by country (Concerted Action EPBD, Citation2013).
This paper is structured as follows. Benchmarking efforts in the City of New York are described in the next section, as it is one of the first and the largest of the US cities to pass a benchmarking law, and because the data gathered help to illustrate the following arguments. Moving to a broader perspective, the structure of Energy Star (ES) is discussed, including its scoring methodology, assumptions and impact. The findings of numerous recent studies from economics and property (real estate) are examined in order to understand how and why the market values ES certification, and to show that particular design decisions in the current scoring methodology do not accurately communicate energy performance and savings to the market. This is followed by a consideration of the changing context of data. Tools and laws – such as Portfolio Manager (PM), the humble user interface to ES, and mandatory energy benchmarking laws passed by multiple cities – are all contributing to the rapid growth of data sources for building energy use, with implications for both analysis and policy-making. The section entitled ‘Self-reported data’ applies theories of data quality developed in other fields such as computer science, statistics and data management to the NYC data in order to show how the quality of self-reported data can be improved for energy benchmarking. The paper concludes with a discussion of further challenges and opportunities for benchmarking using self-reported data, both in the United States and in other countries.
NYC benchmarking data
Case study data
The case study of commercial office buildings in NYC, henceforth referred to as the NYC data, serves as a case study for the rest of this paper in order to illustrate many of the changes now occurring. The NYC data are gathered through ES and PM and were collected by the municipal government for analysis. Also, because of the large size of NYC, the NYC data actually contain more buildings than the US government studies for the entire country, and therefore illustrate some of challenges in ‘down-scaling’ data sampled at the national level to the local level. Finally, the NYC data illustrate some of the challenges involved with using self-reported data for energy benchmarking.
The NYC data were collected in 2011 and 2012 by the municipal government of New York as part of its mandatory energy benchmarking ordinance, Local Law 84 (LL84). This law was passed in 2009 as part of a comprehensive citywide environmental plan called PlaNYC 2030, which heavily emphasized buildings as a major source of local air pollution and GHG emissions. The law came into effect in 2011 for private sector buildings, requiring owners of all commercial and multifamily buildings over approximately 4645 m2 (50 000 ft2) to submit their energy information for online benchmarking through PM. ES ratings and energy use are publicly disclosed on an annual basis, allowing owners to compare their buildings with other similar facility types. Compliance was estimated to be approximately 75% in the first two years of the programme (City of New York, Citation2013).
Description of the data
This section will briefly describe novel aspects of the NYC data collected in 2012, and how the data were selected for use in this case study. For a much more extensive analysis, descriptive statistics and selected metrics of the entire dataset, see the two extensive benchmarking reports published and made available online by the City of New York (Citation2012, Citation2013). The following description is summarized from the most recent report.
The overall dataset gathered was for commercial properties over 4645 m2. In 2012, 13 258 properties and 24 071 buildings were subject to the law, constituting more than 214 million m2 of space. Of the gross floor area, 65% of the space was multifamily, 22% was office and 13% fell into other categories.
The buildings were distributed in a number of ways that describe NYC's unique built environment. The peak years of construction for existing buildings in New York were in the pre-war (1920s) and post-war (1950s–70s) periods. The size distribution of buildings is strongly left-skewed, meaning that there are many more small buildings than large. Most multifamily buildings in New York (65%) have one designated use, while most office buildings (67%) have one or more additional uses, such as ground floor retail, banking or parking.
Extreme variation in energy use and energy-use intensity (EUI) is one of the most notable features of the NYC data. Among the office buildings, the variation in EUI between the 5th and 95th percentile buildings varied by a factor of 3.3, and among the multifamily buildings the factor was 5.8, indicating extremely large variations throughout the population of buildings. Overall, in 2012, multifamily comprised 50% of the total energy use and 55% of the GHG emissions from buildings. Office comprised 30% of the energy use and 26% of the GHG emissions from buildings.
For the purposes of this paper, the case study was limited to large commercial office buildings in NYC, so it could be compared with the largest facility type in the Commercial Buildings Energy Consumption Survey (CBECS), which is discussed below. Also, the case study is limited to offices because an ES technical methodology exists for this category, but not for multifamily buildings. Only buildings that could be matched between the 2011 and 2012 datasets were used, and only single buildings on property lots were used, because of ambiguities in the reporting of multiple buildings on a lot. This resulted in a dataset of 801 large commercial office buildings. Basic descriptive statistics for large commercial office buildings in the NYC data are shown in .
Table 1 Descriptive statistics for New York City large commercial office buildings
Energy Star for benchmarking
How does Energy Star work?
ES was originally introduced by the US Environmental Protection Agency (USEPA) as a voluntary energy efficiency programme that provided labelling for efficient appliances. The impact of ES on energy consumption across all product categories is quantified in several studies (Banerjee & Solomon, Citation2003; Brown, Webber, & Koomey, Citation2002; Sanchez, Brown, Webber, & Homan, Citation2008).
ES began offering voluntary scoring for buildings starting in 1999, and PM was introduced in 2000 to help building owners and managers gather their data and to produce ES scores for selected facility types (USEPA, Citation2009). Since then it has grown to be the single largest energy benchmarking scheme for buildings in the world, with more than 300 000 buildings benchmarked and more than 20 000 buildings certified by the end of 2012 (Zatz, Citation2013). ES has also recently been introduced in, and adapted to, Canada. Similar building energy performance rating programmes have been introduced around the world, throughout Europe and in Asia (Burr, Keicher, & Leipziger, Citation2011).
ES depends critically on external data sources. Much of the knowledge about building stock and energy use in the United States comes from CBECS and the Residential Energy Consumption Survey (RECS). Since 1978, these surveys have employed a complex multi-stage area probability sampling methodology, based on area frames and administrative lists, in order to represent buildings of all sizes and uses in their respective sectors at the national scale (US Energy Information Administration, Citation2014). In the past 20 years these surveys have been performed approximately every five years and are considered to be relatively sophisticated and comprehensive surveys, though the most recent CBECS data available are only from 2003 due to design errors in sampling in 2010. The impact of these sampling methods will be discussed further below. ES also takes advantage of national weather station networks to normalize energy use for variation in local weather.
Describing the ES scoring methodology helps to illustrate some of its inherent design decisions, as well as how it depends on external data sources such as CBECS, RECS and weather data. ES has followed a technical methodology that has remained fairly consistent and is similar to other national benchmarking systems (USEPA, Citation2007, Citation2013); Chung (Citation2011) identifies this as a variant of the ordinary least squares (OLS) benchmarking method. This process has the following stated objectives:
evaluate energy performance for the whole building
reflect actual metered energy use
equitably account for different energy sources
normalize for building activity
provide a peer group comparison
Users enter a full year of monthly energy bills for all fuel types and answer questions about general building characteristics, similar to questions in CBECS and RECS. Next the site (metered) use of each fuel is converted into source (primary) energy using thermal conversion factors calculated by region, and the effects of weather are normalized using a regression model. An actual EUI is then calculated by dividing the source energy by the gross floor area of the building.
A predicted EUI for each building is generalized from CBECS, similar to the statistical adjustments to EUI suggested by Monts & Blissett (Citation1982). After extracting the appropriate facility types from CBECS, and filtering outlier buildings, a regression model is fit to the source EUI observed for each facility type, taking into account factors specific to that facility type. In the CBECS microdata there are 5215 total buildings, representing a total estimated population of 4 858 750 buildings nationally. In the microdata, 736 buildings are considered to be commercial office, bank/financial or courthouse buildings, and after omitting buildings that have unusually high or low values for computer and worker density, hours of operation and unusual fuel types, the base sample of buildings in the regression model is 498 buildings nationwide. Finally, an energy efficiency ratio is then computed between the predicted and actual EUI, as suggested by Sharp (Citation1996). Based on a gamma statistical distribution that is also fit to the CBECS sample, a percentile ranking (or 1–100 score) is calculated for each ratio. This additional step means that all ratios, no matter how high or low, can be fit to the same percentage scale or lookup table.
The stated objectives listed above are intended to be met by standardizing the process of gathering data for the whole building from actual metre bills or data. In order to account equitably for different energy sources, the thermal conversion factors calculate losses in the conversion from source (primary) to site (metered) energy, mainly to account for transmission losses in electricity. Building activity is normalized principally by area but also in terms of computer and worker density, and hours of operation. Using CBECS as a reference provides peer group comparison at the national level.
Finally, ES also offers a certification if buildings meet three criteria: the building must be one of the recognized facility types; the entered information must be verified by a licensed engineer; and the ES score must be over 75, ranked on the 1–100 scale.
Energy Star in the marketplace
This certification process is important because numerous studies have shown that building eco-labels such as ES and LEED certification are working as intended in the real estate and construction industries, particularly in how energy efficiency is valued. Multiple recent studies by researchers in economics and real estate using econometric methods have consistently found these certifications are associated with statistically significant and positive effects on real estate value, in terms of either asset or market value, rental rates or occupancy. These studies variously attribute the premia associated with certification to many possible causes, including but not limited to: scarcity of available buildings to meet demand (Wiley, Benefield, & Johnson, Citation2010); additional occupier benefits, decreased holding costs or risk premia (Fuerst & McAllister, Citation2011b); marketing benefits, novelty effects or market acceptance (Das & Wiley, Citation2014; Fuerst & McAllister, Citation2009); or decreased future operating costs (Eichholtz, Kok, & Quigley, Citation2013).
In order to understand the market context in which these eco-labels and certifications are used, it is important to focus on how these studies control for similar buildings and locations. Most of these studies use data from CoStar, a real estate data provider, to obtain the outcome (dependent) variables of transactions, rental prices or occupancy. Almost all these studies control for the following factors:
specific real estate submarkets, by either comparing or matching eco-labelled buildings with non-labelled buildings nearby, though the matching methods vary
eco-labelling is only measured in terms of ES or LEED certification rather than by the specific numeric point rating or score
ES and LEED certifications are usually treated separately; buildings with both certifications are treated as a separate category to avoid collinearity
building size, age and real estate class (class A, B, etc.)
Additional studies indicate that the premia associated with certification varies for particular subgroups of buildings, such as by submarket or location (Eichholtz, Kok, & Quigley, Citation2010); for multiple certifications (Fuerst & McAllister, Citation2011a); or by age of construction (Fuerst, van de Wetering, & Wyatt, Citation2013).
In summary, studies from economics and real estate almost always control for similar buildings in terms of multiple building characteristics, rather than relying on the normalizing or equitable accounting of ES alone. In order to make valid treatment and control comparisons between the sale or rental value of buildings, numerous other explanatory variables such as real estate submarkets, size and age all need to be included. These are all found to be statistically significant and in many cases to have greater effects on observed building values than the effect of the ES or LEED certification alone.
Finally, from the structure of the real estate and economic studies, it appears that while ES and LEED certifications are valuable, it is not clear what the impact of more detailed 1–100 scale for ratings or scores actually are in the marketplace, since none of the studies explicitly accounts for numerical ES scores, although some studies include the effect of different levels of LEED certification. Detailed numerical ratings on a 1–100 scale are presumably valuable for building managers and owners to manage their energy use year to year, or heuristically depending on changing circumstances, like the change of a major tenant in the building. Different rating scales or systems might be simpler and more effective in practice. Therefore, ES's current practice will be critically examined in the next section to consider how it might be improved or superseded.
Critique of Energy Star
Recent work in economics indicates that people can systematically ignore, make suboptimal decisions or are unable to perceive information in particular settings, even when it is correct (Kahneman, Citation2011). Considerable work in energy efficiency early on indicated that people do not necessarily value decreased operating costs as engineering–economic models do (Howarth & Sanstad, Citation1995; Levine, Koomey, McMahon, & Sanstad, Citation1995; Lutzenheiser, Citation1993). As a result, recent work emphasizes the role of social approaches and norms in energy consumption, and seeks to operationalize these behavioural insights for energy efficiency (e.g. Allcott, Citation2011; Karvonen, Citation2013; Schweber & Leiringer, Citation2012). Markets do not necessarily value the quality of information correctly. Technical information in building engineering (as in every field) can be flawed even if people or the market believe that it is valuable. In short, it is possible to communicate the wrong information to the market and for people to value it (until corrected). How can information for the market therefore be improved?
Considering the ES scoring methodology further in the context of local real estate markets and information reveals that its current implementation has multiple problems. Many of these problems are related to the way that ES depends on CBECS, and how it aggregates national data from CBECS to provide a reference standard.
CBECS data are old
It is already well known that the most recent CBECS data are out of date. CBECS has not been updated since 2003. This problem should be corrected when the next CBECS dataset, collected in 2012 and 2013, is released. Recent discussions have focused on how to improve the relevance and utility of CBECS and RECS by changing their sample size, design and reporting interval (Eddy & Marton, Citation2012).
Heterogeneity and aggregation
The greatest problem with how ES relies upon CBECS is that it cannot describe heterogeneity in local building stock, exaggerates measurement error and aggregates these differences away. While CBECS follows a complex sampling process, sampling is also the central limitation of CBECS: despite the expensive investment of time and effort, it represents a very small portion of the US building stock and therefore is not intended to capture wide variation in energy use within the overall population of buildings.
For example, consider large office buildings. Analysing the CBECS public-use micro-data, there are only 364 buildings nationwide in the sample defined as commercial office and financial buildings larger than 4645 m2. The adjusted weight of this building sample is intended to represent 34 011 similar buildings, or 93 buildings for each building in the sample. The problem is that 364 buildings distributed nationwide cannot possibly reflect the diversity of buildings that exists nationally or in any local submarket. The two smallest geographic units reported in the CBECS micro-data are census divisions, which divide the US into nine regions and five climate zones. shows the frequency table for each region and climate zone, as well as the number of buildings that are represented by their sample weights in CBECS.
Table 2 Commercial Buildings Energy Consumption Survey (CBECS) buildings sampled and weights by census division and climate zone, for office buildings > 4645 m2
While all sampling processes are intended to reduce and analyse overall population using a representative sample, each cell in includes very large and diverse real estate markets. For example, for the largest three metropolitan areas in the United States:
the entire NYC commercial office market is represented by 41 buildings in climate zone 3 in the Middle Atlantic region; however, this also includes the real estate markets of New Jersey, Philadelphia and Pittsburgh
Chicago is represented by 60 buildings in climate zone 2 in the East North Central region, but this also includes Cleveland and Columbus
San Francisco and Los Angeles (combined) are represented by 39 buildings in climate zone 4 in the Pacific region, etc.
Reduced representation is exactly what statistical sampling is intended to do, but it cannot capture the intrinsic heterogeneity of buildings at the local level, or provide meaningful peer-group comparisons for individual buildings, which will be discussed further.
Measurement error
Heterogeneity is also related to the issue of measurement error in the sampled buildings, which is impossible to quantify but very important. As discussed above, in analysis of the NYC data, it has been found that even within the same facility type and for similar size buildings, EUI can vary by a factor of 4 or 5 (City of New York, Citation2012). Given the intrinsic heterogeneity in buildings observed in NYC, it is quite possible that error in either selecting buildings in the sample, or measuring them, would lead to very large variations in subsequent calculations of total energy use, or what the predicted EUI should be for an office building of typical size and energy use. While it may be possible to derive more accurate summary statistics such as mean energy use and EUI across the entire aggregated sample, these two summary statistics may not be representative of distinct subgroups of buildings, as the studies of ES in the marketplace indicated above in the section entitled ‘Energy Star in the marketplace’. Furthermore, these two summary statistics also may not be equally accurate, depending on the size distribution of buildings.
Statistical uncertainty
Since ES is based on a statistical regression model, the predicted EUI and corrections for specific factors are fundamentally uncertain. As a result, there are significant errors intrinsically associated with ES scores that are not reported, which were calculated using the following process. The published ES coefficients and standard errors were applied to the NYC data in order to obtain a range of predicted EUIs. The published parameters for the gamma-distribution, and its standard errors, were then applied to get ES scores. Errors were propagated through both calculations by simulating the standard errors in the coefficients each 5000 times, and the error was then taken as the standard deviation (SD) in the simulated ES ratings.
shows that the mean and median simulated ES score results are very close to the reported ES scores, but that there are significant uncertainties attached to the scores in both statistical models. For example, if errors were reported only from the first-stage regression model fit, then the median uncertainty in ES scores would be ±7.0 points. Including both the first- and second-stage standard errors leads to errors of ±14.8 points. In either case, statistical uncertainty as a result of the ES regression model is significant. summarizes the simulation results by stage. Calculations show that if uncertainty from both stages were included, then 17% of buildings with reported scores of 75 or higher – the threshold for ES certification – would in fact fall below this score. This casts doubt on these scores as part of ES certification.
Figure 1 Statistical uncertainty in Energy Star (ES) scoresNote: A diagonal line indicates a perfect correspondence between simulated scores with official reported ES ratings; dots indicate the simulated versus the official scores; and error bars show uncertainty from the first and second stage regression models
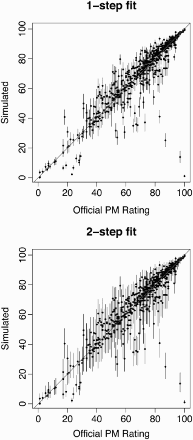
Table 3 Summary of simulation results for Energy Star (ES) scores in New York City data
In addition, without performing any calculations and simply summarizing the differences year over year, ES scores vary greatly. While it is not clear whether this is due to inadequate weather normalization or changing input data, the changes in scores year to year are significant and lead to confusion among users. shows a histogram of differences between ES scores in the first and second year of reporting. shows the median year-over-year difference in ES scores is approximately 4 points.
Table 4 Quantiles of year-over-year difference in Energy Star (ES) scores
Emphasis on EUI
Another problem with the current ES methodology is that it normalizes buildings by EUI, which is in units of energy per area. While this has become a standard metric for buildings, it is not clear if EUI itself is a metric that should be used to measure the efficiency potential or performance of a building. Recent studies by Retroficiency, an energy consulting firm, show little relationship between a building's calculated EUI and the energy efficiency potential that could be realized from specific conservation measures (Retroficiency, Citation2013). Intuitively, it does not make sense that all buildings use energy at the same rate per area, or to assume that the regression model for ES introduced in the third section will affect differently sized buildings in the same way. For example, the EUI ratio for a small office building with total source energy of E and area of A has the same ratio as a much larger building of 10E and 10A, but ES applies correcting factors for key characteristics in the same way to the EUI for both buildings.
Emphasis on the wrong data
ES as it is presently calculated throws away valuable information by aggregating over fuel types and months. In order to calculate an annual EUI, monthly bills for all fuel types over a year must be summed to obtain the total energy use of the building, and then only the annual EUI is used in ES calculations. However, it does not make sense to throw away information about the relative use of different fuel types, or by annualizing raw monthly data, in the absence of consistent and reliable auditing of large populations of buildings. For example, Hsu (Citation2014) finds that monthly utility data are a better predictor of future energy performance than extensive engineering audit information.
Finally, ES uses source (primary) energy rather than site (metered) energy, mostly to account for transmission losses using regional conversion ratios. However, site energy is more relevant and salient to individual building owners because it directly relates to on-site consumption decisions that are under a building owner's control, rather than to transmission on the grid.
Changing the context of data
How is context changing?
The previous section identified flaws in the ES scoring methodology in typical benchmarking practice. ES is, in some sense, a victim of its own success: while it has been wildly successful in growing acceptance of green buildings, some of the original design decisions embodied by ES no longer make sense in the situations in which it is being used, particularly at the local scale.
This section describes the changing context of data in their generation, analysis and use. It also highlights the important role of PM, the humble user interface for ES, in gathering and creating a disaggregated, self-reported dataset that is much larger than CBECS or any other available data source. This section also illustrates the tensions between ES and PM, respectively, as systems for comparing and gathering data about buildings and energy use. This raises the possibility that these systems may diverge in their future potential and use.
Portfolio Manager as a growing data source
PM is a web-based user interface for ES. It is intended to complement ES by making it easy for building managers and owners to store energy data and related building characteristics. PM recently had its interface and front end upgraded to assist in data entry, and to add better automated reporting. However, this upgrade did not significantly change the basic data that PM gathers on space and energy-use information, similar to the questions asked in CBECS.
Despite these rather humble beginnings, PM has become the de facto and dominant system for collecting information about building stock and energy in the United States. shows the rapid growth of ES and PM relative to CBECS and RECS; LEED is also included for comparison. What is striking in is how rapidly ES and PM have grown relative to other information systems, including CBECS, RECS and LEED. While CBECS and RECS are intended to be statistical samples of the overall population, the drawbacks of sampling were previously examined in the third section. In contrast, the rapid growth in PM was certainly driven by general industry acceptance of ES, as described above, as well as the rapid growth in disclosure laws, which will be described in the next section.
Figure 3 Growth in building energy data since 1980Note: Commercial Buildings Energy Consumption Survey (CBECS) and Residential Energy Consumption Survey (RECS) are sampling strategies designed to capture the entire US building population. Leadership in Energy and Environmental Design (LEED) buildings include all those that have been certified or registered.
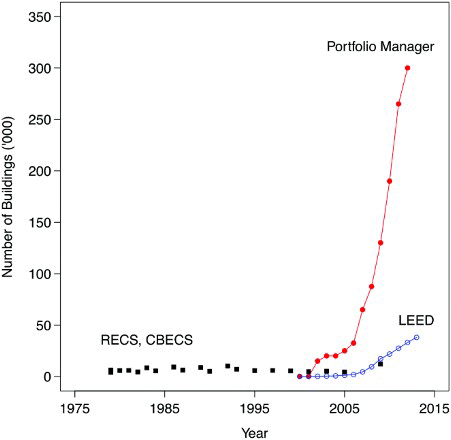
also highlights a divergence between the uses and mutability of both ES and PM. PM may begin to exhibit ‘lock-in’ effects typical of information systems (Katz & Shapiro, Citation1994). Lock-in means that there are significant impediments to changing such a widely used system, and that the overall process of data collection may be difficult to change with so many users already familiar with the present system. Given the number of buildings already in PM – estimated by the USEPA to be 40% of all commercial building stock in the United States by area – there are already significant incentives for other information systems to work with or be compatible with ES and PM, e.g. new LEED ratings for existing buildings require ES certification. Many other leading organizations, associations and companies use ES scores as part of their information and services.
Disclosure laws as drivers of growth
Disclosure laws have been a key driver in the growth of PM. LEED is an important model for how ES has become mandatory in some cities (Burr et al., Citation2011). National Public Radio reported:
LEED is a force to be reckoned with in the construction world. Fourteen federal departments and agencies, 34 states and more than 200 local governments now encourage or require LEED certification. Some places offer incentives to certify. Others, like Washington, mandate it as a kind of code.
Following the same policy trajectory as LEED, ES scoring is rapidly becoming mandatory in some cities and an operating standard for others. Of the nine major cities and two states in the United States that require benchmarking, all use PM to gather data and report ES scores. Furthermore, the growth of disclosure laws at the local level offers much better data about building stock in these local jurisdictions. shows the number of buildings that are currently being measured by benchmarking ordinances in US cities and states.
Table 5 Disclosure laws by city or state
Information disclosure theory
Studies of information disclosure policies offer insight into how to think about how rapidly growing data in PM might be used independently of the existing ES scoring methodology. As a policy instrument, mandatory information disclosure – also known as targeted or regulated transparency – has been enacted in areas as diverse as financial regulation, environmental protection, workplace safety, healthcare, crime prevention, diet choices and food safety. Weil, Fung, Graham, & Fagotto (Citation2006, p. 155) define regulatory transparency as ‘the mandatory disclosure of structured factual information by private or public institutions in order to advance a clear regulatory goal’. Fung, Archon, & Weil (Citation2007) attribute the growth of disclosure laws to three factors, including right-to-know transparency laws; urgent crises that revealed that secrecy led to greater public risks or harms; and the growing body of work by economists and psychologists revealing that markets can be subject to information and communication failures, thus justifying government action. Sunstein (Citation2013) argues that disclosure laws can improve decision-making while preserving freedom of choice.
All these authors in particular focus on the importance of releasing the right amount of information to the marketplace and actors within it, so that it can affect their decisions and spur disclosers to make better choices. Fung et al. (Citation2007) argue that information disclosure must occur within an ‘action cycle’, in which disclosers recognize that users make choices based on new information, leading disclosers and users to improve their choices, practices or products. This concept can be thought of as similar to the idea of continuous improvement, pioneered by the Toyota Company in automotive production, but instead of occurring within a linear assembly line, it occurs between actors in the marketplace.
It is important to recognize that the data-gathering process also shapes subsequent analysis. ES's reliance on CBECS, when compared with the rapidly growing data within PM, may lead to fundamentally different conclusions or insights. PM is mostly populated by information that has been entered voluntarily and self-reported. Licensed consultants are sometimes hired to perform benchmarking, though of more than 300 000 buildings that are in PM, only approximately 22 000 are ES certified. The potential of PM as a data source – separate from ES – will be limited by the quality of the self-reported data, which have their own intrinsic limitations that will be explored in the next section.
Self-reported data
Advantages
Data generated from mandatory local laws have two particular advantages. First, compared with the national CBECS and RECS surveys, they have a much higher spatial resolution and therefore allow a reasonable comparison of buildings built under similar conditions such as local climate, urban density, construction practices, fuel and infrastructure systems, zoning, and regulatory enforcement. Second, since they capture an entire population through compliance rather than by sampling, this captures heterogeneity and by extension should enable better peer-group comparisons by fuel type, size and age. Future research can detail the ways in which these advantages could be realized, but the appeal of more and better data at smaller scales is obvious. However, in order to enable all these advantages, it is also necessary to fix the chief problem with self-reported data, which is data quality (DQ).
Disadvantages
There are many possible sources of error in data that are self-reported. Mistakes in data entry may simply occur when entering benchmarking data, because it requires owners, managers or consultants to enter many unfamiliar multiple digit numbers in order to report energy use and key building characteristics. Users may simply not know the number of workers or computers in their building, and even basic measurements such as occupied gross floor area. In addition, lack of user understanding can lead to invalid data. For example, a user might enter the wrong units, such as megawatt-hours or kBtu instead of kilowatt-hours.
Most of these problems seem to be both ad hoc and obvious once the data are examined closely. Automated upload of information from utilities and range-checking may minimize errors in the entry of new data. However, fixing, cleaning or simply recognizing bad data in an existing large dataset may require many such decision rules, all based on domain knowledge or context. Furthermore, intentional entry of false information (i.e. ‘gaming the system’) is likely to arise as policy-makers increasingly rely on benchmarking data to target and enforce energy efficiency policies, and as penalties for poor energy performance are enacted, as some cities are currently considering. Theories of DQ can help explain how to correct data structures, such as in energy benchmarking data.
Theories of data quality
Enthusiasm about data-driven science continues to grow (Halevy, Norvig, & Pereira, Citation2009; Hey, Tansley, & Tolle, Citation2009), but poor DQ has serious consequences for knowledge, analysis, and action in all fields, including but not limited to statistics, government, finance and healthcare (Barrett & Richard, Citation2009; De Veaux & Hand, Citation2005; Hoffman & Podgurski, Citation2013; Neely & Cook, Citation2011). This has put renewed emphasis on theories of DQ. Although DQ is an issue that underlies all empirical research, much of DQ theory comes from statistics, computer science and process management, fields that have historically dealt with large quantities of data. This section will summarize some useful theoretical insights from the literature to assess and verify DQ in order to understand how to adapt these theories to improve the quality of energy benchmarking data.
A consensus has emerged in the existing literature that frames DQ in terms of decision theory, i.e. the study of how information, values and uncertainties may enter into a decision, action or process, and therefore affect important outcomes (Fisher & Kingma, Citation2001; Karr, Sanil, & Banks, Citation2006). DQ is considered to be both a process and an outcome like benchmarking itself: it is a process of cleaning and assessing the quality of the data, but also with the ultimate goal of improving data to aid better decisions and actions.
However, it is surprisingly difficult to define the practice of DQ for four main reasons. First, judging the impact of information is essentially a qualitative judgement that depends on context and domain knowledge. Data that are of acceptable quality in one context for a particular purpose may not be acceptable for another context or purpose. Second, although the issue of DQ comes up frequently in many fields, it is usually only addressed on the way to some other research problem. Very few empirical papers are specifically devoted to solving DQ problems, and therefore very few examples of best practice exist. Aspects of DQ usually fall under the activities of data cleaning, exploratory data analysis, descriptive statistics and research design, though these activities are implemented with widely varying frequency and rarely reported in the literature (Wang, Storey, & Firth, Citation1995). Third, expectations for when and how data can be used are rapidly changing: in addition to the torrential flow of new data, analysts are now using data for purposes for which they were not originally intended by joining or ‘federating’ datasets together. This, however, often leads to a disconnect between the design of experiments, gathering of data and ‘black-box’ methods for analysis (Dasu & Johnson, Citation2003). Fourth, and as mentioned above, identifying and fixing errors in large datasets potentially requires many decision rules based on specific context and domain knowledge. For all these reasons, it is impossible to summarize the entire prospective range of DQ techniques.
Instead, the DQ literature has generally identified different thematic categories, often referred to as ‘hyper-dimensions’, because they are broad categories that contain other specific issues. Three key DQ hyper-dimensions, adapted from Karr et al. (Citation2006), are of User Needs, Process Control, and Data. Of these hyper-dimensions, only Data itself can be meaningfully measured quantitatively, though quantitative analysis can also assist in understanding the other two hyper-dimensions of User Needs and Process Control, as will be demonstrated further below. The key question is then how to translate each of these various theoretical hyper-dimensions into useful methods to assess DQ. Translating DQ theories into functional methods is best illustrated in practice on actual data. The following sections discuss how this was done in the case study of the NYC data.
Methods for data quality
This section shows how theories of DQ can be put into practice in the context of energy benchmarking data. Each of the hyper-dimensions and dimensions is illustrated using the NYC data as described above. Assessment of the data itself requires exploratory data analysis and profiling, which leads to particular data editing rules, checking of data definitions and dictionaries, and increasing levels of verification and checking with experts (Dasu & Johnson, Citation2003). This section presents only a preliminary description of methods, though a committee of policy-makers, practitioners and researchers worked intensively on a common process of data cleaning, as described in the NYC benchmarking reports (City of New York, Citation2012, Citation2013).
User needs for data
The DQ hyper-dimension of User Needs includes accessibility, relevance, timeliness and integrability. Meeting many of these needs must be done in the initial design of the data collection, in how the analysis is conducted and in how results are subsequently communicated. Since the data are intended to transform the market for energy efficiency by providing information about the energy performance of certain buildings, the benchmarking data need to be accessible for all owners, prospective buyers and tenants. The NYC data are available for public access after the first year, but other cities, such as the City of Seattle, restrict it only to prospective buyers. The NYC data are also intended to be relevant and timely for users by allowing benchmarking comparisons of building ratings on an annual basis.
Policy-makers and researchers are users of the data. A particular area of interest is the relative distribution of buildings, floor area, energy use and GHG emissions. The metrics for each individual subgroup are important, but it is also important to note the magnitude or proportion of metrics within samples relative to the whole population. Sequential cleaning is explored further below.
As was seen with ES above, many parties in the real estate and construction industries use this information to compare their individual buildings with similar buildings. Correct identification of peer groups is therefore a critical activity. However, which buildings in the local population can be considered similar to any particular building requires further normative judgement. At present in analyses of ES scores and in the NYC data buildings are compared against other buildings simply by partitioning by facility type, and then by comparing EUIs. Given the richness of the NYC data, additional research is needed to consider how to identify appropriate subgroups based on other available information, such as similar sizes, ages and fuel consumption.
Data process control
Unlike the DQ hyper-dimension of Data, which lends itself to quantitative analysis, the DQ hyper-dimension of Process Control can only be characterized as a qualitative judgement. The DQ hyper-dimension of Process Control is composed of reliability, metadata, security and confidentiality; this is usually judged using checklists rather than metrics.
One valuable contribution of the DQ literature has been to emphasize the importance of understanding the process by which data are produced. , adapted from Dasu & Johnson (Citation2003), formally structures the steps by which data are gathered and processed. Not all the steps are immediately relevant to benchmarking data, but working through the processes by which data are produced highlights the ways in which DQ can become either degraded through time or improved by making specific changes in the data-gathering process.
Figure 4 Data-gathering process. Source: adapted from Dasu & Johnson (Citation2003)

Partitioning the data, for example, this time by consultant, led to an important insight into the role of benchmarking consultants in producing the data. From the group of buildings that engaged consultants to gather data, there were only 20 or 30 consultants who were responsible for benchmarking more than 80% of all buildings. This led NYC officials to give workshops and targeted feedback as a direct way to improve the reporting of particular consultants.
Data cleansing
The dimensions of completeness, consistency, validity and accuracy commonly describe the DQ hyper-dimension of Data. Completeness is whether the data are completely filled out, or if there are missing data. Consistency is whether information is repeated in the database in a repeated and consistent fashion: misspelling is a frequent source of consistency error. Validity is a weaker but easily measured form of accuracy in which data must be valid in terms of units and format. For example, buildings cannot have ‘A’ numbers of floors, or for that matter ‘12345’ floors. Accuracy is whether the value recorded in the data conveys the intended meaning: this is the most difficult to measure and is impossible to verify without exogenous knowledge.
In order to simplify these dimensions into usable strategies, it is possible to exploit the common structure of benchmarking data in order to identify methods that assess each of these DQ dimensions. The NYC data are represented as a structured two-dimensional table. Each record (or row) represents a distinct building. Each column (or field) represents a particular observation about, or aspect of, each building. Fields may also represent metadata, i.e. data about the origins or nature of other data; delimiting variables, such as acceptable number ranges; unique identifying information; and key variables of interest, such as energy and space uses, GHG emissions, and so on.
Since the NYC data are structured as a two-dimensional table, there are a finite number of ways in which the NYC data can be analysed in comparison with themselves and with external datasets and knowledge. Each panel in diagrams a different way of grouping or slicing the data, so they can be compared with themselves or with exogenous data:
Internal comparison shows how some columns may conflict or contradict one another. Panel A highlights two specific columns from two different data sources. For example, the NYC data were joined with the local property tax database. Floor areas should not be the same in the two databases, since the taxable area does not include basements and common areas, which do affect energy performance. However, since a substantial number of buildings have the exact same area in both fields, it is likely that owners reported these data by simply looking them up in their tax records rather than by actually measuring them.
Partitioning the data into different subgroups, as in panel B, can reveal systematic differences in associated fields. Since EUI can differ significantly based on building type, the data and analysis are partitioned by these types. In this paper, only commercial office buildings are presented in the NYC data, but trimming or removal of outliers can be done for each group, as ES does when applied to CBECS data.
Control groups of high-quality information can be developed by identifying key subgroups in the data, and then comparing or joining them with additional exogenous information, as in panel C. It is also possible to exploit additional knowledge about subgroups within the overall population to understand the whole: this inverts the strategy of using a representative subsample to impute data about the overall population, and instead uses a known subsample to check if the overall population resembles data that are known to be of good quality. For example, city officials had knowledge that some owners and consultants had conducted extensive energy efficiency audits, which were considered to be more reliable. A control group was therefore assembled of office buildings to check the overall EUI and size distribution of buildings. Histograms and quantile–quantile (‘Q-Q’) plots showed that the source EUI distribution of sample buildings is a very close match with the overall office population. Other more advanced statistical methods could be used to compare other control groups with the larger population, but this method generally assesses the DQ dimensions of validity, consistency and accuracy.
External comparisons in panel D are made by introducing other kinds of engineering knowledge to the entire dataset, though exogenous information may have DQ issues of its own; it is often difficult to obtain comparable statistics and knowledge from two different datasets. For example, the reported mean primary EUI for office buildings in the 2003 CBECS is 678.2 kWh/m2. This compares well with the median EUI of 662 kWh/m2 reported for the NYC data in , but CBECS unfortunately does not report comparable means, medians or quartiles for additional comparison.
Figure 5 Strategies for structured dataNote: The highlighted left portion shows the data available (p1 fields, n rows in each group); the unshaded right portion shows where data are either missing or contained in an exogenous dataset (p2). Panel 1 (upper left) shows ‘internal comparisons’: how some columns in the dataset may conflict with one another; panel 2 (upper right) shows the ‘partitioning’ of records by different subgroups (n1, n2, … ) in the data; panel 3 (lower left) identifies ‘control groups’ in the data, i.e. key subgroups that can be compared with exogenous information (p2); and panel 4 (lower right) shows ‘external comparisons’ of aggregated statistics for select subgroups (n1, n2) with exogenous information (p2)
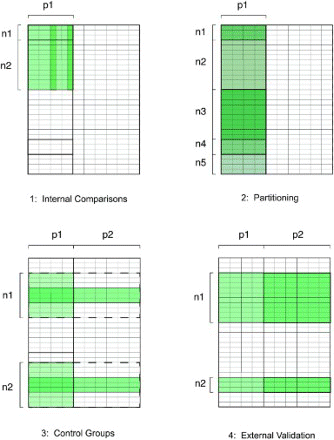
Discussion and conclusions
This paper began with an extensive critique of ES as it is presently implemented; highlighted the importance of PM as a data-gathering tool that is generating unprecedented amounts of data; and then turned to self-reported data, which are rapidly being captured by PM and other similar systems. However, these data need to be cleaned and assessed for quality before they can be used for analysis and comparison.
It was argued that the present ES scoring methodology unnecessarily aggregates buildings by geography, size and fuel types to the point where the scores do not adequately provide useful peer-group comparisons. A review of studies showed that ES indeed has a considerable impact on price premia, but that almost all studies seek to control for local submarkets using readily available building size, age and other real estate data. Finally, ES was shown to rely inappropriately on CBECS and other national samples to benchmark heterogeneous local submarkets.
This critique implies several possible directions for further research and policy-making. First, ES could be modified to remove distortions introduced by the current methodology. Second, the existing PM data could be combined with other sources of building data, as is currently done in econometric studies of ES. Or, third, localized benchmarking systems could be developed based on existing PM data to provide more meaningful peer-group comparisons. All these suggestions stem from the critical fact that while it would be relatively easy to change the ES scoring methodology, the self-reported data contained in PM have grown into a valuable and possibly irreproducible resource. PM continues to grow, and new data sources in the future are more likely to resemble PM than CBECS, with some of the same DQ issues.
The US cities and states that have already passed energy performance disclosure laws represent promising opportunities to try new energy efficiency policies, because they already have rich sources of data. Theories of disclosure provide additional ideas on how policy-makers can shape markets using data, information and knowledge. Similarly, while many other cities are currently interested in pursuing benchmarking, these cities need to think beyond the first step of merely providing information, which may constitute a particularly weak form of market transformation. Additional policies will be needed to make owners act on the information.
These additional policy opportunities, however, depend on the willingness of policy-makers to try new policies that use disclosed data to identify and target inefficient subgroups of buildings. A careful balance must be maintained: since disclosers must have an incentive to release accurate and valid information, energy benchmarking must therefore continue to create value to individual building owners, while discouraging cheating or gaming. For inefficient buildings, penalties and enforcement will have to be balanced carefully with subsidies and incentives. The good news is that this will be a vast improvement over the current structure of utility-based incentives in the United States, which usually provide subsidies and incentives for energy conservation measures regardless of need, leading to inefficiencies because of free-riders.
As the implementation of disclosure and data changes over time, the continuing experience of US cities and states should also be useful to policy-makers and consultants in other countries and regions as they implement their own energy benchmarking policies. The EPBD, since it will be implemented differentially across a large building stock, is also likely to inform experiences in the United States, and vice versa.
Since computing and data are changing so rapidly, it is impossible to predict how building energy data will be used for benchmarking in future. The rapid growth of data contained within the PM system is a promising opportunity to provide the marketplace with more and better data, which will open up more possibilities in applying statistical methods that characterize the popular term ‘big data’ or ‘analytics’. Inertia or ‘lock-in’ in the system of data collection could be overcome by joining or ‘federating’ energy performance data with other data sources that may emerge quickly, such as sub-metering, building intelligence systems, pervasive sensors and computing, or through situational awareness from other devices, such as smartphones that people carry into their workplaces.
On the other hand, the nascent ‘Internet of Things’ is already generating much more data than analysts can currently understand. Maintaining the quality of newly available data will be a continuing challenge that requires in-depth understanding of user needs, process control and the structure of the data themselves. Government, industry and individual building owners all have a role to play in shaping the future development of policies, standards and practices for energy benchmarking. Data analysis methods for energy benchmarking, as in all fields, will evolve with these new data sources as well.
Acknowledgements
The author thanks the editor and three anonymous reviewers for excellent comments that considerably strengthened the manuscript and its conclusions. In addition, the author thanks the City of New York Office of Long-Term Planning and Sustainability for providing the New York City benchmarking data. Thanks also to Hilary Beber, Adam Hinge, Donna Hope, Laurie Kerr, Constantine Kontokosta and Alexandra Sullivan for discussions about the data. Also Michael Zatz, Alexandra Sullivan and John Scofield are thanked for discussions about Energy Star. All errors are of course the author's alone.
Funding
This work was partially funded by the US Department of Energy Efficient Buildings HUB [grant number DE-EE0004261].
References
- Allcott, H. (2011). Social norms and energy conservation. Journal of Public Economics, 95(9–10), 1082–1095. doi:10.1016/j.jpubeco.2011.03.003.
- Andaloro, A. P. F., Salomone, R., Ioppolo, G., & Andaloro, L. (2010). Energy certification of buildings: A comparative analysis of progress towards implementation in European countries. Energy Policy, 38(10), 5840–5866. doi:10.1016/j.enpol.2010.05.039.
- Banerjee, A., & Solomon, B. D. (2003). Eco-labeling for energy efficiency and sustainability: A meta-evaluation of US programs. Energy Policy, 31(2), 109–123.
- Barrett, K., & Richard, G. (2009, October). The management challenge of bad data. Governing. Retrieved from http://www.governing.com/columns/smart-mgmt/The-Management-Challenge-of.html
- Blumstein, C., Krieg, B., Schipper, L., & York, C. (1980). Overcoming social and institutional barriers to energy conservation. Energy, 5(4), 355–371.
- Brown, R., Webber, C., & Koomey, J. G. (2002). Status and future directions of the energy star program. Energy, 27(5), 505–520. doi:10.1016/S0360-5442(02)00004-X.
- Burr, A., Keicher, C., & Leipziger, D. (2011). Building Energy Transparency: A Framework for Implementing U.S. Commercial Energy Rating and Disclosure Policy (p. 82). Institute for Market Transformation. Retrieved from http://www.buildingrating.org
- Cater, F. (2010). Critics say LEED program doesn't fulfill promises. NPR.org. Retrieved October 7, 2013, from http://www.npr.org/templates/story/story.php?storyId=129727547
- Chung, W. (2011). Review of building energy-use performance benchmarking methodologies. Applied Energy, 88(5), 1470–1479. doi:10.1016/j.apenergy.2010.11.022.
- City of New York. (2010). PLANYC 2030 - Greener, Greater Buildings Plan. Retrieved December 9, 2010, from http://www.nyc.gov
- City of New York. (2012). New York City Local Law 84 Benchmarking Report (p. 40). City of New York Office of Long-Term Planning and Sustainability. Retrieved from http://on.nyc.gov/Mi5w7K
- City of New York. (2013). New York City Local Law 84 Benchmarking Report. City of New York Office of Long-Term Planning and Sustainability. Retrieved from http://on.nyc.gov/Mi5w7K
- Concerted Action EPBD. (2013). Implementing the Energy Performance of Buildings Directive (EPBD) -- Featuring country reports 2-12. Portuguese Energy Agency (ADENE). Retrieved from http://www.epbd-ca.org/Medias/Pdf/CA3-BOOK-2012-ebook-201310.pdf
- Das, P., & Wiley, J. A. (2014). Determinants of premia for energy-efficient design in the office market. Journal of Property Research, 31(1), 64–86. doi:10.1080/09599916.2013.788543.
- Dasu, T., & Johnson, T. (2003). Exploratory data mining and data cleaning. Hoboken, NJ: John Wiley & Sons.
- De Veaux, R. D., & Hand, D. J. (2005). How to Lie with Bad Data. Statistical Science, 20(3), 231–238. doi:10.1214/088342305000000269.
- Eddy, W. F., & Marton, K. (Eds.). (2012). Effective tracking of building energy use: Improving the commercial buildings and residential energy consumption surveys. Washington, DC: National Academies Press. Retrieved from http://www.nap.edu/catalog.php?record_id=13360
- Eichholtz, P., Kok, N., & Quigley, J. M. (2010). Doing well by doing good? Green office buildings. The American Economic Review, 100(5), 2492–2509. doi:10.2307/41038771.
- Eichholtz, P., Kok, N., & Quigley, J. M. (2013). The economics of green building. Review of Economics and Statistics, 95(1), 50–63. doi:10.1162/REST_a_00291.
- Fisher, C. W., & Kingma, B. R. (2001). Criticality of data quality as exemplified in two disasters. Information & Management, 39(2), 109–116. doi:10.1016/S0378-7206(01)00083-0.
- Fuerst, F., & McAllister, P. (2009). An investigation of the effect of eco-labeling on office occupancy rates. The Journal of Sustainable Real Estate, 1(1), 49–64.
- Fuerst, F., & McAllister, P. (2011a). Eco-labeling in commercial office markets: Do LEED and Energy Star offices obtain multiple premiums? Ecological Economics, 70(6), 1220–1230. doi:10.1016/j.ecolecon.2011.01.026.
- Fuerst, F., & McAllister, P. (2011b). Green noise or green value? Measuring the effects of environmental certification on office values. Real Estate Economics, 39, 45–69.
- Fuerst, F., van de Wetering, J., & Wyatt, P. (2013). Is intrinsic energy efficiency reflected in the pricing of office leases? Building Research & Information, 41(4), 373–383. doi:10.1080/09613218.2013.780229.
- Fung, A., Graham, M., & Weil, D. (2007). Full disclosure: The perils and promise of transparency. Cambridge. New York: Cambridge University Press.
- Gleick, J. (2011). The information: A history, a theory, a flood. New York, NY: Vintage Books.
- Halevy, A., Norvig, P., & Pereira, F. (2009). The unreasonable effectiveness of data. IEEE Intelligent Systems, 24(2), 8–12. doi:10.1109/MIS.2009.36.
- Halpern, S. (2013, November 7). Are we puppets in a wired world?. The New York Review of Books, 60(17). Retrieved from http://www.nybooks.com/issues/2013/nov/07/
- Hey, T., Tansley, S., & Tolle, K. (Eds.). (2009). The fourth paradigm: Data-intensive scientific discovery. Redmond, WA: Microsoft Research.
- Hoffman, S., & Podgurski, A. (2013). Big bad data: Law, public health, and biomedical databases. The Journal of Law, Medicine & Ethics, 41(s1), 56–60.
- Howarth, R. B., & Sanstad, A. H. (1995). Discount rates and energy efficiency. Contemporary Economic Policy, 13(3), 101–109. doi:10.1111/j.1465-7287.1995.tb00726.x.
- Hsu, D. (2014). How much information disclosure of building energy performance is necessary?. Energy Policy, 64, 263–272. doi:10.1016/j.enpol.2013.08.094.
- Kahneman, D. (2011). Thinking, Fast and Slow. New York, NY: Farrar, Straus and Giroux.
- Karr, A. F., Sanil, A. P., & Banks, D. L. (2006). Data quality: A statistical perspective. Statistical Methodology, 3(2), 137–173.
- Karvonen, A. (2013). Towards systemic domestic retrofit: A social practices approach. Building Research & Information, 41(5), 563–574. doi:10.1080/09613218.2013.805298.
- Katz, M. L., & Shapiro, C. (1994). Systems competition and network effects. The Journal of Economic Perspectives, 8(2), 93–115.
- Kohler, N., Steadman, P., & Hassler, U. (2009). Research on the building stock and its applications. Building Research & Information, 37(5-6), 449–454. doi:10.1080/09613210903189384.
- Levine, M. D., Koomey, J. G., McMahon, J. E., & Sanstad, A. H. (1995). Energy efficiency policy and market failures. Annual Review of Energy and the Environment, 20(1), 535–555.
- Lutzenheiser, L. (1993). Social and behavioral aspects of energy use. Annual Review of Energy and the Environment, 18, 247–289.
- Mills, E. (2011). Building commissioning: A golden opportunity for reducing energy costs and greenhouse gas emissions in the United States. Energy Efficiency, 4(2), 145–173. doi:10.1007/s12053-011-9116-8.
- Monts, J. K., & Blissett, M. (1982). Assessing energy efficiency and energy conservation potential among commercial buildings: A statistical approach. Energy, 7(10), 861–869. doi:10.1016/0360-5442(82)90035-4.
- Neely, M. P., & Cook, J. S. (2011). Fifteen years of data and information quality literature: Developing a research agenda for accounting. Journal of Information Systems, 25(1), 79–108. doi:10.2308/jis.2011.25.1.79.
- Nelson, A. C. (2004). Towards a new metropolis: The opportunity to rebuild America. Washington, DC: Brookings Institution.
- Perez-Lombard, L., Ortiz, J., González, R., & Maestre, I. R. (2009). A review of benchmarking, rating and labelling concepts within the framework of building energy certification schemes. Energy and Buildings, 41(3), 272–278.
- Perez-Lombard, L., Ortiz, J., & Pout, C. (2008). A review on buildings energy consumption information. Energy and Buildings, 40(3), 394–398. doi:10.1016/j.enbuild.2007.03.007.
- Retroficiency. (2013). Building Energy Efficiency Opportunity Report.
- Sanchez, M. C., Brown, R. E., Webber, C., & Homan, G. K. (2008). Savings estimates for the United States environmental protection agency's ENERGY STAR voluntary product labeling program. Energy Policy, 36(6), 2098–2108.
- Schweber, L., & Leiringer, R. (2012). Beyond the technical: a snapshot of energy and buildings research. Building Research & Information, 40(4), 481–492. doi:10.1080/09613218.2012.675713.
- Sharp, T. (1996). Energy benchmarking in commercial office buildings. In Proceedings of the ACEEE (pp. 321–329).
- Sunstein, C. R. (2013). Simpler: The future of government. New York, NY: Simon and Schuster.
- US Energy Information Administration. (2014). How will buildings be selected for the 2012 CBECS? Retrieved from http://www.eia.gov/consumption/commercial/2012-cbecs-building-sampling.cfm
- US Environmental Protection Agency. (2007). Energy star performance ratings: Technical methodology for office, bank/financial institution, and courthouse (p. 14). Washington, DC: U.S. Environmental Protection Agency. Retrieved from http://1.usa.gov/1joZdQ3
- US Environmental Protection Agency. (2009). Celebrating a decade of energy star buildings, 1999-2009. Washington, DC: U.S. Environmental Protection Agency. Retrieved from http://www.energystar.gov/buildings/sites/default/uploads/tools/Decade_of_Energy_Star.pdf?3a75-f8f9
- US Environmental Protection Agency. (2013). Energy Star Portfolio Manager Technical Reference. Washington, DC: U.S. Environmental Protection Agency. Retrieved from http://www.energystar.gov/buildings/tools-and-resources/portfolio-manager-technical-reference-energy-star-score
- Wang, R. Y., Storey, V. C., & Firth, C. P. (1995). A framework for analysis of data quality research. Knowledge and Data Engineering, IEEE Transactions on, 7(4), 623–640.
- Weil, D., Fung, A., Graham, M., & Fagotto, E. (2006). The effectiveness of regulatory disclosure policies. Journal of Policy Analysis and Management, 25(1), 155–181. doi:10.1002/pam.20160.
- Wiley, J. A., Benefield, J. D., & Johnson, K. H. (2010). Green design and the market for commercial office space. The Journal of Real Estate Finance and Economics, 41(2), 228–243. doi:10.1007/s11146-008-9142-2.
- Zatz, M. (2013, July 15). How many buildings are in Portfolio Manager?