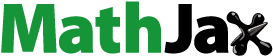
Abstract
In this study, we examine the relationship between financial literacy and bank financial reporting transparency for a sample of banks from the U.S. Following prior literature, we employ discretionary loan loss provisions (DLLP) as our primary measure of bank reporting transparency. We argue that the financial literacy of their customers can influence bank managers’ behaviors with respect to both the mechanics of the loan loss provisioning and their opportunistic actions. Financially literate customers represent more stable sources of funding and have more predictable loan loss provisioning that contributes to more persistent earnings. Financial literacy could also enhance customers’ ability to indirectly follow and monitor bank performance and risk-taking. Therefore, bank managers will be less likely to engage in opportunistic earnings manipulation. Following these arguments, we predict that citizens’ financial literacy is positively associated with bank financial reporting transparency. Consistent with our prediction, we find that the magnitude of bank DLLP is negatively related to state-level financial literacy. Moreover, the association between financial literacy and DLLP is higher for banks with more retail deposits and larger consumer loans, the two channels through which financial literacy could influence bank transparency.
1. Introduction
The global financial crisis following the 2007 subprime meltdown stimulated governments’ interest in improving their citizens’ financial literacy. The Organization for Economic Co-operation and Development (OECD, Citation2013, p. 144) defines financial literacy as the ‘knowledge and understanding of financial concepts and risks, and the skills, motivation and confidence to apply such knowledge and understanding in order to make effective decisions across a range of financial contexts, to improve the financial well-being of individuals and society.’ The rising interest in financial literacy stems from increased individual responsibility (due to factors such as longer life expectancy, higher costs of education, and reductions in state-supported pensions and healthcare benefits) and an increased supply of, and demand for, financial products and services (OECD, 2013). In addition, a lack of financial literacy contributes to poor financial decision-making, which could have devastating consequences, such as personal bankruptcy and market collapse. Consequently, financial literacy is now recognized as an essential element of the policy mix for financial stability. However, OECD (Citation2018) has emphasized that financial education can change the behavior of individuals, but it may not be significant enough to have macro-level implications. Therefore, in this research, we examine one of the broader economic implications of financial literacy: the relationship between citizens’ financial literacy and financial reporting practices in the banking industry.
We argue that financial literacy can influence bank transparency through retail deposits and consumer loans. In particular, retail deposits from financially literate customers are a more stable source of funding for banks. For example, Kim (Citation2016) observes that financially literate depositors make fewer withdrawals after banks receive an enforcement action, implying that financial literacy can mitigate cognitive biases in depositor behavior during bank runs. Customers obtain loans from banks, including mortgages, auto loans, student loans, and credit cards, for individual or family needs. We argue that the expected losses of loans made to financially literate customers tend to be smaller and more predictable, as they have more definitive plans for borrowing and repayment than the expected losses on loans made to less financially literate customers. Lusardi and Tufano (Citation2015) find that individuals who are financially illiterate tend to take on excessive amounts of debt or are unsure of the appropriateness of their debt position. Therefore, when loans are made to financially literate borrowers, the losses are lower and are more predictable. Consequently, banks will experience fewer abnormal delinquencies and lower earnings uncertainty. Additionally, financial literacy could also enhance customers’ ability to indirectly follow and monitor bank performance and risk-taking. Financially literate customers are more likely to read and understand financial news and analyst reports, which may disclose additional information. Therefore, bank managers will be less likely to engage in opportunistic earnings manipulation.
To test our hypothesis, we use a sample that includes 744 individual U.S. banks with 4,825 bank-year observations, covering the post-crisis period of 2009–2019. We obtain banks’ financial data from the Compustat Bank Database and financial literacy data from the 2009, 2012, 2015, and 2018 National Financial Capability Study (NFCS) State-by-State Surveys, each of which consists of nationwide online surveys of more than 25,000 American adults (https://www.usfinancialcapability.org). In measuring the financial literacy of Americans in each state, the surveys in Appendix A include five questions about financial concepts to test respondents’ understanding of the interest rate, inflation, risk diversification, bond, and mortgage. We construct the index of statewide financial literacy (FINLIT) by calculating the average ratio of correct responses to the five financial literacy questions for each state for the years 2009, 2012, 2015, and 2018. Finally, we use multiple imputations to fill in the missing data for the years 2010–2011, 2013–2014, and 2016–2017.Footnote1
We use the magnitude of discretionary loan loss provision (DLLP) as our primary measure of bank transparency. Prior literature has employed DLLP as a measure of earnings quality. For example, Kanagaretnam et al. (Citation2010) document that auditor-fee dependence is associated with earnings management via DLLP. Beatty and Liao (Citation2014) find that banks with a greater magnitude of DLLP tend to file more earnings restatements. Our empirical tests show that the financial literacy index FINLIT is negatively and significantly associated with the absolute value of DLLP (ABSDLLP). In terms of economic significance, a one-standard deviation increase in FINLIT is associated with a 13.5% decrease in Kanagaretnam et al.’s (Citation2010) measure of ABSDLLP and a 16.2% decrease in Beatty and Liao’s (Citation2014) measure of ABSDLLP. These results suggest that citizens’ financial literacy negatively influences bank managers’ discretion to manage earnings. The results remain robust when we employ statewide post-secondary education status as our instrumental variable in a two-stage least squares (2SLS) regression analysis to mitigate potential endogeneity concerns. Our results also remain robust to the exclusion of banks with potential M&A activities and various alternative measures of ABSDLLP, including FINLIT in the estimation of DLLP, and employing signed DLLP. Collectively, our results indicate that financial literacy is positively related to the financial reporting transparency of banks.
Next, we examine the influence of retail deposits and the level of consumer loans, the two specific channels through which financial literacy could influence bank transparency. We find that, when banks have more retail deposits, the attenuating effect of financial literacy on ABSDLLP is more pronounced. The results suggest that, with retail deposits, financial literacy can translate into higher bank transparency. Similarly, we document that the moderating effect of financial literacy on ABSDLLP is more pronounced with higher levels of consumer loans. This is consistent with financially literate borrowers having more predictable loan losses that contribute to less estimation errors in loan loss provisioning.
Our study makes three important contributions. The first contribution is that we direct the financial literacy research from individual and household behaviors to the practices of the banking sector. Arguably, citizens’ financial literacy can support financial and economic stability by strengthening societal resilience to major financial shocks, such as the subprime meltdown (OECD, Citation2018). However, empirical evidence on how this process manifests itself is sparse. For example, the literature is unclear on the influence of financial literacy on the banking industry. Our research indicates that bank earnings quality improves as banks have a larger base of financially literate customers.
The second contribution is that our study provides initial evidence in line with the rising interests in financial literacy policies. Since the 2007–2008 financial crisis, governments have emphasized financial literacy in supporting inclusive and sustainable growth. For instance, on July 15, 2019, the U.S. Department of the Treasury released a report titled ‘Federal Financial Literacy Reform: Coordinating and Improving Financial Literacy Efforts,’ which highlights the importance of financial literacy for Americans, identifies ways to deliver financial education effectively and efficiently, and recommends federal action to improve financial capability for consumers and communities (U.S. Department of the Treasury, Citation2019). If citizens’ financial literacy improves following federal initiatives, their financial behaviors should become more stable and predictable, as suggested by our findings.
Finally, our paper contributes to the literature on understanding the accruals process of loan loss provisioning. Although prior research documents that earnings variability and estimation errors matter for loan loss provisioning, our results provide insight into how this accruals process is influenced by different underlying customer pools. Moreover, our research is also related to the determinants of loan loss provisions. Prior literature documents that regulation, culture, social capital, and policy uncertainty, among other factors, play an important role in determining bank loan loss provisions (e.g. Fonseca & Gonzalez, Citation2008; Jin et al., Citation2019; Kanagaretnam et al., Citation2011). As a supplemental contribution to prior literature, our paper finds that citizens’ financial literacy is an important factor that influences loan loss provisions and bank earnings quality.
The rest of this paper is organized as follows. Section 2 reviews the literature and develops our hypotheses on the relationship between financial literacy and bank accounting discretion. Section 3 describes our data and research design. Section 4 presents our empirical results, and Section 5 reports the results of additional analyses. Section 6 discusses robustness checks, and Section 7 concludes our paper.
2. Literature Review and Hypotheses Development
The literature on financial literacy has focused mainly on the relationships between financial literacy and positive behavioral changes in individuals and households. Research shows that individuals who are financially literate are more likely to save and plan for retirement (Cole et al., Citation2011; Lusardi & Mitchell, Citation2011a, Citation2011b) and to accumulate greater wealth (Stango & Zinman, Citation2009). Calvet et al. (Citation2009) document that financially educated households are less prone to making financial mistakes than other households. Hastings and Tejeda-Ashton (Citation2008) find that individuals who are financially literate are much more likely to choose mutual funds with lower fees; Van Rooij et al. (Citation2011) find that the financially literate are more active investors in the stock market and have better-performing portfolios. Hsiao and Tsai (Citation2018) demonstrate that financial literacy brings a significant benefit to individuals by lowering their entry barriers to the purchase of complex derivative products such as options. Financial literacy also has implications for borrowing and choice of debt. Gerardi et al. (Citation2013) show that, during the 2008 financial crisis, individuals with limited financial knowledge and numerical ability were more likely to default on subprime mortgages. According to Lusardi and Tufano (Citation2015), individuals who are less financially literate tend to incur higher fees and take out higher-interest loans.
Bank financial reporting is important for regulatory and investment purposes. Barth and Landsman (Citation2010) argue that bank regulators use the information supplied in bank financial statements as inputs to calculate regulatory capital measures and enhance financial stability. In addition, shareholders/investors may rely on banks’ financial information to trade banks’ stocks. On the other hand, opacity in bank reporting aids bank managers’ expropriation of resources for self-interest. For example, bank managers may increase provisions in good years and decrease them in bad years to smooth income and mask the underlying riskiness.
We argue that the financial literacy of their customers can influence bank managers’ behaviors with respect to both the mechanics of the accrual process and their opportunistic actions.Footnote2 On the one hand, the mechanics of the accrual process are improved with fewer estimation errors and low variability. Because financially literate depositors are less likely to panic during times of uncertainty (Kim, Citation2016), they represent more stable funding, through which banks can generate more persistent earnings. In addition, the borrowing and repayment behaviors of financially literate borrowers are typically more predictable, and the low variation in risk will reduce provisioning and accruals errors for banks (Lusardi & Tufano, Citation2015).
On the other hand, to the extent that financially literate depositors could be involved in monitoring banks’ financial reporting process and risk-taking, bank managers will be less likely to engage in opportunistic earnings manipulation. Scandals about bank underperformance and risk often lead to media coverage. Financially literate customers are more likely to read and understand financial news and analyst reports, which may disclose additional information. Thus, financially literate individuals can take corresponding actions (e.g. withdraw their deposits) and exercise their monitoring function on banks even if they may not directly read bank financial statements per se to access bank financial condition. Taken together, our hypothesis on the relationship between citizens’ financial literacy and bank financial reporting transparency is as follows:
H1: Citizens’ financial literacy is positively associated with bank financial reporting transparency.
In developing the above hypothesis, we relied on the argument that retail deposits and consumer loans are two important channels through which the financial literacy of retail depositors and borrowers of consumer loans may relate to bank transparency. Retail deposits from financially literate customers are a more stable source of funding for banks. For example, Kim (Citation2016) provides evidence that financial literacy mitigates biases in depositor behavior during bank runs following the issuance of FDIC enforcement actions. He finds that the financial literacy of respondents in nearby branches of banks receiving an enforcement action significantly reduces deposit outflows. When banks have greater funding stability to generate persistent earnings and cash flows, there are fewer incentives for them to engage in earnings management (Jin et al., Citation2018).
Conversely, Maechler and McDill (Citation2006) argue that depositors can monitor banks for poor performance or excessive risk-taking by reducing deposits or demanding a higher risk premium. An extreme form of monitoring by depositors can even lead to bank runs. We argue that, although retail depositors may not directly monitor bank financial performance by going through bank financial statements per se, financially literate depositors could be more likely to search for financial news, which may disclose certain information about their banks. As such, depositors can take corresponding actions by either withdrawing their deposits from banks or demanding higher deposit rates.
At the same time, banks extend personal credit in the form of consumer loans for personal or family use. These loans include mortgages, auto loans, student loans, and credit cards. We argue that the expected losses of loans borrowed by financially literate customers tend to be more predictable, as they have more definitive plans for borrowing and repayment. When loans are made to financially literate borrowers, the deviation of delinquency from expected loan losses is smaller, meaning banks will experience fewer abnormal loan losses and therefore exhibit higher earnings transparency.Footnote3
In our context, when exposed to higher retail deposits and consumer loans, banks will interact more with retail depositors and individual loan borrowers; thus, bank behaviors will be more subject to the influence of these customers. Therefore, we would expect greater bank earnings transparency when banks have more retail deposits and higher consumer loans from financially literate customers. Thus, our second and third hypotheses are as follows:
H2: The association of financial literacy with bank financial reporting transparency is stronger when banks have more retail deposits.
H3: The association of financial literacy with bank financial reporting transparency is stronger when banks have more consumer loans.
3. Data and Research Design
To test our hypotheses, we gather data on bank financial information and citizens’ financial literacy. We obtain bank-level data from the Bank Compustat Database. As the proxy for financial literacy, we use the annual statewide financial literacy index constructed from the NFCS surveys. A higher value of FINLIT indicates customers who are more financially literate. Our financial literacy data come from the 2009, 2012, 2015, and 2018 National Financial Capability Study (NFCS) State-by-State Surveys, which were nationwide online surveys of more than 25,000 American adults (https://www.usfinancialcapability.org). The research objectives of the NFCS are to benchmark key indicators of financial capability and evaluate how these indicators vary with underlying demographic, behavioral, attitudinal, and financial literacy characteristics. The NFCS data have been widely used by prior economics and business studies on financial literacy. For example, Kim and Lee (Citation2018) investigate the relationship between financial literacy and the use of payday loans. Kim et al. (Citation2020) study financial literacy in relation to mortgage repayment delinquency using the 2015 NFCS dataset. In measuring financial knowledge, the surveys include five financial literacy questions (please see Appendix A) to gauge respondents’ knowledge of these terms: interest rate, inflation, risk diversification, bond, and mortgage. For each state, we calculate the average ratio of correct responses to each of these five questions for the years 2009, 2012, 2015, and 2018. Then we use multiple imputations to fill in the years 2010–2011, 2013–2014, and 2016–2017 to construct annual statewide citizens’ financial literacy indices.Footnote4
Bank reporting transparency derives from how closely a bank’s true underlying fundamentals map into reported accounting numbers (Bushman, Citation2016). Following prior literature (e.g. Jiang et al., Citation2016), we focus on loan loss provisions because loan loss provisioning is an important accounting policy choice that directly influences the volatility of bank earnings, as well as the information properties of banks’ financial reports reflecting the risk attributes of loan portfolios (Bushman, Citation2016). To measure bank accounting discretion, we focus on the magnitude of DLLP, through which banks can manipulate both earnings and regulatory capital. Loan loss provision (LLP) is an expense item for banks, representing banks’ current estimates of future losses from defaults on outstanding loans (Cohen et al., Citation2014). LLP is the largest accrual in bank accounting, thereby affording bank managers wide latitude for potential manipulation (Beatty & Liao, Citation2014). There are three important ways to manipulate DLLP to reduce earnings quality. First, banks can smooth their earnings by decreasing DLLP when income is too low and increasing DLLP when income is too high (Fonseca & Gonzalez, Citation2008). Second, bank managers can manage banks’ regulatory capital through DLLP. Banks with low regulatory capital may intentionally decrease their LLP because each one-dollar decrease in LLP increases Tier 1 capital by one dollar times (1-tax rate) given the loan loss allowance is not added back to Tier 1 capital (Beatty & Liao, Citation2014). Third, DLLP can reflect the timely recognition of expected future loan losses (Bushman & Williams, Citation2012). DLLP measures the extent to which banks deviate from their normal LLP level to manage earnings; therefore, it is widely used in prior studies as a measure of bank transparency. For example, Kanagaretnam et al. (Citation2010) find that auditor fee dependence is associated with earnings management via DLLP. Beatty and Liao (Citation2014) find that banks with a greater magnitude of DLLP tend to file more earnings restatements and receive more comment letters from the U.S. Securities and Exchange Commission (SEC).
We proxy for DLLP as the residual from the regression of LLP using Model (1a), which is a modified version of Kanagaretnam et al.’s (Citation2010) model.Footnote5,Footnote6 The residual captures a bank’s deviation from the normal level of LLP, thereby measuring DLLP.
(1a)
(1a)
where LLP is loan loss provision divided by lagged total loans; LLA is loan loss allowance divided by total loans; NPL is nonperforming loans divided by lagged total loans; LOAN is total loans divided by total assets; ΔLOAN is change in total loans divided by lagged total loans; CHO is loan charge-offs divided by lagged total loans; ΔNPL is change in nonperforming loans divided by lagged total loans; ΔGDP is change in state GDP over the year; ΔUNEMP is change in the state unemployment rate over the year; and ΔHPI is the change in the state house price index over the year. We include lagged LLA to account for the fact that banks previously allowing for higher expected loan losses will typically recognize less provision in the current year. CHO is included because loan charge-offs influence the expectation of collecting current loans and, hence, current LLP. We also include the loan growth rate because fast-growing loans might be associated with a decrease in loan quality. YEAR_FIXED_EFFECTS are year dummy variables to account for year fixed effects.
To confirm that our test results are not driven by choice of a specific loan loss provision estimation model, we employ an alternative specification used by Beatty and Liao (Citation2014) to estimate DLLP. As before, we measure DLLP using the residual term of Model (1b).
(1b)
(1b)
where SIZE is the natural logarithm of total assets. This model allows for changes in nonperforming loans in consecutive periods
,
,
, and
, as banks might use current, future, and historical information on nonperforming loans to select LLP. The model also includes bank size (SIZE) because official supervisory oversight could vary with bank size. Basu et al. (Citation2020) suggest that loan charge-offs should also be included in the linear model of LLP after comparing four potential models proposed by Beatty and Liao (Citation2014). They find that loan charge-offs are associated with declines in nonperforming loans and increases in LLP, inducing a V-shaped relationship between LLP and change in nonperforming loans. They indicate that ‘failure to model the asymmetry attributable to loan charge-offs can change inferences about the presence of earnings management and the effects of delayed loan loss recognition in prior papers that assumed linearity.’ Thus, we control for loan charge-offs (CHO) in the first-stage LLP estimation model.Footnote7
We use the absolute value of DLLP estimated from Models (1a) and (1b), ABSDLLP_A and ABSDLLP_B, as our main proxies for bank accounting discretion. Higher values of ABSDLLP_A and ABSDLLP_B indicate greater accounting discretion and lower transparency. To test the influence of citizens’ financial literacy on financial reporting transparency, we estimate the following regression models.
(2)
(2)
ABSDLLP is either ABSDLLP_A or ABSDLLP_B. Our primary variable of interest is the statewide citizens’ financial literacy index, FINLIT, computed from the NFCS Surveys. Based on our expectation that customers’ financial literacy reduces average bank reporting discretion, we predict FINLIT to be negatively and significantly associated with ABSDLLP_A and ABSDLLP_B. V is an array of financial variables to control for bank characteristics that vary with time. Following Jiang et al. (Citation2016) and Jin et al. (Citation2019), we include bank size (SIZE), capital ratio (CAPR), earnings before loan loss provisions (EBP), and assets growth rate (ASG).Footnote8 We control for bank risk, which is measured by Z-score (ZSCORE) as defined by Kanagaretnam et al. (Citation2014). Z-score is a measure of bank stability that indicates the distance from insolvency (Laeven & Levine, Citation2009). We calculate ZSCORE as the natural logarithm of (EBP + CAPR)/σ(EBP), where EBP is the mean of earnings before loan loss provisions divided by total assets over the sample period; CAPR is the mean of total equity divided by total assets over the sample period, and σ(EBP) is the standard deviation of EBP over the sample period. We multiply the score by −1 so that a higher ZSCORE implies more risk. W is an array of state characteristics, including change in per capita GDP (ΔGDP), change in the unemployment rate (ΔUNEMP), and change in the house price index (ΔHPI). In addition, we control for bank fixed effects and year fixed effects. As before, to account for the possibility that the error terms might be correlated, we cluster the standard errors at the state level.
To test H2 and H3 on the influence of retail deposits (DEPOSIT) and consumer loans (CLOAN), we interact them with FINLIT and include the interaction terms in Models (3) and (4), respectively.
(3)
(3)
(4)
(4)
where DEPOSIT is defined as the decile rank of retail deposits, and CLOAN is defined as the decile rank of consumer loans.Footnote9 ABSDLLP, FINLIT, V, and W are defined in the same way as in Model (2). To the extent that the influence of financial literacy on earnings quality is greater for banks with more exposure to retail deposits and consumer loans, we would expect the interaction terms FINLIT*DEPOSIT and FINLIT*CLOAN to be negatively and significantly associated with ABSDLLP.
4. Empirical Results
We present the descriptive statistics for the variables used in the regressions in . After deleting observations with insufficient data, we have a total of 744 unique U.S. banks with 4,788 bank-year observations covering the post-financial crisis period from 2009 to 2019. During the sample period, the mean values of ABSDLLP_A and ABSDLLP_B are both 0.002, while the median values are 0.001. In comparison, the mean and median values of LLP are 0.006 and 0.003, respectively. The financial literacy variable FINLIT has a mean value of 0.596 and a median value of 0.592, suggesting that the average correct response to financial concept questions by U.S. adults is about 60%. As for the control variables, we find that the average capital ratio (CAPR) of U.S. banks is 10.4% and the average asset growth rate (ASG) is about 7.6%. Descriptive statistics also show that the U.S. experienced a growth in GDP, a decline in the unemployment rate, and an increase in housing prices during the sample period.
Table 1. Descriptive statistics.
We report the Pearson correlations of the variables in . FINLIT is significantly and negatively correlated with ABSDLLP_A and ABSDLLP_B at the 1% level, indicating that statewide financial literacy is associated with less accounting discretion via DLLP. Moreover, we find that ABSDLLP_A and ABSDLLP_B are significantly correlated with each other and with all other selected variables at the 1% level.
Table 2. Pearson correlation Matrix.
Table 3. Estimation of discretionary loan loss provisions.
We present the OLS regression results of DLLP estimation in , with Column 1 displaying Kanagaretnam et al.’s (Citation2010) model and Column 2 showing Beatty and Liao’s (Citation2014) model. Most of the estimated coefficients are consistent with those reported by previous studies (e.g. Beatty & Liao, Citation2014; Bushman & Williams, Citation2015; Kanagaretnam et al., Citation2010). Column 1 shows that is significantly and negatively associated with
at the 1% level (t-value = −11.50), consistent with the argument that banks recognize less LLP in the current period if they have already had a high beginning loan loss allowance.
is significantly and positively associated with
at the 5% level (t-value = 2.23), meaning that a larger amount of loans will also require a higher level of loan loss provisions.
is also significantly and positively associated with
at the 1% level (t-value = 48.40), consistent with the argument that current loan charge-offs can influence expectations of the collectability of current loans and, thus, current LLP (Beaver & Engel, Citation1996). In addition,
has a positive and significant relationship with
at the 1% level (t-value = 8.57), implying that an increase in nonperforming loans requires more LLP in the current period. Column 2 reports positive and significant coefficients for
,
,
, and
, suggesting that banks incorporate both current, future, and past information on the change in nonperforming loans to estimate LLP.
has a positive and significant coefficient at the 1% level (t-value = 4.70), indicating that the estimation of LLP depends on the quality of incremental loans. The absolute values of the residuals from Models (1) and (2) are denoted as ABSDLLP_A and ABSDLLP_B, respectively, and are our main proxies for bank reporting transparency.
We report the univariate comparisons of ABSDLLP_A and ABSDLLP_B between high and low FINLIT states in . The mean values of ABSDLLP_A and ABSDLLP_B are 0.0021 and 0.0022 for banks in below-median FINLIT states, which are higher than the mean value of 0.0018 for banks in above-median FINLIT states. The difference is statistically significant at the 1% level. Similar patterns can also be seen in the median values of ABSDLLP_A and ABSDLLP_B, with banks in low FINLIT states reporting significantly more discretionary loan loss provision than their peers in high FINLIT states.
Table 4. Univariate tests.
presents the baseline OLS multivariate regression results for testing Hypothesis 1 on the relationship between financial literacy and discretionary loan loss provision. After controlling for state and year fixed effects, we find that FINLIT is negatively and significantly related with ABSDLLP_A and ABSDLLP_B at the 1% level (t-value = −4.66 and −5.73, respectively) in Columns 1 and 2. After controlling for bank and year fixed effects, we find that FINLIT is negatively and significantly related with ABSDLLP_A and ABSDLLP_B at the 1% level (t-value = −5.72 and −6.21, respectively) in Columns 3 and 4.Footnote10 In terms of economic significance, a one standard deviation increase in FINLIT is associated with a 13.5% decrease in ABSDLLP_A. Specifically, this impact is computed as −0.010 (the coefficient of FINLIT in Column 1 of ) × 0.027 (the sample standard deviation of FINLIT in ) ÷ 0.002 (the sample mean of ABSDLLP_A in ) × 100%. Meanwhile, a one standard deviation increase in FINLIT is associated with a 16.2% decrease in ABSDLLP_B. These results support our hypothesis that financial literacy is associated with less accounting discretion. As for the control variables, we find that ABSDLLP is significantly and negatively associated with EBP and ΔHPI, indicating that banks engage in less accruals manipulation in times of high earnings and high housing price growth.
Table 5. Financial literacy and discretionary loan loss provisions.
Although we have accounted for a variety of control variables in the baseline OLS regressions, our model specification may still suffer from endogeneity issues due to unobservable characteristics. To mitigate the endogeneity concerns, we employ the two-stage least squares (2SLS) instrumental variable approach to verify the robustness of our baseline results.
Prior literature notes that personal financial literacy is influenced by educational attainment (Lusardi & Mitchell, Citation2011b, Citation2011c; Stolper & Walter, Citation2017). For example, Lusardi and Mitchell (Citation2011b) find that in the U.S. 63% of respondents with at least a college degree can correctly answer basic questions about financial literacy, but only 12.6% of respondents without a college degree can. We argue that the education level of the NSCF survey takers can be viewed as an exogenous variable, given that this educational attainment must have been accumulated over a longer period preceding the survey. Furthermore, Lusardi et al. (Citation2010) find that educational attainment is a relevant factor even after controlling for cognitive abilities. Following this line of reasoning, we use educational attainment as our instrumental variable for financial literacy. In other words, education is represented by the NFCS survey respondents’ college status, a dummy variable set at 1 if the survey respondent is at least a college graduate, and 0 otherwise (data source: 2009, 2012, 2015, and 2018 NFCS State-by-State Surveys). We then use COLLEGE to represent the average value of the NFCS survey respondents’ college status dummy variable for each state-year. We expect COLLEGE to have a positive and significant relationship with FINLIT in Model (5). The control variables in Model (5) are an array of state-level variables together with state and year fixed effects.
(5)
(5)
where COLLEGE is the proportion of the population receiving post-secondary education of each state, SIZE is the natural logarithm of total assets, CAPR is the total equity divided by total assets, EBP is the earnings before LLP divided by assets, ASG is the change in assets divided by lagged assets, and ZSCORE is the natural logarithm of (EBP + CAPR)/σ(EBP) multiplied by −1. The remaining variables are defined in Appendix B.
We estimate the first-stage regression using Model (5) to predict the value of FINLIT, and use the predicted value of FINLIT from the first stage to test the relationship between citizens’ financial literacy levels and bank accounting discretion in the second stage in Model (6).
(6)
(6)
where PRE_FINLIT is the predicted value of FINLIT from Model (5).
We report the 2SLS instrumental variable regression results in . Panel A tabulates the results for the first-stage regression using Model (5). Panel B tabulates the results for the second-stage regression using Models (4a) and (4b). In the first-stage regression, we find that COLLEGE is significantly and positively associated with FINLIT at the 1% level (t-value = 3.20), implying that individuals who have received at least a college education are more financially literate than individuals without a college education. The F-statistic is 15.828 at the 1% level, rejecting the null hypothesis that COLLEGE is a weak instrument for FINLIT. In the second stage, we find that PRE_FINLIT, the predicted value of FINLIT, is negatively and significantly correlated with ABSDLLP_A and ABSDLLP_B at the 1% level (t-value = −6.57 and −6.94, respectively). The 2SLS instrumental variable analysis supports our baseline regression results that citizens’ financial literacy constrains bank accounting discretion.
Table 6. Instrumental variable analysis of financial literacy and discretionary loan loss provisions.
In Hypotheses 2 and 3, we conjecture that retail deposits and consumer loans are two potential channels through which citizens’ financial literacy could influence bank accounting discretion. When a bank has a larger depositor base that possesses more advanced financial knowledge, its stability of cash flows will be enhanced, and the scrutiny it receives from its customers will be more intense. We report our test results for the retail deposits channel in . We find that the coefficient of FINLIT*DEPOSIT is negative and significant at the 1% and 5% level (t-value = −3.16 and −2.24, respectively), suggesting that financial literacy has a greater influence on bank accounting discretion when banks have more deposit funding. This result supports our argument that financial literacy relates to bank earning quality through retail deposits.
Table 7. Financial literacy, retail deposits, and discretionary loan loss provisions.
Moreover, financially literate customers tend to have more definitive plans for their bank borrowings and repayments, so their abnormal delinquency is smaller, resulting in fewer loan defaults. We report the results of this test on consumer loans channel in . As predicted, the coefficient of FINLIT*CLOAN is significantly negative at the 1% and 5% levels (t-value = −2.96 and −2.34, respectively), implying financial literacy has a greater influence on bank transparency when banks have a larger exposure to consumer loans.
Table 8. Financial literacy, consumer loans, and discretionary loan loss provisions.
5. Additional Analyses
We argue that retail depositors may not directly monitor bank financial performance by going through bank financial statements per se, but financially literate customers could be more likely to search for media coverage of banks. Specifically, we measure citizens’ search for media coverage of bank-related information (GOOGLE) using Google Trends, which count bank-related keywords for each state-year. Bank-related keywords include ‘bank,’ ‘Federal Reserve,’ and ‘economic’ or ‘economy.’Footnote11 In the first stage, we regress the Google search for media coverage of bank information (GOOGLE) on financial literacy (FINLIT) at the state-year level. If financially literate customers are more likely to read bank news, we would expect a significantly positive relationship between GOOGLE and FINLIT. In the second stage, we interact FINLIT with GOOGLE and include both variables and their interaction term in the regression model of ABSDLLP. If greater attention to media coverage of bank information does not enhance financially literate depositors’ monitoring capacity, we would expect the interaction term FINLIT *GOOGLE to be insignificant with ABSDLLP.
(7)
(7)
where GOOGLE is the index of Google Trends searches of bank-related keywords (including ‘bank,’ ‘Federal Reserve,’ and ‘economic’ or ‘economy’) for each state-year divided by 100. All control variables in Model (7) are aggregated at the state-level together with state and year fixed effects.
(8)
(8)
Variables ABSDLLP, FINLIT, V, and W are defined in the same way as in Model (2).
We report the results for regression Model (7) in Panel A of and the results for Model (8) in Panel B of . In Panel A, we find that GOOGLE is positively and significantly associated with FINLIT at the 1% level (t-value = 3.25), suggesting that citizens with higher financially literacy tend to search for more bank-related news via Google. In Panel B, we find that both FINLIT and GOOGLE have a significantly negative relationship with ABSDLLP. However, we do not observe any significance with the interaction term , implying that greater attention to media coverage of bank information does not directly relate to financially literate depositors’ monitoring.
Table 9. Financial literacy, google search of bank-related information, and discretionary loan loss provisions.
In our main regression model, we focus on the discretionary component of LLP because non-discretionary LLP is required, and only discretionary LLP is subject to managerial discretion/manipulation. In a recent study, Chen et al. (Citation2018) discuss the measurement error and inference issues that arise with a two-stage model in this type of estimation of discretionary accruals. Thus, in an additional test, we regress LLP on FINLIT and all the relevant controls in a single model. We estimate the following regressions models:
(9)
(9)
The results in Column 1 of show that there is a significant and negative correlation between LLP and FINLIT at the 10% level (t-value = −1.76), suggesting that financial literacy decreases banks’ overall provision for loan losses. For the control variables, we find that bank size (SIZE) is significantly and positively associated with LLP, indicating that larger banks tend to recognize more loan losses. We also find a significantly negative coefficient of ASG, implying that high growth banks are associated with lower loan loss provisions. The coefficient of ZSCORE is positive and significant at the 1% level, indicating that banks with high insolvency risks tend to recognize more loan loss provisions.
Table 10. Financial literacy, loan loss provisions, and income-increasing/decreasing discretionary loan loss provisions.
Depositors would be more concerned about income-increasing provisions, as bank managers have the tendency/incentives to overstate earnings. We identify income-increasing DLLP (i.e. DLLP < 0) and income-decreasing DLLP (i.e. DLLP > 0) from these estimation models and use their absolute values as additional measures of earnings opacity. In a robustness test, we use the absolute value of income-increasing DLLP (ABS_II_DLLP) and income-decreasing DLLP (ABS_ID_DLLP) as two additional measures of bank opacity. The results in Columns 2–5 of show that both ABS_II_DLLP and ABS_ID_DLLP are significantly and negatively associated with FINLIT at the 1% level, consistent with the notion that financial literacy reduces the likelihood of earnings management through DLLP.
6. Robustness Checks
We are interested in examining whether the influence of FINLIT is robust to the inclusion of bank characteristics that would reasonably indicate corporate governance or high stakeholder pressure. Thus, we include two governance variables (i.e. board size and proportion of independent directors) in our regressions as a robustness check. Large boards of directors can commit more time and effort to oversee management (Anderson et al., Citation2004). Independent directors demand more transparent information to perform their monitoring and advising roles (Armstrong et al., Citation2014). Board size (BRDSIZE) is measured by the number of board directors. The independent director ratio (INDDIR) is measured by the number of independent directors divided by the total number of directors. We collect the governance variables (i.e. number of board directors, number of independent directors) from the institutional shareholder services (ISS) database. The results reported in Online Appendix 1 show that FINLIT is significantly and negatively associated with ABSDLLP_A and ABSDLLP_B at the 1% level, even after controlling for corporate governance variables (BRDSIZE and INDDIR).
Kanagaretnam et al. (Citation2010) find that auditor industry specialization constrains earnings management in an international setting. We also include auditor industry specialization (AUDIT) to control for the effect of audit quality on bank transparency. Francis and Wang (Citation2021) document that PwC is a common auditor in bank loans—more common than all the other Big 4 audit firms combined. Hence, we define AUDIT as a dummy variable that equals one for a bank audited by PwC and zero otherwise. Consistent with prior literature, the untabulated results show that AUDIT is significantly and negatively associated with ABSDLLP_A and ABSDLLP_B, suggesting that auditor industry specialization constrains bank earnings management. We continue to report a significantly negative association between ABSDLLP and FINLIT even after controlling for audit quality. Prior research shows that social capital, which reflects cooperative norms in society, acts as an informal monitoring mechanism, reduces opportunistic behavior, and increases bank accounting quality (Jha & Chen, Citation2015). We control for social capital, and the untabulated results show that FINLIT continues to have a significant and negative association with ABSDLLP.
One may be concerned that our results are driven by the external regulatory environment. Large banks are subject to greater government regulations and receive more public attention. For instance, the Federal Deposit Insurance Corporation Improvement Act (FDICIA) of 1991 includes strict requirements for annual audit and management’s assessment of the effectiveness of internal control for banks with more than $1 billion (prior to 2005, more than $500 million) in assets. We argue that, due to greater visibility, FDICIA banks should be subject to greater depositor monitoring. However, it is also possible that regulatory monitoring may substitute for individual depositors’ scrutiny. Therefore, the net effect of regulation is an empirical question. The empirical evidence reported in Panels A and B of Online Appendix 2 shows that FDICIA regulation has insignificant impact on the negative association between financial literacy and ABSDLLP.
We examine whether our results are affected by mergers and acquisitions (M&A) activities that may lead to bank managers having different incentives for earnings management. As M&A activities often lead to a large spike in asset growth, we delete banks with more than 10% annual asset growth rate to exclude banks that have potentially engaged in M&A activities. The untabulated results show that FINLIT is negatively and significantly associated with ABSDLLP, suggesting that financial literacy improves earnings quality among banks without major ongoing M&A activities.
The depositor concentration may influence the association between financial literacy of depositors and banks’ earnings management. We partition our sample into two subgroups based on the median value of depositor concentration (measured by the Herfindahl index of bank deposits at the state level). The results reported in Panels A and B of Online Appendix 3 show that FINLIT is significantly and negatively correlated with ABSDLLP for the subgroups of both high depositor-concentration states and low depositor-concentration states. Therefore, our main results do not seem to be driven by depositor concentration level.
7. Conclusion
Since the 2007–2008 global financial crisis, governments, financial sector experts, and academics have emphasized the role of financial literacy in supporting inclusive and sustainable growth as well as the relationship between financial education and broader financial, economic, and social outcomes (OECD, Citation2018). Although financial literacy attracts global interest, not much empirical evidence exists to support its perceived role beyond the documented individual or household changes in financial behaviors, such as savings, equity investments, and borrowing.
Our research takes an initial step toward understanding the association between consumer behaviors and aggregate financial and economic outcomes. Banks are known to play a central role in economic and financial stability because they provide liquidity and capital for individuals and businesses. In this study, we provide early evidence on the impact of financial literacy on bank accounting practices. Our empirical results show that financial literacy is negatively related to bank earnings management and, thus, increases bank earnings transparency. We find that retail deposits and consumer loans are two important channels through which financial literacy influences bank transparency. More specifically, we find that it is the stable funding and fewer delinquencies of financially literate depositors/customers that account for our results.
We recognize that our research is subject to certain limitations. Our research employs the NFCS financial literacy data that are aggregated at the state level. Future research could consider investigating the financial literacy of depositors and borrowers at the branch or office level and testing their relationship with bank transparency. This paper focuses on only one type of bank behavior: bank financial reporting transparency in relation to financial literacy. Future prospective studies can expand the scope of our study by examining the impact of citizens’ financial literacy on bank capitalization, liquidity, and risk-taking activities.
Supplemental data
Supplemental data for this article can be accessed https://doi.org/10.1080/09638180.2021.1965897.
Notes
1 Multiple imputation is a simulation-based statistical technique for handling missing data. Kofman and Sharpe (Citation2003) suggest using multiple imputation in the analysis of incomplete observations in finance. To predict the missing values of FINLIT, a linear regression imputation method (i.e., regressing FINLIT on EDUC, INCOME, GENDER, MARIT, RACE, and state fixed effects) is used. This process of fill-in is repeated multiple times using Monte Carlo simulation by averaging each of these separate analyses. EDUC is the statewide proportion of the population receiving post-secondary education, INCOME is the natural logarithm of statewide per capita income, GENDER is the statewide proportion of the male population, MARIT is the statewide proportion of the married population, RACE is the statewide proportion of the white race. Prior literature documents that education level, marital status, income, and sex are associated with financial literacy (Lusardi & Mitchell, Citation2011a, Citation2011b, Citation2011c).
2 Although FASB has required the use of the incurred-loss model for loan loss provisioning (up to December 15, 2019), the complexity of loan portfolios allows a substantial magnitude of discretion within the prescribed rules (Dugan, Citation2009).
3 It seems that the extent of managerial discretion over loan loss provisions is low for consumer loans to begin with, as bank regulators have requirements for the timeline over which consumer loans are charged off, suggesting that the provisioning could also be mechanical. This is consistent with prior research arguing that banks have more discretion over commercial loans compared to consumer loans (e.g., Liu & Ryan, Citation2006). However, the Federal Reserve Bank of Kansas City (Citation2018) emphasizes the allowance of discretion with pricing or underwriting decisions for consumer loans. Frequently observed discretionary pricing practices include lack of established rate sheets, reliance on unwritten pricing guidelines, reliance on vague and/or unwritten discretionary criteria when making adjustments to established rate sheets (e.g., good customer, large depositor), lack of guidance to select a rate from an established rate range, and inadvertent omission of pricing guidelines for certain credit requests. Therefore, the Fed advocates a compliance management system that includes an evaluation of the bank’s discretionary practices to determine the level of fair lending risk posed by such practices, as well as the controls in place to properly identify, measure, control, and monitor risks.
4 As an alternate proxy for financial literacy, we calculate the financial literacy score (FINLITW) for each multi-state bank by using the weighted average FINLIT based on state-level deposits (aggregated from branch-level data available from FDIC’s Summary of Deposits). We re-estimate all our regression models using FINLITW, and the untabulated results show that ABSDLLP_A and ABSDLLP_B are significantly and negatively associated with FINLITW at the 1% level.
5 As FINLIT is a state-level variable, this test raises the concern that state-level economic factors can affect both financial literacy and bank loan loss provisioning. To account for the different economic conditions, we compute ABSDLLP by estimating the first-stage model by state-year to allow the coefficients of the determinants to vary. In a robustness test, the second-stage model shows that FINLIT is significantly and negatively associated with ABSDLLP computed at the state-year level.
6 Bhat et al. (Citation2019) identify that commonly used measures of LLP timeliness vary substantially across loan types. We examine the relationship between financial literacy and bank loan types, including consumer loans, real estate loans, and commercial loans studied by Bhat et al. (Citation2019). The untabulated results show that the financial literacy of bank customers is significantly and negatively associated with consumer loans and real estate loans, but not commercial loans. This is consistent with prior literature documenting that financially literate consumers are less likely to take excessive amounts of debt (Lusardi & Tufano, Citation2015). In a robustness test, we incorporate bank loan types in the first-stage LLP estimation model. The untabulated results for the second-stage model show that ABSDLLP has a significantly negative relationship with FINLIT even after controlling for consumer loans, real estate loans, and commercial loans.
7 We also follow Beatty and Liao (Citation2014) to exclude loan charge-offs in the first-stage LLP estimation Model (1b). The untabulated results of the second-stage regression remain robust after we exclude loan charge-offs in Model (1b).
8 To empirically control for income smoothing, we include the variable EBP (earnings before loan loss provisions divided by total assets) in our second stage of ABSDLLP regressions to account for the fact that management may manipulate LLP to achieve its desired level of net income.
9 As Hirshleifer et al. (Citation2009) suggest, the decile rank has the advantage of reducing the influence of outliers. It also helps to linearize the relationship between ABSDLLP and FINLIT. We calculate DEPOSIT as the decile rank of the sum of RCON3485, RCONB563, RCON3486, RCON3487, RCONA529, and RCON3469 scaled by RCFD2170 (from Call Reports) or the sum of DPDC, CTTD, DPSC, and MMCD scaled by AT (from Compustat Bank database) if Call Reports data are missing. Similarly, we calculate CLOAN as the decile rank of RCFD1975 scaled by RCON2122 (from Call Reports) or LCACRD scaled by LNTAL (from Compustat Bank database) if Call Reports data are missing.
10 We control for bank fixed effects because many unobservable bank characteristics may affect banks’ discretion on LLP. LLP discretion is sticky within a bank, and FINLIT is likely to be sticky over time. Therefore, we run two separate regressions: (1) including only state and year fixed effects to control for within-state variation and (2) including bank and year fixed effects to control for within-bank variation.
11 The search-based index is obtained from Google Trends (https://trends.google.com/trends/) for a given state in a particular month from January 2009 to December 2018. The index is adjusted by the national average and divided by 100, resulting in the scale from −1 to 1. The greater the number on the scale is, the more intensive the search for the queried terms is. We then aggregate the monthly search index to annual level and calculate the mean value for each state-year.
References
- Anderson, R. C., Mansi, S. A., & Reeb, D. M. (2004). Board characteristics, accounting report integrity, and the cost of debt. Journal of Accounting and Economics, 37(3), 315–342. https://doi.org/https://doi.org/10.1016/j.jacceco.2004.01.004
- Armstrong, C. S., Core, J. E., & Guay, W. R. (2014). Do independent directors cause improvements in firm transparency? Journal of Financial Economics, 113(3), 383–403. https://doi.org/https://doi.org/10.1016/j.jfineco.2014.05.009
- Barth, M. E., & Landsman, W. R. (2010). How did financial reporting contribute to the financial crisis? European Accounting Review, 19(3), 399–423. https://doi.org/https://doi.org/10.1080/09638180.2010.498619
- Basu, S., Vitanza, J., & Wang, W. (2020). Asymmetric loan loss provision models. Journal of Accounting and Economics, 70(2–3), 101359. https://doi.org/https://doi.org/10.1016/j.jacceco.2020.101359
- Beatty, A., & Liao, S. (2014). Financial accounting in the banking industry: A review of the empirical literature. Journal of Accounting and Economics, 58(2-3), 339–383. https://doi.org/https://doi.org/10.1016/j.jacceco.2014.08.009
- Beaver, W. H., & Engel, E. E. (1996). Discretionary behavior with respect to allowances for loan losses and the behavior of security prices. Journal of Accounting and Economics, 22(1–3), 177–206. https://doi.org/https://doi.org/10.1016/S0165-4101(96)00428-4
- Bhat, G., Lee, J. A., & Ryan, S. G. (2019). Using loan loss indicators by loan type to sharpen the evaluation of banks’ loan loss accruals. Available at SSRN 2490670.
- Bushman, R. M. (2016). Transparency, accounting discretion, and bank stability. Economic Policy Review, Issue Aug, 22(1), 129–149. https://www.newyorkfed.org/medialibrary/media/research/epr/2016/epr_2016-transparency-accounting_bushman.pdf?la=en
- Bushman, R. M., & Williams, C. D. (2012). Accounting discretion, loan loss provisioning, and discipline of banks’ risk-taking. Journal of Accounting and Economics, 54(1), 1–18. https://doi.org/https://doi.org/10.1016/j.jacceco.2012.04.002
- Bushman, R. M., & Williams, C. D. (2015). Delayed expected loss recognition and the risk profile of banks. Journal of Accounting Research, 53(3), 511–553. https://doi.org/https://doi.org/10.1111/1475-679X.12079
- Calvet, L. E., Campbell, J. Y., & Sodini, P. (2009). Measuring the financial sophistication of households. American Economic Review, 99(2), 393–398. https://doi.org/https://doi.org/10.1257/aer.99.2.393
- Chen, W., Hribar, P., & Melessa, S. (2018). Incorrect inferences when using residuals as dependent variables. Journal of Accounting Research, 56(3), 751–796. https://doi.org/https://doi.org/10.1111/1475-679X.12195
- Cohen, L. J., Cornett, M. M., Marcus, A. J., & Tehranian, H. (2014). Bank earnings management and tail risk during the financial crisis. Journal of Money, Credit and Banking, 46(1), 171–197. https://doi.org/https://doi.org/10.1111/jmcb.12101
- Cole, S., Sampson, T., & Zia, B. (2011). Prices or knowledge? What drives demand for financial services in emerging markets? Journal of Finance, 66(6), 1933–1967. https://doi.org/https://doi.org/10.1111/j.1540-6261.2011.01696.x
- Dugan, J. (2009). Loan loss provisioning and pro-cyclicality. Remarks by John C. Dugan, Comptroller of the Currency, Before the Institute of International Bankers, 2, 1–10. https://www.occ.gov/news-issuances/speeches/2009/pub-speech-2009-16.pdf
- Federal Reserve Bank of Kansas City. (2018). Discretionary credit practices and its effect on fair lending. https://www.kansascityfed.org/en/banking/fedconnections/archive/discretionary-credit-practices-and-its-effect-on-fair-lending-8-28-18
- Fonseca, A. R., & Gonzalez, F. (2008). Cross-country determinants of bank income smoothing by managing loan-loss provisions. Journal of Banking & Finance, 32(2), 217–228. https://doi.org/https://doi.org/10.1016/j.jbankfin.2007.02.012
- Francis, J. R., & Wang, W. (2021). Common Auditors and Private Bank Loans. Contemporary Accounting Research, 38(1), 793–832.
- Gerardi, K., Goette, L., & Meier, S. (2013). Numerical ability predicts mortgage default. Proceedings of the National Academy of Sciences, 110(28), 11267–11271. https://doi.org/https://doi.org/10.1073/pnas.1220568110
- Hastings, J. S., & Tejeda-Ashton, L. (2008). Financial literacy, information, and demand elasticity: Survey and experimental evidence from Mexico (No. w14538). National Bureau of Economic Research.
- Hirshleifer, D., Lim, S. S., & Teoh, S. H. (2009). Driven to distraction: Extraneous events and underreaction to earnings news. Journal of Finance, 64(5), 2289–2325. https://doi.org/https://doi.org/10.1111/j.1540-6261.2009.01501.x
- Hsiao, Y., & Tsai, W. (2018). Financial literacy and participation in the derivatives markets. Journal of Banking & Finance, 88, 15–29. https://doi.org/https://doi.org/10.1016/j.jbankfin.2017.11.006
- Jha, A., & Chen, Y. (2015). Audit fees and social capital. The Accounting Review, 90(2), 611–639. https://doi.org/https://doi.org/10.2308/accr-50878
- Jiang, L., Levine, R., & Lin, C. (2016). Competition and bank opacity. The Review of Financial Studies, 29(7), 1911–1942. https://doi.org/https://doi.org/10.1093/rfs/hhw016
- Jin, J. Y., Kanagaretnam, K., & Liu, Y. (2018). Banks’ funding structure and earnings quality. International Review of Financial Analysis, 59(3), 163–178. https://doi.org/https://doi.org/10.1016/j.irfa.2018.08.009
- Jin, J. Y., Kanagaretnam, K., Liu, Y., & Lobo, G. J. (2019). Economic policy uncertainty and bank earnings opacity. Journal of Accounting and Public Policy, 38(3), 199–218. https://doi.org/https://doi.org/10.1016/j.jaccpubpol.2019.05.002
- Kanagaretnam, K., Krishnan, G. V., & Lobo, G. J. (2010). An empirical analysis of auditor independence in the banking industry. The Accounting Review, 85(6), 2011–2046. https://doi.org/https://doi.org/10.2308/accr.2010.85.6.2011
- Kanagaretnam, K., Lim, C. Y., & Lobo, G. J. (2011). Effects of national culture on earnings quality of banks. Journal of International Business Studies, 42(6), 853–874. https://doi.org/https://doi.org/10.1057/jibs.2011.26
- Kanagaretnam, K., Lim, C. Y., & Lobo, G. J. (2014). Influence of national culture on accounting conservatism and risk taking in the banking industry. The Accounting Review, 89(3), 1115–1149. https://doi.org/https://doi.org/10.2308/accr-50682
- Kim, D. (2016). Depositor runs and financial literacy. Working Paper.
- Kim, K. T., & Lee, J. (2018). Financial literacy and use of payday loans in the United States. Applied Economics Letters, 25(11), 781–784. https://doi.org/https://doi.org/10.1080/13504851.2017.1366635
- Kim, K. T., Lee, J., & Hanna, S. D. (2020). The effects of financial literacy overconfidence on the mortgage delinquency of US households. Journal of Consumer Affairs, 54(2), 517–540. https://doi.org/https://doi.org/10.1111/joca.12287
- Kofman, P., & Sharpe, I. G. (2003). Using multiple imputation in the analysis of incomplete observations in finance. Journal of Financial Econometrics, 1(2), 216–249. https://doi.org/https://doi.org/10.1093/jjfinec/nbg013
- Laeven, L., & Levine, R. (2009). Bank governance, regulation and risk taking. Journal of Financial Economics, 93(2), 259–275.
- Liu, C. C., & Ryan, S. G. (2006). Income smoothing over the business cycle: Changes in banks’ coordinated management of provisions for loan losses and loan charge-offs from the pre-1990 bust to the 1990s boom. The Accounting Review, 81(2), 421–441. https://doi.org/https://doi.org/10.2308/accr.2006.81.2.421
- Lusardi, A., & Mitchell, O. S. (2011a). Financial literacy and planning: Implications for retirement wellbeing (No. w17078). National Bureau of Economic Research.
- Lusardi, A., & Mitchell, O. S. (2011b). Financial literacy and retirement planning in the United States. Journal of Pension Economics and Finance, 10(4), 509–525. https://doi.org/https://doi.org/10.1017/S147474721100045X
- Lusardi, A., & Mitchell, O. S. (2011c). Financial literacy around the world: An overview. Journal of Pension Economics and Finance, 10(4), 497–508. https://doi.org/https://doi.org/10.1017/S1474747211000448
- Lusardi, A., Mitchell, O. S., & Curto, V. (2010). Financial literacy among the young. Journal of Consumer Affairs, 44(2), 358–380. https://doi.org/https://doi.org/10.1111/j.1745-6606.2010.01173.x
- Lusardi, A., & Tufano, P. (2015). Debt literacy, financial experiences, and over indebtedness. Journal of Pension Economics and Finance, 14(4), 332–368. https://doi.org/https://doi.org/10.1017/S1474747215000232
- Maechler, A. M., & McDill, K. M. (2006). Dynamic depositor discipline in US banks. Journal of Banking & Finance, 30(7), 1871–1898. https://doi.org/https://doi.org/10.1016/j.jbankfin.2005.07.010
- OECD. (2018, 18 May). Effective financial education for sustainable and inclusive growth. 5th OECD-GFLEC Global Policy Research Symposium to Advance Financial Literacy.
- OECD, P. (2013). PISA 2012 assessment and analytical framework: Mathematics, reading, science, problem solving and financial literacy, 2013. OECD Publishing.
- Stango, V., & Zinman, J. (2009). Exponential growth bias and household finance. Journal of Finance, 64(6), 2807–2849. https://doi.org/https://doi.org/10.1111/j.1540-6261.2009.01518.x
- Stolper, O. A., & Walter, A. (2017). Financial literacy, financial advice, and financial behavior. Journal of Business Economics, 87(5), 581–643. https://doi.org/https://doi.org/10.1007/s11573-017-0853-9
- U.S. Department of the Treasury. (2019). Federal financial literacy reform: Coordinating and improving financial literacy efforts. https://home.treasury.gov/system/files/136/FFLRCoordinatingImprovingFinancialLiteracyEfforts.pdf
- Van Rooij, M., Lusardi, A., & Alessie, R. (2011). Financial literacy and stock market participation. Journal of Financial Economics, 101(2), 449–472. https://doi.org/https://doi.org/10.1016/j.jfineco.2011.03.006
Appendices
Appendix A
Five Financial Literacy Questions from NFCS State-by-State Surveys
The National Financial Capability Study (NFCS) is funded by the FINRA Investor Education Foundation and conducted by ARC Research. The overarching research objectives of the NFCS are to benchmark key indicators of financial capability and evaluate how these indicators vary with underlying demographic, behavioral, attitudinal and financial literacy characteristics. The 2009, 2012, 2015 and 2018 NFCS State-by-State Surveys are nationwide online surveys conducted among over 25,000 American adults. State figures are weighted to be representative of each state in terms of age, gender, ethnicity and education.
In measuring respondents’ financial knowledge, the surveys include the following five basic financial concepts questions (correct answer indicated in bold):
‘Suppose you had $100 in a savings account and the interest rate was 2% per year. After 5 years, how much do you think you would have in the account if you left the money to grow?’
More than $102
Exactly $102
Less than $102
Don’t know
Refuse to answer
‘Imagine that the interest rate on your savings account was 1% per year and inflation was 2% per year. After 1 year, with the money in this account, would you be able to buy … ’
More than today
Exactly the same as today
Less than today
Don’t know
Refuse to answer
If interest rates rise, what will typically happen to bond prices?
They will rise
They will fall
They will stay the same
There is no relationship between bond prices and the interest rate
Don’t know
Prefer not to say
A 15-year mortgage typically requires higher monthly payments than a 30-year mortgage, but the total interest paid over the life of the loan will be less.
True
False
Don’t know
Prefer not to say
Buying a single company’s stock usually provides a safer return than a stock mutual fund.
True
False
Don’t know
Prefer not to say
Appendix B. Variable Definitions
Table