Abstract
Purpose
Measuring physical activity informs activity recommendations in clinical practice and provides outcomes in clinical trials that are meaningful to patients. Activity assessment in muscle disease is challenging and there is insufficient evidence to support any single activity measure; however, multi-modal activity measurement might have potential.
Materials and methods
This two-part study included 20 and 95 adults with progressive muscle diseases with mobility ranging from independent to assisted, including wheelchair users. Their activity was measured using a multi-sensor Fitbit activity monitor, for which criterion validity and acceptability were tested in study 1 and validity, reliability, and responsiveness were tested in the longitudinal, home-based study 2.
Results
Study 1: Fitbit was acceptable and had strong criterion validity (rho/kappa ≥0.90), although up to 15% measurement error. Study 2: Fitbit had satisfactory concurrent and construct validity, reliability, and responsiveness. However, Fitbit active minutes registered 75 min more activity per week than gold standard moderate and vigorous physical activity (MVPA) time.
Conclusions
Fitbit had satisfactory measurement properties for monitoring physical activity in adults with progressive muscle diseases. However, Fitbit should not be considered an exact step counter, heart rate monitor or calorimeter and Fitbit active minutes are not synonymous with MVPA time.
People with progressive muscle diseases mobilise independently, with walking aids and with wheelchairs; physical activity measurement can be challenging in this population.
Multisensor smart activity monitoring by Fitbit had satisfactory validity, reliability, responsiveness, and acceptability for the estimation of physical activity in adults with progressive muscle diseases.
Fitbit active minutes are not synonymous with moderate and vigorous physical activity (MVPA) time measured using a research grade accelerometer.
Implications for rehabilitation
Introduction
Measuring physical activity is important for adults with progressive muscle diseases to inform activity recommendations in clinical practice and for outcomes in clinical trials that are meaningful to patients [Citation1,Citation2]. In muscle disease, functional heterogeneity and abnormalities in gait, heart rate and metabolism make activity assessment particularly challenging.
Currently, there is insufficient evidence to support the use of any single physical activity measure for adults with progressive muscle diseases [Citation1,Citation3]. To compensate for limitations in physical activity measurement approaches, combined approaches using multiple activity metrics have been proposed [Citation4,Citation5]. Several multisensor activity monitors, with smart phone connectivity, are commercially available and the technology is regularly evolving. Some smart activity monitors, including Fitbits, have demonstrated satisfactory measurement properties in healthy populations [Citation6]. However, it is not known whether Fitbit multisensor activity monitoring is suitable for adults with progressive muscle diseases.
The aim of this two-part study was to evaluate a smart, multisensor Fitbit activity monitor as a measure of physical activity in adults with progressive muscle diseases. The objective of the first study was to test the criterion validity and acceptability of Fitbit. The objective of the second study was to test the validity, reliability, and responsiveness of Fitbit compared to a research-grade activity monitor.
Methods
Study design and setting
Ethical approval for the first prospective, observational study was granted in 2017 by King’s College London Research Ethics Committee (LRS-16/17-4226). From October 2017 to April 2018, participants were invited for activity monitoring, during lying, sitting, walking, and cycling tasks with a physiotherapist at King’s College London, Guy’s Hospital Campus (n = 16). For those unable to travel to London, activity monitoring was carried out at a gym close to their address (n = 4).
Ethical approval for the second prospective, home-based observational study was granted in 2019 (LRS-18/19-10909). Free-living activity monitoring was carried out remotely; from March 2019 until August 2020 (see ). Longitudinal Fitbit, questionnaire, and accelerometry data were collected at baseline in 2019 and followed up six to nine months later in 2020. Little muscle disease-related functional deterioration was anticipated in under a year [Citation7,Citation8]; thus, any changes measured at follow up were expected to reflect activity behaviour change predominantly.
Participants
In study 1, participants were a purposive sample including 10 people who mobilised with assistance of sticks, crutches, wheeled walkers, and wheelchairs, and 10 who mobilised independently. They were recruited via Muscular Dystrophy UK (MDUK) advertisement (www.musculardystrophyuk.org/progress-in-research/research-projects), newsletter, social media sharing, and word of mouth. Volunteers contacted the King’s College London (KCL) researcher (SRL), who remotely provided study information and followed up with eligibility screening by telephone. Informed consent for study participation, and for contacting their doctor, was obtained by return of signed consent form by post or email. Eligibility was confirmed by physiotherapist (SRL) when sufficient supporting information was provided by the potential participant. In the case of any discrepancy, eligibility was resolved by a neurologist (MR) and/or the potential participant’s GP/consultant. Finally, participants were screened for contraindications to exercise testing by medical clearance from their usual doctor.
In study 2, participants were a convenience sample who opted-in to wear a Fitbit in addition to longitudinal activity monitoring by questionnaire and accelerometry. Participants in the main activity monitoring study [Citation9] were recruited via national muscle disease registries (https://newcastle-muscle.org/clinical) and MDUK. They underwent the same eligibility screening and consent procedures described above, excluding clearance for exercise testing. Screening order was determined chronologically, based on the date of initial contact only, to ensure no selection bias based on demographic information.
In both studies, participants were included if they were UK resident adults with a confirmed diagnosis of inclusion body myositis, myotonic dystrophy, or muscular dystrophy (including facioscapulohumeral dystrophy, limb girdle muscular dystrophy, dysferlinopathy, dystrophinopathy (including manifesting female carriers), or specific congenital myopathies lasting into adulthood). Participants were excluded if they were not UK residents (because KCL research participant insurance only covers UK residents), cognitively impaired, unable to wear an activity monitor, aged <18 years, did not have a confirmed diagnosis or had muscle weakness resulting from other nervous system dysfunction.
Study 1
Participants completed four supervised 10-min tasks (sitting, lying, walking/mobilising, and a cycle ergometer submaximal exercise tolerance test [Citation10]). They wore a Fitbit Charge 2 (Fitbit Inc., San Francisco, CA, discontinued). Fitbit is a wrist-worn, tri-axial accelerometer with continuous optical heart rate monitoring. It syncs with the Fitbit smart phone app. It yields physical activity metrics including steps, calories (converted to intensity in metabolic equivalent minutes (METs) by multiplying Fitbit calories by body weight and dividing by time), active minutes (in bouts of ≥10 min), sleep, and hourly activity (frequency measured by the number of hours between 09:00 and 17:00 daily when ≥250 steps or significant arm movements were registered). Fitabase (an independent research data platform (Small Steps Labs, LLC, San Diego, CA)) was used to access minute-by-minute Fitbit data.
For criterion validity, the gold standard comparators each minute during each task were direct observation of physical activity (including independent-observer, video-verified step counting, and vicarious rating of perceived exertion (RPE) [Citation11]) and miniature electrocardiogram heart rate measurement by Polar H10 (Polar Electro, Espoo, Finland). Testing was repeated a week later. Participants wore their Fitbit during the week between testing sessions and gave feedback on their experiences. Feedback was analysed qualitatively using a framework analysis. Satisfactory criterion validity, for both independent and assisted mobility groups, and no excessive or unresolvable negative feedback in terms of acceptability were prerequisites for study 2 to proceed.
Study 2
At baseline, participants completed demographic and questionnaire data including anthropometrics, employment, disability (Health Assessment Questionnaire (HAQ) [Citation12]) and quality of life (Individualised Neurological Quality of Life (INQoL) [Citation13]). Self-reported physical activity time and estimated metabolic expenditure was measured using a modified version of the International Physical Activity Questionnaire (IPAQ) [Citation14]. Modifications included equivalent wheelchair activities in the vigorous, moderate, and walking activity categories, and “inactive” replacing the word “sedentary” in the final question.
The comparator measure for Fitbit validity and responsiveness was a research-grade tri-axial accelerometer, GENEActiv (ActivInsights Ltd., Kimbolton, UK). The GENEActiv has been validated for adults with progressive muscle diseases [Citation15]. It was posted to participants to wear continuously on their non-dominant wrists for a week, removing it only for washing. The accelerometer sampled continuously at a frequency of 10 Hz. Data were processed automatically using the GGIR package in R (version 3.6.0) (R Foundation for Statistical Computing, Vienna, Austria) [Citation16]. Acceleration data were processed in 1-min epochs in milli-gravitational units (milli-g) with gravitational correction [Citation17]. Overall activity intensity was expressed in mean accelerations (milli-g) per minute each day. Activity frequency was the percentage per day of hourly, non-consecutive movements ≥80 milli-g/min for ≥5 min each hour between 09:00 and 17:00 daily. Intensity cut-points were light ≥30 milli-g/min, moderate ≥100 milli-g/min and vigorous activity ≥400 milli-g/min [Citation18]. These yielded time in minutes of sleep, inactivity, light activity, and bouts of ≥10 min of moderate and vigorous physical activity (MVPA). Objective physical activity was measured using GENEActiv at baseline and nine months later at follow up.
After baseline, participants were posted a Fitbit Inspire HR (Fitbit Inc., San Francisco, CA; like the Fitbit described above, but lighter weight, waterproof, with contact charger and no altimeter). After receiving their Fitbit, participants set it up (with support as required) and allowed the researcher remote access to their activity data. Participants were asked to wear their Fitbit continuously on their non-dominant wrists, removing it only for washing.
At time point 1 (two months after baseline), participants had worn their Fitbit for a week. Their activity data were collected remotely, and they completed the IPAQ and HAQ for the corresponding week. At time point 2, the following week, Fitbit and questionnaire data collection were repeated. For reliability testing, stability between weeks was established by lack of change in IPAQ and HAQ scores.
At follow up (nine months after baseline), participants’ Fitbit data were collected for a final week. During the follow up week, participants simultaneously wore a GENEActiv and a Fitbit, and all baseline questionnaires were repeated. A single researcher (SRL) was responsible for data collection and analysis. To minimise risk of data entry errors, Fitbit, GENEActiv, and electronic questionnaire data were collected into automatically populated spreadsheets using R (R Foundation for Statistical Computing, Vienna, Austria).
Statistical analysis
Statistical analyses were carried out using standardised scripts in R (version 3.6.0) (R Foundation for Statistical Computing, Vienna, Austria). Non-parametric analyses were used if data were not normally distributed. Significance level was set at α = 0.05. Satisfactory thresholds for validity correlation coefficients were based on recommendations for measure evaluation studies [Citation19] (see ).
Table 1. Measurement properties summary of analyses.
Study 1
A sample size of 20 was calculated to yield 95% power for a predicted criterion correlation coefficient of 0.70 [Citation20] (see for a summary of criterion validity and measurement error testing). Categorical agreement of activity level (inactive, light, moderate, and vigorous) between Fitbit and direct observation was tested using Cohen’s kappa (see ). Categorical cut-points applied for Fitbit activity level in METs and directly observed RPE each minute were: inactive (<1.5 METs/RPE), light (<3.0 METs/RPE), moderate (<6.0 METs/RPE), and vigorous exceeding these. A kappa of ≥0.75 was considered satisfactory [Citation21]. Absolute measurement error was calculated using the difference between Fitbit and gold standard comparator; this was divided by the gold standard measurement to calculate percentage error. Minutes with missing data were excluded from analyses. Minute-by-minute analyses were carried out for the whole sample, and by mobility subgroup (independent and assisted) and task subgroup (inactive lying and sitting and active walking/mobilising and cycling).
Study 2
The desired sample size was 100, as recommended for measure evaluation studies [Citation19]. A retrospective power calculation was performed. Validity, measurement error, reliability, and responsiveness testing are summarised in . For discriminative validity, wheelchair users were expected to be significantly less active. Subgroup analyses were carried out by disability (according to HAQ reported ambulant and non-ambulant wheelchair user status). Missing accelerometry data of ≤10 min were included in daily means; days with <23 h monitored were excluded from analyses. Questionnaires were scored using available items; questionnaires with >10% of items missing were excluded from analyses. Participants lost to follow up were excluded from validity and responsiveness analyses.
Results
Study 1
Fitbit data (1.9% missing) from all 20 participants were included. Fitbit had strong criterion validity compared to gold standard comparators for minute-by-minute steps, heart rate and categorical METs based activity intensity level (rho = 0.97, 0.96 and kappa = 0.90, respectively). Mean systematic, absolute and percentage measurement errors for step count were +0.8, 2.2, and 9%, respectively. For heart rate bpm, −3.2, 3.7, and 4%, respectively.
Subgroup analysis revealed consistently acceptable criterion validity between mobility and active/inactive task subgroups. Measurement error for heart rate was also consistent between independent and assisted mobility groups. However, Fitbit step error was lower during independent mobility than for those who mobilised with assistance (5% versus 15%). Step error was also lower during inactive lying and sitting compared to active walking and cycling (0% versus 10%). Fitbit heart rate error was lower during lying than the other tasks (2% versus 4–5%) (see ).
Table 2. Measurement properties of (minute by minute validity and measurement error results from study 1 (N = 20)).
Acceptability of the Fitbit was supported by largely positive feedback from participants (see ). Concerns raised, such as technophobia and dexterity challenges were easily resolved by additional technical support and new equipment for straps and charging. Two minor adverse events were reported; minor skin irritation and bruising from a tight strap.
Study 2
Of 511 participants who responded, 147 were chronologically screened for eligibility until the study reached its target of 110 participants. At baseline, 103 participants completed data collection and of these, 95 opted-in to the Fitbit part of the study. At time points 1 and 2, 94 participants completed Fitbit data collection and, of these, 90 completed data collection at follow-up (6.9 months later (range 6.8–7.5)) (see ). For n = 90 validity correlations, a retrospective power calculation yielded 96% power. summarises participants’ demographic information. All participants included had at least five days of useable activity monitoring data.
Table 3. Demographics and baseline information (N = 94).
Fitbit had generally satisfactory concurrent validity. There was a strong correlation between Fitbit and GENEActiv frequency of hourly movements per day (rho = 0.77). Similarly, there were strong correlations between GENEActiv intensity (daily mean accelerations per minute) and Fitbit intensity (steps and METs) (rho = 0.83 and 0.70). However, only moderate correlations were found for time between GENEActiv (MVPA and sleep) and Fitbit (active minutes and sleep) (rho = 0.68 and r = 0.55). Satisfactory convergent validity of Fitbit frequency, intensity (steps and METs), and time (active minutes) was demonstrated with disability (HAQ) (rho= −0.74 to −0.58), quality of life (INQoL) (rho= −0.49 to −0.40) and self-reported physical activity (modified IPAQ) (rho = 0.43–0.56). Divergent validity of Fitbit activity frequency, intensity, and time was demonstrated by lack of correlation with unrelated constructs age, height, gender, and handedness (rho = 0.03–0.13). Discriminative validity was demonstrated by significant differences in Fitbit activity metrics between ambulant participants and wheelchair users (see ).
Measurement error of Fitbit active minutes compared to GENEActiv MVPA time was examined using Bland–Altman’s plots (see ). The limits of agreement were moderate; however, there was proportional bias (R2=0.30, linear regression coefficient = 0.55, p < 0.000) indicating significantly more measurement error in Fitbit active minutes at greater MVPA time. There was a 72-min systematic error of Fitbit active minutes in excess of GENEActiv MVPA minutes. The absolute measurement error was 75 min per week.
Figure 3. Bland–Altman’s plot comparing Fitbit active minutes with GENEActiv moderate and vigorous physical activity (MVPA) minutes.
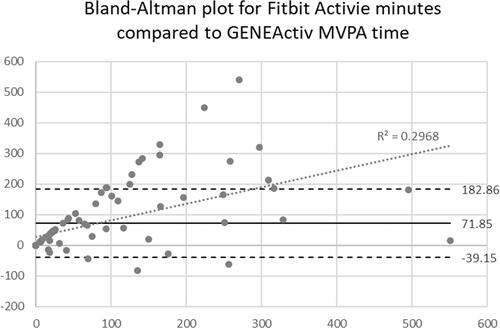
Test–retest reliability was satisfactory between time point 1 and 2 for Fitbit frequency, intensity (steps and METs) and time (active minutes and sleep) (ICC = 0.94, 0.95 and 0.89, 0.78 and 0.77). Stability between testing weeks was confirmed by no significant change in self-reported physical activity (IPAQ) or disability (HAQ). Satisfactory responsiveness to change was demonstrated for Fitbit frequency, intensity (steps and METs), and time (active minutes) (AUC = 0.85, 0.85, 0.89, and 0.78, respectively) (see ).
Figure 4. Receiver operating characteristics (ROC) curves illustrating Fitbit change in activity from baseline to follow up for (A) steps, (B) frequency of hourly movements, (C) METs, and (D) active minutes (there was no significant change in sleep from baseline to follow up). AUC: area under curve; METs: metabolic equivalents.
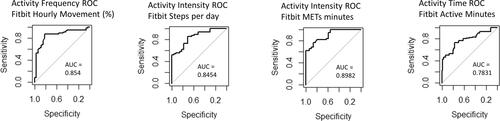
Subgroup analysis of Fitbit steps revealed stronger validity for ambulant participants versus wheelchair users (concurrent validity with GENEActiv rho = 0.76 versus 0.53, convergent validity with disability rho= −0.56 versus −0.48, quality of life rho= −0.32 versus −0.24 and IPAQ score rho = 0.41 versus 0.29). Whereas validity was similar between disability sub-groups for Fitbit frequency, METs, and time. Reliability and responsiveness were similar for all Fitbit metrics between disability sub-groups. Although, there was a trend for slightly stronger measurement properties in the (larger, n = 56) ambulant sub-group (see ).
Table 4. Measurement properties of Fitbit (home-based activity monitoring results from study 2).
Discussion
Fitbit had strong criterion validity and was an acceptable activity monitor. It had broadly satisfactory validity, reliability, and responsiveness for the assessment of physical activity in adults with progressive muscle diseases. This is in concurrence with other studies reporting satisfactory measurement properties in healthy adults and people with neuromuscular diseases and altered mobility [Citation6,Citation22,Citation23]. However, we found Fitbit metrics, including steps, heart rate, and active minutes have measurement error which must be considered when interpreting data.
Fitbit steps had the strongest validity in our study. Other studies have also reported satisfactory validity for Fitbit steps [Citation6,Citation22,Citation23]. Although, most of these studies had ≤50 participants and some used waist or ankle-worn devices. Conversely, several larger studies questioned the accuracy of Fitbit steps [Citation24–27]. All these studies tested older Fitbit models and the evolving nature of activity monitoring technology has been noted [Citation22]. However, our findings concur regarding considerable Fitbit steps measurement error. We also noted greater measurement error during active walking and cycling tasks compared to inactive lying and sitting tasks. Furthermore, we found a general trend for slightly inferior measurement properties for the non-ambulant and assisted-mobility subgroups. Variation in gait patterns, mobility aids, and device movement artefacts may impact Fitbit accuracy [Citation6,Citation28]. Fitbit steps are reportedly less accurate, especially when wrist-worn [Citation24], for impaired mobility [Citation25,Citation26] and in short walking tests compared to longer, free-living assessments [Citation27]. Free-living activity monitoring typically lasts ≥24 h and is, therefore, inclusive of more inactive than active time. Thus, it is likely that Fitbit performs better as a free-living activity monitor, over days or weeks including both active and inactive time, than as a precise step counter for short active tasks, especially for those with mobility impairments.
Fitbit heart rate criterion validity and measurement error were satisfactory. Although the systematic underestimation of 3.7 bpm and improved accuracy in lying mean, the Fitbit should not be used for absolute cardiac monitoring. Activity data derived from heart rate monitoring alone might also be imprecise secondary to reduced heart rate variability in some adults with muscle diseases [Citation29,Citation30]. However, Fitbit algorithms combine heart rate and accelerometry data to estimate physical activity which may temper the effect of individual metric measurement errors.
Fitbit active minutes had unsatisfactory concurrent validity with GENEActiv (rho = 0.68). Fitbit active minutes are derived from combined accelerometry and heart rate data. Therefore, they would not be expected to correlate completely with GENEActiv (solely accelerometer derived) MVPA minutes. In contrast to our findings, a study in healthy adults suggested Fitbit activity time might have stronger validity than steps over a week [Citation27]. Most other studies, in concurrence with our findings, reported good to strong correlations supporting validity of Fitbit active minutes but highlighted a tendency for overestimation [Citation6]. In our study, despite a trend for greater error at higher MVPA times, Fitbit active minutes absolute measurement error of 75 min was nearly equal to the systematic overestimation of 72 min, indicating very little random error. Based on this finding, we can deduce that Fitbit has a lower intensity threshold than the GENEActiv MVPA cut points used in this study. Thus, we can conclude that Fitbit active minutes include light, moderate and vigorous activity and are not synonymous with MVPA time. This caveat must be considered when interpreting Fitbit active minutes data.
Fitbit frequency of hourly movement had satisfactory validity, reliability, and responsiveness in our study. This is a novel finding, as no other studies have evaluated this Fitbit metric, to the authors’ knowledge. Discriminative validity testing revealed Fitbit frequency was potentially more sensitive than GENEActiv to wheelchair users’ hourly movements (6.3% versus 0.0%). Thus, frequency of hourly movement might be a useful activity metric in clinical practice and trials for both able-bodied and disabled adults with neuromuscular disease. Activity frequency also links to activity recommendations such as the World Health Organisation recommendations, which state “every move counts” [Citation31].
Fitbit METs (converted from Fitbit calories) had satisfactory validity, reliability, and responsiveness. These measurement properties were similar across mobility, task, and disability sub-groups, suggesting that combined multisensor inputs might have tempered individual measurement discrepancies. Fitbit METs had strong agreement with observed physical activity level (kappa = 0.90); however, our study did not test the measurement error of Fitbit metabolic expenditure estimations against an energy criterion. Studies, of healthy adults, have reported a tendency for Fitbit metabolic expenditure overestimation during activity, underestimation at rest, and underestimation over free-living days/weeks [Citation6]. Thus, Fitbit should not be considered an accurate calorimeter. Rather, Fitbit is a smart, multisensor activity monitor capable of relative energy expenditure estimation indicative of physical activity level. In muscle disease, any energy expenditure estimations should be treated with caution, as they are potentially inaccurate because of altered muscle metabolism in some muscle diseases [Citation32].
Fitbit was generally acceptable to study participants. Commercial, wrist-worn multisensor devices, like Fitbits, provide user-friendly activity metrics and appear to be convenient, valid, reliable, and responsive. The Fitbit models used in this study were approximately 50% lower in cost than GENEActiv monitors. However, Fitbit accuracy was questionable, and their activity metric algorithms and thresholds are not publicly available. Whereas research-grade devices are transparent for accessing and interpreting raw data and their accuracy is established. Some research-grade devices, like GENEActiv, use known light, moderate, and vigorous activity thresholds to determine active time. Also, the activity measurement epoch is adjustable (e.g., instead of 10-min bouts, 1-min bouts could be used to align better with current WHO activity recommendations [Citation31]). Other research grade monitors have been refined for accuracy in specific metrics, such as heart rate (Actiheart, Camntech) or steps (StepWatch, Cymatech). Research-grade activity monitors might, therefore, be considered “specialists”, whereas commercial, multisensor activity monitors, like Fitbit, might be considered “generalists” for broader activity estimation. Both commercial and research-grade activity monitoring technology is regularly evolving; future models have the potential to address shortcomings of their predecessors.
The key strengths of this study included thorough exploration of multiple measurement properties, inclusion of wheelchair users (previously underrepresented in neuromuscular disease studies of physical activity [Citation33]) and study design inclusive of people with a broad range of function, activity levels, ages, occupations, and locations across the UK. Study 2 also included a larger sample size than previous objective measure evaluation studies in this population [Citation1]. However, despite inclusion of participants from a range of backgrounds, generalisability in terms of co-morbidities, ethnicity, socioeconomic status, and education level is unknown because these data were not formally collected. Other limitations include recruitment of volunteers introducing potential selection bias, and a single researcher being responsible for data collection and analysis. However, risk of selection bias was minimised by the chronological eligibility screening and risk of information/researcher bias was minimised by independent verification of collected data and using automatic data processing and analyses. The study could have been improved by comparing Fitbit with an energy expenditure criterion. A healthy comparison group would have helped further clarify Fitbit measurement properties between populations and a larger sample size of adults with progressive muscle diseases would have allowed subgroup analyses by diagnosis and increased generalisability.
The smart, multisensor Fitbit activity monitor had satisfactory validity, reliability, responsiveness, and acceptability for the assessment of physical activity in adults with progressive muscle diseases. Smart, multisensor monitoring using Fitbit is suitable for the assessment of free-living physical activity intensity, frequency, and time over days or weeks. It might be generalisable for use with able-bodied and disabled adults with a range of comparable conditions. However, Fitbit should not be considered an exact step counter, heart rate monitor, or calorimeter and Fitbit active minutes are not synonymous with MVPA time.
Authors contributions
Dr Mark Ashworth, Dr Claire White, and Dr Michael Rose: PhD supervision, monitoring progress at inception, implementation, analysis, and writing up. Sarah Roberts-Lewis: guarantor with responsibility for design, implementation, honesty, accuracy, and writing up.
Acknowledgements
We would like to thank The John Walton Muscular Dystrophy Research Centre at Newcastle University and the Muscular Dystrophy UK charity for their help with advertising the study.
Disclosure statement
The authors report no conflicts of interest.
Additional information
Funding
References
- Roberts-Lewis SF, Rose MR, White CM, et al. Measurement of physical activity in adults with muscular dystrophy: a systematic review. J Phys Activ Res. 2019;4(1):1–25.
- Mercuri E, Mayhew A, Muntoni F, et al. Towards harmonisation of outcome measures for DMD and SMA within TREAT-NMD; report of three expert workshops: TREAT-NMD/ENMC workshop on outcome measures, 12th–13th May 2007, Naarden, The Netherlands; TREAT-NMD workshop on outcome measures in experimental trials for DMD, 30th June–1st July 2007, Naarden, The Netherlands; conjoint Institute of Myology TREAT-NMD meeting on physical activity monitoring in neuromuscular disorders, 11th July 2007, Paris, France. Neuromuscul Disord. 2008;18(11):894–903.
- Jimenez-Moreno AC, Newman J, Charman SJ, et al. Measuring habitual physical activity in neuromuscular disorders: a systematic review. J Neuromuscul Dis. 2017;4(1):25–52.
- Prahm KP, Witting N, Vissing J. Decreased variability of the 6-minute walk test by heart rate correction in patients with neuromuscular disease. PLoS One. 2014;9(12):e114273.
- Nightingale TE, Rouse PC, Thompson D, et al. Measurement of physical activity and energy expenditure in wheelchair users: methods, considerations and future directions. Sports Med Open. 2017;3(1):10.
- Feehan LM, Geldman J, Sayre EC, et al. Accuracy of Fitbit devices: systematic review and narrative syntheses of quantitative data. JMIR Mhealth Uhealth. 2018;6(8):e10527.
- Andersen G, Dahlqvist JR, Vissing CR, et al. MRI as outcome measure in facioscapulohumeral muscular dystrophy: 1-year follow-up of 45 patients. J Neurol. 2017;264(3):438–447.
- Leung DG, Bocchieri AE, Ahlawat S, et al. Longitudinal functional and imaging outcome measures in FKRP limb-girdle muscular dystrophy. BMC Neurol. 2020;20(1):196.
- Roberts-Lewis SF, Ashworth M, White CM, et al. COVID-19 lockdown impact on the physical activity of adults with progressive muscle diseases. BMJ Neurol Open. 2021;3(1):e000140.
- Gappmaier E. The submaximal clinical exercise tolerance test (SXTT) to establish safe exercise prescription parameters for patients with chronic disease and disability. Cardiopulm Phys Ther J. 2012;23(2):19–29.
- Borg G. Psychophysical scaling with applications in physical work and the perception of exertion. Scand J Work Environ Health. 1990;16(Suppl.1):55–58.
- Bruce B, Fries JF. The Stanford Health Assessment Questionnaire: a review of its history, issues, progress, and documentation. J Rheumatol. 2003;30(1):167–178.
- Vincent KA, Carr AJ, Walburn J, et al. Construction and validation of a quality of life questionnaire for neuromuscular disease (INQoL). Neurology. 2007;68(13):1051–1057.
- Craig CL, Marshall AL, Sjostrom M, et al. International Physical Activity Questionnaire: 12-country reliability and validity. Med Sci Sports Exerc. 2003;35(8):1381–1395.
- Jimenez-Moreno AC, Charman SJ, Nikolenko N, et al. Analyzing walking speeds with ankle and wrist worn accelerometers in a cohort with myotonic dystrophy. Disabil Rehabil. 2018;10:1–7.
- van Hees VT, Sabia S, Anderson KN, et al. A novel, open access method to assess sleep duration using a wrist-worn accelerometer. PLoS One. 2015;10(11):e0142533.
- van Hees VT, Gorzelniak L, Leon ECD, et al. Separating movement and gravity components in an acceleration signal and implications for the assessment of human daily physical activity. PLoS One. 2013;8(4):e61691.
- van Hees VT, Fang Z, Langford J, et al. Autocalibration of accelerometer data for free-living physical activity assessment using local gravity and temperature: an evaluation on four continents. J Appl Physiol (1985). 2014;117(7):738–744.
- Mokkink LB, Prinsen CAC, Patrick DL, et al. COSMIN methodology for systematic reviews of patient-reported outcome measures (PROMs): user manual. In: Mokkink LB, editor. COnsensus‐based standards for the selection of health measurement INstruments. Version 1.0 ed. Amsterdam: Amsterdam Public Health Research Institute; 2018.
- Mondal H, Mondal S. Sample size calculation to data analysis of a correlation study in Microsoft Excel: a hands on guide with example. Int J Clin Exp Physiol. 2016;3(4):180–189.
- Sim J, Wright CC. The kappa statistic in reliability studies: use, interpretation, and sample size requirements. Phys Ther. 2005;85(3):257–268.
- Evenson KR, Goto MM, Furberg RD. Systematic review of the validity and reliability of consumer-wearable activity trackers. Int J Behav Nutr Phys Act. 2015;12:159.
- Tedesco S, Sica M, Ancillao A, et al. Validity evaluation of the Fitbit Charge2 and the garmin vivosmart HR + in free-living environments in an older adult cohort. JMIR Mhealth Uhealth. 2019;7(6):e13084.
- Chu AH, Ng SH, Paknezhad M, et al. Comparison of wrist-worn Fitbit flex and waist-worn ActiGraph for measuring steps in free-living adults. PLoS One. 2017;12(2):e0172535.
- Treacy D, Hassett L, Schurr K, et al. Validity of different activity monitors to count steps in an inpatient rehabilitation setting. Phys Ther. 2017;97(5):581–588.
- Floegel TA, Florez-Pregonero A, Hekler EB, et al. Validation of consumer-based hip and wrist activity monitors in older adults with varied ambulatory abilities. J Gerontol A Biol Sci Med Sci. 2017;72(2):229–236.
- Brewer W, Swanson BT, Ortiz A. Validity of Fitbit's active minutes as compared with a research-grade accelerometer and self-reported measures. BMJ Open Sport Exerc Med. 2017;3(1):e000254.
- O’Connell S, ÓLaighin G, Quinlan LR. When a step is not a step! Specificity analysis of five physical activity monitors. PLoS One. 2017;12(1):e0169616.
- Yotsukura M, Fujii K, Katayama A, et al. Nine-year follow-up study of heart rate variability in patients with Duchenne-type progressive muscular dystrophy. Am Heart J. 1998;136(2):289–296.
- Marca GD, Frusciante R, Scatena M, et al. Heart rate variability in facioscapulohumeral muscular dystrophy. Funct Neurol. 2010;25(4):211–216.
- WHO. Guidelines on physical activity and sedentary behaviour. Geneva: World Health Organization; 2020.
- Cruz Guzman Odel R, Chavez Garcia AL, Rodriguez-Cruz M. Muscular dystrophies at different ages: metabolic and endocrine alterations. Int J Endocrinol. 2012;2012:485376.
- Jones K, Hawke F, Newman J, et al. Interventions for promoting physical activity in people with neuromuscular disease. Cochrane Database Syst Rev. 2021;5:CD013544.