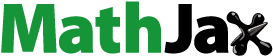
Abstract
Purpose
The current pilot study assesses the use of real-time auditory feedback to help reduce abnormal movements during an active reaching task in patients with chronic stroke.
Materials and methods
20 patients with chronic stroke completed the study with full datasets (age: M = 53 SD = 14; sex: male = 75%; time since stroke in months: M = 34, SD = 33). Patients undertook 100 repetitions of an active reaching task while listening to self-selected music which automatically muted when abnormal movement was detected, determined by thresholds set by clinical therapists. A within-subject design with two conditions (with auditory feedback vs. without auditory feedback) presented in a randomised counterbalanced order was used. The dependent variable was the duration of abnormal movement as a proportion of trial duration.
Results
A significant reduction in the duration of abnormal movement was observed when patients received auditory feedback, F(1,18) = 9.424, p = 0.007, with a large effect size (partial = 0.344).
Conclusions
Patients with chronic stroke can make use of real-time auditory feedback to increase the proportion of time they spend in optimal movement patterns. The approach provides a motivating framework that encourages high dose with a key focus on quality of movement.
Trial Registration: ISRCTN12969079 https://www.isrctn.com/ISRCTN12969079
ISRTCN trial registration REF: ISRCTN12969079
Movement quality during upper limb rehabilitation should be targeted as part of a well-balanced rehabilitation programme.
Auditory feedback is a useful tool to help patients with chronic stroke reduce compensatory movements.
IMPLICATIONS FOR REHABILITATION
Introduction
Stroke is a leading cause of adult disability [Citation1] and upper limb dysfunction is a major contributor to this. Successful rehabilitation of the upper limb requires hundreds of repetitive movement attempts a day [Citation2]. This is hard to achieve in practice owing to a number of factors including limited access to therapists, fatigue, pain, and/or anosognosia [Citation3]. In addition, rehabilitation in the early stages of recovery is typically focused on activities of daily living, independence, adaptive equipment, and home modification to ensure a safe and smooth transition from the hospital. Movement integrity of the affected extremity is often not a main priority during this busy time and patients may rely on compensatory movement to accomplish a task safely and effectively in preparation for discharge. However, prolonged use of compensatory movement can lead to joint contracture, learned non-use and subsequent pain [Citation4] and, as shown in a primate model, can actually limit the extent of impairment reduction [Citation5]. A focus on achieving high quality movement (with a minimum of compensatory movement) is often overlooked, even though approaches which have emphasized training of optimal movement patterns and the reduction of abnormal movements have found large reductions in impairment, even in chronic stroke [Citation6–8].
The aim of the current pilot study was to develop and test an automated digital approach for identifying and signalling abnormal movements using real-time auditory feedback, so that patients could use this information to make postural corrections. The emphasis on self-correction is important: while in a clinical setting, physical harnesses are often used to prevent abnormal movement [Citation9] such an approach does not easily translate to the wider context of home-based rehabilitation, where an awareness of one’s posture and the ability to self-correct is challenging without a therapist. Recent developments around digital technology for rehabilitation have encompassed a range of approaches such as wearable systems [Citation10], biofeedback systems [Citation11] and robotics [Citation12]. These approaches have rarely been used to help correct abnormal movement patterns, and those that have [Citation13] are often cumbersome, expensive, and do not mimic “real-life” situations.
In contrast, the approach developed and tested in the current lab-based study was designed to be low-cost, low-resource and consequently suitable for the home environment in future iterations. The system developed - Sonic Sleeve - uses computer vision and machine learning (ML) algorithms to detect and signal when abnormal movements are occurring. Patients listen to self-selected favourite music while making repetitive target movements. If a repetition includes abnormal movement (predefined through a calibration phase), music is muted (playing in silence) returning to full volume later in the track when the movement is corrected. In a clinical setting, guidance about how to correct would be suggested by a therapist. In contrast, Sonic Sleeve requires participants to determine which postural modification is required, as would be the case in the home environment, where a therapist is not present. Together, the approach combines a motivating and rewarding context for repetitive movement, while incorporating embedded feedback to guide optimal patterns of movement.
In summary, the current study asks whether patients with chronic stroke can make use of auditory feedback (muting of self-selected music) to reduce abnormal movements in a seated active forward reaching task.
Materials and methods
Patients
All patients taking part in the study were enrolled on the Queen Square Upper Limb (QSUL) neurorehabilitation programme [Citation6,Citation14]. 25 patients () were screened with 23 fulfilling the eligibility criteria as follows: (1) diagnosis of stroke resulting in hemiparesis at least 6 months prior to study; (2) ability to give informed consent; (3) ability to follow three-stage commands; (4) ability to lift the affected hand onto a table whilst seated but unaided by their unaffected limb; (5) ability to sit unsupported for at least 10 min; (6) aged between 18 and 75; (7) at least minimal ability to actively extend their elbow. Two patients were excluded as one had no active elbow extension and the other was non-stroke. Two patients dropped out: one due to a family bereavement and the other due to high levels of fatigue. Data for one patient was not collected due to a software failure leaving 20 patients with full datasets. Written informed consent was given by all patients and full ethical approval was attained by the London Dulwich research ethics committee (ref: REC 19/LO/0579).
Figure 1. Flow diagram of patient progress through the pilot study.
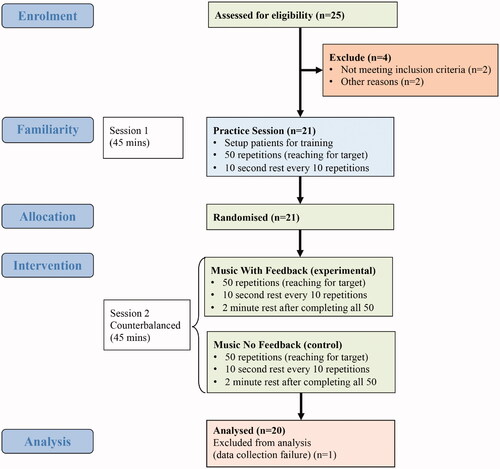
The clinical characteristics of this cohort are shown in . The following outcome measures were assessed as part of QSUL: modified Rankin Scale (mRS), Barthel Index (BI), Neurological Fatigue Index (NFI), Hospital Anxiety and Depression Scale (HADS), Montreal Cognitive Assessment (MoCA), Fugl-Meyer Sensory; modified upper limb Fugl-Meyer (FM-UL); Action Research Arm Test (ARAT); Chedoke Arm and Hand Activity Inventory (CAHAI); Arm Activity Measure (ArmA) both A and B. In order to ensure that patients were able to detect when the music was muted, a sample of music was played and muted at random 10 times. All patients demonstrated that they could detect the onset and offset of the music sample.
Table 1. Demographic and clinical characteristics of patients.
Description of the sonic sleeve system
The Sonic Sleeve system takes kinematic movement data from a 2D webcam using the open-source software OpenPose [Citation15,Citation16] and maps that data to provide real-time auditory feedback to the patient. A ML platform Wekinator [Citation17] was used to record the 2D position data from the patients neck, wrist, elbow and shoulder. In the present study, the auditory feedback was a simple binary signal: the music was either on or off (muted) according to whether the system detected abnormal movements during an active forward reaching task.
Setting system parameters for each patient
Each patient completed a practice session with a physiotherapist (PT) or occupational therapist (OT) from the QSUL programme, along with the researcher. Examples of abnormal movements were recorded into separate ML models, comprising: (i) shoulder abduction (ii) shoulder elevation iii) trunk flexion. Each patient held static positions for five seconds at a time as follows: shoulder abduction by holding the elbow out by ∼15cm; trunk flexion by leaning forward ∼10cm from the back of the chair; and shoulder elevation by raising the shoulder by ∼5cm. These abnormal positions set the maximum upper bounds of the three ML models at 1.0, whereas the lower bounds were set to a value of 0.0 by recording five examples of the target movement performed using the most optimal movement pattern possible. Both the abnormal (upper bound) positions and the optimal movements (lower bounds) were physically supported by the OT or PT when required, ensuring the patients did not become fatigued and to help ensure the upper and lower bounds of the system were set at consistent levels. For example, some patients while undertaking their optimal movements required the OT or PT to guide optimal movement by physically supporting their elbow as they moved. This was to ensure patients movement patterns were optimal and as near normal as possible when recording the kinematic data to set the lower bounds of the system. Feedback thresholds were then set for each of the three abnormal movement ML models in turn and individualised to suit the range of movement for each patient. An OT or PT supported the patient aiming for movement of the affected limb to be as near normal as possible. A precise threshold number was stored in the system for the three supported positions (arm, trunk and shoulder) setting the thresholds above which abnormal movements would trigger real-time feedback. These feedback levels were typically in a range of 0.2–0.3 (i.e., 20 − 30% of the upper bound measurements) and always between the lower bound of 0.0 and the upper bound of 1.0. Abnormal movement above the stored threshold numbers resulted in auditory feedback (muting of the music) until the participant corrected their posture, at which point the music was unmuted.
Study design and protocol
A within-session, within-subject design with two conditions (with auditory feedback vs. without auditory feedback) presented in a randomised counterbalanced order was used. Participants were assigned to their starting condition using a random number generator (https://www.randomizer.org/#randomize) and based on their study ID number.
In the condition with auditory feedback patients were required to undertake 50 repetitions of the target movement – a seated active forward reaching task (). A single repetition began with the mid-point of the ulnar styloid positioned at the edge of the table with elbow in line with the centre of their torso on the hip. From here patients moved their affected limb forward to a button attached to the table (set at an appropriate individualised reaching distance by a PT or OT accounting for varying levels of impairment and elbow extension) before returning to the start position. This movement was undertaken while patients listened to a self-selected favourite piece of music which matched their baseline movement tempo. If a patient used abnormal movement, such as trunk flexion or shoulder abduction, the Sonic Sleeve system automatically muted the music until they corrected their posture. After every 10 repetitions patients took a short rest of 10 s. A longer rest of 2 min was taken between the two study conditions. Patients were made aware that they could rest at any time if they found the exercises too tiring. In the control condition, patients were required to undertake 50 movement repetitions to the same self-selected music, but without any feedback to signal when abnormal movements occurred. Patients were told they did not need to move in time to the music but should focus primarily on movement quality, trying their best to perform the movements with as little abnormal movement as possible. Pre-written scripts were read out by the researcher and available in clear bullet point large font print form for patients who required visual support. Patients undertook the movement repetitions at a comfortable speed that the PT or OT agreed was suitable for their rehabilitation.
Figure 2. The patient seated upright with their wrist on the edge of a height adjusted table. They move their hand forward to reach a target button (marked with X) before returning to the original start position. A 2D webcam positioned at 2 o’clock/60 degrees relative to the patient collects video footage of their movement and sends kinematic data into a machine learning system.
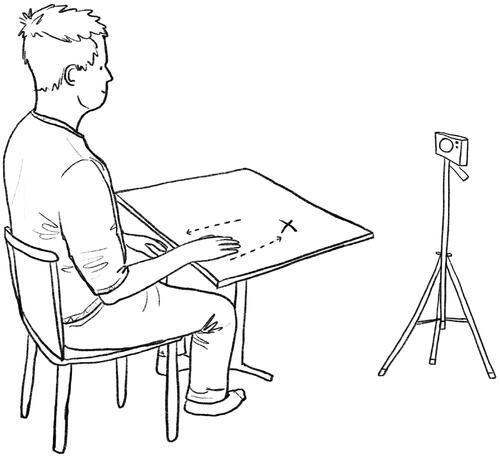
In the practice session preselected music of varying styles was prepared for use, matching the baseline tempo of each patient. Patients undertook 50 movement repetitions with auditory feedback to gain familiarity with the system. The system was calibrated to provide feedback on their abnormal movements such that the movements were moderately challenging. Patients then chose 10 of their favourite pieces of music that would motivate them to perform the movement repetitions during the study. Of these, the researcher selected one that was closest to the patient’s baseline tempo and prepared it for use in the main study session 48 h later.
Data collection and analysis
All data were collected in a research lab alongside the stroke unit where patients were attending the QSUL programme between August 2019 and January 2020. Data file outputs from the Sonic Sleeve system were processed in Python 3.7 (https://www.python.org) using JupyterLab (https://jupyter.org), and SPSS v24 was used to run the statistical tests. The dependent variable was the duration of abnormal movement as a proportion of total movement time. This was calculated for each movement repetition and then averaged across all 50 repetitions. A repeated measures ANCOVA assessed the hypothesis that the presence of auditory feedback would reduce the proportion of time spent in abnormal movement while controlling for any overall differences in movement speed across conditions. A paired samples t-test was run to assess the counterbalanced blocks for potential order effects. The relationship between response to feedback and clinical baseline variables (n = 14 as noted in ) including age, time since stroke and QSUL outcome scores were assessed with Spearman’s rank tests. To assess the 14 baseline variables further Mann-Whitney U tests were run between the 10 highest responders to feedback and the 10 lowest responders. Bonferroni correction (p < 0.05/14 = 0.004) was used to control for multiple comparisons. Further Wilcoxon signed-rank tests were run at the individual participant level to compare the variability across participants.
Results
Clinical baseline scores were available as part of the QSUL routine data set. As seen in , the duration of abnormal movement with auditory feedback was lower 19.3% (SD 18.7%; 95% CI 11.3%–27.3%) compared to without feedback 39.4% (SD = 26.5%; 95% CI 27.5%–51.4%). This was a statistically significant difference, F(1,18) = 9.424, p = 0.007, with a large effect size (partial = 0.344). There was no statistical difference between the two conditions (with feedback vs. without feedback) grouped by block order; t(18) = 0.759, p = 0.461. No associations were found between the magnitude of abnormal movement reduction and clinical baseline variables (p > 0.05). Similarly, the between group tests comparing the 10 highest responders to feedback with the 10 lowest responders on all 14 clinical baseline variables were nonsignificant (p > 0.05).
Figure 3. The duration of abnormal movement for 20 patients undertaking 50 repetitions with auditory feedback compared to 50 repetitions with no feedback. Error bars are adjusted 95% CI removing between-subject variability.
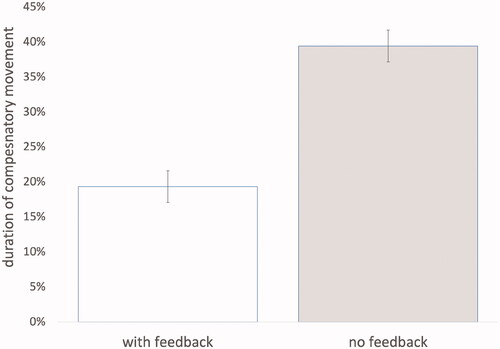
Individual participant level analyses
14 patients showed significant reductions in the duration of abnormal movement with auditory feedback (). Three patients showed no statistically significant difference between conditions and the remaining three patients showed a significant increase in the duration of abnormal movement with auditory feedback.
Figure 4. The duration of abnormal movement for 20 patients who provided full datasets (with and without auditory feedback). Median (dashed line), upper and lower quartiles (dotted lines) are shown.
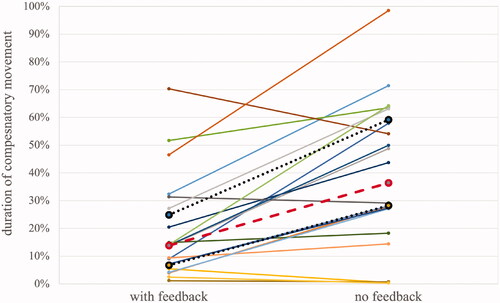
Discussion
The current study assessed a digital approach to upper limb rehabilitation with a focus on quality of movement. At a group level, patients reduced the proportion of time in abnormal movement by 20.1% supporting the hypothesis that auditory feedback would elicit such an effect. The large effect size achieved is comparable to prior research providing real-time force and visual feedback on trunk displacement [Citation18]. In addition, at an individual participant level, 14 of 20 patients achieved statistically significant reductions in this same measure with three patients exhibiting the opposite pattern.
While prior research using real-time feedback has focussed on only one source of abnormal movement – trunk flexion, [Citation19] Sonic Sleeve is capable of detecting abnormal movements from two other common compensatory movement patterns: shoulder abduction and shoulder elevation. While the current study used a single signal to convey abnormal movement from any of these three sources, a future version could, in principle, use different forms of auditory feedback to differentiate abnormal movements coming from each of the three sources, providing more nuanced feedback signals. As the first iteration of Sonic Sleeve, we chose a simple binary manipulation, particularly in order to ensure that the feedback would always be salient given possible perceptual and/or cognitive impairments, but several different parameters of the music could, in principle, be flexibly mapped onto different movement components.
Self-selected music is considered more meaningful to participants than experimenter selected music [Citation20] and provides a motivational framework that can make rehabilitation more enjoyable [Citation21]. In addition to the personal choice that this approach allows, the temporal structure present in almost all music is also a relevant feature. Rhythmic entrainment (the ability to synchronise with the beat of the music) has been linked to strong activations of the auditory and motor regions of the brain [Citation22] and forms the basis of rhythmic auditory cueing (RAC) [Citation23], one of the most widely used approaches for music in neurorehabilitation [Citation24,Citation25]. A protocol devised by van Wijck and colleagues combined self-selected music and RAC using a “tap tempo” paradigm for upper limb rehabilitation with participants required to reach targets in time to the music [Citation26]. In contrast, in the current study, participants were not constrained to entrain to the beat as is the case with RAC because we wanted patients to focus exclusively on reducing abnormal movements rather than trying to keep in time with the music. Nevertheless, the presence of a “beat” was potentially helpful in driving the movement and in our prior research patients achieved hundreds of repetitions every session playing bespoke drum pads as part of a home based rehabilitation programme [Citation27]. The current study used a single piece of music matched to the patient’s baseline tempo, but a more varied playlist could be created for long term rehabilitation to keep interest and encourage variation in the speed of movements. The combination of a motivational medium (self-selected music) with a feedback signal to guide movement towards a more optimal pattern represents a promising approach to focussing on both dose and quality. Although beyond the scope of this initial pilot study, future iterations of Sonic Sleeve with a larger number of participants may help understand what features (such as cognitive deficit or impairment levels) explain those patients that do not respond to feedback.
Rehabilitation training for the upper limb in humans is typically at far lower levels [Citation3] when compared to animal models where many hundreds of daily repetitions are reported [Citation28]. Systems such as Sonic Sleeve can potentially help patients with chronic stroke attain comparably high doses while still focusing on movement quality. Introducing Sonic Sleeve earlier in the acute stages of stroke would be an interesting extension to the current research. Further considerations for the Sonic Sleeve system include moving from the laboratory setting into the home environment and assessing the feasibility of patients receiving auditory feedback from off the shelf motion tracking systems in the home. Sonic Sleeve could also be run off a tablet or smart mobile phone camera lending itself to the home environment at low cost. However, to successfully deploy digital rehabilitation systems at scale that are clinically useful and motivating for patients in the long term an interdisciplinary approach is recommended drawing on expertise and experience from patients, clinicians and engineers.
Conclusions
Patients were able to make use of auditory feedback to self-correct abnormal movement patterns moving towards more normal movement patterns. Embedding this feedback within self-selected favourite music provides a motivational rehabilitation framework, which prioritizes dose and movement quality in combination. This is a promising low cost approach to stroke rehabilitation that can transfer into the home environment with further development.
Acknowledgments
The authors would like to thank all the patients who participated in the study as well as the physiotherapists and occupational therapists from the QSUL programme who helped to recruit and setup the patients for the study: Shauna Feeney, Matthew Pountain, Conor Carville, Rachel Higgins, Fred Baron and Rebecca Wells.
Disclosure statement
No potential conflict of interest was reported by the author(s).
Additional information
Funding
References
- Benjamin EJ, Virani SS, Callaway CW, et al. Heart disease and stroke statistics-2018 update: a report from the American Heart Association. Circulation. 2018;137(12):e67–E492.
- Abdullahi A. Effects of number of repetitions and number of hours of shaping practice during Constraint-Induced movement therapy: a randomized controlled Trial. Neurol Res Int. 2018; 2018:5496408–5496408.
- Lang CE, MacDonald JR, Reisman DS, et al. Observation of amounts of movement practice provided during stroke rehabilitation. Arch Phys Med Rehabil. 2009;90(10):1692–1698.
- Pain LM, Baker R, Richardson D, et al. Effect of trunk-restraint training on function and compensatory trunk, shoulder and elbow patterns during post-stroke reach: a systematic review. Disabil Rehabil. 2015;37:553–562.
- Murata Y, Higo N, Oishi T, et al. Effects of motor training on the recovery of manual dexterity after primary motor cortex lesion in macaque monkeys. J Neurophysiol. 2008;99(2):773–786.
- Ward NS, Brander F, Kelly K. Intensive upper limb neurorehabilitation in chronic stroke: outcomes from the queen square programme. J Neurol Neurosurg Psychiatry. 2019;90(5):498–506.
- Daly JJ, McCabe JP, Holcomb J, et al. Long-dose intensive therapy is necessary for strong, clinically significant, upper limb functional gains and retained gains in severe/moderate chronic stroke. Neurorehabil Neural Repair. 2019;33(7):523–537.
- McCabe J, Monkiewicz M, Holcomb J, et al. Comparison of robotics, functional electrical stimulation, and motor learning methods for treatment of persistent upper extremity dysfunction after stroke: a randomized controlled trial. Arch Phys Med Rehabil. 2015;96(6):981–990.
- Michaelsen SM, Dannenbaum R, Levin MF. Task-specific training with trunk restraint on arm recovery in stroke: randomized control trial. Stroke. 2006;37(1):186–192.
- Wang Q, Markopoulos P, Yu B, et al. Interactive wearable systems for upper body rehabilitation: a systematic review. J Neuroeng Rehabil BioMed Central. 2017;14(1):20.
- Yungher D, Craelius W. Improving fine motor function after brain injury using gesture recognition biofeedback. Disabil Rehabil Assist Technol. 2012;7(6):464–468.
- Huang VS, Krakauer JW. Robotic neurorehabilitation: a computational motor learning perspective. J Neuroeng Rehab. 2009;6(1):1–13.
- Valdés BA, Schneider AN, Van der Loos HFM. Reducing trunk compensation in stroke survivors: a randomized crossover trial comparing visual and force feedback modalities. Arch Phys Med Rehabil. 2017;98(10):1932–1940.
- Kelly K, Brander F, Strawson A, et al. Pushing the limits of recovery in chronic stroke survivors: a descriptive qualitative study of users perceptions of the queen square upper limb neurorehabilitation programme. BMJ Open. 2020;10(10):e036481.
- Cao Z, Simon T, Wei SE, et al. Realtime multi-person 2D pose estimation using part affinity fields. Proc – 30th IEEE Conf Comput Vis Pattern Recognition, CVPR. 2017;2017:7291–7299.
- Wei SE, Ramakrishna V, Kanade T, et al. Convolutional pose machines. Proceedings of the IEEE Computer Society Conference on computer vision and pattern recognition. 2016. p. 4724–4732.
- Fiebrink R, Trueman D, Cook PR. The Wekinator: Software for using machine learning to build real-time interactive systems. 2011.
- Valdés BA, Van der Loos HFM. Biofeedback vs. game scores for reducing trunk compensation after stroke: a randomized crossover trial. Top Stroke Rehabil. 2018;25(2):96–113.
- Valdes Benavides BA. Reducing compensatory movements in stroke therapy through the use of robotic devices and augmented feedback. Vancouver: University of British Columbia; 2017.
- Sihvonen AJ, Särkämö T, Leo V, et al. Music-based interventions in neurological rehabilitation. Lancet Neurol. 2017;16(8):648–660.
- Davis WB, Gfeller KE, Thaut M. An introduction to music therapy: theory and practice. Silver Spring: American Music Therapy Association; 2008.
- Zatorre RJ, Chen JL, Penhune VB. When the brain plays music: auditory-motor interactions in music perception and production. Nat Rev Neurosci. 2007;8(7):547–558.
- Thaut MH, McIntosh GC, Rice RR, et al. Rhythmic auditory stimulation in gait training for parkinson’s disease patients. Mov Disord. 1996;11(2):193–200.
- Altenmüller E, Stewart L. Music supported therapy in neurorehabilitation. In: Dietz V, Ward NS, editors. Oxford textb neurorehabilitation. 2nd ed. Oxford: Oxford University Press; 2020.
- Bradt J, Magee WL, Dileo C, et al. Music therapy for acquired brain injury. Bradt J, editor. Cochrane Database Syst Rev. 2010;2016:CD006787.
- van Wijck F, Knox D, Dodds C, et al. Making music after stroke: using musical activities to enhance arm function. Ann NY Acad Sci. 2012;1252:305–311.
- Kirk P, Grierson M, Bodak R, et al. Motivating stroke rehabilitation through music: a feasibility study using digital musical instruments in the home. Proceedings of the 2016 CHI conference on human factors Computer Systems. 2016. p. 1781–1785.
- Nudo RJ, Wise BM, SiFuentes F, et al. Neural substrates for the effects of rehabilitative training on motor recovery after ischemic infarct. Science. 1996;272(5269):1791–1794.