Abstract
Purpose
To evaluate the effectiveness of mobile health applications (mHealth apps) containing a physical training component on physical function and physical activity in stroke rehabilitation.
Materials and methods
A systematic literature search was conducted in three databases for studies published from inception to 12 July 2022. Clinical trials including mHealth apps with a physical training component were included using outcomes of physical function and physical activity. Quality of life was extracted as a secondary outcome.
Results
Five RCTs, two non-RCTs, and four uncontrolled clinical trials were included with a total of 264 stroke survivors. Eleven apps were identified with a physical training component using features of gamification (six apps), exercise prescription (three apps), and physical activity (two apps). Six out of seven studies reported statistically significant improvements in physical function in favor of the experimental group, with the most robust findings for upper extremity function. For physical activity, statistically significant improvements were seen in the experimental groups. Only one study showed significant improvement in quality of life. Overall study quality was fair.
Conclusions
mHealth apps containing a physical training component are promising for physical function and physical activity in stroke rehabilitation. Further research is warranted to confirm these conclusions.
Design content of mobile apps with a physical training component were focused on gamification, exercise prescription, and physical activity
Using mobile app-delivered therapy seem promising for improving upper extremity function in stroke rehabilitation
Using mobile apps also supported an increase of physical activity in people with stroke
Studies using mobile apps should report more specifically the dosage of physical training and adherence
Using mobile apps seems promising as an additional tool for clinical work, however, more studies are required to understand their effectiveness in stroke rehabilitation.
Implications for rehabilitation
Introduction
Stroke is one of the leading causes of death and long-term disability worldwide, accounting for approximately 12% of total deaths [Citation1]. The absolute number of stroke deaths has increased by 43% in the last 30 years and stroke was the third most common cause of disability worldwide in 2019 [Citation1]. At stroke onset, stroke survivors suffer from very heterogeneous symptoms and signs. Symptoms of stroke vary individually with a wide range of motoric, mental, lingual, sensory, and cognitive impairments that cause functional challenges in daily life and decrease the quality of life (QoL) [Citation2–5]. The most common impairments are paresis experienced in upper (69%) and lower (61%) extremities in the acute stage of stroke [Citation6]. At three years post-stroke, 26% of stroke survivors are still moderately or severely disabled and more than half of the stroke survivors are inactive [Citation7]. Moreover, people with stroke throughout all stages have lower physical activity (PA) levels compared to healthy age-matched individuals [Citation8]. Furthermore, physical inactivity is associated with lower chances of independence in activities of daily living [Citation9]. Also, stroke survivors have reported a decline in QoL [Citation3,Citation10].
To reduce disability after stroke, physiotherapy plays an important role in rehabilitation and the amount of time spent in therapy is highly correlated with functional recovery after stroke [Citation11,Citation12]. In the recent decade, more attention has been given to telerehabilitation, mobile health applications (mHealth apps), or other technological therapy modalities in stroke rehabilitation [Citation13–16]. Due to the rapid increase of smartphone and tablet apps in common daily life, interest in using a mHealth app has also increased in healthcare and rehabilitation services [Citation17]. mHealth app is defined as a health and well-being mobile service delivered using a mobile app or other wireless technology in medical care, which enables two-way health-related information delivery and communication [Citation18]. Interest has grown mainly with the assumption that mHealth apps support specific rehabilitation goals, promote self-management, and increase adherence to home-rehabilitation exercises [Citation19]. Moreover, one systematic review concluded that mHealth apps have the potential to facilitate adherence to chronic disease management in diabetes mellitus, cardiovascular diseases, and chronic lung diseases, but the current evidence of the association between mHealth apps and adherence to disease management is still rather mixed [Citation20]. For instance, only 58% of the studies reported usability, feasibility, or acceptability of mHealth apps in the previous review [Citation20]. Moreover, the usefulness of mHealth apps in stroke rehabilitation is still unclear. Another review provided an overview of the number of commercially available mHealth apps in stroke rehabilitation to offer a low-cost strategy and utility in the rehabilitation of stroke survivors in everyday life [Citation21]. However, the mHealth apps were not reviewed for its scientific evidence on particular outcomes of interest in stroke rehabilitation, such as physical function (PF), PA, and QoL.
Another topic related to the growth of mHealth apps is the various content that the apps can provide for stroke rehabilitation. A previous scoping review listed 39 mHealth apps with a vast heterogeneity in the content of apps, of which the most common focuses were upper extremity function (32%), medical management and secondary prevention (26%), exercises, PA, or mobility (24%) [Citation16]. Other previous scoping or systematic reviews have also indicated similar directions where mHealth apps have been implemented in stroke rehabilitation, namely physical, cognitive, and language rehabilitation [Citation22,Citation23]. These previous reviews show that mHealth apps are becoming an interest of study in stroke rehabilitation and their findings show its potential where such apps have been targeted in stroke rehabilitation [Citation24]. However, previous reviews have not yet focused on particular outcomes such as PF, PA, or QoL. Nor there has not been an overview of mHealth apps that can be targeted to physical training at home settings [Citation23].
The aim of this systematic review is to evaluate the effectiveness of mHealth apps containing a physical training component on PF, PA, and QoL in stroke rehabilitation. Moreover, this review includes apps that have the potential to be applied independently of the therapist.
Methods
Search strategy
A systematic literature search was conducted using three databases: Pubmed, Web of Science, and Scopus for studies published from inception to 16 April 2021. An updated search was conducted from the same databases from studies published between 17 April 2021 and 12 July 2022. A combined flow chart of the study selection is presented in . Inclusion criteria were designed according to the PICOS (patient, intervention, comparison, outcome, study designs) framework as follows: P) adult post-stroke survivors in any stages who experienced an ischemic or hemorrhagic stroke. Post-stroke was defined in four stages (acute: < 2 weeks; subacute: 3 weeks to 11 weeks; early chronic: 12–24 weeks; chronic: > 24 weeks [Citation25–27]), I) intervention using a mHealth app on a smartphone or a tablet containing any type of physical training component (e.g., physical exercises, physical therapy, or physical activity) for the person to use the app independently of a therapist but the help of a caregiver was allowed, C) any type of control group (if applicable), O) any type of outcome measures of PF or PA. A secondary outcome related to QoL was extracted if QoL was a subject of investigation in studies including PF or PA, S) randomized controlled trials (RCTs), non-randomized clinical trials (non-RCTs), or uncontrolled clinical trials published in English. Articles were excluded if the intervention used stationary technological devices such as computers, television screens, or robotics, the mHealth apps were used for assessment, medication control, education, cognitive, or speech rehabilitation, or the mHealth app was only used by a therapist during training. Furthermore, systematic reviews, discussion or short reports, abstracts, qualitative studies, non-clinical trials, and study protocols were excluded from the review.
Figure 1. Flow chart of study selection.
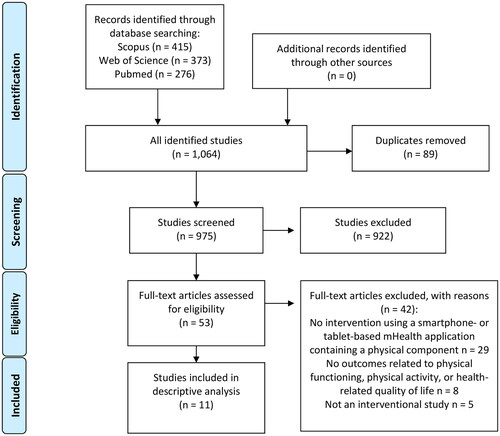
Two researchers (LE and EP) performed the searches in the selected databases. Search terms included keywords describing the technology, rehabilitation, and outcomes of PF, PA, and QoL. An example of original search strategies is described in Supplementary File 1. The search strategy used a medical subject or keyword headings. An additional manual search was conducted using references mentioned in the retrieved studies.
Two reviewers (LE and EP) independently screened the titles and abstracts of the articles in line with the Preferred Reporting Items for Systematic Reviews and Meta-analysis guidelines using the PICOS criteria [Citation28]. In case of doubt, the article was kept and chosen to be screened during the full-text screening. Then, articles were independently evaluated for full-text assessment by two reviewers (LE and EP). A third reviewer (PF or AR) evaluated the studies in case of a disagreement. If needed, corresponding authors of the included studies were contacted for obtaining additional information.
Methodological quality of the studies
Study quality assessment was performed independently by two reviewers (LE and EP), and in case of uncertainty, a third reviewer (PF or AR) was consulted.
For RCTs, methodological quality was assessed using the PEDro scale [Citation29,Citation30]. The scale consists of 11 items and each item is rated (yes or no). If the study met the criterion, the item was rated “yes.” Points were given if a criterion was reported, except for item 1. Therefore, a total score of 10 points was derived where the higher score indicated a higher level of quality. A score below 4 points was considered as “poor,” a score from 4 to 5 as “fair,” a score between 6 and 8 as “good,” and a score between 9 and 10 as “excellent” [Citation29].
For non-RCTs and uncontrolled clinical trials, we used the modified Downs and Black checklist [Citation31,Citation32]. The checklist consists of 27 items and includes domains for study reporting (10 items), external validity (3 items), internal validity (bias and confounding) (13 items), and power (1 item) [Citation31,Citation32], with a total possible score of 28 for randomized and 25 for non-RCTs [Citation31]. An item was scored 1 (Yes) if the criterion was fulfilled or 0 if inadequately reported, unable to determine, or not applicable. Overall quality rating per study was assessed using the corresponding quality levels as previously reported [Citation31]: excellent (26–28); good (20–25); fair (15–19); and poor (≤ 14).
Data extraction and statistical synthesis
The characteristics concerning study methods, participants, type of intervention, name of the mHealth apps, delivery method of the app (mobile phone or tablet), and results were extracted from the included articles and analyzed descriptively. For mHealth apps, we derived the findings into categories based on their content design if possible (e.g., gamification, exercise prescription, or another type). To determine the effectiveness of mHealth apps using a physical training component in stroke rehabilitation on the selected outcomes, we performed a vote counting analysis for RCTs and non-RCTs to compare the number of studies reporting statistically significant findings between experimental and control groups [Citation33].
Results
The literature search identified 975 studies after the duplicate articles were manually removed. Screening of 53 full-text studies revealed 11 studies that fulfilled the inclusion criteria [Citation34–44]. No relevant articles were identified in the additional manual search.
Overall seven (63.6%) controlled clinical trials (five RCTs and two non-RCTs) and four (36.4%) uncontrolled clinical trials were included (). A flow chart of the screening process is presented in . Extracted data are presented in and . Five studies were conducted in Europe (United Kingdom, Spain, the Netherlands, and Israel) [Citation34,Citation38,Citation40,Citation42,Citation44] and three in Asia (South Korea and the Philippines) [Citation36,Citation37,Citation39]. Other studies were conducted in North America (the United States) [Citation41], South America (Chile) [Citation35], and Africa (Ghana) [Citation43].
Table 1. Study design and methods of interventions using a smartphone- or tablet-based mHealth application containing a physical training component (11 studies).
Table 2. Intervention details and results of studies using a smartphone- or tablet-based mHealth application containing a physical training component (11 studies).
Description of the participants
The selected studies included a total of 264 stroke survivors of which 215 reported in the controlled clinical trials and 49 reported in the uncontrolled clinical trials. Of the 215 stroke survivors in the controlled clinical trials, 114 were reported in the experimental group and 101 in the control group. From the total sample, the median (IQR) age of the participants was 59.3 (55.3 to 61.0) years and 41.7% were women. The median (IQR) disease duration was 18.9 (14.8 to 45.6) months. Seven studies (63.6%) included stroke survivors in the chronic stage, two (18.2%) studies subacute stage, one (9.1%) subacute and chronic stages, and one (9.1%) combination of acute, subacute, early chronic, and chronic stages.
Individual studies used different main inclusion criteria for eligibility to participate in an mHealth intervention study. These were defined with measurements of upper extremity impairments [Citation37], ability to move wrist and fingers [Citation36,Citation39,Citation40], functional ambulation classification (FAC) score between 3 and 5 points [Citation38,Citation42], able to use a mobile app independently [Citation41], independent walk with or without using an aid or orthosis [Citation34], balance (e.g., Berg Balance Scale < 50 points out of 56) [Citation35], the level of ADL (e.g., modified Rankin score of 1 to 4) [Citation43], or a score equal to or less than 10 in the Trunk Impairment Scale (TIS) [Citation44]. Hence, measurements of impairment levels varied across studies; however, overall synthesis indicated that most participants had either mild or moderate symptoms of stroke ().
Methodological quality
The overall methodological quality of the studies was fair when taking into account results taken from the PEDro scale and the modified Downs and Black checklist (). For RCTs, a general defect of the methodological quality was blinding procedures. Other single issues related to methodological quality was random allocation [Citation37], concealed allocation [Citation39,Citation44], baseline comparability [Citation37], adequate follow-up (> 85%) [Citation38], and reporting of point measures and measures of variability [Citation37]. For two non-RCTs and four uncontrolled clinical trials, low external validity was observed where the source population was not adequately reported. Other main methodological defects were made concerning internal validity, of which most issues were related to blinding, randomization, and concealment of allocation. Moreover, none of the six studies reported sufficient power to detect the treatment effect at the significance level of 0.05.
Table 3. Methodological quality assessment of studies including mHealth applications containing a physical training component in stroke rehabilitation (11 studies).
Interventions in the experimental groups and control groups
Interventions in the experimental groups
Of the total 11 mHealth apps, eight apps were delivered using a mobile phone and three apps were delivered using a tablet (). The sample size in the interventions ranged from 3 to 66 participants. The training volume ranged between one single session and 12 weeks (median of 5 weeks). Seven (63.6%) out of 11 studies used a smartphone-based mHealth solution in their interventions [Citation34,Citation35,Citation37,Citation38,Citation41–44], of which three studies used apps in a combination with other devices such as inertial movement unit (IMU) sensors [Citation35], tablet [Citation37], and pedometer [Citation38]. Three (27.3%) studies used a tablet-based mHealth solution only [Citation36,Citation39,Citation40] and one (9.1%) study used either an app or a tablet [Citation44]. Concerning the content of mHealth apps in the experimental groups, three main categories were classified (): gaming, exercise prescription, and monitoring. Six (54.5%) out of 11 studies used a gaming app where the intervention was given in a virtual environment either in a hospital setting [Citation36,Citation37,Citation39,Citation40] or at home [Citation35,Citation41]. Three (27.3%) studies used the mHealth app as an exercise prescription (e.g., mobility, upper and lower limb strengthening, sitting, standing balance, walking endurance, and core exercises) which were implemented either completely at home or combination of inpatient and home settings [Citation42–44]. Two (18.2%) studies used a monitoring app registering the levels of PA which both were applied at home [Citation34,Citation38].
Figure 2. Overview of categories for mHealth applications in studies using a smartphone- or tablet-based mHealth application containing a physical training component (11 studies).
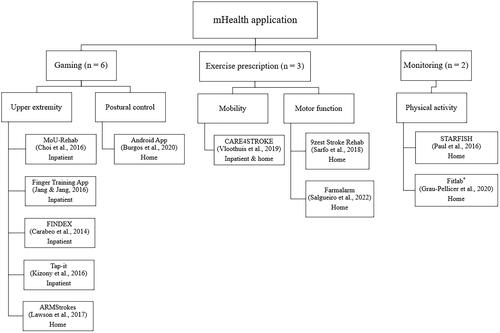
Six (54.5%) out of 11 mHealth apps were personalized to the individual needs of the participants, namely, to modify gaming difficulty level [Citation35,Citation37], set individualized step goals [Citation34], build exercises based on patient goals [Citation42,Citation43], or generate exercises based on the findings of the range of motion and movement [Citation41].
In addition to what other treatments the experimental groups received with the apps, five controlled clinical trials provided usual care consisting of supervised aerobic exercises, task-orientated training, balance training, muscle training, gait and posture training, or stretching exercises [Citation35,Citation37,Citation38,Citation42,Citation44].
Six (54.5%) out of 11 studies reported no adverse events from using the apps during the intervention [Citation34,Citation35,Citation37–39,Citation42]. Five (45.5%) studies did not report whether or not adverse events occurred while using the apps [Citation36,Citation40,Citation41,Citation43,Citation44].
Interventions in the control groups
In five (71.4%) out of seven controlled clinical trials, the control group received usual care given by a trained therapist in clinical settings. Usual care was mainly focused on improving range of motion, muscle strength, gait, trunk stability, and daily life functioning. Two (28.6%) studies did not include any active rehabilitation for the control group [Citation34,Citation39].
Content of mHealth apps containing a physical training component
The content design of the mHealth apps in the experimental groups was heterogeneous. We identified three categories: gaming apps, exercise prescription apps, and monitoring apps (). mHealth apps related to gaming were mostly related to PF for upper extremity (MoU-Rehab, unnamed finger training app, FINDEX, Tap-it, and ARMStrokes) and postural control (unnamed Android app). Exercise prescription apps were related to mobility (CARE4STROKE) and motor function (9zest Stroke Rehab and Farmalarm). Monitoring apps focused on physical activity (Fitlab® and STARFISH). Below we have described the apps more in detail.
Gaming apps
Six (54.5%) out of 11 mHealth apps targeted PF related to the upper extremity and postural control. Only two studies reported or visualized a stationary sitting/standing position of the patient [Citation37,Citation41].
MoU-Rehab app included a mobile upper extremity rehabilitation program containing exercises to improve upper extremity strength, endurance, range of motion, control, speed, and accuracy [Citation37]. The MoU-rehab app contained four mobile game apps. While playing the games viewed on the tablet PC, the smartphone was attached to the patient’s arm to detect upper extremity movements. In this way, participants acquired visual and auditory feedback on their movements. Participants were encouraged to use the app for 30 min five times a week for two weeks alongside traditional occupational therapy of 30 min per week.
An unnamed finger tapping app was built to improve finger motor function [Citation39]. The app consisted of five games containing finger stretching, flexion, extension, opposition, or thumb abduction exercises. Depending on the game, participants had to place, click, lift, or move their fingertips on the tablet screen. One session was programmed six times a week (31 min per session) for four weeks.
Findex app aimed to improve fine finger dexterity based on activities of daily living [Citation36]. When the participant logs in to the app, a house foyer is viewed. In the house foyer, the participant can enter three rooms. These rooms represented three games. The first game aimed to exercise finger control with a task to place pizza toppings on the corresponding spaces within 2 min. The second game targeted finger isolation and coordination where a participant had to tap piano keys using specific fingers. Finally, the third game included a stretching task to increase the range of motion (ROM) in fingers where a participant had to water flower plots by keeping their thumb on the watering can and tapping the flowers that needed water. Participants were asked to use the app for 30 min one to three times a week in conjunction with their standard therapy for four weeks.
Tap-it app aimed to improve hand dexterity [Citation40]. In this game, a participant had to hold their thumb on an anchor while using their other fingers to tap on colored shapes that appear and disappear. The app was used only for one session including two trials.
ARMStrokes app targeted upper extremity motor function which contained two mobile games, namely “Climbing Monkey” and “Astronaut” [Citation41]. A participant could perform eight exercises for the upper extremity by holding the smartphone in their hand to detect upper extremity movements. In the game, a monkey or an astronaut performed a specific task that the participant completed with a correct movement. The goal in the “Climbing Monkey” game was to pick bananas from a tree. The goal of the “Astronaut” game was to explore space. Both games included auditory and vibration feedback when the monkey or the astronaut accomplished the task goal. The amount of training was not reported in the study.
An unnamed android app was developed for the study to improve the postural control of the participants [Citation35]. The smartphone app consisted of six exergames that focused on anterior-posterior stability limits, mediolateral stability limits, sit-to-stand transfer, standing, reactive balance, and postural control. Two wireless IMUs were used where one IMU was positioned at the lumbar level and the other IMU was placed at the anterior thigh of the paretic side. IMUs recorded the movements of the participant which created the possibility to receive feedback from the exercises. Remote app training was programmed for 30 min per session and nine times a week (in a total of 4 h 30 min) in addition to usual physiotherapy of 40 min sessions three times a week for four weeks.
Exercise prescription apps
Three (27.3%) out of 11 mHealth apps targeted PF on mobility and motor function at home or home/inpatient environment.
CARE4STROKE app program aimed at improving mobility which was built as a tool for therapists [Citation42]. A therapist selected a set of standardized exercises presented in the smartphone app, which was executed with a caregiver at home. The app was used at home where the interaction with a therapist was available through telephone, video conferencing, or email when appropriate. Participants were asked to use the app five times a week (30 min per session) with their caregiver for eight weeks.
9zest Stroke Rehab® app was targeted to improve general motor function at home [Citation43]. In the app, a participant received an exercise program consisting of four categories, namely (1) mobility, upper and lower limb strengthening, (2) dexterity, (3) seated and standing balance, and (4) walking endurance. The app was used in combination with supervised inpatient therapy sessions and at home with a caregiver. Participants were encouraged to use the app five times a week (one session 30–60 min) for 12 weeks.
Farmalarm app provided overall 32 exercises (description, photo, and video) designed for core-stability training in a supine position, sitting positing, or sitting on an unstable surface [Citation44]. Exercises were provided in order of difficulty and the participants had the possibility to navigate and choose freely the exercises with an encouragement to perform 10 repetitions of each exercise five days a week for 12 weeks. The app was used at home with a combination of maintaining their usual dose of treatment during the study.
Monitoring apps
Two (18.2%) out of 11 mHealth apps monitored PA using the number of steps per day and/or walking and sitting time at home ().
Fitlab® app aimed to supervise adherence to PA and to reach a moderate-intensity PA level (150 min) per week in addition to twice a week of 1 h exercise program session and regular daily walking for eight weeks [Citation38]. This daily ambulation progressive program at home was monitored with an app and a pedometer was used to register walking distance and walking speed.
STARFISH app was designed as a behavioral change intervention to encourage the participant to become more physically active [Citation34]. The app used a metaphor of a fish tank and virtual groups of four people were represented by colored fish within the fish tank. Real-time feedback was provided in the app. A fish swam and blew bubbles in the virtual fish tank when the corresponding participant was physically active. Each participant had a step count target for five days per week which participants were asked to follow for six weeks.
Effectiveness of mHealth apps on physical function, physical activity, and quality of life in RCTs and non-RCTs
PF
Outcomes of PF were assessed in all controlled clinical trials (five RCTs and two non-RCTs). Overall, five RCTs and one non-RCT reported statistically significant improvement in PF outcomes in favor of the experimental group compared to control groups of usual care or no rehabilitation (). In the next paragraphs, we provide more detailed results on each outcome (percentages are reported from the total of seven controlled clinical trials included in this review).
Upper extremity function was assessed using five different outcomes in two (28.6%) RCTs, namely the manual muscle testing (MMT) of the upper extremity or wrist and fingers, Fugl-Meyer Assessment of the upper extremity (FMA-UE), Brünnstrom stage (B-stage) for the arm and hand, Manual Function Test (MFT), and Purdue Pegboard Test (PPT) [Citation37,Citation39]. In both studies, statistically significant improvements in upper extremity function were achieved by using gaming apps [Citation37,Citation39]. Choi et al. [Citation37] reported statistically significant improvements for upper extremity outcomes in favor of the experimental group compared to usual care alone and Jang and Jang [Citation39] showed similar results only focusing on finger function using a finger tapping app compared to a control group of no training, except for MMT of the finger and wrist flexors.
Lower extremity function was assessed only in one (14.3%) RCT study using the Fugl-Meyer Assessment of the lower extremity (FMA-LE) and the Motricity Index of the lower extremity leg (MI-LE) [Citation42]. Statistically significant differences were not observed in the experimental group using the mHealth app targeted to exercise description for mobility (CARE4STROKE) with usual care compared to usual care alone.
Balance was assessed in two RCTs and one non-RCT (42.9%) using the Mini-BESTest (MBT) and/or Berg Balance Scale (BBS) [Citation35,Citation42,Citation44]. Only Burgos et al. [Citation35] observed a statistically significant improvement in BBS scales in the experimental group compared to usual care when the experimental group used a gaming app targeted to postural control [Citation35]. For other studies or other balance outcomes, statistically significant between-group differences were not reported.
Walking speed was assessed in two RCTs and one non-RCT (42.9%) using the 10-Meter Walking Test (10MWT) [Citation34,Citation38,Citation42]. Walking endurance was assessed in only two clinical trials using the 6-Minute Walking Test (6MWT) [Citation38,Citation42]. For both outcome measures, contradictory results were observed, namely, only one study reported statistically significant improvements for both walking outcomes in favor of the experimental group using a mHealth app for PA monitoring when compared to usual care [Citation38]. Vloothuis et al. [Citation42] and Paul et al. [Citation34] did not observe statistically significant differences between the groups on walking outcomes (mHealth apps targeted to exercise prescription and PA monitoring).
Functional mobility and risk of falling were assessed using the Timed Up and Go (TUG) test in two RCTs (28.6%) [Citation38,Citation42]. Neither studies did not report statistically significant improvements in functional mobility between the groups where experimental groups received mHealth interventions targeted to either PA monitoring [Citation38] or exercise prescription for mobility [Citation42].
Activities of daily living were assessed in three RCTs and one non-RCT (57.1%) using Barthel Index (BI), modified Barthel Index (MBI), or Stroke Impact Scale-mobility (SIS-mobility) [Citation35,Citation37,Citation38,Citation42]. Two studies reported statistically significant improvement in the levels of BI in favor of the experimental groups compared to usual care where the experimental group used a mHealth app targeted to gaming and postural control [Citation35] and PA monitoring [Citation38]. The other two studies did not find statistically significant differences between the experimental group using a mHealth app content of gaming and upper extremity exercises [Citation37] and exercise prescription for mobility [Citation42] and usual care.
Core stability was assessed in one (14.3%) RCT using TIS [Citation44]. Statistically significant improvements in TIS-balance and TIS-total were observed in favor of the experimental group compared to usual care when the experimental group used an exercise prescription app targeted to core-stability training.
Function in sitting and postural assessment were assessed also in one RCT (14.3%) using the Function in Sitting Test (FIST) and the Postural Assessment Scale for Stroke Patients (PASS) [Citation44]. Both outcomes and PASS subscales (mobility and balance) improved in the experimental group but the group difference was not statistically significant.
PA
The level of PA was assessed in two (28.6%) controlled clinical trials (one RCT and one non-RCT) out of seven controlled clinical trials at home settings [Citation34,Citation38]. PA was measured by the number of steps per day and/or walking and sitting time. Both trials showed a significantly higher walking time in the experimental group in comparison to the control group consisting of either no active rehabilitation [Citation34] or usual care [Citation38]. Both studies also used a mHealth app targeted to PA monitoring. Paul et al. [Citation34] reported a statistically significant increase in the number of steps per day and a higher decrease in sitting time in the experimental group compared to a control group with no rehabilitation. Grau-Pellicer et al. [Citation38] reported a statistically significant difference in sitting time in favour of the experimental group compared to usual care.
QoL
As a secondary outcome of mHealth interventions reporting PF or PA outcomes, 4 (36.4%) clinical trials (3 RCTs and 1 non-RCT) out of 11 clinical trials assessed the level of QoL [Citation34,Citation37,Citation38,Citation42]. Health-related QoL was measured by EuroQol-5 Dimensions (EQ-5D), Stroke Specific Quality of Life Scale (SS-QOL), or Stroke Impact Scale-emotion (SIS-emotion). Only one out of four studies reported a statistically significant improvement in QoL (EQ-5D) in the experimental group using an app for PA monitoring compared to a control group of usual care [Citation38] whereas the other three studies did not find differences between the groups when comparing mHealth app intervention to usual care [Citation37,Citation42] and no active rehabilitation [Citation34].
Effectiveness of mHealth apps on physical function, physical activity, and quality of life in uncontrolled clinical trials
All four uncontrolled clinical trials included only PF outcomes (). In three (75.0%) out of four uncontrolled clinical trials, upper extremity function was assessed using the general performance levels achieved on the mHealth app. The performance was measured by time taken and/or accuracy (percentage of successful performance of the app). Overall, higher improvements in performance on the mHealth apps were achieved in all three studies using apps developed for gaming [Citation36,Citation40,Citation41]. Only one study including the mHealth app targeted to exercise prescription reported a statistically significant change within the group after 70 sessions on motor function [Citation43].
Adherence to the use of mHealth apps
Only 4 (36.4%) clinical trials out of 11 studies reported some type of adherence to the use of mHealth apps with very heterogeneous findings [Citation38,Citation40,Citation43,Citation44]. Only one of these studies reported a high level of adherence (an average of 5.7 sessions per week of motor function exercises with a program of 5 sessions per week) [Citation43]. Other two studies reported adherence of 50.0% consisting of daily walking and 150 min per week of moderate physical activity [Citation38] and 14.0% including core-stability exercises five days a week [Citation44]. Also, one study reported that 15 (75.0%) out of 20 participants were able to complete the two trials of the tapping task in the app [Citation40].
Discussion
Our findings from the 11 included studies contained 11 different mHealth apps in stroke rehabilitation. Of those apps, the most key features in the mHealth apps were gaming, exercise, and monitoring. Although mostly our findings were heterogeneous in terms of the content of the apps, duration of the interventions, and sample sizes, findings of this review representing 163 stroke survivors who used a mHealth app containing a physical training component indicated either statistically significant, a similar effect, or a slightly positive trend (improvement but not statistically significant findings) on PF, PA, and QoL. Our results confirm previous systematic reviews that assessed the effects of mobile tablet- and computer-based therapies on physical function in stroke survivors [Citation22,Citation23]. However, previous reviews did not narrow their inclusion to apps including a physical training component. Furthermore, our review focused on interventional studies and non-stationary devices (i.e., portable and remote to be used also in home settings if possible), whereas previous reviews included other study methods and rehabilitation technology that was not clearly defined as remote or portable (i.e., computer-based) [Citation22,Citation23], which could therefore potentially decrease their translation to daily rehabilitation in a home environment.
The most promising evidence was found for upper extremity function in the mHealth app designed for gaming, where both included studies reported statistically significant differences in favor of the experimental group when compared to usual care [Citation37] and no training [Citation39]. Similar findings were also demonstrated in a review by Pugliese et al. [Citation22], where one of the most common therapeutic interventions was targeted to fine motor skills for people with chronic stroke including also studies other than interventional studies (e.g., cohort studies). The difference to our current review is that our inclusion criteria focused on targeted rehabilitation outcomes (i.e., PF) with providing an overview of existing evidence from clinical trials. For other PF outcomes, two out of four studies found statistically significant improvements in activities of daily living in favor of the experimental group compared to usual care when mHealth apps were designed for gaming (postural control) or PA monitoring [Citation35,Citation38]. The other two found no differences between experimental groups and usual care [Citation37,Citation42] which may indicate a similar effect between the groups. These findings are in line with a previous systematic review with a meta-analysis of six RCT studies investigating any type of technology in distance physical rehabilitation interventions, where the authors found a similar effect on activities of daily living compared to traditional treatments [Citation13]. The difference in our review was that our review targeted only to mHealth technology. These findings indicate that rehabilitation technology, including mHealth apps, may have its benefits as an additional treatment strategy to improve activities in daily living in people with stroke [Citation13], but more robust evidence is needed. Continuing with other PF outcomes, our review also found positive trends in walking, balance, and lower extremity function for both groups, which can be viewed as a positive note to continue to develop mHealth apps targeted to people with stroke. Although only a few of the studies reported statistically significant differences in favor of mHealth interventions, other studies found no differences between mHealth app interventions and usual care. These findings encourage us to further research mHealth app interventions targeted to PF to increase more robust evidence. Similar promising findings were reported in Zhou et al. [Citation23] review for mHealth interventions improving physical function in stroke survivors, but the lack of studies challenges further clear recommendations. For instance, our review provided an overview of outcomes in PF only in interventional studies, which on the other hand makes it closer to the rehabilitation settings, but also the observed heterogeneity of the content of the interventions makes it difficult to conclude more precise clinical implications.
Our review also extracted PA outcomes which were measured in two studies using mHealth apps designed for monitoring PA. The level of PA was improved in both experimental groups when the app was applied at home, and when the effect was compared to either no active rehabilitation [Citation34] or usual care [Citation38]. This is also in line with previous systematic reviews that have similar improvements in PA in interventions targeted to all types of technology-based distance rehabilitation compared to usual care and other treatments in stroke and MS rehabilitation [Citation13,Citation45]. It is worthwhile to continue to explore and investigate the use of mHealth apps to target changes and monitor the levels of PA, as PA has been one of the most studied outcomes in stroke rehabilitation with shown benefits. However, it is still less investigated using a mHealth app in a home environment [Citation14,Citation46].
This systematic review demonstrated that it is still too early to make any firm suggestions on the usefulness of mHealth apps using a physical training component in outcomes of QoL although some positive trends were seen in our overview that mHealth apps may improve QoL. Discrepancies between the included studies may be due to the inability to detect a change in QoL surveys for a short clinical trial period, and therefore, may require more longitudinal use of mHealth apps in a research setting. However, this is still early speculation and more research on this aspect is required.
When we view the findings of this review from the key features and training dosages in the apps, we see that the current state of such apps in stroke rehabilitation has been mostly designed for gaming, exercises, or monitoring with a high variety of training dosages reported in the study protocols. Such features are expected to increase in the near future due to the developments of mobile networks and mobile phones, especially when the technology provides more features and higher sophisticated designs to be included in future apps, such as augmented reality [Citation47]. However, our findings on reported levels of adherence indicate that we cannot confirm whether the features and training dosages presented in our review are meaningful. While only four (36%) studies reported any level of adherence with two studies reporting low levels of adherence, there are risks that the apps may not be used as expected. These examples may confound our findings in this review, especially when most of the studies did not report the level of adherence to the use of the app. Reporting adherence in future studies is crucial to understand to whom such mHealth apps are more feasible and whether such apps are needed to target some specific content and training dosages. Also, involving key stakeholders (e.g., stroke survivors and health care professionals) in the development process of mHealth features are strongly recommended to take into account the voice of the users.
The overall methodological quality of the included trials was fair. The included controlled clinical trials had mainly inadequate quality for selection bias, performance bias, and co-intervention bias. Concerning selection bias, the majority of included controlled clinical trials had a limited sample size (ranging from 3 to 66 participants) which lowers the statistical power. This may partly explain also the null findings of several included studies, as a low sample size study has the risk to miss a significant effect [Citation48]. Regarding performance bias, 7 out of 11 studies did not apply or reported blinding of participants and/or therapists. Given the type of the interventions, the difficulty of blinding participants or therapists is comprehensible. Lastly, participants in four studies simultaneously received also usual care as a standard treatment alongside the mHealth app (co-intervention bias) which can be considered a confounding factor to conclude the robust effectiveness of mHealth apps in stroke rehabilitation.
Studies in our review included mostly participants with chronic stroke and mild or moderate disability of stroke with the ability to function independently from most of the daily life activities or were independently ambulatory. Also, when we look at the findings from the eligibility criteria of participants included in the selected studies, 8 out of 11 clinical trials reported an inclusion criterion of no severe cognitive impairment at baseline, and three included studies excluded stroke survivors with visual disturbances. These aspects suggest that mHealth solutions may not be suitable for a proportion of stroke survivors and this aspect is required to take into account when designing such rehabilitation interventions. For instance, up to one-third of stroke survivors develop some form of cognitive impairment and up to 65% develop visual impairment early after stroke [Citation49,Citation50]. However, one positive note was that mHealth apps may be opted for many purposes for people with chronic stroke and can be also applied in a home setting.
The strength of this systematic review is its focus on mHealth applications including a physical training component, which gives the first-in-kind overview of such mHealth applications in stroke rehabilitation. Also, this review followed the guidelines of the Preferred Reporting Items for Systematic Reviews and Meta-analysis (PRISMA) using a pre-defined PICOS strategy [Citation28]. However, this systematic review also contains some limitations. First, a selection bias cannot be ruled out during the literature screening of this systematic review. When studies did not explicitly report that the interventions applied an mHealth app-delivered therapy, these studies were excluded. It may be plausible that some studies were not screened due to a lack of reporting in the abstract of the published article. Second, the results of this systematic review are weakened due to a lack of included high-evidence research and a low sample size of individual studies. Lastly, the generalizability of the results is also limited due to most studies published in developed countries and the small number of included trials with heterogeneity in the content of mHealth apps and therapy modalities. Once more studies are published, these factors can be analyzed using meta-analysis or metaregression to identify the underlying mechanisms of the effects. These aspects diminish a firm conclusion of the benefits of mHealth apps in stroke rehabilitation.
Current research supports the use of mHealth as an additional tool alongside traditional care on physical function and physical activity for stroke survivors. However, this review was limited to the information provided in each study, especially adherence was poorly reported in the included studies. Also, the content and availability of these apps for commercial use may have changed during or after this review. Other aspects that may increase the challenges of using mHealth apps in clinical care are possible additional costs to use the app and sufficient mobile or internet connectivity in the patient’s living environment. Future studies are encouraged to report more specific details such as adherence, availability of the app, costs of the apps, and the feasibility of the app to be used in clinical care.
Conclusion
The use of mHealth apps containing a physical training component on physical function and physical activity is promising in stroke rehabilitation and can be considered as additional support for post-stroke care. Further high-quality RCT studies are needed to determine the benefits of mHealth-only interventions and their indicators thereof compared to traditional therapy treatments.
Disclosure statement
No potential conflict of interest was reported by the author(s).
References
- Vos T, Lim S, Abbafati C, et al. Global burden of 369 diseases and injuries in 204 countries and territories, 1990–2019: a systematic analysis for the Global Burden of Disease Study 2019. Lancet. 2020;396(10258):1204–1222.
- Almhdawi KA, Alazrai A, Kanaan S, et al. Post-stroke depression, anxiety, and stress symptoms and their associated factors: a cross-sectional study. Neuropsychol Rehabil. 2021;31(7):1091–1104.
- Cameron LJ, Wales K, Casey A, et al. Self-reported quality of life following stroke: a systematic review of instruments with a focus on their psychometric properties. Qual Life Res. 2022;31(2):329–342.
- Embrechts E, Van Criekinge T, Schröder J, et al. The association between visuospatial neglect and balance and mobility post-stroke onset: a systematic review. Ann Phys Rehabil Med. 2021;64(4):101449.
- Thilarajah S, Mentiplay BF, Bower KJ, et al. Factors associated with post-stroke physical activity: a systematic review and meta-analysis. Arch Phys Med Rehabil. 2018;99(9):1876–1889.
- Yew KS, Cheng EM. Diagnosis of acute stroke. Am Fam Physician. 2015;91(8):528–536.
- Patel MD, Tilling K, Lawrence E, et al. Relationships between long-term stroke disability, handicap and health-related quality of life. Age Ageing. 2006;35(3):273–279.
- Fini NA, Holland AE, Keating J, et al. How physically active are people following stroke? Systematic review and quantitative synthesis. Phys Ther. 2017;97(7):707–717.
- Rist PM, Capistrant BD, Mayeda ER, et al. Physical activity but not BMI predicts less disability before and after stroke. Neurology. 2017;88(18):1718–1726.
- Sturm JW, Donnan GA, Dewey HM, et al. Quality of life after stroke: the North East melbourne stroke incidence study (NEMESIS). Stroke. 2004;35(10):2340–2345.
- Foley N, Mcclure JA, Meyer M, et al. Inpatient rehabilitation following stroke: amount of therapy received and associations with functional recovery. Disabil Rehabil. 2012;34(25):2132–2138.
- Kuliński W, Bębenek O. Evaluation of the effects of physical therapy on everyday functioning in stroke patients. Wiad Lek. 2018;71(8):1497–1503.
- Rintala A, Päivärinne V, Hakala S, et al. Effectiveness of technology-based distance physical rehabilitation interventions for improving physical functioning in stroke: a systematic review and meta-analysis of randomized controlled trials. Arch Phys Med Rehabil. 2019;100(7):1339–1358.
- Thilarajah S, Clark RA, Williams G. Wearable sensors and mobile health (mHealth) technologies to assess and promote physical activity in stroke: a narrative review. Brain Impair. 2016;17(1):34–42.
- Dicianno BE, Parmanto B, Fairman AD, et al. Perspectives on the evolution of mobile (mHealth) technologies and application to rehabilitation. Phys Ther. 2015;95(3):136–139.
- Burns SP, Terblanche M, Perea J, et al. mHealth intervention applications for adults living with the effects of stroke: a scoping review. Arch Rehabil Res Clin Transl. 2021;3(1):100095.
- Istepanian RS, Lacal JC. Emerging mobile communication technologies for health: some imperative notes on m-health. In: Proceedings of the 25th Annual International Conference of the IEEE Engineering in Medicine and Biology Society (IEEE Cat. No. 03CH37439); 2003 Sep 17-21; Cancun, Mexico. IEEE; 2003. p. 1414–1416.
- World Health Organization. mHealthNew horizons for health through mobile technologies. Global observatory for eHealth series. Vol. 3. Geneva: WHO; 2011.
- Ramey L, Osborne C, Kasitinon D, et al. Apps and mobile health technology in rehabilitation: the good, the bad, and the unknown. Phys Med Rehabil Clin N Am. 2019;30(2):485–497.
- Hamine S, Gerth-Guyette E, Faulx D, et al. Impact of mHealth chronic disease management on treatment adherence and patient outcomes: a systematic review. J Med Internet Res. 2015;17(2):e52–15.
- Ortega-Martín ME, Lucena-Antón D, Luque-Moreno C, et al. Aplicaciones móviles En El abordaje terapéutico del ictus: Revisión En repositorios comerciales Y búsqueda De evidencia. Rev Esp Salud Pública. 2019;93:e201906035.
- Pugliese M, Ramsay T, Johnson D, et al. Mobile tablet-based therapies following stroke: a systematic scoping review of administrative methods and patient experiences. PLoS One. 2018;13(1):e0191566.
- Zhou X, Du M, Zhou L. Use of mobile applications in post-stroke rehabilitation: a systematic review. Top Stroke Rehabil. 2018;25(7):489–499.
- Whitehead L, Seaton P. The effectiveness of self-management mobile phone and tablet apps in long-term condition management: a systematic review. J Med Internet Res. 2016;18(5):e4883.
- Cramer SC. Repairing the human brain after stroke: I. Mechanisms of spontaneous recovery. Ann Neurol. 2008;63(3):272–287.
- Wu P, Zeng F, Li YX, et al. Changes of resting cerebral activities in subacute ischemic stroke patients. Neural Regen Res. 2015;10(5):760–765.
- Rehme AK, Eickhoff SB, Rottschy C, et al. Activation likelihood estimation meta-analysis of motor-related neural activity after stroke. Neuroimage. 2012;59(3):2771–2782.
- Moher D, Shamseer L, Clarke M, et al. Preferred reporting items for systematic review and meta-analysis protocols (PRISMA-P) 2015 statement. Syst Rev. 2015;4(1):1–9.
- de Morton NA. The PEDro scale is a valid measure of the methodological quality of clinical trials: a demographic study. Aust J Physiother. 2009;55(2):129–133.
- Maher CG, Sherrington C, Herbert RD, et al. Reliability of the PEDro scale for rating quality of randomized controlled trials. Phys Ther. 2003;83(8):713–721.
- Hooper P, Jutai JW, Strong G, et al. Age-related macular degeneration and low-vision rehabilitation: a systematic review. Can J Ophthalmol. 2008;43(2):180–187.
- Downs SH, Black N. The feasibility of creating a checklist for the assessment of the methodological quality both of randomised and non-randomised studies of health care interventions. J Epidemiol Community Health. 1998;52(6):377–384.
- Verbeek J, Ruotsalainen J, Hoving JL. Synthesizing study results in a systematic review. Scand J Work Environ Health. 2012;38(3):282–290.
- Paul L, Wyke S, Brewster S, et al. Increasing physical activity in stroke survivors using STARFISH, an interactive mobile phone application: a pilot study. Top Stroke Rehabil. 2016;23(3):170–177.
- Burgos PI, Lara O, Lavado A, et al. Exergames and telerehabilitation on smartphones to improve balance in stroke patients. Brain Sci. 2020;10(11):773.
- Carabeo CGG, Dalida CMM, Padilla EMZ, et al. Stroke patient rehabilitation: a pilot study of an android-based game. Simul Gaming. 2014;45(2):151–166.
- Choi Y-H, Ku J, Lim H, et al. Mobile game-based virtual reality rehabilitation program for upper limb dysfunction after ischemic stroke. Restor Neurol Neurosci. 2016 May;34(3):455–463.
- Grau-Pellicer M, Lalanza JF, Jovell-Fernández E, et al. Impact of mHealth technology on adherence to healthy PA after stroke: a randomized study. Top Stroke Rehabil. 2020;27(5):354–368.
- Jang SH, Jang WH. The effect of a finger training application using a tablet PC in chronic hemiparetic stroke patients. Somatosens Mot Res. 2016;33(2):124–129.
- Kizony R, Zeilig G, Dudkiewicz I, et al. Tablet apps and dexterity: comparison between 3 age groups and proof of concept for stroke rehabilitation. J Neurol Phys Ther. 2016;40(1):31–39.
- Lawson S, Tang Z, Feng J. Supporting stroke motor recovery through a mobile application: a pilot study. Am J Occup Ther. 2017;71(3):7103350010p1–7103350010p5.
- Vloothuis JDM, Mulder M, Nijland RHM, et al. Caregiver-mediated exercises with e-health support for early supported discharge after stroke (CARE4STROKE): a randomized controlled trial. PLoS One. 2019;14(4):e0214241.
- Sarfo FS, Adusei N, Ampofo M, et al. Pilot trial of a tele-rehab intervention to improve outcomes after stroke in Ghana: a feasibility and user satisfaction study. J Neurol Sci. 2018;387:94–97.
- Salgueiro C, Urrútia G, Cabanas-Valdés R. Influence of core-stability exercises guided by a telerehabilitation app on trunk performance, balance and gait performance in chronic stroke survivors: a preliminary randomized controlled trial. IJERPH. 2022;19(9):5689.
- Rintala A, Hakala S, Paltamaa J, et al. Effectiveness of technology-based distance physical rehabilitation interventions on physical activity and walking in multiple sclerosis: a systematic review and meta-analysis of randomized controlled trials. Disabil Rehabil. 2018;40(4):373–387.
- Wendel-Vos GCW, Schuit AJ, Feskens EJM, et al. Physical activity and stroke. A meta-analysis of observational data. Int J Epidemiol. 2004;33(4):787–798.
- Mubin O, Alnajjar F, Jishtu N, et al. Exoskeletons with virtual reality, augmented reality, and gamification for stroke patients’ rehabilitation: systematic review. JMIR Rehabil Assist Technol. 2019;6(2):e12010.
- Button KS, Ioannidis JPA, Mokrysz C, et al. Power failure: why small sample size undermines the reliability of neuroscience. Nat Rev Neurosci. 2013;14(5):365–376.
- Hepworth L, Rowe F, Walker M, et al. Post-stroke visual impairment: a systematic literature review of types and recovery of visual conditions. Opthamol Res. 2016;5(1):1–43.
- Mijajlović MD, Pavlović A, Brainin M, et al. Post-stroke dementia - a comprehensive review. BMC Med. 2017;15(1):11–12.