Abstract
Purpose
Producing triggers to switch between modes of myoelectric prosthetic hands has proven to be difficult. We evaluated whether digital training methods were feasible in individuals with an upper limb defect (ULD), whether myosignals in these individuals differ from those of non-impaired individuals and whether acquired skills transfer to prosthesis use.
Materials and methods
Two groups participated in a 9-day pre-test–post-test design study with seven 45-minute training sessions. One group trained using a serious game, the other with their myosignals digitally displayed. Both groups also trained using a prosthesis. The pre- and post-tests consisted of an adapted Clothespin Relocation Test and the spherical subset of the Southampton Hand Assessment Procedure. After the post-test, the System Usability Scale (SUS) was administered. Clinically relevant performance measures and myosignal features were analysed.
Results
Four individuals with a ULD participated. SUS-scores deemed both training methods feasible. Three participants produced only a few correct triggers. Myosignals features indicated larger variability for individuals with a ULD compared to non-impaired individuals (previously published data [Citation1]). Three participants indicated transfer of skill.
Conclusions
Even though both training methods were deemed feasible and most participants showed transfer, seven training sessions were insufficient to learn reliable switching behaviour.
Trial registration: The study was approved by the medical ethics committee of the University Medical Center Groningen (METc 2018.268).
Switching between pre-programmed modes of a myoelectric prosthetic hand can be learned, however it does require training.
Serious games can be considered useful training tools for trigger production in early phases of myoelectric prosthesis control training.
In order to evoke transfer of skill from training to daily life both task-specificity and focus of attention during training should be taken into account.
Implications for rehabilitation
Introduction
A substantial proportion of people with a congenital upper extremity deficiency or an acquired upper limb defect (ULD, either congenital or acquired) are fit with a prosthesis to (partially) restore arm and hand function. Prosthesis users can choose a prosthesis that is controlled through surface electromyography (sEMG), also called myosignals. The original myoelectric prostheses had only one controllable joint to open and close the hand, whereas the modern generations of myoelectric prosthetic hands possess multiple joints [Citation2]. These multi-articulating hands offer pre-programmed modes which give the user different grip-types such as pinch grip, key grip, index pointing [Citation3] and wrist rotation.
The introduction of multi-articulating hands provided the users with a challenge: controlling multiple modes using a limited number of myosignals. Several control mechanisms have been developed to overcome this issue, such as conventional control using switches (CCS), machine learning control, ultrasound, or foot control [Citation4–6]. Here we focus on the CCS strategy, which is currently used by the vast majority of users of multi-articulating hands. In the CCS two myosignals, measured by two electrodes on either flexor or extensor muscles, are used to produce sequential-proportional signals [Citation7,Citation8]. That is, in CCS, a mode (hand grip type or wrist movement) has to be selected using a trigger signal, such as double pulses (DP) or co-contractions (CO). After producing a trigger, the hand switches to a mode that can be controlled proportionally. Proportional control here means that the opening and closing speed are proportionally related to the amplitude of the myosignals.
Even though individuals with a ULD use multi-articulating hands with CCS quite commonly in their daily lives, only limited knowledge is developed on how to train the production of triggers. In our previous studies on trigger production, we investigated non-impaired individuals. In the current study, we focused on individuals with a ULD who followed a 9-day protocol with the goal of learning to produce triggers using two different training methods. For the proportional control part of CCS, studies showed that task specificity appeared to be important to achieve transfer of skills acquired in a digital environment to actual prosthesis use [Citation9,Citation10]. These results in non-impaired people imply that actual prosthetic users should train (parts of) the specific task that they aim to perform with the prosthesis in daily life. In order to extend the knowledge on proportional as well as switching control we previously showed that task specificity appeared to be less important when training to produce triggers [Citation1]. Participants trained switching using an actual prosthesis while their myosignals were displayed on a screen or they trained in a digital gaming environment controlled with their myosignals. Training improved the production of the number of accurate switches with an actual prosthesis independent of the type of training received [Citation1]. What stood out in these findings was that even though switching performance improved, it was unclear which aspects of the myosignals participants adjusted to achieve this improvement. This was striking, since in order to meet the requirements of a switch, myosignals with specific characteristics have to be generated (e.g., the amplitude of the peaks in the myosignals needs to reach a certain level and the peaks need to be precisely timed). For the current study, we examined training of switching behaviour in individuals with a ULD to establish whether the findings in able-bodied participants on training of switching are different. Here we focused on the same digital/virtual environment as used previously [Citation1,Citation11], but now this training was combined with training with an actual prosthesis. Digital environments have some advantages which make them interesting for rehabilitation training programs. They (1) provide (augmented) feedback on an individual level, (2) increase the training intensity which is crucial for consistent and dexterous prosthesis use [Citation12,Citation13], and because minimal supervision is required, users may (3) begin training prior to prosthesis fitting by placing electrodes on muscle groups using elastic bands.
The current study investigated individuals with a transradial upper limb defect using an upper limb prosthesis to meet three aims: (1) assessing the feasibility of training switching behaviour in a virtual environment, (2) comparing (changes in) the myosignal characteristics over training with results of non-impaired participants, and (3) assessing the transfer of skills acquired in virtual training to real prosthesis use.
Methods
Participants
Patient recruitment was performed within a consortium of multiple studies (see also [Citation14]). Information letters and application forms were sent to patients from two Dutch rehabilitation departments: department for Rehabilitation Medicine of the University Medical Center Groningen (UMCG), Groningen, and the Hoogstraat Rehabilitation, Utrecht, both in the Netherlands. After completing applications, patients were assigned to the study for which they met the inclusion criteria.
Four persons with a ULD using a myoelectric prosthesis were recruited. These users had a well-fitting socket with two sEMG electrodes and a one-degree-of-freedom hand that could be detached from the socket all participants were 18 years or older, had a unilateral ULD below the elbow, had no co-morbidities influencing the study outcomes and spoke Dutch. All four participants were unfamiliar with using a multi-articulating prosthetic hand and had no experience with producing triggers. The measurements were performed at the department of Human Movement Sciences of the University of Groningen (RUG), the department of Rehabilitation Medicine of the UMCG or at OIM Groningen (orthopaedic workplace). Written informed consent was obtained from all participants prior to the start of the study. Using a randomization program in Matlab (2019b, Mathworks Inc, Natick, MA, USA) the participants were randomly assigned to one of two experimental groups; the game group that trained using a game controlled by myosignals or the EMG group that trained their myosignals while they were displayed on a screen. The study was conducted in agreement with the Declaration of Helsinki and approved by the medical ethics committee of the University Medical Center Groningen (METc 2018.268). Travel expenses were reimbursed. Upon completion of all training sessions and the pre-test and post-test, each participant received €500.
We compared the results of the four individuals with a ULD with able-bodied individuals (Aim 2). To this end, we used previously collected data from non-impaired participants. Data concerning co-contractions have previously been published [Citation1]), whereas the data concerning double pulse switches has not been published before. The able-bodied participants were mostly students, of 18 years or older, who were right handed, had normal or corrected to normal vision, were free of any (history of) disorders of the arms and upper body and had no prior experience in using a myoelectric device.
Of the 30 non-impaired participants, 20 trained producing co-contraction triggers using either the same game as the game group in the current study (10 participants) or the identical myosignal displaying as the current EMG group (10 participants). The remaining 10 participants trained producing the double pulse trigger using a prosthesis hand (i-Limb Revolution Ultra (Touch Bionics) mounted on a prosthesis simulator (see also [Citation15–17]). All the 30 individuals participated in a pre-test–post-test design study with five training sessions on consecutive days.
Design
A pre-test, post-test design study with seven training sessions was applied in the current study. Before the pre-test, a familiarization session took place to introduce the participants to the triggers they should produce. After the post-test feasibility of the training programs and participants’ experiences during the training were evaluated using questionnaires (see Procedure & materials).
Procedure & materials
Familiarization
During the familiarization session, an iLimb Ultra (Touch Bionics) prosthetic hand was attached to the participants’ socket. This multi-articulating hand allows 18 different grip types. Two electrodes were used to measure the sEMG of two muscle groups: the extensors and flexors of the wrist. The hand was set to respond to three trigger types; co-contraction, double pulse and hold open. For co-contractions, both the flexor and the extensors muscles had to be contracted and relaxed simultaneously, creating a fairly short, sharp myosignal peak in both signals. For double pulses two short consecutive muscle contractions with the extensors of the wrist had to be produced while the flexors remained relaxed. For hold open, the extensor muscles had to be contracted during three consecutive seconds. During the familiarization phase, the hand was connected via Bluetooth to the BioSim software (Touch Bionics, Livingston, UK) to show the participants their myosignals. They received verbal instructions about how to produce the trigger signals (co-contractions and double pulse). After the instructions they had six try-out sessions of 1.5 min each to produce the co-contraction and double pulse signals and to get a sense of how the hand responded. During these 6 sessions, co-contraction (3 sessions) and double pulse (3 sessions) were alternated. Hold open was not trained because this trigger was only used as a reset trigger during the actual training sessions.
Questionnaires: System usability scale (SUS)
This 10-item questionnaire was developed to score usability of a product or system [Citation18]. No specific questionnaire exists to evaluate trigger production and the SUS was previously used to evaluate usability and feasibility of usage of wrist angles and forces to control a serious game [Citation19]. Therefore, we used this scale to score the feasibility of training CCS using a virtual environment. Participants could score 10 statements using one of five responses ranging from ‘strongly agree’ to ‘strongly disagree’. A score between 0 and 100 is calculated based on the answers [Citation18]. Bangor et al. [Citation20] introduced an ‘acceptability range’ to the SUS scores consisting of; Not acceptable 0–50, Marginal low 50–62, Marginal high 62–70, and Acceptable 70–100.
Questionnaire with open questions
We asked eight open questions about users’ experiences during the training. Since these questions were not the focus of this study, they will not be further discussed in this article.
Pre- and post-test
The pre-test was conducted on the same day as the familiarization session. The post-test was planned at least 48 h and maximally 1 week after the last training session. During both the pre- and post-test participants wore their own socket with the iLimb Ultra attached to it. With this prosthetic hand, participants performed the Partial-SHAP (Partial-Southampton Hand Assessment Procedure) and an adapted Clothespin Relocation Test (CRT) [Citation21].
Partial-SHAP: The spherical subset [Citation22] from the original SHAP (see [Citation23,Citation24]) was used. This spherical subset consists of two abstract object tasks: grasping and transferring a heavy sphere and grasping and transferring a light sphere, and two activities of daily living tasks; opening a jar and pouring water from a carton. Participants received the instructions as originally supplied with the SHAP. At the start of each task the participants started the timer (part of the SHAP set-up) and after completing the task they stopped that timer, resulting in a completion time that was transformed in a score on each task. The order of the four tasks was similar for all participants and also for the pre- and post-test.
Adapted CRT: Since this study focused on mode-switching behaviour we adapted the original CRT [Citation21] in order to force participants to switch between grip-types when placing subsequent clothespins (cf. [Citation1]). Before starting, the prosthesis hand was set in the neutral mode. The participants were instructed to replace a total of six clothespins: three horizontally placed red pins and three vertically placed yellow pins, in alternating order. The horizontally placed pins needed to be moved to the vertical bar using the lateral grip which could be achieved by performing a co-contraction. The vertically placed pins had to be moved to the horizontal bar by using the fine pinch grip mode, which could be selected by producing a double pulse. For all six triggers, the participants had a time window of one minute per trigger. If no successful trigger was made within this time window, a hold open signal had to be made to return to the neutral mode. If a correct trigger was produced within the given time, the participant was allowed to finish the relocation of the pin even if the total attempt of relocating this pin would take longer than the permitted 60 s. Both the time needed to relocate all clothespins and the number of times a clothespin was dropped were noted.
Training sessions
All participants performed seven training sessions within a period of 3 weeks. The training sessions lasted approximately 45 min each. Each training session consisted of two parts: the Virtual training in which no prosthesis was used (socket was doffed) and the Functional training performed with the prosthesis donned. The Virtual training differed between the two groups: (1) the game group played a serious game using their myosignals to control a virtual grabber by producing both trigger signals and proportional control, (2) the EMG group trained producing trigger signals with their myosignals while observing their myosignal patterns displayed on a computer screen. The second part of the training was similar for both groups and consisted of a functional training. In order to perform this part of the training, the participant put their socket on and the researcher attached the multi-articulating hand.
Virtual training
For the first part of each training session, game group and EMG group participants were fitted with two sEMG electrodes (13E200 electrodes, MyoBock, Otto Bock Healthcare Products, Vienna, Austria) which were placed on the indentations left by the electrodes of their own socket. These electrodes were kept in place using 1–2 elastic sweatbands. The electrodes were connected to a MyRio using a Custom LabView software (National Instruments Corporation, Austin, TX, USA). Custom LabView software on the MyRio was used to digitally filter (band filter, cut-off frequency 10 Hz; low pass filter, cut-off frequency 35 Hz) and normalize the signals (between 0 and 1). For this last step, participants were asked to flex and extend their wrist slowly until they reached their maximum force. The normalized signal was fed from the MyRio to a laptop computer via wireless User Datagram Protocol at 200 Hz.
Virtual training: game group
Using the normalized myosignals the game group participants played a serious myogame consisting of three phases: (A) following a light beam by controlling a laterally moving platform, (B) switching from the platform to a virtual grabber, and (C) catching falling objects with the virtual grabber (for image see [Citation1]). In part A, participants were instructed to use their myosignals to move the platform in order to follow the appearing light beam as closely as possible. The speed of the platform was proportionally related to the amplitude of the myosignals. Contracting the extensors of the wrist would move the platform to the right and contracting the flexors of the wrist would move the platform to the left. The direction and speed of the light beam did not change within a trial. Over trials direction and speed of the light beam were randomly changed by the game.
In part B, a trigger had to be produced in order to switch the platform into a grabber. The colour of the cannon at the top of the screen indicated the type of switch (co-contraction or double pulse). An hourglass indicated the 15 s time window in which the participants had to perform the trigger. If the trigger was performed correctly, the plateau would switch into a grabber for part C. However, if no correct trigger was performed the screen would show part C, but no action could be performed. If a correct trigger was made, the emerged grabber could be opened and closed using the two myosignals.
In part C, participants were instructed to catch the object falling from the top of the screen using the grabber. The falling objects differed in size and breakability between trials. Closing the grabber too quickly resulted in crushing the object, opening the grabber too wide would first induce shaking (at 1.5 times the diameter of the object) and if opened wider (1.7 times the size of the object) this would result in short-circuiting (indicated with sparks) and thus malfunctioning of the grabber (cf. [Citation10]). Participants were instructed to scale the aperture and the opening and closing speed of the grabber to the object size and breakability. The opening and closing speed of the grabber were proportionally related to the amplitude of the myosignal. The three phases together formed one repetition of the game group’s task. One level of the game consisted of six repetitions of the A–B–C phases. For more details about this game, we refer to [Citation1]. In total, six levels were played during the training, in which the co-contraction levels and the double pulse levels in part B were alternated.
Virtual training: EMG group
Participants were instructed to produce co-contractions and double pulses while looking at their normalized myosignals displayed on a screen in front of them. Each training session consisted of six blocks of 2 min, alternated with 30 s of rest. During the even numbered blocks, participants were instructed to make as many co-contractions as possible. During the uneven numbered blocks, participants were asked to perform double pulses. The participants received feedback on their switching performance in the form of blocks appearing around correct triggers on the screen. When a correct co-contraction was made a black box would appear, for a correct double pulse a grey box appeared (for image see [Citation1,Citation11]). The participants were verbally instructed which trigger they had to produce.
Functional training: game group and EMG group
During the functional training, the participants wore their socket with the iLimb Ultra hand. A filled 0.5 L water bottle, a single-use plastic cup and a plastic board placed on either a table or box (21 cm) had to be moved. In total, four movements were required: (1) pick up a bottle from the table and move it onto the box using a pinch grip, (2) move the board from the box to the table using a key grip, (3) move the plastic cup from the table onto the box using the pinch grip, and (4) place the board back on the box using the key grip.
These four steps were repeated for 2.5 min. The order of the tasks remained the same during and over sessions. The 2.5 min session was repeated twice; once while sitting and once while standing, which was done to familiarize the participants during the training with different muscle tones and controllability. No data were collected during this part of the training. The goal was to let participants adjust to the weight and movement speed of the prosthetic hand. The pinch grip and key grip had to be used alternately, enforcing production of triggers.
Data handling and outcome measures
To answer each research aim, one or more outcome measures were calculated per aim. For the first aim (feasibility), the SUS scores were calculated as instructed for the scale [Citation18]. The training method was deemed ‘feasible’ if the SUS showed scores of 62 or higher [Citation20].
For the second aim (comparison of myosignal characteristics), all sEMG data collected during the training sessions was processed and analysed using customized Matlab scripts. Nine outcome measures were calculated and categorized as either a clinically relevant performance measure, reflecting behavioural measures of task performance (, three upper rows, see Appendix A for figure) or as a myosignal feature (, six bottom rows, see Appendix A for figure). The myosignal features were either derived from or identical to the parameters previously described [Citation1,Citation11,Citation25] and were calculated per attempted switch (either successful or not). The outcomes of individuals with a ULD were then compared to outcomes of non-impaired individuals: differences in (changes in) performance measures over training, and differences in (changes in) myosignal features over training were examined.
Table 1. Definitions of the outcome measures.
For the third aim (transfer), outcome measures from the pre- and post-tests were compared. If individuals were more successful during the post-test than during the pre-test, we took this to indicate transfer of acquired skill.
Due to the small number of participants, the data were presented in a descriptive way and no statistical tests were performed.
Results
Three males and one female (median age 62, IQR 9) participated. Three of them had an acquired ULD and one a congenital deficiency, all transradial. All had at least 7 years of prosthetic experience (range 7–53 years) ().
Table 2. Description participants.
Feasibility of switching
SUS scores
Two of the participants scored the system as Acceptable (highest category), one as Marginally high, and one as Marginally low (). Three out of the four participants considered the switching method as feasible (SUS scores > 62).
Comparisons with non-impaired individuals
Clinically relevant performance measures
For the co-contractions and the double pulses, the game group made almost no correct triggers, whereas the EMG group produced quite some correct triggers. EMG participant 1 was better at producing double pulses than co-contractions, while this was the other way around for EMG participant 2 ( and see Appendix B for additional figure). For EMG participant, two performance seemed to mainly improve during the last or last two training sessions. When comparing the number of correctly performed co-contractions, it appeared that the game group scored worse than the non-impaired game group, whereas the EMG group scored only slightly lower than the non-impaired EMG group. Time needed to switch and Accuracy of switching can only be calculated for triggers that were correctly performed. The occurrence of the correct triggers was too scarce in most participants to give insight into the improvement over training and therefore we could not compare the changes over training in participants with a ULD to the changes over training in non-impaired individuals.
Table 3. Number of correct triggers per training session.
Myosignal features
The assessment of the myosignal features was not affected by the number of triggers that was performed correctly, since also non-successful attempts could be analysed for these dependent measures. The results of myosignal features regarding CO and DP triggers are reported separately below (see also and , respectively).
Figure 1. Co-contractions: comparison of outcome measures of individuals with an upper limb defect (ULD) and non-impaired individuals (NI). Left: Game group (GG), right: EMG group (EG). In the left column the black dots represent GG participant 1 and the white dots GG participant 2, in the right column the black dots represent EG participant 1, and the white dots EG participant 2. All panels contain the information of a group of 10 non-impaired individuals (grey area), and data points of two participants GG (left panel) or EG (right panel) (black and white dots) over the course of 5 (non-impaired individuals) or 7 (individuals with an ULD) training sessions. Regarding the data of the non-impaired group: the middle line represents the mean, the grey area represents the standard deviation around that mean. For the individuals with a ULD individual data points are shown per training session. From top to bottom, the following outcome measures are shown: attempts, amplitude, phasing, and width.
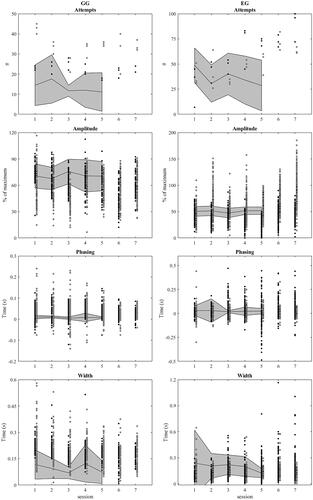
Figure 2. Double pulse: comparison of outcome measures of individuals with an upper limb defect (ULD) and non-impaired individuals (NI). All six panels contain four columns of points. The first two represent Game group-ULD (GG-ULD), the last two represent EMG group-ULD (EG-ULD). The grey area behind these columns represents the information of a group of 10 non-impaired individuals (NI). The lines in the middle of this grey area represent the median of the outcome measure for the NI group data, the area itself represents the standard deviation. Both GG-UD and EG-ULD performed 7 training sessions, the NI performed. Left column: attempts, amplitude difference, Extensor valley. Right column: mean amplitude, width, flexor amplitude.
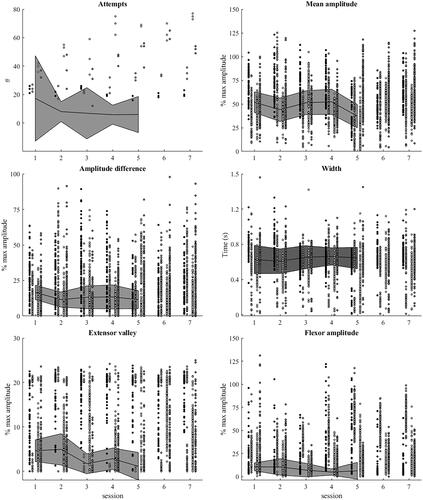
CO-contractions (CO, )
Attempts
For both the game group and the EMG group, the number of attempts was within the upper half or above the values of respectively non-impaired game group and non-impaired EMG group, suggesting that more attempts were made in the group of individuals with a ULD [, top row left (game group) and top row right (EMG group)]. Within the game group, no systematic changes could be seen over training. For the EMG group, the number of attempts increased over the training sessions, whereas for the non-impaired EMG group, the number of attempts seemed to decrease.
Amplitude
The spread of the amplitude data seemed higher for both the game group and the EMG group than for the non-impaired game group and the non-impaired EMG group, respectively (, second row), indicating less consistent contractions. For the game group, the amplitude was slightly lower than for the non-impaired game group. A lower amplitude might indicate poorer performance or more fatigue. For the EMG group, the amplitude appeared lower for EMG participant 1 but partly higher for EMG participant 2 who showed a very large variability. Over time no systematic changes in amplitude could be observed for the game group participants 1 and 2 and EMG participant 1, while for EMG participant 2, amplitudes seemed to increase over training. All four participants occasionally reached amplitudes above the calibrated maximum, but note that for EMG participant 2, this happened quite often.
Phasing
The game group appeared to have a higher variability than the non-impaired game group, whereas over time the variability of the game group seemed to decrease (, third row left). The variability in the EMG group seemed closer to the variability in the non-impaired EMG group, in particular in the first sessions (, third row right). the EMG group appeared to have no systematic changes over time.
Width
The game group data might be interpreted as showing higher values and more variability than the non-impaired game group, whereas for the EMG group, the values and variability were very close to the non-impaired EMG group (, bottom row left). Over time width appeared to decrease for the game group. For the EMG group, the variability seemed to increase over time (, bottom row right). Since a smaller width improves chances of a signal to be recognized as a trigger, a decrease in width indicates better performance.
Double pulses (DP, )
As described in the Method section, the data from the non-impaired groups regarding double pulses come from a separate set of 10 non-impaired participants that trained double pulses production using a prosthetic hand. Since this group did not train with either a game or myosignals displayed on a screen, in , the data from the non-impaired group are presented with both the game group and the EMG group in one plot instead of separately as was done for the co-contraction data.
Attempts
The number of attempts and the variability within that number seemed to be higher for both the game group and the EMG group than for the non-impaired group. Over time most participants from the ULD groups (the game group and the EMG group) appeared to increase the number of attempts, however this increase was smaller for the game group than for the EMG group.
Amplitude
The mean amplitude of both ULD groups appeared to centre around the mean of the non-impaired group, however the individuals with a ULD seemed to show more variability, indicating less consistent contractions. No systematic changes over time could be observed and no differences seemed to be present between the game group and the EMG group.
Amplitude difference
The amplitude difference indicated more variability for both the game group and the EMG group than for the non-impaired group while the mean seemed to be slightly higher for the two ULD groups. This might indicate that impaired participants had more trouble contracting both muscles with similar intensity.
Width
The mean value of width of both the game group and the EMG group seemed similar to the non-impaired group mean. However, the variability appeared higher, indicating less stable behaviour for both the game and EMG groups. In double pulses, a lower width is a sign of better/higher contraction and relaxation speeds. The graphs indicated no systematic changes over time.
Extensor valley
The Extensor valley appeared to be higher and more variable for both the game group and the EMG group than for the non-impaired group. A lower extensor valley indicates better relaxation between the two pulses, leading to better performance. Whereas the Extensor valley seemed to decrease over time for the non-impaired group, this was not the case for both ULD groups.
Flexor amplitude
Flexor amplitude indicated higher values and higher variability for both ULD groups than for the non-impaired group. A lower flexor amplitude is a sign of higher capability of isolating the muscle groups/myosignals, resulting in better performance. The graphs indicated no systematic changes over time.
Transfer of skill to prosthesis use
Between the pre- and post-test no time differences could be observed in the results of the Partial-SHAP (, left), suggesting that no transfer of skill took place.
Figure 3. Pre- and post-test data. In all panels pre-test bars are represented with a dotted edge and post-test bars with a solid edge. Left panel: Times for completing the Partial-Southampton Hand Assessment Procedure. The four tasks are represented by the four different shades of grey, from left to right: heavy sphere, light sphere, jar opening, water pouring. Per task the outcomes are shown for all four participants. In all cases lower values indicate faster, and thus better performance. Middle & right panels: number of correctly relocated clothespins from the adapted clothespin relocation test. The middle panel shows outcomes for co-contraction, the right for double pulses. The colours refer to the clothespins that were moved; darkest = first, middle tone = second, lightest = third.
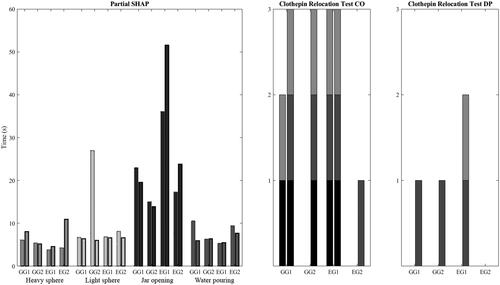
Adapted CRT: co-contraction
During the pre-test, two participants were able to correctly produce co-contraction triggers within the time limit and thus relocate the clothespins (, middle subplot). Three participants seemed to improve from pre- to post-test and thus indicated transfer of skill. EMG group participant 1 appeared to show a ceiling effect: the maximal total number of clothespins (three) were relocated in the pre-test and this performance was reproduced in the post-test.
Adapted CRT: double pulse
During the pre-test, no correct double pulse triggers were produced within the time limit and thus no clothespins were relocated (, right subplot). During the post-test game group, participants 1 and 2 and EMG participant 1 replaced one and two clothespins, respectively. EMG participant 2 was unable to replace the clothespins in both the pre- and the post-test. Game group participants 1 and 2 and EMG participant 1 might show some transfer of learned skills, but EMG participant 2 did not.
Discussion
Both training methods for CCS seemed to be feasible; participants were able to perform the tasks and three out of four participants scored the systems as feasible. However, three of the four individuals with a ULD produced only a few correct triggers during the training, in contrast to the non-impaired group. The group of individuals with a ULD performed more attempts than the non-impaired group, but these attempts did not lead to more successfully produced triggers. In all successful and non-successful attempts, the individuals with a ULD seemed to show more variation than the non-impaired individuals. These differences between ULD participants and non-impaired participants might not only be due to the apparent anatomical differences in (remnant) forearm musculature between both groups, but they might also be due to less usage or relative disuse of flexors and extensors in the ULD participants as a result of the ULD.
The small number of correct triggers produced by the participants with a ULD, suggested that trigger production appeared to be hard. Importantly, the low number of correct triggers was not related to the understanding of the task of the participants. Moreover, there seemed to be an effect of training on actual prosthesis use, but the provided training did not lead to reliable skills that can be used as a means to switch between grips in daily life activities. Too little switch attempts resulted in a (correct) switch, meaning that in daily life for each switching action multiple attempts would have to be made which would be too time- and energy consuming. It is important to note that although most participants in our study saw CCS as feasible, previous research showed that upper limb prosthesis users do not always see trigger production as a useful way to switch between grip types, calling it inconvenient, unintuitive, time-consuming, and unreliable [Citation4,Citation13,Citation26]. The training methods and the training scheme (frequency, intensity, order, duration, feedback) must be improved in order to result in better performance in actual prosthesis use. The current paper examined virtual training but this was only partially effective.
With respect to the training, the results showed that during the training sessions the EMG group performed more correct triggers than the game group, independent of whether the triggers were co-contractions or double pulses. For producing co-contractions, the differences between the impaired and the non-impaired participants seemed smaller for the EMG group than for the game group, in particular for Phasing and Width. For producing double pulses, all four participants with a ULD seemed to be less capable of isolating the myosignals and also seemed to be less capable of actively relaxing the muscles than the non-impaired participants. Non-impaired people possess the ability to isolate and/or synchronize muscle activity (cf. [Citation27,Citation28]). Hence, the lack of this ability in ULD could have affected the training of producing triggers.
An important difference between the types of training followed by the game group and the EMG group is the location of the focus of attention. This focus of attention can either be on the action itself (internal) or on the effect of an action on the environment (external) (see e.g., [Citation29–31]). In the current study the ‘action’ is contracting the muscles and the ‘effect on the environment’ is the change of the grip-type. In the game task, the focus was external, all attention was directed to whether the grabber on the screen changed its form due to a correct trigger. In the EMG task, the focus of attention was internal, the attention was focussed on the contractions of the muscles. Overall it is reported in the literature that an external focus of attention is more beneficial in training, which may explain the better transfer effects in the game group (cf. [Citation29]). However, the EMG group had more successful attempts than the game group. To understand this effect, note that prosthesis users refer to the production of triggers as non-intuitive and mentally exhausting, indicating that switching, most likely, requires conscious control [Citation13]. Moreover, in the literature on focus of attention, it is hypothesized that an internal focus of attention requires involvement of a performer’s working memory that is related to consciousness processes involved in motor control [Citation32]. Hence, training the EMG task might address these conscious processes, which might explain the higher number of successful attempts in this group.
We suggest that a more optimal training might consist of first a part of the task performed by the EMG group in which the focus of attention is more internal. This part should be followed by a training part with a game where the external focus might increase the degree of transfer to actual prosthesis use. This idea is in accordance with Peh et al. [Citation31] who suggest that, even though an external focus of attention is generally more effective in training, more research is needed to examine whether learners of a novice task alternate between internal and external foci of attention while learning.
Transfer of learned skill from training to prosthesis use was suggested in three of the four participants. Interestingly, transfer seemed to be slightly higher for the game group than for the EMG group. The game training was designed to show proof of principle that gaming could be used to improve trigger production in prosthesis control. An issue with game training is task-specificity, of which the importance has been shown for proportional control [Citation9,Citation10]. However, regarding the training of trigger production, we previously did not find a clear effect of task-specificity [Citation1]. As long as triggers were trained, participants improved, regardless of the task or the environment. In proportionally controlled activities such as picking up an object, feedback can be given on a plethora of things such as hand aperture, movement/opening/closing speeds etc. Instead, trigger production is a very abstract and short task and the required action does not consist of multiple components, does not vary between individuals and can only be performed in one way in order to be recognised as correct. Based on our findings, we conclude that for transfer of training of trigger production task-specificity seems less important than type of feedback, compared to proportional control.
Limitations
We included four individuals with an ULD, which is a small sample size. Our study should therefore be regarded as an explorative study. Only participants who were completely naïve for CCS, but who owned a socket with a detachable hand were eligible, which greatly reduced the eligible population. In addition, for a number of mainly elderly people, the elaborate training and testing schedule of nine sessions was seen as quite daunting. Due to the small sample size, conclusions can only be drawn with caution and generalizing to the entire population of those with an upper limb defect should be done with caution. However, the findings of this exploratory study might help in designing future studies regarding how to train switching in prosthesis users. Besides having a small sample size our individuals with a ULD were older than the participants in the non-impaired group. Both cognitive functioning and fine motor function might deteriorate as age increases, which might have had an effect on performance in this study. For future research, we would recommend including aged matched controls.
Due to the set-up of the training, the game group spent less time training actual trigger production than the EMG group. Part of the game training consisted of proportional training whereas that was not the case for EMG task. The game group might have performed better if training times would have been equal to those of the EMG group. Furthermore, the number of training sessions differed between the individuals with a ULD and the non-impaired individuals. Even though the non-impaired group had less training sessions, they showed less variability than both ULD groups. Equalizing the number of training sessions by increasing the number of sessions in the non-impaired group would most likely decrease variability further. As a consequence, the difference between the non-impaired group and the ULD groups would become even more substantial.
The thresholds for the rise and fall time of the amplitude for co-contractions in our developed software were based on values provided by Touch Bionics by Össür. During data analysis of our previous study [Citation1], we found that these values were stricter than the values implemented in the prosthesis hand. In the new version of the software, that we did use in this study, these thresholds could be adjusted per individual. However, we used the thresholds as set in the standard factory settings to keep our protocol identical among participants. The transfer effect in this study might have been greater if the algorithm for trigger detection had been similar for training and actual prosthesis use.
Conclusions
The current study suggested that training trigger production in individuals with a ULD using either a serious game or by showing myosignals on a screen seems to be feasible. The main differences in performance measures and myosignals features between the individuals with a ULD and non-impaired participants were found in the number of correct triggers and in features related to the ability to isolate muscle contractions. Where the EMG group showed myosignal features closer to the non-impaired participants, in the post-test outcomes the game group slightly outperformed the EMG group. Three out of the four participants seemed to show transfer of skill from training to prosthesis use.
Ethics approval and consent to participate
The study was conducted in agreement with the Declaration of Helsinki and approved by the medical ethics committee of the University Medical Center Groningen (METc 2018.268).
Consent for publication
Written informed consent for collection and publication of the data was obtained from all participants prior to the start of the experiment.
Supplemental Material
Download MS Word (359.9 KB)Acknowledgements
The authors gratefully acknowledge Hylke van Dijk (NHL, Leeuwarden, The Netherlands) for his help with developing the serious game and Wim Kaan for developing the LabView switch-detection software and for his technical assistance.
Disclosure statement
The authors declare that they have no competing interests.
Availability of data and materials
The datasets used and/or analysed during the current study are available from the corresponding author on reasonable request.
Additional information
Funding
References
- Heerschop A, van der Sluis CK, Bongers RM. Transfer of mode switching performance: from training to upper-limb prosthesis use. J Neuroeng Rehab. 2021;18(1):85.
- Belter JT, Segil JL, Dollar AM, et al. Mechanical design and performance specifications of anthropomorphic prosthetic hands: a review. J Rehabil Res Dev. 2013;50(5):599–618.
- Atzori M, Müller H. Control capabilities of myoelectric robotic prostheses by hand amputees: a scientific research and market overview. Front Syst Neurosci. 2015;9:162. Available from: http://www.ncbi.nlm.nih.gov/pmc/articles/PMC4663252/.
- Kuiken TA, Miller LA, Turner K, et al. A comparison of pattern recognition control and direct control of a multiple degree-of-Freedom transradial prosthesis. IEEE J. Transl. Eng. Health Med. 2016;4:1–8.
- Chen X, Zheng Y-P, Guo J-Y, et al. Sonomyography (SMG) control for powered prosthetic hand: a study with normal subjects. Ultrasound Med Biol. 2010;36(7):1076–1088.
- Resnik L, Klinger SL, Etter K, et al. Controlling a multi-degree of freedom upper limb prosthesis using foot controls: user experience. Disabil Rehabil Assist Technol. 2014;9(4):318–329.
- Farina D, Jiang N, Rehbaum H, et al. The extraction of neural information from the surface EMG for the control of upper-limb prostheses: emerging avenues and challenges. IEEE Trans Neural Syst Rehabil Eng. 2014;22(4):797–809.
- Vilarino M, Moon J, Pool KR, et al. Outcomes and perception of a conventional and alternative myoelectric control strategy: a study of experienced and new multiarticulating hand users. J. Prosthetics Orthotics. 2015;27:10.
- van Dijk L, van Sluis CK, van der Dijk HW, et al. Learning an EMG controlled game: task-specific adaptations and transfer. PLoS One. 2016;11:e0160817.
- van Dijk L, van Sluis CK, van der Dijk HW, et al. Task-oriented gaming for transfer to prosthesis use. IEEE Trans Neural Syst Rehabil Eng. 2016;24:1384–1394.
- Heerschop A, van der Sluis CK, Otten E, et al. Looking beyond proportional control: the relevance of mode switching in learning to operate multi-articulating myoelectric upper-limb prostheses. Biomed Signal Process Control. 2020;55:101647.
- Østlie K, Lesjø IM, Franklin RJ, et al. Prosthesis use in adult acquired major upper-limb amputees: patterns of wear, prosthetic skills and the actual use of prostheses in activities of daily life. Disabil Rehabil Assist Technol. 2012;7(6):479–493.
- Franzke AW, Kristoffersen MB, Bongers RM, et al. Users’ and therapists’ perceptions of myoelectric multi-function upper limb prostheses with conventional and pattern recognition control. PLoS One. 2019;14(8):e0220899.
- Kristoffersen MB, Franzke AW, Bongers RM, et al. User training for machine learning controlled upper limb prostheses: a serious game approach. J Neuroeng Rehab. 2021;18(1):32.
- Bouwsema H, van der Sluis CK, Bongers RM. Changes in performance over time while learning to use a myoelectric prosthesis. J NeuroEngineering Rehabil. 2014;11(1):16.
- Bouwsema H, van der Sluis CK, Bongers RM. Learning to control opening and closing a myoelectric hand. Arch Phys Med Rehabil. 2010;91(9):1442–1446.
- Romkema S, Bongers RM, van der Sluis CK. Intermanual transfer in training with an Upper-Limb myoelectric prosthesis simulator: a mechanistic, randomized, pretest–posttest study. Phys Ther. 2013;93(1):22–31.
- Brooke J. SUS: a quick and dirty usability scale. In Usability Evaluation in Industry. London: CRC Press; 1995, p. 189.
- Rozevink SG, van der Sluis CK, Garzo A, et al. HoMEcare aRm rehabiLItatioN (MERLIN): telerehabilitation using an unactuated device based on serious games improves the upper limb function in chronic stroke. J Neuroeng Rehab. 2021;18(1):48.
- Bangor A. Determining what individual SUS scores mean: adding an adjective rating scale. J. Usability Stud. 2009;4:10.
- Hussaini A, Kyberd P. Refined clothespin relocation test and assessment of motion. Prosthet Orthot Int. 2017;41(3):294–302.
- Vasluian E, Bongers RM, Reinders-Messelink HA, et al. Preliminary study of the Southampton hand assessment procedure for children and its reliability. BMC Musculoskelet Disord. 2014;15:199.
- Light CM, Chappell PH, Kyberd PJ. Establishing a standardized clinical assessment tool of pathologic and prosthetic hand function: normative data, reliability, and validity. Arch Phys Med Rehabil. 2002;83(6):776–783.
- Resnik L, Borgia M, Cancio JM, et al. Psychometric evaluation of the Southampton hand assessment procedure (SHAP) in a sample of upper limb prosthesis users. J. Hand Therapy. 2021; [cited 2021 Sep 25]; Available from: https://www.sciencedirect.com/science/article/pii/S0894113021001113.
- Tabor A, Bateman S, Scheme E. Evaluation of myoelectric control learning using multi-session game-based training. IEEE Trans Neural Syst Rehabil Eng. 2018;26(9):1680–1689.
- Hargrove L, Miller L, Turner KL, et al. Myoelectric pattern recognition outperforms direct control for transhumeral amputees with targeted muscle reinnervation: a randomized clinical trial. Sci Rep. 2017;7(1):1–9.
- Radhakrishnan SM, Baker SN, Jackson A. Learning a novel myoelectric-controlled interface task. J Neurophysiol. 2008;100(4):2397–2408.
- Ison M, Artemiadis P. Enhancing practical multifunctional myoelectric applications through implicit motor control training systems. 2014 36th Annual International Conference of the IEEE Engineering in Medicine and Biology Society. Chicago, IL: IEEE; 2014. [cited 2020 Aug 17]. p. 3525–3528. Available from: http://ieeexplore.ieee.org/document/6944383/.
- Wulf G, Prinz W. Directing attention to movement effects enhances learning: a review. Psychon Bull Rev. 2001;8(4):648–660.
- Totsika V, Wulf G. The influence of external and internal foci of attention on transfer to novel situations and skills. Res Q Exerc Sport. 2003;74(2):220–225.
- Peh SY-C, Chow JY, Davids K. Focus of attention and its impact on movement behaviour. J Sci Med Sport. 2011;14(1):70–78.
- Poolton JM, Maxwell JP, Masters RSW, et al. Benefits of an external focus of attention: common coding or conscious processing? J Sports Sci. 2006;24(1):89–99.