Abstract
This article investigates the influence of socio-psychological factors on farmers’ intention to perform result-based agri-environmental schemes for the improved provision of biodiversity and soil quality. We present a Structural Equation Model, which uses an extended Technology Acceptance Model as a theoretical basis for better understanding farmers’ decision-making. Results from a survey of 235 Austrian farmers show that behavioural factors such as self-efficacy, perceived risk, and subjective norm directly or indirectly impact farmers’ intention to perform result-based schemes. Findings stress the importance of farmers’ surrounding networks for the successful implementation of such schemes and the need for including mechanisms into their design, which are suited to diversify risk and increase self-efficacy. In conclusion, our results emphasize that considering the influence of socio-psychological factors on farmers’ decision-making may offer new levers in the design of more environmentally effective and efficient agri-environmental-climate measures.
1. Introduction
To counteract global biodiversity and climate crisis, efforts to promote the provision of Agri-Environmental and Climate Public Goods (AECPG) and to reduce the negative impacts of agriculture on these goods need to be strengthened. Within the European Union, a central tool for steering farmers’ decision-making towards the adoption of more environmentally and climate-friendly management are the Agri-Environmental and Climate Measures (AECMs). Implemented under the framework of the Common Agricultural Policy (CAP) (European Commission Citation2018; European Court of Auditors Citation2011), AECMs are monetary incentives, based on voluntary participation. Currently designed to be predominantly action-oriented, AECMs tie farmers’ financial compensation to the implementation of certain environmentally friendly management measures on their farms/fields (European Court of Auditors Citation2011). Extensive financial resources are spent to finance AECMs (Science for Environment Policy Citation2017), and if conceptualised and realised well, AECMs represent an effective tool to foster environmentally beneficial farming (Batáry et al. Citation2015; Walker et al. Citation2018).
To drive forward the sustainable transformation of European farming, the European Green Deal and its Farm-to-Fork strategy also aim to secure and strengthen the role of AECMs in the future (e.g. Pe’er et al. Citation2020; Scown, Brady, and Nicholas Citation2020). However, the cost and environmental effectiveness of the classical, action-oriented AECMs is increasingly criticized (e.g. Cullen et al. Citation2021; European Court of Auditors Citation2011; Pe’er et al. Citation2019, Citation2014; Science for Environment Policy Citation2017). Poor spatial and content-related targeting, as well as shallow environmental objectives, have hampered the achievement, and especially the measurement, of real environmental improvements in the past (European Court of Auditors Citation2011). Also, a long-term transition to more sustainable management practices among farmers requires a fundamental behavioural change, supported by the building of social and cultural capital, a component mostly lacking in current AECMs (Burton and Schwarz Citation2013; Burton and Paragahawewa Citation2011).
Against this background, more effective solutions for improving the provision of AECPG are needed: As such, novel Agri-Environmental and Climate Schemes (AECS), implementing result-based (RB) payment approaches, are promoted as promising instruments to increase the delivery of AECPG (de Snoo et al. Citation2013; Burton and Schwarz Citation2013). In RB schemes, payments to farmers are based on the achievement of measurable environmental results rather than on the implementation of prescribed management measures (Burton and Schwarz Citation2013; Gerowitt, Isselstein, and Marggraf Citation2003). In recent years, interest in RB payment schemes has grown rapidly among researchers and policymakers. The European Commission particularly promotes such schemes among the 2030 Commitments in the EU Biodiversity Strategy (European Commission Citation2020). Within the framework of the CAP, pilot RB payment schemes have been transformed into full AECS. In Austria, for example, an RB pilot scheme for biodiversity conservation was transferred into a full scheme within the Austrian Agri-environmental Programme for the period 2023–2027 (AMA Citation2023). In Germany, a result-based scheme for flowering species in grassland has been programmed as a First Pillar eco-scheme (Runge et al. Citation2022). Also, private initiatives implementing RB schemes are starting to develop, most of them with a strong focus on improving biodiversity, but also soil quality and carbon sequestration (Eichhorn et al. Citation2022). The rising popularity of RB schemes is due to recognized advances in their effectiveness over action-based measures (Wuepper and Huber Citation2022): By linking the payment to the environmental outcome, RB schemes enable the direct control of their environmental impact. The direct remuneration of results enhances conditionality and, moreover, encourages farmers to step into the schemes with land producing the best environmental outcome (Matzdorf, Kaiser, and Rohner Citation2008). Therefore, RB schemes can clearly lower the risk of ‘adverse selection’. In addition, the freedom of making own management decisions with the aim of achieving results (Herzon et al. Citation2018) can increase farmers’ innovation capacity (Allen et al. Citation2014; Matzdorf and Lorenz Citation2010). The freedom of management decisions can also enhance ecological performance, as it allows farmers to adapt their management to local conditions and climatic variations (Sabatier, Doyen, and Tichit Citation2012), as well as to their experience and local knowledge (Burton and Schwarz Citation2013; Gibbons et al. Citation2011). On the other hand, RB schemes are associated with some disadvantages and challenges: the definition of effective and legitimate indicators and their thresholds, for the verification of environmental improvements, is highly demanding. This is particularly true as indicators have to provide a balance between measurability, accuracy and reliability in relation to the targeted AECPGs (Burton and Schwarz Citation2013; Birge et al. Citation2017; Allen et al. Citation2014). Moreover, the perceived higher economic risk of external factors (e.g. weather conditions, natural processes, neighbouring farmers, etc.) limiting environmental outcomes and therefore the issuing of payments (Burton and Schwarz Citation2013; Wezel et al. Citation2018; Derissen and Quaas Citation2013; Ayambire and Pittman Citation2021), can represent an obstacle to farmers’ participation. Also, scepticism towards new approaches (Stolze et al. Citation2015) and difficulties in understanding the implications of RB schemes in practice (Wezel et al. Citation2018), pose potential barriers to farmers’ acceptance.
The question of farmers’ acceptance is directly related to the question of farmers’ decision-making. Understanding, modelling, and predicting farmers’ behaviour in adopting environmentally friendly management, policies, and innovations, represents an important research topic within the field of agricultural economics (Willock et al. Citation1999). However, purely economic models, based on Expected Utility Theory, treating farmers’ decision-making as a predictable response to economic stimuli, have proven insufficient to fully explain farmers’ behaviour (Brown et al. Citation2017, Citation2021; Edwards-Jones Citation2006; Dessart, Barreiro-Hurlé, and Van Bavel Citation2019). The complexity of farmers’ decisions is neglected in these models by the basic assumption that farmers’ decisions are based on normative theory and only driven by individuals’ actions to maximize profits (Austin et al. Citation1998; Willock et al. Citation1999). In contrast, literature suggests that understanding the cognitions that underlie farmers’ behaviour (Kaplan and Kaplan Citation2008), as well as considering social and cultural factors (Edwards-Jones Citation2006) can contribute to the development of effective new AECMs. Reacting to these shortcomings and pursuing a more interdisciplinary approach, psychosocial models have been applied in behavioural economics to improve the explanatory power of economic models (Camerer, Loewenstein, and Rabin Citation2004). Also in agricultural economics, theoretical psychological frameworks have been increasingly incorporated to better understand farmers’ decision-making (Borges et al. Citation2014; Adnan, Nordin, and bin Abu Bakar Citation2017; Daxini et al. Citation2019). Particularly for decision-making with respect to the acceptance of novel policy schemes, Dessart, Barreiro-Hurlé, and Van Bavel (Citation2019) have pointed out that the analysis of behavioural factors such as risk aversion, perceived cost, and perceived control is crucial for understanding how farmers will respond to new approaches.
Against these backgrounds, the research motivation of our study is to better understand farmers’ acceptance and decision-making as regards the adoption of RB schemes for an improved delivery of AECPG. Also, we aim to derive how farmers can best be approached and recruited for such schemes. We develop and apply a Structural Equation Model (SEM), aimed at investigating the influence of socio-psychological factors on farmers’ intention to perform RB schemes for the enhanced provision of either biodiversity or soil conservation. SEM is a statistical data analysis technique that enables the analysis of complex social phenomena, including their latent characteristics, relationships, and multiple variables (Tarka Citation2018). As our theoretical model, we adapt the Technology Acceptance Model (TAM) developed by Davis (Citation1986, Citation1989) to the context of the intention to perform novel AECS. The main contribution of our study is to (1) apply the TAM-framework, originally developed for analysing the acceptance of new technologies, in an agri-political context, (2) provide an understanding of the causal relationships between the basic TAM constructs and an extended version of the TAM in this specific context and (3), generate fresh insights into the factors influencing farmers’ intention to perform RB schemes and thus provide policy recommendations for better scheme design. The study is based on a farmer survey conducted in Austria in 2021.
2. Theoretical foundation and hypotheses
2.1. Technology Acceptance Model – basic model
The Technology Acceptance Model (TAM) developed by Davis in 1989 (Davis Citation1989; Davis, Bagozzi, and Warshaw Citation1989) is one of the best-known models for explaining the acceptance of systems and technologies, encompassing novelties or innovations. Adapted from the Theory of Reasoned Actions (TRA) (Fishbein and Ajzen Citation1975; Ajzen and Fishbein Citation1980) and the Theory of Planned Behaviour (TPB) (Ajzen Citation1988, Citation1991), the TAM was originally designed to explain factors determining users’ behaviour towards new information technologies (Davis, Citation1989). Davis (Citation1986, 7), describes the TAM as “a theoretical basis for a practical ‘user acceptance testing’-methodology that would enable system designers and implementers to evaluate proposed new systems prior to their implementation.” Today, the TAM is recognized as an empirically validated, effective and parsimonious model for capturing and explaining different behaviours (Venkatesh and Davis Citation2000; King and He Citation2006), also beyond the technology sector. It has been successfully applied in the agricultural context (e.g. Adnan, Nordin, and bin Abu Bakar Citation2017; Naspetti et al. Citation2017; Rezaei, Safa, and Ganjkhanloo Citation2020; Schaak and Mußhoff Citation2018; Schulze and Spiller Citation2010), as well as in the policy context (Pierce et al. Citation2014).
The TAM assumes that the intention to use a new methodology, and in a subsequent step the actual use, is determined by the attitude towards use (Davis Citation1986; Davis, Bagozzi, and Warshaw Citation1989). Attitude towards use is described as a person’s negative or positive feelings about undertaking a particular behaviour (Fishbein and Ajzen Citation1975). Corresponding to Fishbein and Ajzen’s (Citation1975) attitude theory, attitude towards use is considered a mediator, directly influencing behavioural intention or actual behaviour (Sheppard, Hartwick, and Warshaw Citation1988). Furthermore, attitude towards use is influenced by individual beliefs regarding perceived ease of use and perceived usefulness (Ducey and Coovert Citation2016; Verma, Bhattacharyya, and Kumar Citation2018). Perceived usefulness is defined as “the degree to which an individual believes that using a particular system would enhance his or her [job] performance” (Davis Citation1986, 26). In contrast, perceived ease of use is defined as “the degree to which an individual believes that using a particular system would be free of physical and mental effort” (Davis Citation1986, 26): The more useful a new system is and the easier it is to use, the more likely the user is to use it (Davis Citation1989). Moreover, the two concepts of perceived usefulness and perceived ease of use are interrelated: the process of building opinion on perceived usefulness depends on the perceived ease of use (Venkatesh and Davis Citation2000), i.e. perceived ease of use directly impacts perceived usefulness (Davis Citation1993).
Based on the basic TAM, we hypothesize that a farmer will form a positive attitude towards a novel RB scheme if she/he believes that the implementation is easy, technically simple (perceived ease of use) (H3), and that the implementation of the scheme is useful (H2). Thus, the more a farmer sees the implementation of an RB scheme as positive, the more she/he is likely to implement it (H1). Moreover, the easier the farmer believes the RB scheme is to implement, the greater the usefulness (H4).
These considerations lead to the derivation of our basic TAM model in the context of novel AECS, resulting in the following hypotheses:
H1: Attitude towards use has a significant positive effect on the intention to perform RB schemes.
H2: Perceived usefulness has a significant positive effect on the attitude towards use of RB schemes.
H3: Perceived ease of use has a significant positive effect on the attitude towards use of RB schemes.
H4: Perceived ease of use has a significant positive effect on perceived usefulness.
2.2. Extended TAM
To explain the complex process of creating acceptance, literature suggests that the basic TAM is insufficient. Indeed, several studies have recommended extending the TAM by adding additional constructs, thereby strengthening the model and improving its explanatory power (e.g. Amberg, Hirschmeier, and Wehrmann Citation2004). In a meta-analysis of 88 independent TAM studies, King and He (Citation2006) found several possible model modifications, such as upstream or external factors as determinants of perceived ease of use and perceived usefulness (Venkatesh Citation2000; Venkatesh and Davis Citation2000). Venkatesh and Davis (Citation2000) responded to the criticism of the basic TAM in their well-known work by including, e.g. subjective norm as a social process variable, and Venkatesh (Citation2000) added self-efficacy as a determinant of perceived ease of use. Based on these suggestions, we extended our model by adding subjective norm and self-efficacy as upstream/external factors and self-efficacy as a direct determinant of the intention to perform. Furthermore, we included perceived risk in the extended TAM model, as risk is a topic frequently discussed in the context of RB schemes (Uthes and Matzdorf Citation2013; Burton and Schwarz Citation2013; Dessart, Barreiro-Hurlé, and Van Bavel Citation2019; Wezel et al. Citation2018). The extensions of the TAM model are examined in the following sections regarding their suitability for our research aim.
2.2.1. Subjective norm
Farmers’ intention to perform sustainable practices can be influenced by perceived social pressure to perform this particular behaviour, called subjective norm within the TPB (Ajzen Citation1991). Subjective norm refers to a person’s perception of how important others evaluate the behaviour in question and the motivation to act in accordance with this judgment (Ajzen Citation1985). Venkatesh and Davis (Citation2000) added subjective norm as an external stimulus to perceived usefulness as one of the first TAM extensions (known as TAM2). Also, TAM studies related to sustainable agriculture expanded their models with subjective norm (or social influence) (Rezaei, Safa, and Ganjkhanloo Citation2020; Naspetti et al. Citation2017). This expansion is well reasoned. Studies showed that farmers’ decision-making processes are receptive to influences from neighbours (Cullen et al. Citation2020), the farming community, fellow farmers (Blasch et al. Citation2022), family, other trusted sources of information (Rose, Keating, and Morris Citation2018), as well as society (Defrancesco et al. Citation2008), while these influences are often underestimated. As regards the importance of subjective norm in the context of RB approaches, Birge et al. (Citation2017) corroborate a strong influence of peers. According to their results, farmers assume that farming colleagues and society have a positive view on RB approaches, while at the same time they indicate that they take their colleagues’ opinions into account when making decisions (Birge et al. Citation2017). Consequently, we formulated the hypothesis that subjective norm positively influences the perceived usefulness of RB schemes.
H5: Subjective norm has a significant positive effect on the perceived usefulness of RB schemes.
2.2.2. Self-efficacy
Self-efficacy is defined as “people’s beliefs about their capabilities to produce effects” or, in other words, to successfully perform a particular task (Bandura Citation1994, 2). Bandura (Citation1994) argues that people with high self-confidence in their abilities perceive difficult tasks rather as challenges to be conquered, than threats to be circumvented or avoided. Already in the work by Venkatesh (Citation2000), self-efficacy has been used as a predictor of perceived ease of use. Also a more recent meta-analysis of 107 TAM studies highlighted self-efficacy to be a powerful and often used determinant of perceived ease of use (Abdullah and Ward Citation2016). Within the context of farmers’ behaviour, the influence of self-efficacy on perceived ease of use was confirmed by a study by Rezaei et al. (Citation2020) on farmers’ decision-making as regards more sustainable pest management. Beyond the influence of self-efficacy on perceived ease of use, a study by Sharifzadeh et al. (Citation2017) additionally confirmed the direct influence of self-efficacy on intention to perform. Defrancesco et al. (Citation2008), moreover, showed that farmers are more likely to participate in AECMs if they believe they can easily adopt the practices. For the specific case of RB schemes, and in comparison to action-based approaches, farmers’ knowledge and competences are even more relevant (Mack, Ritzel, and Jan Citation2020). Massfeller et al. (Citation2022) revealed that a perceived lack of control (i.e. self-efficacy) was the main obstacle to RB scheme adoption. The question of self-efficacy in RB schemes is of relevance, as farmers normally lack experience in performing such schemes due to their still mostly experimental nature. We, therefore, hypothesize that farmers who have a strong belief in their capability to implement RB schemes will have a more positive perception towards the ease of use of these schemes and be more willing to implement them.
This leads us to hypotheses H6 and H7:
H6: Self-efficacy has a significant positive effect on the intention to perform RB schemes.
H7: Self-efficacy has a significant positive effect on the perceived ease of use of RB schemes.
2.2.3. Perceived risk
Perceived risk or uncertainty affects people’s confidence in their decisions (Im, Kim, and Han Citation2008). Risky situations can be those in which the probabilities of the occurrence of a particular outcome are unknown (Im, Kim, and Han Citation2008). Producers’ perceived risk has already been identified as a barrier to the adoption of sustainable practices (Trujillo-Barrera, Pennings, and Hofenk Citation2016). In addition, risk aversion represents an important determinant of participation in agri-environmental programs (Wąs et al. Citation2021). In the case of RB schemes, risk is a topic frequently discussed (Uthes and Matzdorf Citation2013; Burton and Schwarz Citation2013; Dessart, Barreiro-Hurlé, and Van Bavel Citation2019; Wezel et al. Citation2018; Zabel and Roe Citation2009): The shift of management decisions from public entities to the farmers entails both a higher responsibility and greater entrepreneurial risk. Entrepreneurial risk is even higher if farmers lack the specific knowledge or skills needed to achieve the environmental goals (Uthes and Matzdorf Citation2013; Wezel et al. Citation2018). Also, external factors such as weather conditions, natural processes, neighbouring farmers, etc., can influence environmental outcomes and, thereby, increase the risk of not achieving the set objectives (Schwarz et al. Citation2008; Wezel et al. Citation2018; Ayambire and Pittman Citation2021). Beyond that, participation in RB schemes per se can result in risks for farmers, such as the appearance of unwanted plants/species, or the loss of nutritional value of products/plants (Wezel et al. Citation2018). A study addressing RB schemes in arable farming confirmed that perceived risk among farmers can be an obstacle to farmers’ willingness to adopt such schemes (Massfeller et al. Citation2022). To counteract these risks, farmers need to undertake a considerable effort in planning and management and, if necessary, adjust both continuously, in order to control and influence the environmental outcome. Against these backgrounds we therefore, hypothesize that a high perceived risk of implementing an RB scheme, will have a negative impact on the perceived ease of use and thus indirectly on the intention to perform RB schemes.
Based on the literature, we propose the following hypothesis (H8):
H8: Perceived risk has a significant negative effect on the perceived ease of use of RB schemes.
3. Materials and method
3.1. Theoretical research framework
Based on the literature review presented above, we developed our theoretical model () for determining the factors influencing farmers’ intention to perform RB schemes. In our model, seven latent constructs are included, namely “Intention to perform a result-based scheme” (ITP), “Attitude towards use” (ATT), “Perceived ease of use” (PEOU), “Perceived usefulness” (PU), “Self-efficacy” (SE), “Subjective norm” (SN) and “Perceived risk” (PR). The basic TAM hypotheses are that ATT is influenced by PU (H2) and PEOU (H3), that PEOU impacts on PU (H4) and that ATT influences ITP (H1). In addition, the extended TAM suggests that SN impacts on PU (H5), and SE influences ITP (H6) and PEOU (H7). Finally, PR influences PEOU (H8). The latent variables within the TAM framework are hypothetical constructs, meaning they cannot be observed or measured directly. To statistically test the relationships within our TAM framework, we, therefore, use structural equation modelling (SEM).
3.2. SEM implementation
SEM is a set of statistical methods for analysing relationships between measured and latent variables, as well as between the latent variables themselves, to test a system of theoretical hypotheses with empirical data (Bagozzi Citation1980; Bollen Citation1989; Jöreskog and Sörbom Citation1979). SEM consists of a measurement model and a structural model. The measurement model measures the latent variables while the structural model tests hypothesized relationships based on path analysis (Kline Citation2016). As latent variables cannot be observed or measured directly, sets of indicator variables are needed (Homburg, Pflesser, and Klarmann Citation2008). These variables are illustrated in a system of linear equations and are estimated with model parameters in such a way that the empirical data are reproduced as well as possible (Weiber and Mühlhaus Citation2014).
We opted for the SEM method for three reasons: First, SEM allows for a complex, multidimensional, and more precise analysis of empirical data than other approaches and statistical models, taking into account different aspects of the studied reality, as well as theoretical constructs (Tarka Citation2018). SEM thus enables the analysis of the theoretical constructs (latent variables) within our theoretical TAM model. Second, SEM, together with the linear mixed model/hierarchical linear model, is one of the most widely recognized statistical solutions for latent variable models in social science and can be used for explaining economic phenomena (Tarka Citation2018). Third, SEM is a preferred method for testing the TAM in general (see the works of Davis Citation1986; Venkatesh and Davis, Citation2000) and more recently also in the field of agricultural management decision-making (Naspetti et al. Citation2017; Rezaei, Safa, and Ganjkhanloo Citation2020).
Our SEM was estimated using maximum likelihood (ML) estimation. Rather than a variance analytical approach, we applied a covariance analytical approach, which provides advantages such as simultaneous parameter estimation and exclusion of measurement errors. The covariance analytical approach is particularly beneficial when testing established theoretical models such as the TAM. In contrast, variance analytical approaches (e.g. Partial Least Squares – PLS) are better suited for exploratory endeavours and the development of new models.
3.3. Questionnaire and data
Data used were gathered from a sample of Austrian farmers through an online survey conducted in Summer 2021. Although two small-scale RB schemes existed in Austria at the time of the survey (Eichhorn et al. Citation2020), a low level of familiarity among farmers was assumed (Wezel et al. Citation2018). To increase farmers’ understanding, we developed a hypothetical RB scheme for the improvement of concrete environmental public goods. For answering the survey, participants could choose between two RB schemes, which were basically identical as regards scheme design, but differed as regards the public goods targeted. One hypothetical RB scheme aimed at improving the public good of biodiversity, the other aimed at improving the public good of soil quality. We designed the RB scheme as a strongly result-based “in-production scheme,” without any additional management requirements (Burton and Schwarz Citation2013) and with progressive payments (higher environmental improvement leads to higher payment). A detailed description of the hypothetical RB schemes is presented in Appendix A1 (online supplementary material). To increase understandability, a short video (102 s) explaining the scheme accompanied the questionnaire (see Appendix A2 [online supplementary material]). A follow-up question asked whether participants perceived difficulty in understanding.
As described in section 3.1, we used the TAM as a theoretical framework to analyse farmers’ behaviour towards novel AECS. However, originally the TAM was developed to understand factors that determine users’ behaviour toward new information technologies (Davis, Citation1989). Therefore, in order to still guarantee reliable measurement, for the latent variables, we used measurement scales whose validity has already been confirmed in studies applying the TAM in agricultural contexts (e.g. Daxini et al. Citation2019; Deng et al. Citation2016; Naspetti et al. Citation2017; Rezaei et al. Citation2020; Sharifzadeh et al. Citation2017) (references in Appendix B [online supplementary material]). In order to better relate to the subject under study, further adjustments were made to the terminology of the measurement items. All items were evaluated on a Likert scale from 1 (strongly disagree) to 5 (strongly agree). The number of items per construct ranged from three to four, as advised by Bollen (Citation1989) as an optimal number of indicators. The construct of “perceived risk” was treated differently due to its exceptional role in RB schemes and was developed and formulated by this study’s authors. Three indicators were introduced describing the construct of perceived risk: financial risk (Matzdorf and Lorenz Citation2010; Trujillo-Barrera, Pennings, and Hofenk Citation2016), the overall risk to achieving the outcome (Wezel et al. Citation2018), and the risk of external factors affecting the outcome (Wezel et al. Citation2018; Burton and Schwarz Citation2013).
In Appendix B (online supplementary material), we give an overview of all seven latent variables including measurement items and summary statistics. To test our model and the measurement items, we conducted two pretests. The first pretest tested for comprehensibility, accuracy, and answerability of the survey and included six scientists and two farmers. The second pretest, carried out by 31 farmers, tested the quality of the measurement models.
3.4. Data analysis
We used the statistical software packages SPSS Statistics 27 and SPSS AMOS 27 to analyse the data. To check the quality of the reflective measurement models, we carried out a reliability and validity test (for pretest and main survey respectively). We applied a covariance analytical approach based on confirmatory factor analysis. Finally, an assessment of the overall model fit was performed.
3.4.1. Reliability and validity testing of the measurement model
Exploratory factor analysis (EFA) was used to confirm the assignment of indicators to constructs and the unidimensional measurement of the indicator sets (Homburg and Giering Citation1998). Kaiser–Meyer–Olkin (KMO) values above 0.6 (from 0.636 to 0.797) (Kaiser and Rice Citation1974), significant Bartlett tests, Measure of Sampling Adequacy (MSA) values above 0.5 (between 0.589 and 0.907), as well as communalities above 0.5 (except PU3 = 0.336Footnote1) approved our indicator allocation and are presented in . Additionally, the pattern matrix extracted only one factor for each construct, indicating a unidimensional measurement (Weiber and Mühlhaus Citation2014). Discriminant validity was tested by checking the individual indicators “group” with the imputed constructs under simultaneous consideration of all constructs. The pattern matrix confirmed our presumed structure (see Appendix C1 [online supplementary material]). Cronbach alpha coefficients higher than 0.70 (Cortina Citation1993) confirmed internal consistency (ranged from 0.798 to 0.919) ().
Table 1. Reliability and validity testing.
Confirmatory Factor Analysis (CFA) with AMOS and Excel (for second-generation reliability criteria) was used to calculate Indicator Reliability/Squared Multiple Correlation (SCM), Factor Reliability (FR)/Composite Reliability (CR), and Average Variance Extracted (AVE). All variables reached the required SCM of 0.4 (Bagozzi and Baumgartner Citation1994) (from 0.5 to 0.903; except PU3 0.365Footnote2) and CR of 0.7 (Hair et al. Citation2010) (from 0.8 and 0.920) (). AVE values higher than 0.5 (from 0.571 to 0.794) indicated convergent validity (Fornell and Larcker Citation1981) (). To verify discriminant validity, an unrestricted model and a restricted model were calculated and compared regarding the chi-square χ2. χ2-difference values above 3.84 indicated discriminant validity (see Appendix C2 [online supplementary material]) (Homburg, Citation1998). AVE values were higher than the square of the corresponding inter-construct correlations, also showing acceptable discriminant validity based on the Fornell/Larcker criterion (Fornell and Larcker Citation1981). Overall, reliability and validity checks showed acceptable to good model quality; therefore, the hypothesis system could be tested in the structural model.
3.4.2. Model fit of the structural model
We estimated the structural equation model with AMOS, using ML estimation. The ML method is the most commonly used and precise estimation algorithm, if a multinormal distribution, as well as a sufficiently large sample size, is givenFootnote3. This requirement applies to our dataset, which is complete with no substantial violation of the normal distribution (univariate), as skewness and kurtosis statistics are below 1 (Temme and Hildebrandt Citation2009) (Appendix C3 [online supplementary material]). To assess the overall fit, various goodness-of-fit criteria were considered (see ). CMIN/DFFootnote4 (2.224; 2.058), RMSEA valueFootnote5 (0.072; 0.067), SRMRFootnote6 (0.0693; 0.0793), CFIFootnote7 (0.964; 0.936), IFIFootnote8 (0.964; 0.936) and TLIFootnote9 (0.954; 0.925) were all in the required range (cut-off values from the literature) for both the basic and extended TAM, thus indicating an acceptable to good model quality.
Table 2. Quality indicators for the assessment of the overall fit of the basic and extended TAM.
4. Results
4.1. Descriptive results
In total, 235 valid responses from farmers were received (for details on farm and farmer characteristics please see Appendix D1 [online supplementary material]). 54.9% of the participants had experience with AECMs and 13.6% with RB schemes. 56.2% of the farmers chose to answer the questionnaire for the AECPG of soil quality and 43.8% for the AECPG of biodiversity. For the majority (66.6%) of the participants, the hypothetical RB scheme was easy or very easy to understand; 23.4% of the participants responded with a neutral score and only 8.5% of the interviewees stated that it was difficult or very difficult to understand.
As regards the general intention to perform, results show that farmers have a slightly positive intention to perform RB schemes, on average (mean construct score = 3.12). Farmers’ attitudes towards the implementation of RB schemes are even more positive, with an average score of 3.59. Also, the perceived ease of use (3.20) and the perceived usefulness (3.27) of RB schemes were assessed slightly positively. The social pressure farmers perceived for performing an RB scheme was moderately high, with a mean of 3.40 between the items used to measure subjective norm. Concerning self-efficacy, the results reveal a slightly moderate positive level in farmers’ beliefs about their capabilities to perform RB schemes: the indicators used to measure self-efficacy reach a mean of 3.22. Finally, the risk farmers perceived for performing an RB scheme was slightly positive, with a mean of 3.10 between the items used to measure perceived risk.
4.2. Hypothesis testing
In the basic TAM, the squared multiple correlation coefficient (R2) of farmers’ ITP for RB schemes was 0.343, indicating that 34.3% of the variable’s variance was explained by the included constructs ().
Table 3. Basic TAM and extended TAM – squared multiple correlations R².
Moreover, the results presented in and indicate that ATT had a positive relationship with farmers’ ITP. Similarly, PU and PEOU had significant positive effects on ATT. PEOU significantly affected PU. Therefore, H1, H2, H3, and H4 were supported in the basic TAM, meaning that farmers form a positive attitude towards an RB scheme if they believe that the implementation is technically simple (H3) and that the implementation of the RB scheme is useful (H2). Thus, the more a farmer sees the implementation of an RB scheme as positive, the higher the intention to perform it (H1). Moreover, the easier the farmer believes the scheme is to perform, the greater she/he perceives its usefulness (H4).
Figure 2. Tested extended TAM structural model with standardized path coefficients and explained variances.
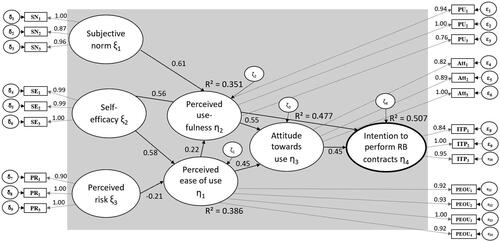
Table 4. Tested structural model – results.
By extending the basic TAM by the constructs SE, SN, and PR, the R2 increased from 0.335 to 0.507, which corresponds to an increase of 17.2% of the variance.
Concerning the hypothesised structural relationships, the results show that H1, H2, H3, and H4 are supported in the extended TAM similar to the basic TAM (). Additionally, the standardized path coefficient between PR with PEOU was significant, indicating a negative, albeit weak, relationship between perceived risk and perceived ease of use. Furthermore, SE had a significant positive impact on PEOU, and on ITP. This implies that farmers with a strong belief in their capability of coping with RB schemes (high self-efficacy) have a more positive perception of their easier use (strongly positive) (H7) and are more willing to perform such schemes (H6). Finally, SN impacts on PU, indicating that subjective norm (perceived social influence through other farmers, farming community, and surrounding network) is positively (medium-strong) influencing the perceived usefulness of RB schemes (H5).
5. Discussion
Our study investigated factors influencing farmers’ intention to perform result-based schemes for the improved provision of biodiversity and soil quality by applying a structural equation model based on an extended Technology Acceptance Model. With an explained variance of 34.3%, our study showed that the application of a TAM was efficient for studying farmers’ intention to perform novel AECS. Comparable figures are also found in the literature, where applications of basic TAMs typically clarify variances of about 40% in users’ behaviour (Venkatesh and Davis Citation2000). Moreover, the extended TAM explained 50.7% of the variance in farmers’ intention to perform RB schemes. This represents a significant improvement over the original TAM, underlining that the extended TAM is more suitable for predicting farmers’ behaviour regarding the intention to perform RB schemes.
5.1. Discussion of the relationships in the basic TAM
Moving into the confirmed hypotheses within the basic TAM, it is notable that our findings align well with existing literature. Meta-analyses of TAM studies consistently underscore the stability of relationships modelled in the basic TAM (e.g. King and He Citation2006; Lee, Kozar, and Larsen Citation2003), also in the context of sustainable agriculture (e.g. Rezaei, Safa, and Ganjkhanloo Citation2020). Furthermore, studies investigating farmers’ willingness to participate in AECS or to adopt environmentally-friendly farming practices have shown that farmers’ attitudes play an important role in understanding their willingness to implement environmental measures (Defrancesco, Gatto, and Mozzato Citation2018; Sattler and Nagel Citation2010). Also, the positive influence of perceived ease of use on farmer attitudes and indirectly on implementation intentions shown by our results, are consistent with the observations of Defrancesco et al. (Citation2008), that the more farmers believe they can easily implement practices, the more likely they are to participate in an environmental program. A previous study has also revealed a relationship between farmers’ decision to participate and the fit between current farm management and AECS requirements, especially in complex AECS (Van Herzele et al. Citation2013).
5.2. Discussion of the relationships in the extended TAM
Turning our attention to the relationships explored within the extended TAM prompts a deeper understanding of their significance. In the extended TAM, the significant influence of subjective norm (perceived social influence through other farmers, farming community, and surrounding network) on the perceived usefulness of RB schemes is in line with the results of several previous studies in other contexts (e.g. Park et al. Citation2014; Venkatesh and Davis Citation2000). This result reinforces the idea that farmers’ connections within their social network, and the impact of peers on their decision to participate in AECS, play a pivotal role in the decision-making process (Siebert, Toogood, and Knierim Citation2006; Rose, Keating, and Morris Citation2018; Cullen et al. Citation2020). This influence also extends to novel RB schemes (Chaplin, Mills, and Chiswell Citation2021; Birge et al. Citation2017), highlighting the enduring importance of social relationships in shaping farmers’ decisions, particularly in the context of emerging AECS.
Also, the proven impact of self-efficacy on perceived usefulness (Abdullah and Ward Citation2016; Rezaei, Safa, and Ganjkhanloo Citation2020; Venkatesh Citation2000) and on intention to perform (Sharifzadeh et al. Citation2017) is in accordance with other studies utilising TAM. In this respect, our findings match the results of studies within the field of sustainable agriculture, revealing that farmers, who believe they have sufficient time and skills to perform a specific action, are also more likely to do so. This is evident, for example, in conversion to organic farming (Läpple and Kelley Citation2013), adoption of soil conservation practices (Wauters et al. Citation2010), or participation in AECMs (Defrancesco et al. Citation2008; Josefsson et al. Citation2017). Massfeller et al. (Citation2022) revealed, moreover, that perceived lack of control – whereby control is defined as the perceived capability to adopt a scheme – was the main obstacle for RB adoption.
Finally, the role of risk, as a general factor influencing participation in AECMs, is proven by the literature (e.g. Wąs et al. Citation2021). For the specific case of RB schemes, the literature suggests that farmers are exposed to even higher risks of achieving the objectives, as environmental improvements might be influenced by external factors (nature, climate, neighbours) (Burton and Schwarz Citation2013). This can result in additional costs for farmers (i.e. extra management and opportunity costs), as they have to meet the environmental targets under more “difficult” conditions (such as droughts) (Ayambire and Pittman Citation2021). Surprisingly, our study found the influence of risk to be rather weak (H8: β = −0.214). With this result we are, however, in line with Matzdorf and Lorenz (Citation2010), as well as Massfeller et al. (Citation2022). Both studies indicate that the issue of risk (perceived risk or financial risk) in the context RB scheme adoption is not as relevant as expected. A possible explanation for this result is the lack of experience among farmers in performing RB schemes, which makes an estimation of their own risk (financial risk, risk of external factors influencing outcome, and risk of not achieving results) difficult. In addition, our RB hypothetical scheme did not include an indication of the amount of payment, limiting farmers’ ability to assess the risk regarding their potential benefit.
5.3. Discussion of the methodology
A methodological limitation of this study is the way the construct of perceived usefulness was developed: One indicator (PU3 “RB schemes can reduce costs by reducing bureaucracy”) performed poorly on the validity and reliability tests, in particular regarding the communality and SCM (see ). PU3, therefore, had to be considered less important in our study, although we retained PU3 as an indicator to avoid a two-indicator construct. In future research, however, we recommend the development and use of another indicator replacing PU3.
In addition, the study involves a methodological particularity by adding the construct of perceived risk which was not based on existing constructs from the literature but was fully developed and formulated by this study’s authors. The construct is based on three indicators, namely financial risk, overall risk of achieving the outcome, and risk of external factors affecting the outcome (indicators are presented in Appendix B [online supplementary material]). Our reliability and validity checks proved an acceptable construct design for our analysis (cf. ). Nevertheless, for the future design, we recommend reformulating or exchanging the indicator of financial risk to capture the variable of perceived risk even better and to further increase construct quality.
Finally, by using SEM as a methodological approach instead of, for example, regression analysis, we were able to incorporate latent variables (such as self-efficacy and perceived risk) into a complex set of relationships and test their direct and indirect influences on intention to perform. In addition, the strength and direction of the relationships were also successfully calculated. This opens the possibility, first, to understand the complexity of farmers’ decision-making processes to implement novel AECMs and, second, to derive more targeted recommendations for their design and implementation, which we will provide in our conclusion.
5.4. Policy implications
The application of the TAM, extended by the behavioural factors of perceived risk, subjective norm, and self-efficacy, and applied in an agricultural policy context, happens – to our knowledge – for the first time and allows us to make more targeted policy recommendations for the design of novel RB schemes under the CAP: as regards the proven role of subjective norm in farmers’ intention to perform RB schemes, we emphasize that novel agri-environmental schemes should also be promoted in farmers’ surrounding networks, as the opinion of e.g. other farmers or the farming community is influencing farmers’ decision-making. A key priority in the introduction phase of novel schemes should therefore be to broaden the communication in the direction of farm advisory services, farm organisations, farmers associations, etc., by e.g. organising and providing in-depth information material and events. Moreover, setting up schemes involving so-called demonstration farms or leading farmers can be beneficial. Through farm visits, vocational exchange, and “word of mouth” through respected farmers and the farming community, farmers might better understand the usefulness of the scheme as well as the means of implementation, which can, on the one hand, increase trust and, on the other activate farmers to participate.
Taking into account, the critical role of self-efficacy in the acceptance and introduction of novel AECS, we suggest implementing actions to increase farmers’ self-efficacy, such as the provision of knowledge support, training, and advice, especially in the introduction phase of the schemes. Giving the farmers a point of reference “how do I perform compared to other farmers,” e.g. via technological support, can further help to improve self-efficacy “I see the measures I take lead in the right direction” and to increase the transparency of the scheme “why do I get less or no funding”. Also, guaranteeing a low entry barrier to RB schemes, e.g. an introduction phase during which farmers can easily opt out if they feel they will not succeed, might mitigate the fear of failure and the related lack of motivation due to lower levels of self-efficacy. Over the lifespan of the scheme, application knowledge, skills, etc. may then increase, and with it, self-efficacy.
As we could show that risk is negatively influencing perceived ease of use, we also suggest the consideration of mechanisms for risk distribution, such as the implementation of hybrid solutions, e.g. by combining action-based basic AECMs with (on-top) result-based elements in one scheme. The inclusion of force majeure clauses in the contract design could address external factors influencing the results, such as extreme weather events or unexpected natural processes. Further potential means for risk reduction might be progressive payment designs or the embedding of thresholds.
As regards the further development of the CAP, and the intended development towards more RB schemes, our results allow the drawing of some further conclusions: The design of “real” RB schemes, which achieve environmental additionality and are not only “action-based schemes in disguise”, place high demands on the scheme designers: According to our results, farmers are willing to perform RB schemes if they are useful to them (profitable) and easy to implement. For scheme designers, this requirement means the need for setting up a simple and competitive scheme, at best with simple measurement indicators easily comprehensible for farmers, but simultaneously guaranteeing that the original aim of the RB schemes is obtained, namely a real improvement in the environmental objective targeted. To achieve this balance, good and efficient collaboration between the institution(s) responsible for policy design, the control authorities, most probably ecologists, and, eventually, agronomists is essential, a precondition which might not be given everywhere. Institutional preconditions might also be a key factor for the success of RB schemes if the role of self-efficacy comes into play. In countries or regions, where farmers’ knowledge about the causal links between management measures and environmental effects might be lower due to lower education and/or little previous experience with AECMs, farmers’ self-efficacy is also expected to be low, the introduction of RB schemes might be a challenge. Measures, however, to increase self-efficacy, such as enhancing education and/or providing knowledge support and training, place high demands on institutional structures. Especially in regions or countries, where extension services structures or other networks for knowledge transfer are not well developed or even lacking, the implementation of RB schemes may fail, either due to the lack of acceptance by the farmers or, in the worst case, due to the incapability of the farmers to develop and implement own management measures to achieve the environmental objectives. Finally, in light of climate change and the biodiversity crisis, external factors, i.e. circumstances outside the control of the farmers such as extreme weather events or other unexpected natural processes, will possibly even further increase and thereby enhance farmers’ risk of non-compliance.
In conclusion, we want to give food for thought that the decision for introducing RB schemes should be taken with caution, and consider institutional capacities and framework conditions, as such schemes might not work in every context, and for every AECPG targeted.
6. Conclusion
The relatively simplistic conceptualization of farmer behaviour, through the overemphasis of economic factors in policy design, compromises the effectiveness of environmental payments in the CAP (Brown et al. Citation2021). Literature suggests that socio-psychological behavioural factors need to be better understood, as they might hamper or promote the acceptance of novel AECS (Dessart, Barreiro-Hurlé, and Van Bavel Citation2019). In order to address this knowledge gap, our study utilises a basic TAM (Davis, Citation1989), incorporating socio-psychological factors as determinants for farmers’ intention to perform RB schemes. In general, we show that the TAM is suitable for predicting farmers’ intention to perform RB schemes. By extending the basic TAM with additional factors, we achieve an improved explanatory power for the factors influencing farmers’ decisions.
Our study reveals a positive inclination among Austrian farmers towards participating in RB schemes. This positive inclination, together with the characteristic of RB schemes, tying payments to measurable improvements in biodiversity or soil quality, positions them as a valuable means to effectively enhance the provision of public goods on agricultural land. Our study also shows that the behavioural factors of self-efficacy, perceived risk, and subjective norm directly or indirectly impact farmers’ intentions to perform RB schemes. Given the demonstrated significance of subjective norm, for successful implementation of RB schemes promoting them within farmers’ social networks might boost adoption, as the opinions of fellow farmers and the farming community play a crucial role in influencing farmers’ decisions to participate. In light of the influence of self-efficacy, actions to enhance farmers’ ability to implement such schemes, including knowledge support, training, and advice, especially during the introductory phase of the schemes, is recommended. Addressing the role of risk, we further suggest implementing mechanisms for their distribution. Hybrid solutions, such as combining action-based basic AECMs with additional result-based elements within a single scheme, might serve as an effective means.
Ethics
The study has received funding from the project CONSOLE within the European Union’s Horizon 2020 Research and Innovation Programme (grant agreement No 817949). It was carried out in fulfilment of the ethical requirements mandatory for EU Horizon 2020 project grant agreements, concerning (a) the identification and recruitment of research participants, (b) informed consent procedures implemented for the participation of humans and (c) personal data processing and data protection in line with Regulation (EU) 2016/679 on the protection of natural persons with regard to the processing of personal data and on the free movement of such data (General Data Protection Regulation) and, therefore, adheres to the principles of good research practice, including confidentiality, written informed consent queries and anonymity of participants.
Supplemental Material
Download MS Word (454.3 KB)Acknowledgements
The content of this article does not reflect the official opinion of the European Commission. The views expressed in this article are solely those of the authors.
Disclosure statement
No potential conflict of interest was reported by the author(s).
Supplemental data
Supplemental data for this article can be accessed here.
Additional information
Funding
Notes
1 Indicating that PU3 provides a smaller explanatory contribution to the construct PU, to avoid only two indicators we did not exclude it.
2 Only the indicator PU3 was slightly under the SCM value of 0.4 and is thus considered less important.
3 The sample size (N) should be bigger than or equal to five times the number of parameters (t) (in this study N = 235 and t = 22) (Loehlin Citation1987).
4 Minimum Discrepancy Function by Degrees of Freedom divided.
5 Root Mean Square Error of Approximation.
6 Standardized Root Mean Squared Residual.
7 Comparative Fit Index.
8 Incremental Fit Index.
9 Tucker-Lewis Index.
References
- Abdullah, Fazil, and Rupert Ward. 2016. “Developing a General Extended Technology Acceptance Model for E-Learning (GETAMEL) by Analysing Commonly Used External Factors.” Computers in Human Behavior 56: 238–256. doi:10.1016/j.chb.2015.11.036.
- Adnan, Nadia, Shahrina Md Nordin, and Zulqarnain bin Abu Bakar. 2017. “Understanding and Facilitating Sustainable Agricultural Practice: A Comprehensive Analysis of Adoption Behaviour among Malaysian Paddy Farmers.” Land Use Policy 68: 372–382. doi:10.1016/j.landusepol.2017.07.046.
- Ajzen, Icek, and Martin Fishbein. 1980. Understanding Attitudes and Predicting Social Behavior. Englewood Cliffs, NJ: Prentice-Hall.
- Ajzen, Icek. 1985. “From Intentions to Actions: A Theory of Planned Behavior.” In Action Control, 11–39. Berlin, Heidelberg: Springer. doi:10.1007/978-3-642-69746-3_2.
- Ajzen, Icek. 1988. Attitudes, Personality, and Behavior. Chicago: Dorsey.
- Ajzen, Icek. 1991. “The Theory of Planned Behavior.” Organizational Behavior and Human Decision Processes 50 (2): 179–211. doi:10.1016/0749-5978(91)90020-T.
- Allen, B., K. Hart, G. Radley, G. Tucker, C. Keenleyside, R. Oppermann, E. Underwood, et al. 2014. “Biodiversity Protection through Results Based Remuneration of Ecological Achievement: Report Prepared for the European Commission.” DG Environment, Contract No ENV.B.2/ETU/2013/0046. London: Institute for European Environmental Policy. http://ec.europa.eu/environment/nature/rbaps/handbook/docs/rbaps-report.pdf.
- AMA. 2023. “ÖPUL 2023 - Ergebnisorientierte Bewirtschaftung.” https://www.ama.at/getattachment/8de8d7a0-5955-4012-89b4-09f4a2c51ee8/O6_19_Ergebnisorientierte_Bewirtschaftung_2023_04.pdf.
- Amberg, Michael, Markus Hirschmeier, and Jens Wehrmann. 2004. “The Compass Acceptance Model for the Analysis and Evaluation of Mobile Services.” International Journal of Mobile Communications 2 (3): 248–259. doi:10.1504/IJMC.2004.005163.
- Austin, E. J., J. Willock, I. J. Deary, G. J. Gibson, J. B. Dent, G. Edwards-Jones, O. Morgan, R. Grieve, and A. Sutherland. 1998. “Empirical Models of Farmer Behaviour Using Psychological, Social and Economic Variables. Part II: Nonlinear and Expert Modelling.” Agricultural Systems 58 (2): 225–241. doi:10.1016/S0308-521X(98)00067-5.
- Ayambire, Raphael Anammasiya, and Jeremy Pittman. 2021. “Adaptive Co-Management of Environmental Risks in Result-Based Agreements for the Provision of Environmental Services: A Case Study of the South of the Divide Conservation Action Program.” Journal of Environmental Management 295: 113111. doi:10.1016/j.jenvman.2021.113111.
- Bagozzi, Richard P., and H. Baumgartner. 1994. “The Evaluation of Structural Equation Models and Hypotheses Testing.” In Principles of Marketing Research, edited by Richard P Bagozzi, 386–422. Cambridge: Blackwell.
- Bagozzi, Richard P. 1980. Causal Modeling in Marketing. New York: John Wiley & Sons.
- Bandura, Albert. 1994. “Self-Efficacy.” In Vol 4 of International Encyclopedia of the Social & Behavioral Sciences: Second Edition, edited by V.S. Ramachaudran, 71–81. New York: Academic Press.
- Batáry, Péter, Lynn V. Dicks, David Kleijn, and William J. Sutherland. 2015. “The Role of Agri-Environment Schemes in Conservation and Environmental Management.” Conservation Biology: The Journal of the Society for Conservation Biology 29 (4): 1006–1016. doi:10.1111/cobi.12536.
- Birge, Traci, Marjaana Toivonen, Minna Kaljonen, and Irina Herzon. 2017. “Probing the Grounds: Developing a Payment-by-Results Agri-Environment Scheme in Finland.” Land Use Policy 61: 302–315. doi:10.1016/j.landusepol.2016.11.028.
- Blasch, J., B. Van Der Kroon, P. Van Beukering, R. Munster, S. Fabiani, P. Nino, and S. Vanino. 2022. “Farmer Preferences for Adopting Precision Farming Technologies: A Case Study from Italy.” European Review of Agricultural Economics 49 (1): 33–81. doi:10.1093/erae/jbaa031.
- Bollen, K. 1989. Structural Equation Models with Latent Variables. New York: John Wiley & Sons.
- Borges, João Augusto Rossi, Alfons G. J. M. Oude Lansink, Claudio Marques Ribeiro, and Vanessa Lutke. 2014. “Understanding Farmers’ Intention to Adopt Improved Natural Grassland Using the Theory of Planned Behavior.” Livestock Science 169: 163–174. doi:10.1016/j.livsci.2014.09.014.
- Brown, Calum, Eszter Kovács, Irina Herzon, Sergio Villamayor-Tomas, Amaia Albizua, Antonia Galanaki, Ioanna Grammatikopoulou, Davy McCracken, Johanna Alkan Olsson, and Yves Zinngrebe. 2021. “Simplistic Understandings of Farmer Motivations Could Undermine the Environmental Potential of the Common Agricultural Policy.” Land Use Policy 101 (105136): 105136. doi:10.1016/j.landusepol.2020.105136.
- Brown, Calum, Peter Alexander, Sascha Holzhauer, and Mark D. A. Rounsevell. 2017. “Behavioral Models of Climate Change Adaptation and Mitigation in Land-Based Sectors.” Wiley Interdisciplinary Reviews: Climate Change 8 (2): E 448. doi:10.1002/wcc.448.
- Browne, Michael W., and Robert Cudeck. 1992. “Alternative Ways of Assessing Model Fit.” Sociological Methods & Research 21: 230–258. doi:10.1177/0049124192021002005.
- Burton, Rob J. F., and G. Schwarz. 2013. “Result-Oriented Agri-Environmental Schemes in Europe and Their Potential for Promoting Behavioural Change.” Land Use Policy 30 (1): 628–641. doi:10.1016/j.landusepol.2012.05.002.
- Burton, Rob J. F., and Upananda Herath Paragahawewa. 2011. “Creating Culturally Sustainable Agri-Environmental Schemes.” Journal of Rural Studies 27 (1): 95–104. doi:10.1016/j.jrurstud.2010.11.001.
- Camerer, Colin F., George Loewenstein, and Matthew Rabin. 2004. Advances in Behavioral Economics. New York: Russel Sage Foundation.
- Chaplin, S. P., J. Mills, and H. Chiswell. 2021. “Developing Payment-by-Results Approaches for Agri-Environment Schemes: Experience from an Arable Trial in England.” Land Use Policy 109: 105698. doi:10.1016/j.landusepol.2021.105698.
- Cortina, Jose M. 1993. “What Is Coefficient Alpha? An Examination of Theory and Applications.” Journal of Applied Psychology 78 (1): 98–104. doi:10.1037/0021-9010.78.1.98.
- Cullen, Paula, Mary Ryan, Cathal O’Donoghue, Stephen Hynes, Daire Huallacháin, and Helen Sheridan. 2020. “Impact of Farmer Self-Identity and Attitudes on Participation in Agri-Environment Schemes.” Land Use Policy 95: 104660. doi:10.1016/j.landusepol.2020.104660.
- Cullen, Paula, Stephen Hynes, Mary Ryan, and Cathal O'Donoghue. 2021. “More than Two Decades of Agri-Environment Schemes: Has the Profile of Participating Farms Changed?” Journal of Environmental Management 292: 112826. doi:10.1016/j.jenvman.2021.112826.
- Davis, Fred D. 1986. “A Technology Acceptance Model for Empirically Testing New End-User Information Systems: Theory and Results.” PhD diss., Massachusetts Institute of Technology.
- Davis, Fred D. 1989. “Perceived Usefulness, Perceived Ease of Use, and User Acceptance of Information Technology.” MIS Quarterly 13 (3): 319. doi:10.2307/249008.
- Davis, Fred D. 1993. “User Acceptance of Information Technology: System Characteristics, User Perceptions and Behavioral Impacts.” International Journal of Man-Machine Studies 38 (3): 475–487. doi:10.1006/imms.1993.1022.
- Davis, Fred D., Richard P. Bagozzi, and Paul R. Warshaw. 1989. “User Acceptance of Computer Technology: A Comparison of Two Theoretical Models.” Management Science 35 (8): 982–1003. http://www.jstor.org/stable/2632151. doi:10.1287/mnsc.35.8.982.
- Daxini, Amar, Mary Ryan, Cathal O’Donoghue, and Andrew P. Barnes. 2019. “Understanding Farmers’ Intentions to Follow a Nutrient Management Plan Using the Theory of Planned Behaviour.” Land Use Policy 85: 428–437. doi:10.1016/j.landusepol.2019.04.002.
- Defrancesco, Edi., Paola Gatto, and Daniele Mozzato. 2018. “To Leave or Not to Leave? Understanding Determinants of Farmers’ Choices to Remain in or Abandon Agri-Environmental Schemes.” Land Use Policy 76: 460–470. doi:10.1016/j.landusepol.2018.02.026.
- Defrancesco, Edi., Paola Gatto, Ford Runge, and Samuele Trestini. 2008. “Factors Affecting Farmers’ Participation in Agri-Environmental Measures: A Northern Italian Perspective.” Journal of Agricultural Economics 59 (1): 114–131. doi:10.1111/j.1477-9552.2007.00134.x.
- Deng, Jian, Pingsheng Sun, Fazhu Zhao, Xinhui Han, Gaihe Yang, and Yongzhong Feng. 2016. “Analysis of the Ecological Conservation Behavior of Farmers in Payment for Ecosystem Service Programs in Eco-Environmentally Fragile Areas Using Social Psychology Models.” The Science of the Total Environment 550: 382–390. doi:10.1016/j.scitotenv.2016.01.152.
- Derissen, Sandra, and Martin F. Quaas. 2013. “Combining Performance-Based and Action-Based Payments to Provide Environmental Goods under Uncertainty.” Ecological Economics 85: 77–84. doi:10.1016/j.ecolecon.2012.11.001.
- Dessart, François J., Jesús Barreiro-Hurlé, and René Van Bavel. 2019. “Behavioural Factors Affecting the Adoption of Sustainable Farming Practices: A Policy-Oriented Review.” European Review of Agricultural Economics 46 (3): 417–471. doi:10.1093/erae/jbz019.
- Ducey, Adam J., and Michael D. Coovert. 2016. “Predicting Tablet Computer Use: An Extended Technology Acceptance Model for Physicians.” Health Policy and Technology 5 (3): 268–284. doi:10.1016/j.hlpt.2016.03.010.
- Edwards-Jones, G. 2006. “Modelling Farmer Decision-Making: Concepts, Progress and Challenges.” Animal Science 82 (6): 783–790. doi:10.1017/ASC2006112.
- Eichhorn, Theresa, Jochen Kantelhardt, Lena Schaller, Fabio Bartollini, Inga Berzina, Tracy Bradfield, Micaela Cosgrove, et al. 2022. Deliverable 2.6, CONSOLE Project (No. 817949): Catalogue of Updated Factsheets of European in-Depth Case Studies. CONSOLE Project (No. 817949). https://drive.google.com/file/d/1zW6lLEX6Y84s26BzwNYF0noNer89XHZS/view.
- Eichhorn, Theresa, Jochen Kantelhardt, Lena Schaller, Flora Amery, Maria Andreoli, Fabio Bartollini, Inga Berzina, et al. 2020. Deliverable D2.1,CONSOLE Project (No. 817949): Catalogue of Descriptive Factsheets of All European Case Studies. CONSOLE Project (No. 817949). https://console-project.eu/Deliverables/Deliverable 2.1.pdf.
- European Commission. 2018. Evaluation of the Impacts of the CAP on Habitats, Landscape, Biodiversity. Brussels: European Commission.
- European Commission. 2020. “EU Biodiversity Strategy for 2030 - Bringing Nature Back into Our Lives.” COM(2020) 380 Final.” Brussels: European Commission.
- European Court of Auditors. 2011. Is Agri-Environment Support Well Designed and Managed? Special Report No 7. Luxembourg: European court of auditors.
- Fishbein, Martin, and Icek Ajzen. 1975. Belief, Attitude, Intention and Behavior: An Introduction to Theory and Research. Vol. 6. Reading, MA: Addison-Wesley. doi:10.2307/2065853.
- Fornell, Claes, and David F. Larcker. 1981. “Evaluating Structural Equation Models with Unobservable Variables and Measurement Error.” Journal of Marketing Research 18 (1): 39–50. doi:10.2307/3151312.
- Gerowitt, B., J. Isselstein, and R. Marggraf. 2003. “Rewards for Ecological Goods - Requirements and Perspectives for Agricultural Land Use.” Agriculture, Ecosystems and Environment 98 (1-3): 541–547. doi:10.1016/S0167-8809(03)00112-9.
- Gibbons, James M., Emily Nicholson, E. J. Milner-Gulland, and Julia P. G. Jones. 2011. “Should Payments for Biodiversity Conservation Be Based on Action or Results?” Journal of Applied Ecology 48 (5): 1218–1226. doi:10.1111/j.1365-2664.2011.02022.x.
- Hair, Joseph, William C. Black, Barry J. Babin, and Rolph E. Anderson. 2010. Multivariate Data Analysis. 7th ed. Prentice Hall, NJ: Pearson.
- Herzele, Ann., Van, Anne Gobin, Peter Van Gossum, Lilibeth Acosta, Tom Waas, Nicolas Dendoncker, and Bruno Henry de Frahan. 2013. “Effort for Money? Farmers’ Rationale for Participation in Agri-Environment Measures with Different Implementation Complexity.” Journal of Environmental Management 131: 110–120. doi:10.1016/j.jenvman.2013.09.030.
- Herzon, I., T. Birge, B. Allen, A. Povellato, F. Vanni, K. Hart, G. Radley, et al. 2018. “Time to Look for Evidence: Results-Based Approach to Biodiversity Conservation on Farmland in Europe.” Land Use Policy 71: 347–354. doi:10.1016/j.landusepol.2017.12.011.
- Homburg, Christian. 1998. Kundennähe Von Industriegüterunternehmen. 2nd ed. Wiesbaden: Gabler.
- Homburg, Christian, and Annette Giering. 1998. Konzeptualisierung Und Operationalisierung Komplexer Konstrukte: Ein Leitfaden Für Die Marketingforschung. Suttgart: Schäffer-Poeschel.
- Homburg, Christian, Christian Pflesser, and Martin Klarmann. 2008. “Strukturgleichungsmodelle Mit Latenten Variablen: Kausalanalyse.” In Handbuch Marktforschung: Methoden, Anwendungen, Praxisbeispiele, edited by Andreas Herrmann, 547–577. Wiesbaden: Gabler.
- Homburg, Christian, and Hans Baumgartner. 1995. “Beurteilung von Kausalmodellen: Bestandsaufnahme Und Anwendungsempfehlungen.” Marketing: Zeitschrift Für Forschung Und Praxis 17 (3): 162–176.
- Im, Il., Yongbeom Kim, and Hyo Joo Han. 2008. “The Effects of Perceived Risk and Technology Type on Users’ Acceptance of Technologies.” Information & Management 45 (1): 1–9. doi:10.1016/j.im.2007.03.005.
- Jöreskog, K., and D. Sörbom. 1979. Advances in Factor Analysis and Structural Equation Models. New York: University Press of America.
- Josefsson, Jonas, Anne Marike Lokhorst, Tomas Pärt, Åke Berg, and Sönke Eggers. 2017. “Effects of a Coordinated Farmland Bird Conservation Project on Farmers’ Intentions to Implement Nature Conservation Practices – Evidence from the Swedish Volunteer & Farmer Alliance.” Journal of Environmental Management 187: 8–15. doi:10.1016/j.jenvman.2016.11.026.
- Kaiser, Henry F., and John Rice. 1974. “Little Jiffy, Mark Iv.” Educational and Psychological Measurement 34 (1): 111–117. doi:10.1177/001316447403400115.
- Kaplan, Rachel, and Stephen Kaplan. 2008. “Bringing Out the Best in People: A Psychological Perspective.” Conservation Biology: The Journal of the Society for Conservation Biology 22 (4): 826–829. doi:10.1111/j.1523-1739.2008.01010.x.
- King, William R., and Jun He. 2006. “A Meta-Analysis of the Technology Acceptance Model.” Information & Management 43 (6): 740–755. doi:10.1016/j.im.2006.05.003.
- Kline, Rex. 2016. Principles and Practice of Structural Equation Modeling. New York: The Guilford Press. doi:10.15353/cgjsc.v1i1.3787.
- Läpple, Doris, and Hugh Kelley. 2013. “Understanding the Uptake of Organic Farming: Accounting for Heterogeneities among Irish Farmers.” Ecological Economics 88: 11–19. doi:10.1016/j.ecolecon.2012.12.025.
- Lee, Younghwa, Kenneth A. Kozar, and Kai R. T. Larsen. 2003. “The Technology Acceptance Model: Past, Present, and Future.” Communications of the Association for Information Systems 12: 752–780 doi:10.17705/1CAIS.01250.
- Loehlin, J. C. 1987. Latent Variable Models. Hillsdale: Lawrence Erlbaum Associates.
- Mack, Gabriele, Christian Ritzel, and Pierrick Jan. 2020. “Determinants for the Implementation of Action-, Result- and Multi-Actor-Oriented Agri-Environment Schemes in Switzerland.” Ecological Economics 176: 106715. doi:10.1016/j.ecolecon.2020.106715.
- Massfeller, Anna, Manuela Meraner, Silke Hüttel, and Reinhard Uehleke. 2022. “Farmers’ Acceptance of Results-Based Agri-Environmental Schemes: A German Perspective.” Land Use Policy 120: 106281. doi:10.1016/j.landusepol.2022.106281.
- Matzdorf, Bettina, and Jana Lorenz. 2010. “How Cost-Effective Are Result-Oriented Agri-Environmental Measures? – An Empirical Analysis in Germany.” Land Use Policy 27 (2): 535–544. doi:10.1016/j.landusepol.2009.07.011.
- Matzdorf, Bettina, Thomas Kaiser, and Maria Sofie Rohner. 2008. “Developing Biodiversity Indicator to Design Efficient Agri-Environmental Schemes for Extensively Used Grassland.” Ecological Indicators 8 (3): 256–269. doi:10.1016/j.ecolind.2007.02.002.
- Naspetti, Simona, Serena Mandolesi, Jeroen Buysse, Terhi Latvala, Philippa Nicholas, Susanne Padel, Ellen J. Van Loo, and Raffaele Zanoli. 2017. “Determinants of the Acceptance of Sustainable Production Strategies Among Dairy Farmers: Development and Testing of a Modified Technology Acceptance Model.” Sustainability 9 (10): 1805. doi:10.3390/su9101805.
- Park, Namkee, Mohja Rhoads, Jinghui Hou, and Kwan Min Lee. 2014. “Understanding the Acceptance of Teleconferencing Systems Among Employees: An Extension of the Technology Acceptance Model.” Computers in Human Behavior 39: 118–127. doi:10.1016/j.chb.2014.05.048.
- Pe’er, G., L. V. Dicks, P. Visconti, R. Arlettaz, A. Báldi, T. G. Benton, S. Collins, et al. 2014. “EU Agricultural Reform Fails on Biodiversity.” Science (New York, N.Y.) 344 (6188): 1090–1092. doi:10.1126/science.1253425.
- Pe’er, Guy., Aletta Bonn, Helge Bruelheide, Petra Dieker, Nico Eisenhauer, Peter H. Feindt, Gregor Hagedorn, et al. 2020. “Action Needed for the EU Common Agricultural Policy to Address Sustainability Challenges.” People and Nature 2 (2): 305–316. doi:10.1002/pan3.10080.
- Pe’er, Guy., Yves Zinngrebe, Francisco Moreira, Clélia Sirami, Stefan Schindler, Robert Müller, Vasileios Bontzorlos, et al. 2019. “A Greener Path for the EU Common Agricultural Policy.” Science (New York, N.Y.) 365 (6452): 449–451. doi:10.1126/science.aax3146.
- Pierce, Tamra P., Christopher Willy, Robert Roncace, and John Bischoff. 2014. “Extending The Technology Acceptance Model: Policy Acceptance Model (PAM).” American Journal of Health Sciences 5 (2): 129–144. doi:10.19030/ajhs.v5i2.8963.
- Rezaei, Rohollah, Leila Safa, and Mohammad Mahdi Ganjkhanloo. 2020. “Understanding Farmers’ Ecological Conservation Behavior Regarding the Use of Integrated Pest Management: An Application of the Technology Acceptance Model.” Global Ecology and Conservation 22: e00941. doi:10.1016/j.gecco.2020.e00941.
- Rose, D. C., C. Keating, and C. Morris. 2018. Understanding How to Influence Farmers’ Decision-Making Behaviour: A Social Science Literature Review, Report for the Agriculture and Horticulture Development Board, Supported by UEA Consulting Ltd. https://projectblue.blob.core.windows.net/media/Default/Imported Publication Docs/FarmersDecisionMaking_2018_09_18.pdf.
- Runge, Tania, Uwe Latacz-Lohmann, Lena Schaller, Kristina Todorova, Carsten Daugbjerg, Mette Termansen, Jaan Liira, et al. 2022. “Implementation of Eco-Schemes in Fifteen European Union Member States.” EuroChoices 21 (2): 19–27. doi:10.1111/1746-692X.12352.
- Sabatier, Rodolphe, Luc Doyen, and Muriel Tichit. 2012. “Action Versus Result-Oriented Schemes in a Grassland Agroecosystem: A Dynamic Modelling Approach.” PloS One 7 (4): e33257. doi:10.1371/journal.pone.0033257.
- Sattler, Claudia, and Uwe Jens Nagel. 2010. “Factors Affecting Farmers’ Acceptance of Conservation Measures – A Case Study from North-Eastern Germany.” Land Use Policy 27 (1): 70–77. doi:10.1016/j.landusepol.2008.02.002.
- Schaak, Henning, and Oliver Mußhoff. 2018. “Understanding the Adoption of Grazing Practices in German Dairy Farming.” Agricultural Systems 165: 230–239. doi:10.1016/j.agsy.2018.06.015.
- Schulze, Holger, and Achim Spiller. 2010. “Farmers’ Acceptance of the Organic Certification System in Germany: A Partial Least Squares Model.” Journal of International Food & Agribusiness Marketing 22 (1-2): 7–36. doi:10.1080/08974430903372724.
- Schwarz, Gerald, Andrew Moxey, Davy McCracken, Sally Huband, and Roger Cummins. 2008. “An Analysis of the Potential Effectiveness of a Payment-by-Results Approach to the Delivery of Environmental Public Goods and Services Supplied by Agri-Environment Schemes.” Report to the Land Use Policy Group, UK. Peterborough: Macaulay Institute, Pareto Consulting and Scottish Agricultural College.
- Science for Environment Policy. 2017. Agri-Environmental Schemes: Impacts on the Agricultural Environment. Edited by UWE. Bristol: Issue produced for the European Commission DG Environment by the Science Communication Unit. doi:10.2779/633983.
- Scown, Murray W., Mark V. Brady, and Kimberly A. Nicholas. 2020. “Billions in Misspent EU Agricultural Subsidies Could Support the Sustainable Development Goals.” One Earth (Cambridge, Mass.) 3 (2): 237–250. doi:10.1016/j.oneear.2020.07.011.
- Sharifzadeh, Mohammad Sharif, Christos A. Damalas, Gholamhossein Abdollahzadeh, and Hossein Ahmadi-Gorgi. 2017. “Predicting Adoption of Biological Control among Iranian Rice Farmers: An Application of the Extended Technology Acceptance Model (TAM2).” Crop Protection 96: 88–96. doi:10.1016/j.cropro.2017.01.014.
- Sheppard, Blair H., Jon Hartwick, and Paul R. Warshaw. 1988. “The Theory of Reasoned Action: A Meta-Analysis of Past Research with Recommendations for Modifications and Future Research.” Journal of Consumer Research 15 (3): 325. doi:10.1086/209170.
- Siebert, Rosemarie, Mark Toogood, and Andrea Knierim. 2006. “Factors Affecting European Farmers’ Participation in Biodiversity Policies.” Sociologia Ruralis 46 (4): 318–340. doi:10.1111/j.1467-9523.2006.00420.x.
- Snoo, Geert R. de., Irina Herzon, Henk Staats, Rob J. F. Burton, Stefan Schindler, Jerry van Dijk, Anne Marike Lokhorst, et al. 2013. “Toward Effective Nature Conservation on Farmland: Making Farmers Matter.” Conservation Letters 6 (1): 66–72. doi:10.1111/j.1755-263X.2012.00296.x.
- Stolze, M., R. Frick, O. Schmid, S. Stöckli, D. Bogner, V. Chevillat, M. Dubbert, et al. 2015. Result-Oriented Measures for Biodiversity in Mountain Farming Result-Oriented Measures for Biodiversity in Mountain Farming A Policy Handbook. Frick, Switzerland: Research Institute of Organic Agriculture (FIBL).
- Tarka, Piotr. 2018. “An Overview of Structural Equation Modeling: Its Beginnings, Historical Development, Usefulness and Controversies in the Social Sciences.” Quality & Quantity 52 (1): 313–354. doi:10.1007/s11135-017-0469-8.
- Temme, Dirk, and Lutz Hildebrandt. 2009. “Gruppenvergleiche Bei Hypothetischen Konstrukten—Die Prüfung Der Übereinstimmung Von Messmodellen Mit Der Strukturgleichungsmethodik.” Schmalenbachs Zeitschrift Für Betriebswirtschaftliche Forschung 61 (2): 138–185. doi:10.1007/BF03372818.
- Trujillo-Barrera, Andres, Joost M. E. Pennings, and Dianne Hofenk. 2016. “Understanding Producers’ Motives for Adopting Sustainable Practices: The Role of Expected Rewards, Risk Perception and Risk Tolerance.” European Review of Agricultural Economics 43 (3): 359–382. doi:10.1093/erae/jbv038.
- Uthes, Sandra, and Bettina Matzdorf. 2013. “Studies on Agri-Environmental Measures: A Survey of the Literature.” Environmental Management 51 (1): 251–266. doi:10.1007/s00267-012-9959-6.
- Venkatesh, Viswanath, and Fred D. Davis. 2000. “Theoretical Extension of the Technology Acceptance Model: Four Longitudinal Field Studies.” Management Science 46 (2): 186–204. doi:10.1287/mnsc.46.2.186.11926.
- Venkatesh, Viswanath. 2000. “Determinants of Perceived Ease of Use: Integrating Perceived Behavioral Control, Computer Anxiety and Enjoyment into the Technology Acceptance Model.” Information Systems Research 11 (4): 342–365. doi:10.1287/isre.11.4.342.11872.
- Verma, Surabhi, Som Sekhar Bhattacharyya, and Saurav Kumar. 2018. “An Extension of the Technology Acceptance Model in the Big Data Analytics System Implementation Environment.” Information Processing & Management 54 (5): 791–806. doi:10.1016/j.ipm.2018.01.004.
- Walker, L. K., A. J. Morris, A. Cristinacce, D. Dadam, P. V. Grice, and W. J. Peach. 2018. “Effects of Higher-Tier Agri-Environment Scheme on the Abundance of Priority Farmland Birds.” Animal Conservation 21 (3): 183–192. doi:10.1111/acv.12386.
- Wąs, Adam, Agata Malak-Rawlikowska, Matteo Zavalloni, Davide Viaggi, Paweł Kobus, and Piotr Sulewski. 2021. “In Search of Factors Determining the Participation of Farmers in Agri-Environmental Schemes – Does Only Money Matter in Poland?” Land Use Policy 101: 105190. doi:10.1016/j.landusepol.2020.105190.
- Wauters, Erwin, Charles Bielders, Jean Poesen, Gerard Govers, and Erik Mathijs. 2010. “Adoption of Soil Conservation Practices in Belgium: An Examination of the Theory of Planned Behaviour in the Agri-Environmental Domain.” Land Use Policy 27 (1): 86–94. doi:10.1016/j.landusepol.2009.02.009.
- Weiber, Rolf, and Daniel Mühlhaus. 2014. Strukturgleichungsmodellierung. Berlin Heidelberg: Springer-Verlag.
- Wezel, Alexander, Audrey Vincent, Heike Nitsch, Otto Schmid, Monika Dubbert, Erich Tasser, Philippe Fleury, Sybille Stöckli, Matthias Stolze, and Daniel Bogner. 2018. “Farmers’ Perceptions, Preferences, and Propositions for Result-Oriented Measures in Mountain Farming.” Land Use Policy 70 (May 2017): 117–127. doi:10.1016/j.landusepol.2017.10.020.
- Willock, Joyce, Ian J. Deary, Gareth Edwards-Jones, Gavin J. Gibson, Murray J. McGregor, Alistair Sutherland, J. Barry Dent, Oliver Morgan, and Robert Grieve. 1999. “The Role of Attitudes and Objectives in Farmer Decision Making: Business and Environmentally-Oriented Behaviour in Scotland.” Journal of Agricultural Economics 50 (2): 286–303. doi:10.1111/j.1477-9552.1999.tb00814.x.
- Wuepper, David, and Robert Huber. 2022. “Comparing Effectiveness and Return on Investment of Action- and Results-Based Agri-Environmental Payments in Switzerland.” American Journal of Agricultural Economics 104 (5): 1585–1604. doi:10.1111/ajae.12284.
- Zabel, Astrid, and Brian Roe. 2009. “Optimal Design of Pro-Conservation Incentives.” Ecological Economics 69 (1): 126–134. doi:10.1016/j.ecolecon.2009.08.001.