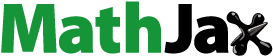
ABSTRACT
Numerous eligible German students do not apply for heavily subsidized student aid (BAföG). Our theoretical model shows how imperfect information, risk aversion, debt aversion, and student income can incentivize rational students to abstain from an application. We use GSOEP data from 2001 to 2013 to simulate BAföG eligibility and find that in particular risk averse students with low income are discouraged if they have little information about the application procedure. Moreover, debt-averse students are more reluctant to apply. This suggests that a non-transparent and complicated student aid system disproportionally discourages poor students with little experience with the BAföG system.
Introduction
Tuition fees at German federal institutions of higher education are virtually non-existent, and only small administration fees have to be paid by students. What remains to be covered are expenses for basic needs and accommodation. Among several options to finance living expenses, students can receive financial help from their parents, work to earn their own income, or apply for scholarships and student federal aid, also known as BAföG, which is provided by the Federal Training Assistance Act (Bundesausbildungsförderungsgesetz). Depending on various socioeconomic factors, students can receive monthly BAföG payments for the nominal length of their studies. Half of the total BAföG aid is a grant that does not have to be paid back and the other half is an interest-free loan with the repayment being capped at 10,000 (Bundesministerium für Bildung und Forschung Citation2017). According to the latest student survey ‘21. Sozialerhebung’ published by the Federal Ministry of Education and Research, approximately 79% of students who receive BAföG state that without federal aid they would have not been able to study (Middendorff et al. Citation2017).
In 2012, approximately 67% of students were formally eligible to receive BAföG, i.e. they met the prerequisite to be still under 30 years old when starting their first full-time study program (Herber and Kalinowski Citation2019). Out of these formally eligible students, only 28% received BAföG (Deutscher Bundestag Citation2014). There are two potential explanations behind the low share of recipients: Either the students fail the means test for student aid, or they choose to not apply for aid even though they are eligible for a positive aid amount. While the former explanation is in line with the purpose of the BAföG system, the latter would mean that it fails to support students that are targeted by the system.
The previously mentioned student survey Sozialerhebung provides some insights why some of the students did not file a BAföG application form in the first place. As the top runner, 76% of students state that according to their own expectations, either their parents’ or partner's income was too high, followed by 30% of students saying that their own income was too high. Remarkably, 25% of all students state that they did not apply due to the fact that they did not want to accumulate debt. When only students from lower educational backgrounds are considered, the share of students who state that making debt was the main reason for non-application increases to 37% (Middendorff et al. Citation2017).
This paper shows how the combination of information frictions, attitude towards risk, student income and debt aversion can rationalize this behavior. A student who is eligible for aid but does not apply forgoes financial resources, considering that in the German student aid system, half of the payment is a free subsidy to the student and the other half is a zero-interest loan. Essentially, not applying for BAföG directly translates into rejecting free money. This is particularly controversial for students in Germany, since upward mobility for German students to tertiary education is still low compared to other OECD countries (OECD Citation2016; Herber and Kalinowski Citation2019). Germany has a low participation rate in higher education and also the lowest growth rate in tertiary education in Europe, which Powell and Solga (Citation2011) call ‘German exceptionalism’. Student aid is supposed to increase enrollment rates to higher education institutions (Cornwell, Mustard, and Sridhar Citation2006; John and Noell Citation1989) and encourage potential students to enroll earlier to universities (Steiner and Wrohlich Citation2012). Moreover, financial aid can also raise the probability that a student finishes her studies successfully instead of dropping out early (Glocker Citation2011).
We first build a theoretical model that illustrates the tradeoff of a rational student. In the presence of imperfect information about the entitled amount of aid, risk aversion, debt aversion, and a costly application process, a student might find it optimal to refrain from applying for aid. The model shows that stronger information frictions give rise to a higher likelihood for non-application. While in general, poorer students are more likely to apply for aid, they are also more affected by these information frictions. Moreover, the more risk-averse a student is, the higher is the likelihood to turn down the application. A student with higher risk aversion reacts also more severely to any changes in the information level as well as changes in student income. Lastly, a high degree of debt aversion deters students from applying for BAföG, irrespective of all other factors.
These hypotheses are tested using panel data provided by the GSOEP (German Socio-Economic Panel, Goebel et al. (Citation2019)), in which students during the years 2001–2013 are observed. Since the GSOEP does not include information on students’ eligibility for BAföG, we simulate for each student potential BAföG amounts. We only keep eligible students in the sample, which then reduces the number to observations or
students over 13 years. We first use a pooled probit model to predict the probability to not receive BAföG conditional on being eligible. We then control for unobserved heterogeneity with a correlated random effects (CRE) probit model and find evidence for unobserved heterogeneity. The CRE probit shows that an increase of the monthly parental gross labor income by 1% is associated with a remarkable 34.4% increase of the probability to not apply for BAföG despite eligibility (significant at 0.1%-level). This suggests that the misconceptions about eligibility increase with parental income. If the age of a student increases by 1 year, the probability to reject BAföG is 3.3% higher, significant at a 0.1% level in the pooled probit. Accordingly, older students assume to not be eligible even though they are still within the actual age limit. A three-way interaction testing for the relationship between risk aversion, income and information level is highly significant at a 0.1% significance level.
Students with high risk aversion and strong information frictions (no siblings receiving BAföG or being an only child) are particularly likely to not apply for aid. Additional information via a sibling receiving BAföG is associated with a lower non-take-up rate, whereas there is a stronger relationship for poorer students. Students with low risk aversion are more likely to apply for student aid in general, while the level of information has a very moderate effect on that probability. Moreover, we find that if a student is more accustomed to debt because her family is paying back monthly credit rates, the probability to reject BAföG decreases by 6.9% at a 10% significance level in the pooled probit. This suggests that debt-averse students are more likely to refrain from an application for aid.
The remainder of the paper is structured as follows: Chapter 2 provides some background information about the BAföG system and reviews the most relevant literature. In chapter 3, we build a simple theoretical model to illustrate the student's application decision and derive several testable hypotheses. Chapter 4 tests these hypotheses using GSOEP data and discusses the empirical results. The 5th chapter concludes.
Literature review and background information
Financing studies in Germany
The Sozialerhebung reports that the average disposable income of a student was 918 EUR per month in 2016, where the most important income source was financial help from parents and student's own labor income. Only 1/4 of all students received BAföG with an average amount of 435 EUR per month. Other minor income sources were savings, financial help from relatives and partners, scholarships, and student loans such as the KfW credit (Middendorff et al. Citation2017).
When introduced in 1970, BAföG was a 100% grant for students and about 45% of all students were receiving it. Since then, the system has been adjusted and reformed multiple times. In 1979, the age limit was decreased from 35 to 30 (Deutscher Gewerkschaftsbund Citation2016). Starting in 1982, students could only receive an interest-free loan as aid, while in 1991, BAföG has been modified to a grant-loan scheme. In the past decades, the share of students who have been supported by BAföG has decreased over time. Nowadays, the downward trend of eligibility for aid is still ongoing and the government tries to cope with this problem by increasing income set-offs and maximum aid entitlements. Recent reforms capped the total amount of debt at 10,000 EUR (2001), introduced exemptions for child allowances by the government (2001) and popular ‘mini-jobs’ with low salaries (2008), increased entitlements by 2% (2010) and rose the age limit for Master students from originally 30 to now 35 years (Deutsches Studentenwerk Citation2017).
In the 21th BAföG report the federal government states that the total number of students has increased by 15% (from 2,358,000 to 2,709,000) between 2012 and 2016. Also, the share of formally eligible students increased by 9% (from 1,572,000 to 1,709,000). However, the number of aid-receiving students has decreased by 14.3% (from 440,000 to 377,000) (Deutscher Bundestag Citation2017).
In contrast to the FAFSA that serves as an application for multiple grants (esp. the Pell Grant and FSEOG) and various federal loans (both subsidized and unsubsidized) in the USA, the application for the German BAföG is solely used to determine eligibility for one standardized type of student aid. One-half of the BAföG aid is paid as a grant and the other half as a zero-interest loan that is capped at 10,000 EUR and comes with a 5-year grace period. The application process for the German BAföG is generally perceived to be too complex by itself, intimidating students and posing an additional hurdle for the application. Amongst other data, the applicant must provide information on 14 different types of own income, 16 different types of assets and debt, the parents have to fill out a 4-page form with information on their different types of income and potentially siblings, while interestingly, the parents’ wealth is irrelevant. Additional forms must be supplied by students who are non-citizens, want to study abroad, and students with own children. Before 2016, applications could only be submitted in hardcopy form. First-time applicants need on average 335 min to fill out the BAföG form (261 min for a continuation form). The administration needs on average 64 min to handle an application (52 min for a continuation form), and students wait on average 54 days until a decision has been made, with some exceptional cases taking up to 6 months (Bundeskanzleramt und Nationaler Normenontrollrat Citation2010).
Literature
Federal student aid via BAföG is a specific type of governmental aid and its implementation might be subject to barriers that are similar to other public aid measures. Bruckmeier et al. (Citation2013) analyze a sample survey of income and expenditure in 2008 and find that approximately 34–43% of all the people who are eligible for unemployed benefits (Arbeitslosengeld II) in Germany do not apply for public assistance. Kayser and Frick (Citation2000) analyze social assistance (Sozialhilfe) for German households via the GSOEP in 1996 and find that the non-take-up rateFootnote1 accounts to 63.1%, whereas stigma, application cost and social ties affect the decision of a(n) (non-) application.
While there is no evidence that such a stigma is attached to receiving student aid, imperfect information and a complicated formal procedure can prevent students from applying for aid. Bettinger et al. (Citation2012) consider the complexity of the Free Application for Student Federal Aid (FAFSA). They show that assisting students through the application process and providing more information about the program increases the likelihood of FAFSA completions as well as the likelihood to attend college, persevere until graduation, and receive aid. In contrast, Booji, Leuven, and Oosterbeek (Citation2012) don’t find evidence that informing Dutch students about loan conditions gives rise to higher participation rates. Dynarski and Scott-Clayton (Citation2006) find that FAFSA compliance costs are higher for students from low-income families, which counteracts the goal of helping especially students with limited financial support. King (Citation2004) reports that 1.7 million low- to middle-income students did not apply for FAFSA in 1999–2000 and estimates that ‘850,000 students who did not file a FAFSA were likely to have been eligible for a Pell Grant.’ Kofoed (Citation2017) confirms these findings with a multinomial logit, reporting ‘that students who are lower middle income, white, male and independent from parents are less likely to complete FAFSA even when they are eligible for aid’. Moreover, he uses propensity score matching to estimate that each year, students lose $9,741.05 ($24 billion for all students combined) in total aid.
Some students might worry about the lack of sufficiently high income in order to pay back the loan part of BAföG. Approximately 28% of Bachelor students (11% of Master students) in Germany drop out of higher education before graduation (Heublein et al. Citation2014). Ortiz-Nuñez (Citation2014) finds that a student's willingness to take financial risk increases the probability to take out a student loan. Monge-Naranjo (Citation2016) considers youth unemployment right after graduation as a reason for the relatively low take-up rate of student loans and suggests that, for instance, students need to have an unemployment compensation scheme within the loan program. A related effect is already implemented in the German student aid system: German students who received BAföG during their studies have a grace period of 5 years after the nominal end of their first degree. Afterwards, they can apply for an extension of this grace period if their income is below a threshold, which was 1145 EUR for singles in 2018.
In the student survey 21. Sozialerhebung, 25% of all students who have never applied for BAföG state their unwillingness to accumulate debt as a reason for not applying (Middendorff et al. Citation2017). Cunningham and Santiago (Citation2008) find that family and societal characteristics matter for the degree of debt aversion. In a related study, Caetano, Palacios, and Patrinos (Citation2011) analyze the psychological aversion to debt and show that the word ‘debt’ is negatively perceived and intimidates students to apply for federal aid, whereas ‘Human Capital Contract’ seems to be a more agreeable term to promote student aid take-up. Eckel et al. (Citation2007) point out that students might already have high debt levels that keep them from entering further debt contracts. Cadena and Keys (Citation2013) use a linear probability model to show for the US that ‘students who live off-campus are 8.0 percentage points less likely to accept their loans than are students in the same financial situation living on campus’, arguing that off-campus students without self-control might consciously prevent themselves from spending the loan that is partially paid in cash on non-educations related activities.
In a study that focusses on student aid in Germany (BAföG), Herber and Kalinowski (Citation2019) build a micro-simulation model to distinguish between actually eligible and non-eligible students, restricting their sample to the former group. Their basic results from the pooled probit model show that if the student's potential aid amount increases, the non-take-up rate is lower. For students who have older siblings that claimed BAföG before, the non-take-up rate decreases, which indicates that siblings mitigate the problem of information restrictions. Also, impatient and impulsive students have a higher non-take up rate, which the authors interpret as debt-averse attitude. Herber and Kalinowski (Citation2019) focus on the effect of the potential aid amount on the probability of non-take up. Bearing this in mind, they take into account the possibility that endogeneity of the level of benefits affects their results, and hence, use empirical models like the IV probit, TSLS and Heckprobit (Herber and Kalinowski Citation2019).
Steiner and Wrohlich (Citation2012) find that an increase of the BAföG amount increases the average enrollment rate in higher education. However, their
estimate of a relatively small enrollment elasticity with respect to the amount of financial student aid implies that financial incentives alone will not achieve the policy goal of substantially increasing the share of students in university education within age cohorts at feasible fiscal costs.
We address this issue by modifying and extending a simple micro-approach by Kofoed (Citation2017) to illustrate the interaction between imperfect information, attitude towards risk, and student income. We then derive several hypotheses and empirically test the interaction effect on the probability of non-take-up. Moreover, we take into consideration that our results might be driven by unobserved heterogeneity, and hence use a CRE model within the probit framework.
A model of student aid
Model setup
We assume that the exogenous non-aid income of the student is and
in the two periods. Students receive the signal
right before they can apply for aid and start studying (period 1), correlated to the actual amount
the student would receive conditional on applying for aid. A student who applies for student aid must spend additional time and resources
in period 1 to do so. As a benefit, the student will receive the additional amount
as student aid in period 1 and must pay back (a/2) in period 2. Information frictions distort the actual signal
by some noise
such that
. We assume that this distortion
is uniformly distributed according to the density function
(1)
(1)
where the exogenous can be interpreted as the extent of the information frictions. We use Epstein- Zin preferences (Epstein and Zin Citation1991, Citation1989) to separate the effect of risk aversion from the inter-temporal elasticity of substitution. The expected utility must be aggregated over the different levels of
, giving rise to
(2)
(2)
where can be interpreted as the weight of future utility relative to present utility,
as the preference for intertemporal consumption smoothing, and
as the degree of risk aversion.
Proposition 1: If (2) is strictly increasing in
.
Proof of Proposition 1 See appendix.Footnote2
Given a signal , the student will apply for student aid if
(3)
(3)
According to proposition 1, the LHS of (3) is strictly increasing in , while the RHS is constant. Thus, there exists a unique signal threshold
for which the student is indifferent between applying or not, implicitly defined by
(4)
(4)
Using the uniform distribution of , we compute the probability that a student with the eligibility for the aid amount
finds it optimal to not apply for student aid:
(5)
(5)
Simulation
We simulate the decision for students with different levels of risk aversion , high and low information frictions
, and student income
varying from monthly 100 EUR up to 400 EUR.Footnote3 We assume that the student under consideration would receive a rather moderate aid amount of 150 EUR a month, conditional on applying for student aid. In order to obtain significant rejection rates, we need to assume significant application costs of 100 EUR.Footnote4 All parameters can be found in .
Table 1. List of Parameters.
shows the outcome of the simulation for students with varying income with a rather imprecise signal (
, ‘no sibling’) and a more precise signal (
, ‘sibling’), and two different degrees of risk aversion. depicts the absolute change of the probability to not apply for an increase of x from x = 0.375 to x = 0.75. We use the outcome of the simulation to derive several hypotheses from our basic theoretical model.
Hypothesis 1 The probability to not apply for student aid is increasing in a student's income as this reduces the marginal utility of aid during her studies (see ).
Hypothesis 2 The probability to not apply for student aid is increasing in the extent of information frictions (see ).
Hypothesis 3 The effect of information on the probability to decline student aid (hypothesis 2) is stronger for poorer students. That is, the effect of an increase in on Prob(no application)
(5) declines with
(see ).
Figure 1. Prob (no application) for students with high risk aversion or low risk aversion (
) and imprecise (
, ‘no sibling’) or more precise (
, ‘sibling’) signals.
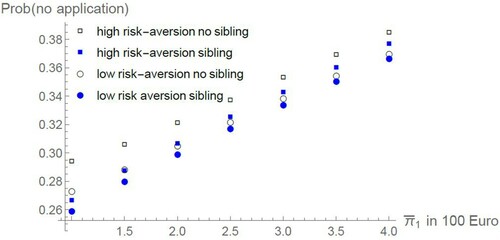
Figure 2. The effect of a less precise signal on the probability to decline student aid for students with high risk aversion or low risk aversion
.
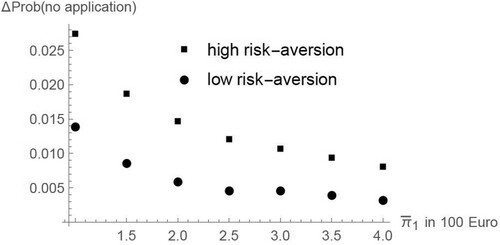
If we use to discuss the effect of risk aversion for given income and information frictions
, we obtain hypothesis 4.
Hypothesis 4 Students with higher risk-aversion are more likely to not apply for the ‘risky’ student aid.
Finally, we use again to study the interaction between risk aversion, income, and information more in detail.
Hypothesis 5 The effect of information on the probability to not apply for student aid is stronger and declines faster with income for students with high risk aversion.
As the 21. Sozialerhebung (Middendorff et al. Citation2017) and several studies suggest, aversion against debt may well deter students from applying for aid. If the effect of debt on utility is independent of the level of debt, we can modify (4) to
(6)
(6) where
denotes the disutility of having any level of debt. However, we would expect that the disutility of owing debt is also increasing in the debt amount and modify (2) to
(7)
(7) where
denotes the disutility caused by the debt level
, with
,
and
.
The effect of debt aversion is rather obvious: An increase in general debt aversion or an upward shift in
for all
raises the signal threshold
.
Hypothesis 6 An increase in debt aversion reduces the probability to apply for aid.
Empirical application
Data and sample construction
We use data from the German Socio-Economic Panel (GSOEP) (Socio-Economic Panel Citation2015), which offers microdata for research in the social and economic sciences. This longitudinal study is conducted by the DIW (Deutsches Institut für Wirtschaftsforschung) and contains information from annual surveys of private households (with a minimum respondent age of 17 years) in Germany since 1984.Footnote5
The panel contains data on a wide range of topics such as education, employment, income and health, but also on the willingness to take risk and debt. GSOEP data is used not only for academic research, but also for policy-related social reports directed at a broader audience.
We restrict the observation period of the sample to the years 2001–2013 and only include GSOEP-respondents who were studying during the time of the survey and for whom it is traceable whether they received BAföG or not. Before 2007, one variable covers the receipt of both student funding schemes. Starting in 2007, the GSOEP included an additional variable in order to explicitly identify BAföG payments. We use the variable that includes both funding schemes because this variable is available for the entire period from 2001–2013 and only very few students receive scholarships in Germany.Footnote6 We drop individuals for which we cannot identify parents, siblings and, if applicable, the partner. This restriction reduces our sample from 9,170 to 5,892 observations.
Unfortunately, the GSOEP does not provide information on the actual eligibility of a student for BAföG, let alone the amount a student does or would receive after an application. Thus, we determine eligibility by simulating potential BAföG amounts using the formula suggested by Steiner and Wrohlich (Citation2012):Footnote7
(8)
(8) where students’ eligibility for aid amount a is a function of the following variables. The upper limit for BAföG,
, is reduced if the student's main/side job income,
, exceeds the exemption threshold
. The same logic applies to parental (and if married spousal) income,
, and the total exemption threshold for parental (and spousal) income,
.
determines the rate at which the deduction is conducted, starting at 0.50 for students who have no siblings and continuously increasing by 0.05 for each sibling under the age of 18. A detailed explanation of the simulation model including information on the maximum aid amount,
and income exemptions
and
is provided in the Appendix.
Eventually, the simulation leaves us with 4,238 observations for whom we simulate aid amounts, . After considering age requirements, 4,057 observations (95.73%) are formally eligible for BAföG payments.Footnote8 Among these eligible individuals, mean aid is estimated to be 345 EUR per month in the sample over the years 2001–2013. This is reasonably close to Middendorff et al. (Citation2017), who report a mean BAföG amount in 2012 of 436 EUR per month, considering that our sample contains also observations from the previous decade. We use the simulated aid amounts to create an indicator that shows whether observation
is eligible for a positive aid amount in period
:
(9)
(9)
shows that 1,480 observations are simulated to be actually eligible (36.48%), whereas 2,577 are simulated to be non-eligible (63.52%). The numbers are in accordance with Herber and Kalinowski (Citation2019)'s results who consider within their 2,827 formally eligible observations 41% to be actually eligible and 59% to be non-eligible. For 370 observations (9.12% out of all formally eligible individuals), students report to receive BAföG even though the simulation estimated them to be non-eligible. As a comparison, the ‘wrongly estimated’ cases (the beta-error) for Herber and Kalinowski (Citation2019) accounts to 6% of their entire student sample. The different beta-errors may result from the fact that the simulation in this paper uses the approach of Steiner and Wrohlich (Citation2012). As Herber and Kalinowski (Citation2019) further emphasize, beta-errors may arise from the fact that the BAföG variable used also includes scholarships in the GSOEP.Footnote9
Table 2. Simulation results.
Choice of variables
According to hypothesis 1 from the theoretical model, we expect that a student's non-aid income (own labor income, transfer from parents) has a positive effect on the probability to not apply for student aid despite eligibility. Hypothesis 2 suggests that this effect should increase with information frictions. We are also able to test for hypothesis 3, i.e. whether students with low income react more severely to changes in information than high income students in terms of their probability to reject BAföG.
We determine a student's non-aid income based on different income sources from the GSOEP survey, including self-reported student income from main and side jobs, orphan payments, transfers received outside the household and child allowance payments. We create the income variable starting with current gross labor income, replacing it with last month's labor income if the current one is missing. We add gross income from any side/part-time jobs last month and consider any orphan's pension payments, as well as transfers received from people outside the household. Unfortunately, the GSOEP does not contain transfers received by parents, although these are the largest source of income for students in Germany (Middendorff et al. Citation2017). In order to use as much information on income as possible and also include as many observations as possible, we add a child allowance (Kindergeld) variable. The child allowance variable can also be interpreted as proxy for the minimum amount of parental help to their children and hence, it reduces the measurement error to a certain extent for the income variable itself. Child allowance that is paid by the government depends on the year the questionnaire was conducted, the age of the student (because it is paid to students under the age of 25) and the number of siblings. Average amounts of Kindergeld are reported in the Appendix (Table A1). This procedure allows us to improve our variable for the income of students. However, this variable most likely entails some measurement error as it only includes several income sources that are reported in the GSOEP. In order to keep the sample size as large as possible, we assume that missing information does not cause sample selection problems.
Next, we consider both parents’ gross labor income. Conditional on the extent of information frictions, the student might overestimate the effect of increasing parental income on eligibility. We also include student age, parents’ education and migration status, and a student’s siblings who are currently enrolled in higher education and also receiving BAföG. They can serve as source of information when it comes to the existence of such an aid scheme, answer questions or assist in the application process.
In order to test hypotheses 4 and 5, we incorporate a variable including the attitude towards risk. In the personal questionnaires of the GSOEP in 2004, 2006 and 2008–2013, risk aversion is covered by the question: ‘Are you generally a person who is fully prepared to take risks or do you try to avoid taking risks?’ ranging from high (0) to low (10) risk aversion. Like Herber and Kalinowski (Citation2019), we use this variable as measure for risk aversion, but handle missing data in a different way. In order to reduce the number of missings, we assume that the personal willingness to take on risk does not significantly change over time. If a student has information on her risk attitude for more than one year, we replace missing values and existing ones by a mean score of all of her answers over time. We recode the variable to a binary one, where 0–5 stands for highly risk averse and 6–10 for individuals with low risk aversion.Footnote10
We add another variable from the GSOEP that shows whether a student's family is repaying any monthly debt amounts: ‘Aside from debts on loans for home and property ownership, are you currently paying back loans or interest on loans that you took out to make large purchases or other expenditures?’ An individual who is already familiar with debt should be more comfortable taking on debt, and thus is considered to be less debt-averse. According to Cadena and Keys (Citation2013), students who live at home choose to do so because they are debt-averse and try to restrict their consumption.Footnote11
Descriptive statistics
The sample, which only includes eligible individuals, consists of a total of 1,480 observations with 677 students who are observed in an unbalanced panel over 13 years and appear on average more than three times. About 72.49% of students who receive aid in one year also receive it the year after, while about 89.27% of students who do not receive aid continue to not receive it the year after. For descriptive purposes we will only consider a smaller sample consisting of observations (
students) that have no missings in any of the explanatory variables. The mean parental gross labor income equals 4,588 EUR per month, whereas mean student income equals reasonable 228 EUR per month (no BAföG included). Half of the cases display a rather risk-averse behavior with the remaining half being less risk-averse. Roughly 10% of the observations have siblings who were also receiving BAföG during the observation period. More than 2/3 of the cases are still living at home with at least one parent, and approximately 22% report that the household they live in is currently in debt .Footnote12
Table 3. Descriptive statistics.
Modeling the probability of rejection
We construct the dependent variable , which equals 1 if eligible students do not apply for BAföG and 0 otherwise. The probability not to apply for BAföG can be modeled by
(10)
(10)
The response probability takes on values strictly between zero and one, following the standard normal distribution function . The individual specific time invariant trait,
, is unobservable.
We begin with a basic pooled estimation, disregarding the fact that we have panel data. The total size of the pooled sample equals observations, where students are treated to be independent observations even though on average they appear multiple times in the sample. We cluster observations over personal id's and compute standard errors robust to serial correlation with the underlying pooled data available (Andreß, Golsch, and Schmidt Citation2013).
We continue with an estimation strategy that recognize the panel structure and deal with unobserved heterogeneity. For instance, a student's general motivation to study might determine her choice whether to apply for aid. Therefore, a student who is more motivated might be better informed and therefore, her individual probability of applying for BAföG is higher compared to c.p. less motivated students. Motivation is a personality trait that usually does not change significantly over time, such that it might serve as candidate for unobserved individual specific heterogeneity. However, motivation itself cannot be observed and it is captured in the error. Thus, the problem of serial correlation would arise. Only if there is no individual effect or the individual effect is the same for every observation, the parameters can be consistently estimated by pooled probit (Andreß, Golsch, and Schmidt Citation2013):
(11)
(11)
If assumption (10) does not hold, pooled estimates are inconsistent (Longhi and Nandi Citation2015). Hence, we address this issue with a CRE probit model that, in contrast to fixed effects, allows for some correlation between the unobserved and the explanatory variables by assuming that
(12)
(12) where unobserved heterogeneity is a linear function of the time averages of all time variant explanatory variables,
, with ξ representing the vector of parameters for these averages. The error
is assumed to be independent of all explanatory variables and follows a standard normal distribution representing the ‘pure random effect’. Note that only the effects of time-varying elements in
are estimated, so there should not be any time constant explanatory variables included into the model. If the model, however, contains a time-constant explanatory variable (e.g. risk aversion), it can still be included as an explanatory variable, subject to the condition that
is partially assumed to be uncorrelated with risk aversion. Furthermore, note that time dummies do not vary across observed units and they are omitted from
. Essentially, the mean of (time-variant) explanatory variables for each unit
is added as a control variable to the original equation, which allows us to estimate the effect of explanatory variables while holding time averages fixed.
Results
shows the results of both models with coefficients and average marginal effects (AME's). An increase of parental income by 1% is associated by a 34.4% increase of the probability not to apply for BAföG at a 0.1% significance level, as opposed to the results by Herber and Kalinowski (Citation2019), who find no relationship for this specific source. This result suggests that students whose parental income is c.p. higher expect (falsely) not to be eligible for BAföG and hence, refrain from applying. Thus, information frictions might play a role.
Table 4. Estimation results.
In line with Hypothesis 1, an increase in student income seems to be associated with a slightly but significantly (at ) higher rejection probability. More specifically, for an increase of student income by 100 EUR, the probability to reject BAföG is 2.5% higher if the student is simultaneously low risk-averse and has siblings who could provide information on actual eligibility for student aid. In order to determine the relationship between information, income and risk aversion, we add two-way and a three-way interaction variable that shows whether the student has any siblings receiving BAföG, a student's attitude towards risk as well as her personal income owned. A test for the three-way interaction term turns out significant at a 0.1% significance level. Solely interpreting the AME's of each variable on its own does not provide any important insights and is not really realistic. For instance, one might think that for students who do not have any siblings receiving BAföG, the probability not to take up BAföG increases by remarkable 30.5% (significant at 0.001-level).
However, we should be cautious with this interpretation, as the effect is only valid if the student has low aversion (base level for risk averse dummy) and an income equal to zero. Nevertheless, this is evidence in support of hypothesis 2. Similarly, the effect of the dummy variable depicting a student's risk aversion is only valid if the student has siblings who receive BAföG (base level for siblings dummy) and student income equals zero. For students with high risk aversion, the probability to reject BAföG increases by 12.2% at a significance level of 1% (hypothesis 4), again considering the aforementioned base categories.
Consequently, all two-way and the three-way interaction terms can be explained in the same manner. The crucial part for the interpretation is to obtain correct AME's of the three-way interaction term, which makes the interpretation more complex than its two-way counterpart. For the interpretation, we vary the level of income between 100 and 500 EUR and set the level of risk aversion once to high and once to low in order to get the AME's of the ‘no siblings variable’. The AME's are listed in . For students with low risk aversion, the absence of siblings who could provide additional information is associated with an only moderately higher rejection probability irrespective of their level of income (at any conventional significance level). Conversely, for highly risk-averse students with low income (100-300 EUR), P(NoBAföG) is significantly reduced (level) in the presence of information frictions as suggested by hypothesis 5. For instance, for a highly risk averse student with a monthly income of 300 EUR, the probability of non-application is 32.7% higher at a significance level of 1% if she has no siblings receiving BAföG, i.e. if less information on BAföG is available.
Table 5. AME's of having less information depending on the level of risk aversion.
depicts the probability of not applying for BAföG for different combinations of income, information and risk aversion. For both groups, the rejection probability when having no siblings receiving BAföG is higher than the rejection probability when they have siblings who receive BAföG (Hypothesis 2). However, this relationship is stronger for students with high risk aversion (Hypothesis 5). For instance, for more risk-averse students with an income level equal to 200 EUR, the probability to reject BAföG is predicted to be 33.8% (significant at a 1% level) as opposed to students who have no siblings receiving BAföG or no siblings at all, where the probability is predicted to be 76% (at -value = 0.0001).
Figure 3. Predicted probabilities of turning down BAföG depending on student income, information gap and risk aversion. Source: GSOEP data 2001–2013.
Notes: Depicted are the predicted probabilities of students rejecting BAföG depending on their level of income, risk averse behavior and information level of BAföG with 95% confidence intervals (CI's). The dummy variable of students not having any siblings receiving BAföG also contains students who do not have any siblings at all.
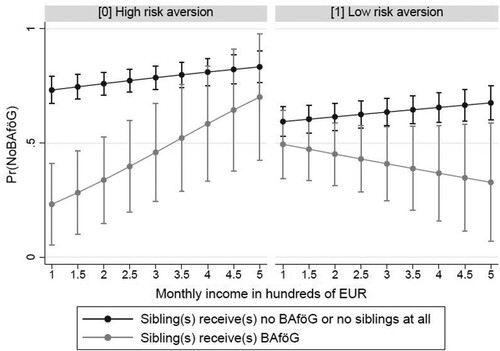
In line with hypothesis 4, the predicted probabilities curve for students with low risk aversion without siblings lies lower than the curve for highly risk averse students. Students with low risk aversion are expected to be less ‘worried’ and hence show smaller estimated probabilities for rejecting BAföG over all income levels. In line with Hypothesis 1, a rise in income is associated with an increase in the predicted rejection probability continuously in almost all cases. As an exception, the curve for students with low risk aversion and siblings receiving BAföG is downward sloping. We would have expected a flatter curve, and hence no response to different income levels. However, this result is based on a relatively small number of observations.
The results for the three-way interaction term seem to confirm the expected relationships from theory referring to information restrictions and risk aversion. In contrast to our estimation, Herber and Kalinowski (Citation2019) find no significance for parental income and degree, also attitude towards risks seems not to have a significant relationship with the Pr(NoBaföG). In line with our results, they find that the presence of a sibling is associated with a significantly lower probability of non-take up. Our model combines the information-income-risk dimension and finds a significant relationship on the Pr(NoBAföG).
If the student belongs to a household which pays back a credit on a monthly basis, the probability to reject BAföG is lower, supporting Hypothesis 6; however, the relationship is only significant at 10% significance level. Students living at home is associated with a 11.3% higher probability to reject BAföG (significant at 1% level). This is in line with the hypothesis that students living at home tend to be more cautious in their spending in order to prevent overspending/debt accumulation. Herber and Kalinowski (Citation2019) consider debt aversion with an interaction between impulsivity and impatience which is associated with a significantly higher probability of a non-take-up.
To allow for potential correlation of the explanatory variables with the composite error term, we also consider the CRE results in the second column of . There is evidence that individual specific traits play a role as . A likelihood ratio test, testing the non-existence of unobserved heterogeneity can be rejected at a 0.1% significance level. Regarding personal and regional variables, note that especially the size of the age-variable increases (8.8% instead of 3.3% for the pooled probit at a 0.1% significance level). In general, there are no remarkable differences in the estimated AMEs. Some of the AMEs become less significant and there is no change in the sign of the estimated results except for the variable ‘parental university degree’, which is however insignificant at any conventional significance level.
Sensitivity analysis and concerns
As a robustness check, we additionally simulate eligibility by not considering own student income as an additional restriction, which obviously gives rise to a larger student sample . Figure A1 in the appendix depicts the predicted Pr(NoBAföG) for the new simulation. The results show that especially for students with low risk aversion, the predicted probabilities for different income levels are smaller, and the curve flatter than the original graph in .
Herber and Kalinowski (Citation2019) use the aid amount as explanatory variable, which they find to be highly significant. As a robustness check, we include this additional variable into our model in Table A5 (first column). This does not significantly add explanatory power to predict the probability of not receiving BAföG. The coefficient for aid amount is small but still significant at a 1% significance level: An increase of the aid amount by 100 EUR is associated with a 3.1% lower probability to reject BAföG. With respect to the remaining variable estimates, the inclusion does not change the direction of the estimates and only affects slightly the significance levels. However, note that in contrast to Herber and Kalinowski (Citation2019), we here do not control for endogeneity of the benefit amounts.
In the second column, we analyze the same estimation equation with the dependent variable only considering the reception of BAföG (in contrast to the ‘combined’ variable that includes BAföG and scholarships), which is only available for 2007–2013, reducing our sample size to . This shortcoming already suggests that this version of the model might not provide reliable results. Comparing the estimated AME's to the pooled probit results in column 1 of does not provide significant differences to the main model.
Conclusion
Our simple theoretical model shows that it can be optimal for rational students to not apply for BAföG even though they would be eligible for a positive aid amount, including a free subsidy: Information frictions can deter students from filing for aid, while the effect is stronger for poorer and more risk-averse students. Moreover, debt aversion should reduce the take-up rate even further. With data from the GSOEP, we simulate the eligibility status of each student in the sample and only keep eligible individuals. We apply a pooled probit model and, to control for unobserved heterogeneity, a CRE probit model. Between both models, the main effects do not vary much. An increase of parental income by 1% is associated with a 34% increase of the probability not to apply for BAföG, suggesting that information frictions play a role in the take-up decision. The interaction of the three-way variable between a student's level of information (depending on siblings), the attitude towards risk and student income is highly significant at 0.1% for any of the models. Students who are more risk-averse seem to be more affected by information frictions. For them, having siblings who receive BAföG decreases their predicted probability to not apply for BAföG, particularly significantly at lower income levels. Students who are less risk-averse are less affected by information frictions. Moreover, students whose families are currently paying off any debt, which we consider to be less debt-averse, are to be more likely to apply for student aid, even though the relationship is just marginally significant.
Middendorff et al. (Citation2017) report that 37% of students from lower educational backgrounds state that making debt was the main reason for non-application. This suggests that debt-averse behavior is especially pronounced for students from lower educational backgrounds. Moreover, as Glocker (Citation2011) shows, the probability of finishing university successfully increases when students receive BAföG. A policy that aims to subsidize poor students, independent of their attitude towards risk, must take these effects into consideration. A clear communication about the application process, what the repayment scheme looks like, and also emphasizing that information centers exist can decrease information frictions and resolve misconception about eligibility. Even though our results show that a highly risk-averse attitude increases non-take up, they also show that it is possible to decrease this effect once more information is available. However, even if information among students would be perfect, some individuals might still dislike the idea of bearing debt so much that they refrain from applying because they know that they will pay back a part of the aid at some point in the future. One suggestion for these highly debt-averse students might be to let them decide between a loan-grant BAföG scheme and a grant-only BAföG scheme. As such, students with high debt aversion would accept the grant-only BAföG (obviously receiving only half of their actual aid entitlements) and thus, more students could be reached.
Supplemental Material
Download MS Word (30.6 KB)Data availability
Socio-Economic Panel. doi:10.5684/soep.v30
Disclosure statement
No potential conflict of interest was reported by the author(s).
Additional information
Funding
Notes
1 The non-take-up rate shows the percentage of people not applying for aid conditional on being eligible for aid.
2 The appendix is available as separate file.
3 These rather low income levels have no effect on the eligibility or amount of student aid.
4 We show later that we can assume lower costs once we allow for debt aversion.
5 GSOEP questionnaires and a more detailed discussion of all items is provided by the DIW at https://www.diw.de/en/diw_02.c.222729.en/questionnaires.html#671288.
6 For 2007-2013, about 11% of the observations give contradictory answers, which might give rise to a measurement error in the dependent variable.
7 A complex micro-simulation model is introduced by Herber and Kalinowski (Citation2019) with an observation period set from 2002-2013. The simulation by Steiner and Wrohlich (Citation2012) includes previous years from 2000–2006 and a more intuitive simulation model, which is not too complex and approximates student aid amounts. For instance, instead of considering siblings' income, siblings only increase parental income allowances.
8 In practice, formal eligibility requires further information, such as whether the student passes obligatory exams during studies or whether the nominal length of studies is adhered to. Unfortunately, the GSOEP does not contain any information on, e.g., the nominal length of a student's study program. Moreover, it is required that students are enrolled in their first-time study program. However, since we do not have any information on their previous degree level, we have to assume that they are enrolled in their first-time study program.
9 To test this hypothesis, we compute the beta-error by solely considering the ‘correct’ 2007–2013 BAföG variable of the GSOEP, which leads to a decrease of the beta-error to 5.03%.
10 Herber and Kalinowski (Citation2019) include risk attitudes into their analysis as well. However, we go a step further and analyze several interaction effects, as will be explained in the following.
11 Herber and Kalinowski (Citation2019) also include a variable that determines whether a student is living at home. They argue that students' needs are lower when living at home. This paper rather emphasizes the fact that they restrict themselves in their consumption when still living at home.
12 The Appendix provides some additional information on coding and also the level of measurement concerning explanatory variables.
References
- Andreß, H.-J., K. Golsch, and A. W. Schmidt. 2013. Applied Panel Data Analysis for Economic and Social Surveys. Vol. 1. Berlin: Springer-Verlag, 203–247.
- Bettinger, E. P., B. T. Long, P. Oreopoulos, and L. Sanbonmatsu. 2012. “The Role of Application Assistance and Information in College Decisions: Results from the H&R Block FAFSA Experiment.” The Quarterly Journal of Economics 127 (3): 1205–1242. doi:https://doi.org/10.1093/qje/qjs017.
- Booji, A. S., E. Leuven, and H. Oosterbeek. 2012. “The Role of Information in the Take-up of Student Loans.” Economics of Education Review 31 (1): 33–44. doi:https://doi.org/10.1016/j.econedurev.2011.08.009.
- Bruckmeier, K., J. Pauser, U. Walwei, and J. Wiemers. 2013. “Simulationsrechnungen zum Ausmaß der Nicht-Inanspruchnahme von Leistungen der Grundsicherung. Institut für Arbeitsmarkt- und Berufsforschung IABForschungsbericht No. 5/2013.”
- Bundeskanzleramt und Nationaler Normenontrollrat. 2010. “Einfacher zum Studierenden-BAföG.”
- Bundesministerium für Bildung und Forschung. 2017. “Darlehensrückzahlung - Rückzahlung von zinsfreien Staatsdarlehen nach dem Bundesausbildungsförderungsgesetz (BAföG).”
- Cadena, B. C., and B. J. Keys. 2013. “Can Self-Control Explain Avoiding Free Money? Evidence from Interest-Free Student Loans.” Review of Economics and Statistics 95 (4): 1117–1129. doi:https://doi.org/10.1162/REST_a_00321.
- Caetano, G. S., M. Palacios, and H. A. Patrinos. 2011. “Measuring Aversion to Debt: An Experiment Among Student Loan Candidates.” World Bank Policy Research Working Paper No. 5737.
- Cornwell, C., D. B. Mustard, and D. J. Sridhar. 2006. “The Enrollment Effects of Merit-Based Financial Aid: Evidence from Georgia's HOPE Program.” Journal of Labor Economics 24 (4): 761–786. doi:https://doi.org/10.1086/506485.
- Cunningham, A. F., and D. A. Santiago. 2008. Student Aversion to Borrowing: Who Borrows and Who Doesn't. Washington, DC: Institute for Higher Education Policy. https://files.eric.ed.gov/fulltext/ED503684.pdf.
- Deutscher Bundestag. 2014. “Zwanzigster Bericht nach §35 des Bundesausbildungsförderumgsgesetz zur Überprüfung der Bedarfssätze, Freibeträge sowie Vomhundertsätze und Höchstbeträge nach §21 Absatz 2.” Unterrichtung des Deutschen Bundestags.
- Deutscher Bundestag. 2017. “Einundzwanzigster Bericht nach §35 des Bundesausbildungsförderungsgesetzes zur Überprüfung der Bedarfssätze, Freibeträge sowie Vomhundertsätze und Höchstbeträge nach §21 Absatz 2.” Unterrichtung des Deutschen Bundestags.
- Deutscher Gewerkschaftsbund. 2016. “Alternativer BAföG-Bericht.”
- Deutsches Studentenwerk. 2017. “Geschichte und Statistik zum BAföG.”
- Dynarski, S. M., and J. E. Scott-Clayton. 2006. “The Cost of Complexity in Federal Student Aid: Lessons from Optimal Tax Theory and Behavioral Economics.” National Bureau of Economic Research (NBER) Working Paper No. 12227.
- Eckel, C., C. Johnson, C. Montmarquette, and C. Rojas. 2007. “Debt Aversion and the Demand for Loans for Postsecondary Education.” Public Finance Review 35 (2): 233–262. doi:https://doi.org/10.1177/1091142106292774.
- Epstein, L. G., and S. E. Zin. 1989. “Substitution, Risk Aversion, and the Temporal Behavior of Consumption and Asset Returns:a Theoretical Framework.” Econometrica 57 (4): 937–969. doi:https://doi.org/10.2307/1913778.
- Epstein, L. G., and S. E. Zin. 1991. “Substitution, Risk Aversion, and the Temporal Behavior of Consumption and Asset Returns: An Empirical Analysis.” Journal of Political Economy 99 (2): 263–286. doi:https://doi.org/10.1086/261750.
- Glocker, D. 2011. “The Effect of Student Aid on the Duration of Study.” Economics of Education Review 30 (1): 177–190. doi:https://doi.org/10.1016/j.econedurev.2010.08.005.
- Goebel, J., M. M. Grabka, S. Liebig, M. Kroh, D. Richter, C. Schröder, and J. Schupp. 2019. “The German Socio-Economic Panel Study (Soep).” Journal of Economics and Statistics 239 (2): 345–360. doi:https://doi.org/10.1515/jbnst-2018-0022.
- Herber, S. P., and M. Kalinowski. 2019. “Non-take-up of Student Financial Aid: A Microsimulation for Germany.” Education Economics 27 (1): 52–74. doi:https://doi.org/10.1080/09645292.2018.1490698.
- Heublein, U., J. Richter, R. Schmelzer, and D. Sommer. 2014. “Die Entwicklung der Studienabbruchquoten an den deutschen Hochschulen. Statistische Berechnungen auf der Basis des Absolventenjahrgangs 2012. Deutsches Zentrum für Hochschul- und Wissenschaftsforschung (DZHW).”
- John, E. P. S., and J. Noell. 1989. “The Effects of Student Financial Aid on Access to Higher Education: An Analysis of Progress with Special Consideration of Minority Enrollment.” Research in Higher Education 30 (6): 563–581. doi:https://doi.org/10.1007/BF00992391.
- Kayser, H., and J. R. Frick. 2000. “Take It or Leave It: (Non-) Take-up Behavior of Social Assistance in Germany.” Deutsches Institut für Wirtschaftsforschung (DIW) Discussion Paper No. 210.
- King, J. E. 2004. Missed Opportunities: Students Who Do Not Apply for Financial Aid. Washington, DC: American Council on Education Issue Brief.
- Kofoed, M. S. 2017. “To Apply or Not to Apply: FAFSA Completion.” Research in Higher Education 58: 1–39. doi:https://doi.org/10.1007/s11162-016-9418-y.
- Longhi, S., and A. Nandi. 2015. A Practical Guide to Using Panel Data. Vol. 1, 197–211. London: SAGE Publications Ltd.
- Middendorff, E., B. Apolinarski, J. Poskowsky, M. Kandulla, and N. Netz. 2017. “Die wirtschaftliche und soziale Lage der Studierenden in Deutschland 2016. Sozialerhebung des Deutschen Studentenwerks durchgeführt durch das HIS-Institut für Hochschulforschung.”
- Monge-Naranjo, A. 2016. “Student Loans Under the Risk of Youth Unemployment.” Federal Reserve Bank of St. Louis Review 98 (2): 129–158.
- OECD. 2016. “Education at a Glance 2016: OECD Indicators.”
- Ortiz-Nuñez, A. 2014. “Attitudes Toward Risk and Socioeconomic Factors Related To Educational Loans.” Contemporary Economic Policy 32 (4): 710–718. doi:https://doi.org/10.1111/coep.12040.
- Powell, J. J., and H. Solga. 2011. “Why Are Higher Education Participation Rates in Germany So Low? Institutional Barriers to Higher Education Expansion.” Journal of Education and Work 24 (1–2): 49–68. doi:https://doi.org/10.1080/13639080.2010.534445.
- Socio-Economic Panel. 2015. “Data for Years 1984-2013.” Version 30. doi:https://doi.org/10.5684/soep.v30.
- Steiner, V., and K. Wrohlich. 2012. “Financial Student Aid and Enrollment in Higher Education: New Evidence from Germany.” The Scandinavian Journal of Economics 114 (1): 124–147. doi:https://doi.org/10.1111/j.1467-9442.2011.01669.x.