Abstract
Prospective memory (PM) refers to remembering to perform an action in the future. One hundred and twenty-nine students completed a laboratory event–based PM task as well as depression and anxiety questionnaires. The data were analysed with the beta-MPT version of the multinomial processing tree model of event-based PM. Thereby, the prospective and retrospective components of PM were estimated for each participant and were then correlated with depression and anxiety. State anxiety was negatively correlated with the prospective component of PM. Neither depression nor trait anxiety were related to either component of PM.
We thank Pia Ewerdwalbesloh for assisting with the data collection and Jane Zagorski for providing comments on a prior version of the manuscript. This research was part of Nina R. Arnold's doctoral dissertation conducted under Ute J. Bayen's supervision.
Part of this research was presented at the Conference “Tagung experimentell arbeitender Psychologen” in Vienna, Austria (2013), and at the “4th International Conference on Prospective Memory”, in Naples, Italy (2014).
In a prospective memory (PM) task, we must remember to perform an action at an appropriate time in the future (e.g., Einstein & McDaniel, Citation1990). For event-based PM (e.g., McDaniel & Einstein, Citation2007), the appropriate time is defined by the occurrence of a specific event (e.g., taking medicine when chest pain occurs), whereas for time-based PM, the appropriate time is defined by a certain point in time (e.g., switching off the oven after 20 minutes). PM is very important in everyday life as PM failures may have serious consequences. For example, forgetting to take medicine may result in health impairments, and forgetting to turn off the oven may result in an inedible meal or—even worse—in a house fire.
In daily life, performing a PM action often interrupts an ongoing activity. For example, one may have to stop watching a movie to take medicine. The interruption of an ongoing activity has been incorporated into many laboratory studies of PM; that is, the PM task is usually embedded in an ongoing activity. For event-based PM tasks, the appropriate action must be carried out in response to specific target events that may appear at any time during an ongoing task. For example, participants may be asked to press a certain key on a computer keyboard when a PM target event appears while they are busily engaged in a short-term memory task (e.g., Einstein & McDaniel, Citation1990). For time-based PM tasks, participants must remember to initiate the appropriate action at a certain point in time while engaged in a different ongoing activity.
It is important to distinguish between two different components of PM, namely, the prospective component and the retrospective component (Einstein & McDaniel, Citation1990). The prospective component refers to remembering that one must do something. In a laboratory paradigm, this means remembering that there is an additional task. The retrospective component refers to remembering what action to perform and when to perform it. In a laboratory paradigm, the retrospective component may be remembering which of the events occurring during the ongoing task are PM target events.
Several researchers have pointed out the role of mental health in PM (e.g., Harris & Cumming, Citation2003; Kliegel & Jäger, Citation2006; Rude, Hertel, Jarrold, Covich, & Hedlund, Citation1999). As PM is very important for everyday functioning, it is important to know how emotions and affective disorders influence PM.
The purpose of our study was to investigate the relationship between event-based PM and mental health using an innovative data analytical technique that allowed us to disentangle the prospective and retrospective components of PM for each participant and to correlate them with measures of depression and anxiety.
PM AND DEPRESSION
Depression and event-based PM performance
Performance in event-based PM tasks is usually measured as the proportion of target events that participants respond to with the required PM action. So far, only a few studies have examined the influence of depression on event-based PM performance.
According to the resource allocation model of depression (Ellis & Ashbrook, Citation1988), depression limits the amount of resources that can be allocated to a task and, therefore, negatively affects task performance. In support of this view, studies have shown that clinical depression accompanies working-memory impairments (De Lissnyder et al., Citation2012; Joormann, Levens, & Gotlib, Citation2011; Rose & Ebmeier, Citation2006). Impairments in cognitive resources should particularly affect performance in resource-demanding self-initiated processes that are often required to perform PM tasks.
Livner, Berger, Karlsson, and Bäckman (Citation2008) examined a sample of older nonclinically depressed adults with a naturalistic PM task. Participants had to remind the experimenter to do something at the end of the experiment. Performance was not influenced by depressive symptoms. On a similar task, Cuttler and Graf (Citation2008) also did not detect a relationship between depressive symptoms and PM for patients with obsessive-compulsive disorder. Lee et al. (Citation2010) used a similar task with patients with bipolar disorder and healthy controls and found no difference in PM performance. Harris and Menzies (Citation1999) found no influence of (nonclinical) depression on event-based PM performance.
However, the PM tasks used in these studies had only a single PM target. Event-based PM tasks with multiple different targets require more cognitive resources than tasks with single targets (Einstein & McDaniel, Citation2010). Only two studies that examined the relationship between depression and event-based PM used multiple targets. Altgassen, Kliegel, and Martin (Citation2009) used tasks with four PM trials, each with a different PM target. They found that event-based PM performance was impaired in a clinically depressed group as compared with a control group for nonfocal PM tasks but not for focal PM tasks. Focality concerns with whether the PM task requires the same type of item processing as the ongoing task. For instance, if the ongoing task involves colour discrimination, then a specific colour would be a focal PM target. By contrast, a specific word would be a nonfocal task on such an ongoing colour-discrimination task. Nonfocal tasks have been shown to require more cognitive resources than focal tasks (e.g., Brewer, Knight, Marsh, & Unsworth, Citation2010; McDaniel & Einstein, Citation2000). Accordingly, a relationship between working memory and PM performance has been shown for nonfocal tasks only (e.g., Brewer et al., Citation2010; Rose, Rendell, McDaniel, Aberle, & Kliegel, Citation2010). Thus, the results obtained by Altgassen et al. (Citation2009) suggest that depression-related PM impairments are more likely when the task requires relatively high amounts of cognitive resources as is the case with nonfocal tasks and multiple targets. However, Kliegel and Jäger (Citation2006) also used a nonfocal PM task with multiple targets. They did not find a relationship between depression scores in a nonclinical group of participants and event-based PM performance.
Depression and the prospective component of PM
It has been suggested that a lack of cognitive resources affects the prospective component of PM in particular, especially on nonfocal tasks (e.g., Smith, Citation2003; Smith & Bayen, Citation2004, Citation2005). In fact, studies in which the prospective component was measured separately have shown that this component was positively related to working-memory span (Arnold, Bayen, & Smith, Citationin press; Smith & Bayen, Citation2005; Smith, Persyn, & Butler, Citation2011). Hence, if the PM task requires high levels of self-initiated processing (as is expected on working-memory–demanding nonfocal tasks), we should find a negative relationship between the prospective component and depression.
Other tasks that demand high levels of self-initiation are time-based PM tasks, as they always require self-initiated time monitoring (e.g., through clock checking). In accordance with the resource allocation model (Ellis & Ashbrook, Citation1988), depression scores have been found to negatively affect time-based PM tasks (Kliegel & Jäger, Citation2006; Lee et al., Citation2010; Rude et al., Citation1999). In fact, depression-related deficits are more evident on time-based PM tasks, which always require self-initiated processes, than on event-based PM tasks, which require self-initiated processes to varying degrees depending on task characteristics. This finding supports the idea that difficulties with self-initiation might be the reason for PM impairments in depression (Einstein, McDaniel, Richardson, Guynn, & Cunfer, Citation1995).
Depression and retrospective memory
The retrospective component of PM tasks may also contribute to depression-related differences in task performance. In accordance with the resource allocation model, disruptive negative emotions, such as depressed mood, have been found to negatively affect retrospective memory (Ellis & Ashbrook, Citation1988). This was also found in studies on clinical depression (e.g., Potts, Camp, & Coyne, Citation1989). Participants with clinical depression frequently showed deficits in recognition memory (Brand, Jolles, & Gispen-de Wied, Citation1992; Hertel & Milan, Citation1994; Ramponi, Murphy, Calder, & Barnard, Citation2010; Watts, Morris, & MacLeod, Citation1987).
There are two studies that examined both PM performance and retrospective memory with separate tests. Livner et al. (Citation2008) found a negative relationship between depressive symptoms and both free and cued recall. Harris and Menzies (Citation1999) found no relationship of nonclinical depression with recall. As most studies have shown a negative relationship between depression and retrospective memory including recognition, we assume that depression affects the retrospective component of PM that involves recognising target events.
If depression negatively affects retrospective memory, then it is possible that the effects of depression on PM performance might be due to differences in the retrospective component alone. Therefore, to test the hypothesis that depression affects a resource-demanding prospective component, it would be essential to measure the two components separately. None of the prior studies on depression and PM performance have disentangled the two underlying components. Thus, the results of these studies cannot be ascribed to either component.
PM AND ANXIETY
Anxiety is a construct closely related to depression (Last, Strauss, & Francis, Citation1987; Overall, Citation1962), and therefore, both are often included in the same study. Trait anxiety refers to a person's basic level of anxiety, whereas state anxiety usually refers to the anxiety that a person is currently experiencing.
Anxiety and event-based PM performance
Trait anxiety
The results regarding the relationships between event-based PM and both trait and state anxiety have been mixed. Harris and Menzies (Citation1999) as well as Kliegel and Jäger (Citation2006) found a significant negative relationship between trait anxiety and performance on nonfocal event-based PM tasks with nonclinical samples. However, Cuttler and Graf (Citation2008) did not find a relationship between trait anxiety and event-based PM with a naturalistic nonfocal PM task involving one target only. Harris and Cumming (Citation2003) did not find a relationship between trait anxiety and a focal event–based PM task.
State anxiety
Harris and Cumming (Citation2003) found that participants with higher levels of state anxiety showed poorer performance than participants with lower levels of state anxiety in a focal event–based PM task. By contrast, Cuttler and Graf (Citation2008) did not find a relationship between state anxiety and event-based (nonfocal) PM. Cockburn and Smith (Citation1994) stated that the relationship between state anxiety and a naturalistic event–based nonfocal PM task resembled a complex curvilinear function.
Anxiety and the prospective component of PM
Similar to depression, trait anxiety impairs working memory (Eysenck, Citation1985; MacLeod & Donnellan, Citation1993). Stout and Rokke (Citation2010) also found a link between working memory and anxiety. Highly anxious participants were found to have lower working-memory capacity than participants with low levels of anxiety (Darke, Citation1988). Therefore, we expected that higher levels of trait and state anxiety would accompany a lower prospective component of PM.
Anxiety and retrospective memory
Trait anxiety and retrospective memory
De Pascalis and Morelli (Citation1990) found that trait anxiety did not affect recognition memory. Beato, Pulido, Pinho, and Gozalo (Citation2013) found that trait anxiety did not affect correctly and falsely recognised words in the Deese-Roediger-McDermott (DRM) paradigm in both a correlational analysis and an extreme-groups analysis. Mathews and MacLeod (Citation1985) compared patients who were treated for an anxiety disorder and a matched control group. The groups differed significantly in trait anxiety but not in recognition memory for both threat-related and neutral words.
State anxiety and retrospective memory
Eysenck, Derakshan, Santos, and Calvo (Citation2007) proposed that state anxiety may not impair recognition memory because it may lead to the use of compensatory strategies. Beato et al. (Citation2013) found no effect on correctly and falsely recognised words in the DRM paradigm in both a correlational analysis and an extreme-groups analysis. The groups in the study by Mathews and MacLeod (Citation1985) also differed significantly in state anxiety but not in recognition memory for threat-related and neutral words.
However, the prospective and retrospective components of PM were not disentangled in any of the cited studies. In some of the studies, both a PM task and a retrospective memory task were administered, but the PM task was still a conglomerate of both components. Separating the two components not only important for theoretical reasons but also crucial for interventions. If retrospective memory is impaired, people might write lists, learn mnemonic techniques, or use other tools to remember target events. If, however, the prospective component of PM is impaired, people need different types of interventions such as reminders or special routines to remember to implement their everyday PM tasks.
THE MULTINOMIAL PROCESSING TREE MODEL OF EVENT-BASED PM
Smith and Bayen (Citation2004) developed a multinomial processing tree (MPT) model of event-based PM. This is a stochastic model that allows the prospective and retrospective components of PM to be measured separately. This model can be used to determine how different variables affect each of the two components and how this contributes to PM performance. In our study, we applied the beta-MPT version of this model (Smith & Batchelder, Citation2010) to yield individual parameter estimates that we then compared to measures of anxiety and depression. Thus, we examined the effects of mental health on the retrospective and prospective components of PM separately.
MPT models are useful tools when attempting to unravel latent cognitive processes (Batchelder & Riefer, Citation1999) and have been applied in various domains of cognitive psychology (see Erdfelder et al., Citation2009, for a review). Smith and Bayen (Citation2004) developed an MPT model that disentangles the retrospective and prospective components of PM. It has been applied frequently (e.g., Pavawalla, Schmitter-Edgecombe, & Smith, Citation2012; Schnitzspahn, Horn, Bayen, & Kliegel, Citation2012; Smith & Bayen, Citation2005, Citation2006; Smith, Bayen, & Martin, Citation2010; Smith et al., Citation2011) and validated thoroughly (Horn, Bayen, Smith, & Boywitt, Citation2011; Smith & Bayen, Citation2004).
The model was developed for ongoing tasks with two response options and an embedded PM task. We will explain the model using the example of the ongoing colour-matching task that was used in a number of prior studies (e.g., Horn et al., Citation2011; Smith & Bayen, Citation2004, Citation2006; Smith et al., Citation2010) as well as in the current study. Participants are shown four coloured rectangles followed by a coloured word. The ongoing task is to decide whether the colour of the word matches the colour of one of the preceding rectangles. The embedded PM task is to press a special key when specific words appear. There are four possible trial types in this task: (1) a PM target appears, and the colours match (target, match); (2) a PM target appears, but the colours do not match (target, nonmatch); (3) no PM target appears, but the colours match (nontarget, match); and (4) no PM target occurs, and the colours do not match (nontarget, nonmatch).
The model () includes one tree for each trial type in which any of the three responses (“match”, “nonmatch” and “PM target”) can occur. The first tree refers to PM targets occurring in trials in which the colours match. Parameter C1 denotes the probability that a colour match is detected. The complementary probability of 1 − C1 is the probability that a colour match goes undetected. P is the probability that the participant remembers that there was an additional PM task (the prospective component). Thus, 1 − P is the probability that the participant does not remember the PM task. Detecting a colour match but not remembering the PM task leads to a “Match” response. If the participant detects the colour match and remembers the PM task, M1 is the probability that the PM target is recognised, leading to a “PM” response. If the target is not recognised (with a probability of 1 − M1), the participant must guess whether a target is present. With probability g, the participant guesses that there is a target, leading to a “PM” response, whereas with probability 1 − g, the participant guesses that there is no target, leading to a “Match” response.
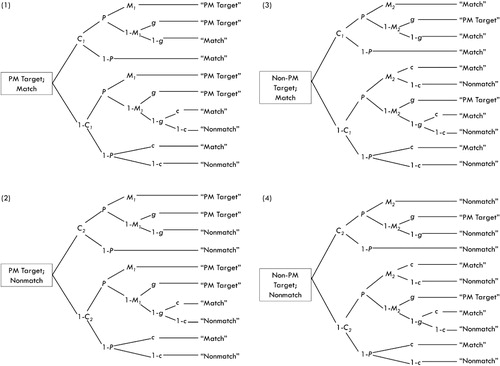
The lower part of the first tree illustrates trials in which the colour match was not detected (with a probability of 1 − C1). Nevertheless, the participant may remember the PM task (with probability P) or may not remember the PM task (with probability 1 − P). If the PM task is remembered, a target is recognised with probability M1. If the PM target is not recognised (1 − M1), guessing leads to a “PM” response with probability g. 1 − g is the probability of guessing that no PM target is present. Because a colour match was initially not detected (with probability 1 − C1), the participant must guess whether the colours in the trial matched (guessing probability c) or not (1 − c), leading to a “Match” or a “Nonmatch” response, respectively. Similarly, if the PM task was not remembered (with probability 1 − P), participants must guess whether there was a colour match (c) or not (1 − c).
The second tree refers to PM targets occurring in trials in which the colours do not match. It is similar to the first tree. The main difference is that C1 is replaced by C2, which denotes the probability that a participant detects that the colours do not match. As a result, the upper half of the tree is the same with the only difference being that remembering the PM task but not recognising the PM target can lead to a “Nonmatch” response. Additionally, not remembering the PM task results in a “Nonmatch” response. The lower half of the second tree is exactly the same as the lower half of the first tree.
The third tree, for trials in which the colours match but without a PM target, is also similar to the first tree with the exception that if participants remember the PM task, M2 is the probability of recognising that a word is not a PM target, resulting in a “Match” response. If participants fail to recognise that the word is not a PM target (1 − M2), they must guess. Guessing, again, can lead to a “PM” response, with probability g, or to a “Match” response, with probability 1 − g.
The fourth tree refers to trials in which the colours do not match but without a PM target. It is almost identical to the third tree. The only difference is that C1 is replaced by C2, that is, the probability of detecting that the colours do not match. Therefore, the responses in the upper half of the fourth tree are different from the responses in the upper half of the third tree. That is, instead of leading to “Match” responses, the upper half of the fourth tree leads to “Nonmatch” responses. Again, the lower half of the fourth tree is exactly the same as the lower half of the third tree.
As described in detail in Smith and Bayen (Citation2004), the model is identifiable only after posing some theoretically motivated constraints on the model parameters. The guessing parameter c is set to the probability of a colour match occurring during the task. Similarly, the guessing parameter g is set to the probability of a PM target occurring during the ongoing task. M1 = M2 reflects the assumption that recognising a PM target and recognising that an item is not a PM target are equally likely. All in all, these constraints limit the number of free parameters in the MPT model to four: P, M, C1 and C2.
BETA-MPT MODELS
In traditional MPT modelling, all participants and all items are assumed to be homogeneous, that is, to have the same parameter values (Smith & Batchelder, Citation2008). If this assumption does not hold, parameter estimates may be biased (Klauer, Citation2006, Citation2010; Smith & Batchelder, Citation2008, Citation2010). In addition, the traditional approach yields only groupwise parameter estimates. Smith and Batchelder (Citation2010) introduced beta-MPT models, which can be used to yield individual parameter estimates and thus to investigate individual differences. Beta-MPT models use a hierarchical modelling approach. These approaches specify parameters at two levels. At the group level, the group parameters—comparable to the parameter estimates in traditional MPT models—are specified. At the population level, the model is specified by hyperparameters that describe the variability in participants. Beta-MPT models assume that each parameter is independently beta distributed. Beta distributions are parameterised by two different variables, namely α and β, which can range between 0 and ∞. The resulting range of parameter values lies between 0 and 1, thus meeting the requirements for probabilities. This provides us with individual model parameter estimates and thus allows us to run correlations with other variables. Smith and Batchelder (Citation2010) described a method for using beta distributions with the pair-clustering MPT model (Batchelder & Riefer, Citation1980, Citation1986), and Arnold, Bayen, Kuhlmann, and Vaterrodt (Citation2013) applied it to the MPT model of source monitoring (Bayen, Murnane, & Erdfelder, Citation1996). We customised this method for the MPT model of event-based PM. The method uses Markov Chain Monte Carlo (MCMC) methods that can be applied using the WinBUGS software (Lunn, Thomas, Best, & Spiegelhalter, Citation2000). Thereby, we obtained individual parameter estimates as well as estimates for the hyperparameters α and β, including uncertainty estimates.
AIM OF THE STUDY AND HYPOTHESES
None of the studies on PM and mental health cited above used an MPT model. Therefore, it would be difficult to ascribe the results to the prospective or retrospective components of PM or to determine how anxiety or depression influences the contributions of the underlying processes to PM performance. In the current study, we investigated whether depression, state anxiety and trait anxiety influence PM performance. If depression or anxiety influences PM performance, it would be of great interest to determine whether this effect is due to differences in the prospective component or the retrospective component of PM. To compute correlations between the participants' model parameters and their scores on different tests of depression and anxiety, we applied the beta-MPT version of the MPT model by Smith and Bayen (Citation2004).
We used an ongoing colour-matching task with a nonfocal PM task. The ongoing task focused on the colours of rectangles and words, whereas the PM task focused on the words themselves and was thus nonfocal with respect to the ongoing task. We used this nonfocal PM task with multiple targets because such a task is very resource demanding and thus allowed us to test the hypothesis that depression-related resource deficits would negatively affect the prospective component as measured by model parameter P. The M parameter of the MPT model denotes the probability with which participants will recognise PM targets. As reviewed above, depression has been shown to affect recognition memory. Thus, we expected estimates of the M parameter to decrease as levels of depression increased. As reviewed earlier, most studies have shown a negative relationship between PM performance and anxiety. As we explained above, the prospective component P is related to working-memory capacity on nonfocal tasks with multiple targets. Similar to depression, trait anxiety (Eysenck, Citation1985; MacLeod & Donnellan, Citation1993) and state anxiety (Darke, Citation1988) have been found to impair working memory. Therefore, both state anxiety and trait anxiety were predicted to negatively influence the prospective component P. As outlined above, neither state (Eysenck et al., Citation2007) nor trait anxiety (De Pascalis & Morelli, Citation1990) affects recognition memory. Therefore, we did not predict a correlation between the retrospective component M and anxiety. As anxiety is assumed to have a negative impact on the estimate of parameter P, it was also expected to negatively influence PM performance.
METHODS
Participants
Participants were 129 students at the University of Düsseldorf. Seventy-nine of them were female; their ages ranged from 18 to 52 years (M = 22.31, SD = 5.48).Footnote1 All of them were native German speakers, and none of them suffered from achromatopsia. They received either course credit or € 10 for their participation. One additional participant never gave a “PM” response, thus, presumably did not understand the instructions and was, therefore, excluded from all analyses. The resulting statistical power to detect a medium effect of r = .30 with alpha = .05 was .97.
Measures and materials
The Beck Depression Inventory II
We used the Beck Depression Inventory II (BDI-II; Beck, Steer, & Brown, Citation1996; translated into German by Hautzinger, Keller, & Kühner, Citation2006) to measure depression. The BDI-II has a high retest reliability, ranging from rα = .74 to rα = .96 and is strongly correlated with other depression scales, r = .68 to r = .89 (Hautzinger et al., Citation2006). For 21 items, participants select the statement that applies most to them. Each of the statements is designated a value between 0 and 3. The BDI-II is scored by simply adding the values of the statements. Thus, the total scores awarded on the BDI-II can range from 0 to 63, with higher scores indicating stronger depression.
Hospital Anxiety and Depression Scale
The German version of the Hospital Anxiety Depression Scale (HADS-D; Herrmann, Buss, & Snaith, Citation1995) was adapted from the original version developed by Zigmond and Snaith (Citation1983). This self-report scale is composed of two subscales, one measuring depression, and the other one measuring anxiety. Each subscale consists of seven items with four different response alternatives. Every response alternative is assigned a value ranging from 0 to 3. The values are added separately for each of the subscales, thus leading to total scores on the subscales that range between 0 and 21, with higher scores indicating more severe depression or anxiety. The HADS-D has a satisfactory validity and its retest reliability is r = .71 (Herrmann et al., Citation1995).
State-Trait Anxiety Inventory
The State-Trait Anxiety Inventory (STAI) was developed by Spielberger, Gorsuch, and Lushene (Citation1970) and translated into German by Laux, Glanzmann, Schaffner, and Spielberger (Citation1981). The STAI assesses levels of state anxiety and trait anxiety. It contains two subscales with 20 items each, which are rated on a 4-point Likert scale. The state anxiety subscale asks participants to indicate their momentary feelings by rating the intensity of the anxiety they are experiencing. The trait anxiety subscale is aimed at determining a person's level of general anxiety by collecting information about how the person feels in general. The STAI is evaluated by adding the values of each response alternative ranging from 1 to 4 separately for each subscale. Higher scores indicate higher levels of anxiety. The STAI has satisfactory reliability and validity (Laux et al., Citation1981).
Word items for the PM task
We selected 168 words from the CELEX database (Baayen, Piepenbrock, & Gulikers, Citation1995). The words were between five and eight letters long with two or three syllables and a frequency between 16 and 40 per million. Emotional valence varied between −1 and 3; arousal varied between 1.3 and 4.1. From these 168 words, we chose 15 to serve as PM targets, leaving 153 words to serve as distractor items. The target and distractor items were matched in length, emotional valence and frequency. The targets and distractor words were the same for each participant.
Procedure
The ongoing task with the embedded PM task was computer based. Participants were tested in groups of up to six in separate computer booths. Computerised instructions informed them that the study was aimed at investigating colour-matching abilities, followed by the instructions for the colour-matching task. The assignments of the “M” and “V” keys to the answers match and nonmatch were counterbalanced. Both speed and accuracy were emphasised. After reading the instructions, participants were given 10 trials of the colour-matching task for practice. For this task, we selected five different colours: yellow, red, blue, green, and white. The colours were shown as rectangles that were 166 × 120 pixels in size. One trial consisted of four coloured rectangles presented in the middle of a black screen for 500 ms each, followed by a blank screen for 250 ms before the next rectangle was presented. After the fourth rectangle, a coloured word appeared in the middle of the screen in a 24-point font size. The participants judged whether the colour of the word matched one of the colours of the previously presented rectangles (colour-match vs. colour-nonmatch). The word stayed on the screen until the participants pressed a key. The first rectangle in the next trial appeared after a blank screen that lasted for 250 ms.
After the practice trials, the PM task was introduced. Participants were told that they would see five words that they should memorise. Participants were instructed to press the space bar instead of the match or nonmatch keys if one of these words appeared during the colour-matching task. The five PM targets were then consecutively presented on the screen for 5 s each. After that, a 2-minute retention interval followed during which participants filled out a demographics questionnaire.
The ongoing task with the embedded PM task consisted of three blocks with 112 trials each. We divided the 168 words into three sets comparable in frequency, emotional valence and arousal with every set appearing in one of three blocks of the PM task. The order of the sets was counterbalanced across participants. Each set consisted of five PM targets and 51 distractor words. After the first half of each block, participants took a break of 60 s before the second half started. It contained the same words as the first half and was also followed by a 60-s break. Following this break, five new PM targets were presented, and the participants were asked to memorise them. Again, presentation of the PM targets was followed by a 2-minute break, designated to filling out the demographics questionnaire. Then the second block of the PM task began. After completing the second half of this block, the new PM targets for the third block were presented. Again, the presentation of the PM targets was followed by a 2-minute break during which participants answered questions on the demographics questionnaire. Following the last block, participants were asked some questions concerning the PM task; namely, whether they perceived speed or accuracy as more important during the task, whether they remembered which key they were supposed to press upon encountering a PM target whether they remembered to press the space bar at all during the experiment, and whether they applied a certain strategy for memorising the PM targets. Then the 15 PM targets and the same number of distractor words were presented one after the other in a random order, and participants were asked to indicate whether each of the presented words was a PM target. This recognition-memory test was self-paced.
After the PM task, participants completed several questionnaires that we administered in a paper-and-pencil format. These questionnaires included, in order, a questionnaire on caffeine consumption, the short version of a questionnaire investigating achievement motivation (Leistungsmotivationsinventar-kurz; Schuler & Prochaska, Citation2001), and German versions of the following tests: Fagerström Test for Nicotine Dependence (Bleich, Havemann-Reinecke, & Kornhuber, Citation2002), the Epworth Sleepiness Scale (Bloch, Schoch, Zhang, & Russi, Citation1999), the Karolinska Sleepiness Scale (Åkerstedt & Gillberg, Citation1990), the Prospective and Retrospective Memory Questionnaire (Crawford, Henry, Ward, & Blake, Citation2006; German translation by Kaschel, Citation2002), the HADS-D (Herrmann et al., Citation1995), the BDI-II (Hautzinger et al., Citation2006), the STAI (Laux et al., Citation1981) and a translation of the passionate love scale (Hatfield & Sprecher, Citation1986). For the present study, we will report the results of the BDI-II, HADS-D and STAI only. Finally, the participants were debriefed and paid.
RESULTS
PM task and ongoing task
On average, participants pressed the PM key on M = 20.91 (SD = 6.38) PM trials; hence, 69.69% of the PM targets were correctly responded to (SD = 0.21). For the ongoing task, participants answered M = 274.45 trials (SD = 23.44) correctly; that is, 89.69% of the trials (SD = 0.08). If participants corrected themselves (i.e., they pressed a key even though they had already submitted an answer), we used the second corrected answer instead of the first one. However, using only the first answer did not change the pattern of the results.
We calculated MPT model parameters by adapting the version of the MCMC algorithm of Smith and Batchelder (Citation2010) to the MPT model of event-based PM.Footnote2 The algorithm was run with the computer programme WinBUGS 14 (Lunn et al., Citation2000) with 1,000,000 iterations. The first half of these iterations was removed as a burn-in period. Convergence was assessed using the potential scale reduction factor provided by the R2WinBUGS package (Sturtz, Ligges, & Gelman, Citation2005). For all parameters,
< 1.05, indicating good convergence. The group parameter estimates are presented in .
TABLE 1 Parameters of the hierarchical beta distributions
Depression and anxiety
Descriptive statistics for the BDI-II, HADS-D and STAI are listed in . One participant's BDI-II data were not included in the analysis because he failed to fill out the second page. The mean BDI-II score of 9.64 indicates that the sample was minimally depressed (Hautzinger et al., Citation2006). Only one participant scored higher than 29 on the BDI-II, indicating severe depression. However, nine participants produced scores between 20 and 28, indicating moderate depression. On the depression subscale of the HADS-D, five participants produced scores equal to or above the cut-off value of 9 for clinical depression.
TABLE 2 Scores on the BDI-II, HADS-D and STAI
On the anxiety subscale of the HADS-D, the scores of 19 participants equalled or exceeded the cut-off value of 11 for clinical anxiety. The STAI does not provide cut-off values.
Anxiety measures were correlated with each other, r > .53, p < .001. Similarly, depression measures were correlated, r = .67, p < .001. Depression and anxiety were also correlated, r > .54, p < .001.
PM and depression
We calculated correlations between individual MPT model parameter estimates and depression scores (see ). For all analyses, we set α to .05. The BDI-II scores were not significantly correlated with the prospective component P or the retrospective component M. Similarly, the depression scale of the HADS-D did not produce significant results when correlated with Parameter P and Parameter M. Additionally, neither depression score was significantly correlated with the number of PM hits (BDI: r = −.03, p = .358; HADS: r = −.03, p = .367).Footnote3
TABLE 3 Correlations between parameters M and P of the beta-MPT model, PM performance and depression and anxiety scores
PM and anxiety
Means and standard deviations for the anxiety measures are listed in ; coefficients for correlations between anxiety and PM are presented in . The HADS anxiety subscale was not significantly related to the number of PM hits, the prospective component P or the retrospective component M (all ps > .05). However, excluding those 19 participants who exceeded the cut-off value of the HADS anxiety subscale led to a negative correlation between both PM performance and the prospective component P with the anxiety scores (PM hits: r =−.20, p = .02; P: r =−.17, p = .04).
The state-anxiety subscale of the STAI was significantly correlated with PM performance as measured by the number of PM hits. That is, participants with lower state anxiety showed better PM performance. The beta-MPT parameter estimates showed that this difference was due to the negative correlation between state anxiety and the prospective component P. There was no significant correlation with the retrospective component M. Finally, the trait subscale of the STAI was not significantly correlated with any of the parameter estimates or with the number of PM hits (all ps > .05).
DISCUSSION
The main purpose of our study was to examine the effects of anxiety and depression for the different components of PM separately, namely, the prospective and retrospective components. We found a negative correlation between state anxiety and the prospective component of PM. State anxiety was not correlated with the retrospective component of PM. Neither depression nor trait anxiety were significantly correlated with either component of PM.
Most of the previous studies examining the role of mental health in PM used the traditional measure of PM performance (i.e., PM hits). This is the first study to investigate how the different underlying components of PM are influenced by depression and anxiety. By applying the new beta-MPT approach combined with the MPT model of event-based PM, we were able to look at individual differences in depression, anxiety and different components of PM simultaneously.
The results concerning the relationship between depression and PM were not consistent with our hypotheses. Like most of the previous studies, we did not find a relationship between depression and PM performance. Depression was not related to the Parameters P and M, nor to PM hits. This is in line with the results obtained by Cuttler and Graf (Citation2008) and Harris and Menzies (Citation1999), although we included more observations of PM performance. Our task was an event-based PM task. Depression has been shown to influence time-based PM (e.g., Kliegel & Jäger, Citation2006), but event-based PM only with a nonfocal task and multiple targets (Altgassen et al., Citation2009). Because the negative relationship between depression and time-based PM is assumed to be due to a lack of self-initiation (Kliegel et al., Citation2005; Rude et al., Citation1999), performance on nonfocal event-based PM tasks (which also require self-initiated processes) may be more vulnerable to effects of depression than focal tasks (cf. McDaniel & Einstein, Citation2000). Furthermore, because working memory is related to the P parameter on nonfocal tasks, and the M parameter measures recognition memory, we predicted that deficits in working memory and recognition memory, as found in depressed individuals, might lead to a decrease in the corresponding parameter estimates on our nonfocal event-based task. The resource allocation model (Ellis & Ashbrook, Citation1988) also predicted a negative relation between depression and retrospective memory. However, the model may not be applicable here because likely the retrospective recognition component with only few target items did not require a lot of resources, but heavily relied on automatic familiarity processes.
As these hypotheses were not confirmed, we may draw several conclusions. First, it is possible that depression-related deficits occur only in time-based PM as opposed to event-based PM. As Einstein and McDaniel (Citation1990) argued, time-based PM requires more self-initiation than any type of event-based PM, and this difference may account for the absence of a depression-related impairment in Parameter P and in PM performance in the present study.
Second, sample characteristics should be considered. As we used a normal student sample, it is possible that levels of depression were not high enough to produce PM impairments. Event-based PM may be impaired in clinically depressed individuals, but not in nonclinical samples. Supporting this idea, only Altgassen et al. (Citation2009) who used a clinically depressed sample found group differences in nonfocal event-based PM compared with normal controls. However, the MPT model requires a large number of observations to produce reliable parameter estimates. This can be achieved by maximising the number of participants or by maximising the number of observations per participant. We collected data from a nonclinical student sample to yield a high number of participants and to be able to conduct an experiment with many trials that lasted about one hour and is probably too cognitively demanding for a clinical sample.
In interpreting the results of our study, we should also consider that the retrospective component M was quite high at .89. Some participants reached the ceiling, and thus a restriction of range might account for the lack of significant correlations between this parameter and the depression scores. Replication of our study with samples including a larger range of both depression scores and cognitive abilities would be desirable.
Our hypothesis regarding a negative influence of anxiety on PM was confirmed in part. State anxiety as measured with the STAI was negatively correlated with the prospective component P and with PM performance. This result was as predicted because the prospective component P is related to working-memory capacity (Arnold et al., Citationin press; Smith & Bayen, Citation2005; Smith et al., Citation2011), and state anxiety has been found to impair working memory (Darke, Citation1988). However, neither trait anxiety as measured with the STAI nor the anxiety subscale of the HADS-D showed a relationship with PM performance or the prospective component P. This is problematic for the working-memory explanation, because trait anxiety has been found to impair working memory, too.
Excluding those participants who exceeded the cut-off value of the HADS anxiety subscale lead to a negative correlation between PM performance and the prospective component P with the anxiety scores. We may therefore assume that the absence of a correlation was due to outliers in the HADS anxiety subscale.
As hypothesised, none of the anxiety measures were significantly correlated with the retrospective component M. As a conclusion for everyday life, the results suggest that if someone is anxious for some reason, it is important to set reminders for PM tasks. For example, before an upcoming test or job talk, one may consider setting an alarm for taking medicine.
The results obtained for anxiety emphasise the importance of differentiating between state anxiety and trait anxiety. Only state anxiety was correlated with the prospective component and with PM performance. State anxiety scores are closely linked to the situation in which a test is taken and thus, situational influences on PM seem to be quite important. Trait anxiety, on the other hand, which is not specific to the situation but is more robust and long-lasting, left PM unaffected. The same holds for measures of depression; the HADS-D and BDI-II are both questionnaires that refer to time periods of several weeks and may therefore be less related to PM.
Working-memory deficits that are related to state anxiety (Stout & Rokke, Citation2010) may provide an explanation for the negative correlation between state anxiety and Parameter P. However, trait anxiety did not impair PM, although working-memory deficits are also assumed to exist in trait anxiety (Eysenck, Citation1985; MacLeod & Donnellan, Citation1993). The present study is the first to apply the MPT model of event-based PM to examine depression and anxiety in relation to PM. We found that state anxiety was correlated only with the prospective component but not the retrospective memory component, thus demonstrating the usefulness of distinguishing between these components when measuring PM.
Notes
1 Excluding four outliers (ages 43, 47, 50 and 52 years) regarding age (remaining age range: 18–31 years) did not change the pattern of results.
2 We report medians of the MCMC chains for the parameter estimations, because they are less sensitive to outliers than means.
3 When we excluded participants who exceeded the cut-off values, the pattern of results did not change.
REFERENCES
- Åkerstedt, T., & Gillberg, M. (1990). Subjective and objective sleepiness in the active individual. International Journal of Neuroscience, 52, 29–37.
- Altgassen, M., Kliegel, M., & Martin, M. (2009). Event-based prospective memory in depression: The impact of cue focality. Cognition and Emotion, 23, 1041–1055.
- Arnold, N. R., Bayen, U. J., Kuhlmann, B. G., & Vaterrodt, B. (2013). Hierarchical modeling of contingency-based source monitoring: A test of the probability-matching account. Psychonomic Bulletin & Review, 20, 326–333.
- Arnold, N. R., Bayen, U. J., & Smith, R. E. (in press). Hierarchical multinomial modeling approaches: An application to prospective memory and working memory. Experimental Psychology.
- Baayen, R. H., Piepenbrock, R., & Gulikers, L. (1995). The CELEX lexical database (CDROM). Philadelphia, PA: Linguistic Data Consortium, University of Pennsylvania.
- Batchelder, W. H., & Riefer, D. M. (1980). Separation of storage and retrieval factors in free recall of clusterable pairs. Psychological Review, 87, 375–397.
- Batchelder, W. H., & Riefer, D. M. (1986). The statistical analysis of a model for storage and retrieval processes in human memory. British Journal of Mathematical and Statistical Psychology, 39, 129–149.
- Batchelder, W. H., & Riefer, D. M. (1999). Theoretical and empirical review of multinomial process tree modeling. Psychonomic Bulletin & Review, 6, 57–86.
- Bayen, U. J., Murnane, K., & Erdfelder, E. (1996). Source discrimination, item detection, and multinomial models of source monitoring. Journal of Experimental Psychology: Learning, Memory, and Cognition, 22, 197–215.
- Beato, M. S., Pulido, R. F., Pinho, M. S., & Gozalo, M. (2013). Reconocimiento falso y ansiedad estado/rasgo [False recognition and state/trait anxiety]. Psicológica, 34, 299–311.
- Beck, A. T., Steer, R. A., & Brown, G. K. (1996). Manual for the Beck Depression Inventory-II. San Antonio, TX: Psychological Corporation.
- Bleich, S., Havemann-Reinecke, U., & Kornhuber, J. (2002). Fagerström-Test für Nikotinabhängigkeit (FTNA) [Fagerström-Test for Nicotine Dependence]. Göttingen: Hogrefe Verlag.
- Bloch, K. E., Schoch, O. D., Zhang, J. N., & Russi, E. W. (1999). German version of the Epworth Sleepiness Scale. Respiration, 66, 440–447.
- Brand, A. N., Jolles, J., & Gispen-de Wied, C. (1992). Recall and recognition memory deficits in depression. Journal of Affective Disorders, 25, 77–86.
- Brewer, G. A., Knight, J. B., Marsh, R. L., & Unsworth, N. (2010). Individual differences in event-based prospective memory: Evidence for multiple processes supporting cue detection. Memory & Cognition, 38, 304–311.
- Cockburn, J., & Smith, P. T. (1994). Anxiety and errors of prospective memory among elderly people. British Journal of Psychology, 85, 273–282.
- Crawford, J. R., Henry, J. D., Ward, A. L., & Blake, J. (2006). The Prospective and Retrospective Memory Questionnaire (PRMQ): Latent structure, normative data and discrepancy analysis for proxy-ratings. British Journal of Clinical Psychology, 45, 83–104.
- Cuttler, C., & Graf, P. (2008). Sub-clinical checking compulsions are related to impaired prospective memory independently of depression, anxiety and distractibility. Journal of Anxiety Disorders, 22, 642–654.
- Darke, S. (1988). Anxiety and working memory capacity. Cognition & Emotion, 2, 145–154.
- De Lissnyder, E., Koster, E. H. W., Everaert, J., Schacht, R., Van den Abeele, D., & De Raedt, R. (2012). Internal cognitive control in clinical depression: General but no emotion-specific impairments. Psychiatric Research, 199, 124–130.
- De Pascalis, V., & Morelli, A. (1990). Anxiety and individual differences in event-related potentials during the recognition of sense and nonsense words. Personality and Individual Differences, 11, 741–749.
- Einstein, G. O., & McDaniel, M. A. (1990). Normal aging and prospective memory. Journal of Experimental Psychology: Learning, Memory, and Cognition, 16, 717–726.
- Einstein, G. O., & McDaniel, M. A. (2010). Prospective memory and what costs do not reveal about retrieval processes: A commentary on Smith, Hunt, McVay, and McConnell (2007). Journal of Experimental Psychology: Learning, Memory, and Cognition, 36, 1082–1088.
- Einstein, G. O., McDaniel, M. A., Richardson, S. L., Guynn, M. J., & Cunfer, A. R. (1995). Aging and prospective memory: Examining the influences of self-initiated retrieval processes. Journal of Experimental Psychology: Learning, Memory, and Cognition, 21, 996–1007.
- Ellis, H. C., & Ashbrook, P. W. (1988). Resource allocation model of the effects of depressed mood states on memory. In K. Fiedler & J. Forgas (Eds.), Affect, cognition and social behavior (pp. 25–42). Toronto: Hogrefe.
- Erdfelder, E., Auer, T.-S., Hilbig, B. E., Aßfalg, A., Moshagen, M., & Nadarevic, L. (2009). Multinomial processing tree models: A review of the literature. Journal of Psychology, 217, 108–124.
- Eysenck, M. W. (1985). Anxiety and cognitive-task performance. Personality and Individual Differences, 6, 579–586.
- Eysenck, M. W., Derakshan, N., Santos, R., & Calvo, M. G. (2007). Anxiety and cognitive performance: Attentional control theory. Emotion, 7, 336–353.
- Harris, L. M., & Cumming, S. R. (2003). An examination of the relationship between anxiety and performance on prospective and retrospective memory tasks. Australian Journal of Psychology, 55, 51–55.
- Harris, L. M., & Menzies, R. G. (1999). Mood and prospective memory. Memory, 7, 117–127.
- Hatfield, E., & Sprecher, S. (1986). Measuring passionate love in intimate relationships. Journal of Adolescence, 9, 383–410.
- Hautzinger, M., Keller, F., & Kühner, C. (2006). BDI II: Beck Depressions-Inventar—Manual [BDI II: Beck Depression-Inventory—Manual]. Frankfurt am Main: Harcourt Test Services.
- Herrmann, C., Buss, U., & Snaith, R. P. (1995). HADS-D: Hospital Anxiety and Depression Scale—Deutsche Version—Ein Fragebogen zur Erfassung von Angst und Depressivität in der somatischen Medizin [HADS-D: Hospital Anxiety and Depression Scale—German Version—A Questionnaire Measuring Anxiety and Depression in Psychosomatic Medicine]. Bern: Verlag Hans Huber.
- Hertel, P. T., & Milan, S. (1994). Depressive deficits in recognition: Dissociation of recollection and familiarity. Journal of Abnormal Psychology, 103, 736–742.
- Horn, S. S., Bayen, U. J., Smith, R. E., & Boywitt, C. D. (2011). The multinomial model of prospective memory: Validity of ongoing-task parameters. Experimental Psychology, 58, 247–255.
- Joormann, J., Levens, S. M., & Gotlib, I. H. (2011). Sticky thoughts: Depression and rumination are associated with difficulties manipulating emotional material in working memory. Psychological Science, 22, 979–983.
- Kaschel, R. (2002). Erinnern von Erledigungen [Remembering of Plans]. Retrieved from http://www.psy.ed.ac.uk/psy_research/documents/prmq/grprmq-1.pdf
- Klauer, K. C. (2006). Hierarchical multinomial processing tree models: A latent-class approach. Psychometrika, 71, 7–31.
- Klauer, K. C. (2010). Hierarchical multinomial processing tree models: A latent-trait approach. Psychometrika, 75, 70–98.
- Kliegel, M., & Jäger, T. (2006). The influence of negative emotions on prospective memory: A review and new data. International Journal of Computational Cognition, 4, 1–17.
- Kliegel, M., Jäger, T., Phillips, L. H., Federspiel, E., Imfeld, A., Keller, M., & Zimprich, D. (2005). Effects of sad mood on time-based prospective memory. Cognition and Emotion, 19, 1199–1213.
- Last, C. G., Strauss, C. C., & Francis, G. (1987). Comorbidity among childhood anxiety disorders. Journal of Nervous and Mental Disease, 175, 726–730.
- Laux, L., Glanzmann, P., Schaffner, P., & Spielberger, C. D. (1981). STAI: Das State-Trait-Angstinventar [STAI: The State-Trait Anxiety Inventory]. Weinheim: Beltz Testgesellschaft.
- Lee, E., Xiang, Y.-T., Man, D., Au, R. W. C., Shum, D., Tang, W.-K., … Ungvari, G. S. (2010). Prospective memory deficits in patients with bipolar disorder: A preliminary study. Archives of Clinical Neuropsychology, 25, 640–647.
- Livner, A., Berger, A.-K., Karlsson, S., & Bäckman, L. (2008). Differential effects of depressive symptoms on prospective and retrospective memory in old age. Journal of Clinical and Experimental Neuropsychology, 30, 272–279.
- Lunn, D. J., Thomas, A., Best, N., & Spiegelhalter, D. (2000). WinBUGS—A Bayesian modelling framework: Concepts, structure, and extensibility. Statistics and Computing, 10, 325–337.
- MacLeod, C., & Donnellan, A. M. (1993). Individual differences in anxiety and the restriction of working memory capacity. Personality and Individual Differences, 15, 163–173.
- Mathews, A., & MacLeod, C. (1985). Selective processing of threat cues in anxiety states. Behaviour Research and Therapy, 23, 563–569.
- McDaniel, M. A., & Einstein, G. O. (2000). Strategic and automatic processes in prospective memory retrieval: A multiprocess framework. Applied Cognitive Psychology, 14, S127–S144.
- McDaniel, M. A., & Einstein, G. O. (2007). Prospective memory: An overview and synthesis of an emerging field. Thousand Oaks, CA: Sage.
- Overall, J. E. (1962). Dimensions of manifest depression. Journal of Psychiatric Research, 1, 239–245.
- Pavawalla, S. P., Schmitter-Edgecombe, M., & Smith, R. E. (2012). Prospective memory after moderate-to-severe traumatic brain injury: A multinomial modeling approach. Neuropsychology, 26, 91–101.
- Potts, R., Camp, C., & Coyne, C. (1989). The relationship between naturally occurring dysphoric moods, elaborative encoding, and recall performance. Cognition and Emotion, 3, 197–205.
- Ramponi, C., Murphy, F. C., Calder, A. J., & Barnard, P. J. (2010). Recognition memory for pictorial material in subclinical depression. Acta Psychologica, 135, 293–301.
- Rose, E. J., & Ebmeier, K. P. (2006). Pattern of impaired working memory during major depression. Journal of Affective Disorders, 90, 149–161.
- Rose, N. S., Rendell, P. G., McDaniel, M. A., Aberle, I., & Kliegel, M. (2010). Age and individual differences in prospective memory during a “virtual week”: The roles of working memory, vigilance, task regularity, and cue focality. Psychology and Aging, 25, 595–605.
- Rude, S. S., Hertel, P. T., Jarrold, W., Covich, J., & Hedlund, S. (1999). Depression-related impairments in prospective memory. Cognition and Emotion, 13, 267–276.
- Schnitzspahn, K. M., Horn, S. S., Bayen, U. J., & Kliegel, M. (2012). Age effects in emotional prospective memory: Cue valence differentially affects the prospective and retrospective component. Psychology and Aging, 27, 498–509.
- Schuler, H., & Prochaska, M. (2001). LMI: Leistungsmotivationsinventar [AMI: Achievement Motivation Inventory]. Göttingen: Hogrefe Verlag.
- Smith, J. B., & Batchelder, W. H. (2008). Assessing individual differences in categorical data. Psychonomic Bulletin & Review, 15, 713–731.
- Smith, J. B., & Batchelder, W. H. (2010). Beta-MPT: Multinomial processing tree models for addressing individual differences. Journal of Mathematical Psychology, 54, 167–183.
- Smith, R. E. (2003). The cost of remembering to remember in event-based prospective memory: Investigating the capacity demands of delayed intention performance. Journal of Experimental Psychology: Learning, Memory, and Cognition, 29, 347–361.
- Smith, R. E., & Bayen, U. J. (2004). A multinomial model of event-based prospective memory. Journal of Experimental Psychology: Learning, Memory, and Cognition, 30, 756–777.
- Smith, R. E., & Bayen, U. J. (2005). The effects of working memory resource availability on prospective memory: A formal modeling approach. Experimental Psychology, 52, 243–256.
- Smith, R. E., & Bayen, U. J. (2006). The source of adult age differences in event-based prospective memory: A multinomial modeling approach. Journal of Experimental Psychology: Learning, Memory, and Cognition, 32, 623–635.
- Smith, R. E., Bayen, U. J., & Martin, C. (2010). The cognitive processes underlying event-based prospective memory in school-age children and young adults: A formal model-based study. Developmental Psychology, 46, 230–244.
- Smith, R. E., Persyn, D., & Butler, P. (2011). Prospective memory, personality, and working memory: A formal modeling approach. Journal of Psychology, 219, 108–116.
- Spielberger, C. D., Gorsuch, R. L., & Lushene, R. E. (1970). Manual for the State-Trait Anxiety Inventory. Palo Alto, CA: Consulting Psychologists Press.
- Stout, D. M., & Rokke, P. D. (2010). Components of working memory predict symptoms of distress. Cognition and Emotion, 24, 1293–1303.
- Sturtz, S., Ligges, U., & Gelman, A. (2005). R2WinBUGS: A package for running WinBUGS from R. Journal of Statistical Software, 12, 1–16.
- Watts, F. N., Morris, L., & MacLeod, A. K. (1987). Recognition memory in depression. Journal of Abnormal Psychology, 96, 273–275.
- Zigmond, A. S., & Snaith, R. P. (1983). The Hospital Anxiety and Depression Scale. Acta Psychiatrica Scandinavica, 67, 361–370.