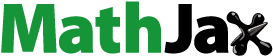
Abstract
Interpretive marine turtle tours in Cyprus yields an alluring ground to unfold the complex nature of pro-environmental behavior among travelers in nature-based destinations. Framing on Collins (2004) interaction ritual concept and the complexity theory, the current study proposes a configurational model and probes the interactional effect of visitors’ memorable experiences with environmental passion and their demographics to identify the causal recipes leading to travelers’ sustainable behaviors. Data was collected from tourists in the marine protected areas located in Cyprus. Such destinations are highly valuable not only for their function as an economic source for locals but also as a significant habitat for biodiversity preservation. Using fuzzy-set Qualitative Comparative Analysis (fsQCA), this empirical study revealed that three recipes predict the high score level of visitors’ environmentally friendly behavior. Additionally, an adaptive neuro-fuzzy inference system (ANFIS) method was applied to train and test the patterns of visitors’ pro-environmental behavior in a machine learning environment to come up with a model which can best predict the outcome variable. The unprecedented implications on the use of technology to simulate and encourage pro-environmental behaviors in sensitive protected areas are discussed accordingly.
Introduction
There is a consensus among scholars that optimizing visitors’ experience is the key to destinations’ success and competitiveness (Ellis & Rossman, Citation2008; Loureiro, Citation2014, Ramkissoon & Uysal, Citation2014, Citation2018: Ramkissoon, Citation2020). Additionally, a shift in the global economy from service-based to experienced-based economy has triggered the focus on the creation and delivery of meaningful and memorable experiences in consumers’ minds by service providers particularly in the tourism industry (Kelly, Citation2020; Kim & Chen, Citation2019; Sthapit et al., Citation2019). The pivotal role of visitors’ experience in elucidating their behavioral intentions such as revisiting, positive word of mouth and recommendation has also exacerbated the attention to the study of memorable tourism experiences (MTE) recently (Chen & Rahman, Citation2018; Dewnarain et al., Citation2019; Gohary et al., Citation2020; Kim & Ritchie, Citation2014; Loureiro, Citation2014; Triantafillidou & Petala, Citation2016; Wong et al., Citation2019). The multidimensional concept of MTE is referred to as an experience that can be recalled and remembered easily after the event (Kim et al., Citation2012). Such emotional assessment of real experience during the travel can impact one’s intention and behavior (e.g., Gohary et al., Citation2020; Sthapit et al., Citation2019).
Although literature evidences a range of studies on MTE (e.g., Akhshik et al., Citation2020; Wong et al., Citation2019), more clarification is required for conclusive results. Findings from previous research reflect that not all dimensions of tourism experience affect travelers in the same way at the post-experience stage (e.g. Kim & Ritchie, Citation2014; Triantafillidou & Petala, Citation2016). This issue highlights the necessity of understanding the mechanism through which various dimensions of MTE may influence visitor behavior.
Additionally, the contribution of MTE to visitors’ behavior in nature-based tourism environments has comparatively received less attention in spite of scholars’ recommendation for the exploration of MTE in new and novel contexts (Huang et al., Citation2019). Further, as indicated in the study of Kim and Chen (Citation2019), revisit intentions and positive word of mouth have been two most likely observed outcomes of MTE research in the literature (e.g., Chen & Rahman, Citation2018; Gohary et al., Citation2020; Wong et al., Citation2019). The study of other significant variables such as travelers’ pro-environmental behavior have received comparatively lesser attention in MTE studies (Lee et al., Citation2015).
Furthermore, an understanding of travellers’ behaviours and intentions in protected areas in non-Western contexts and markets is relatively neglected and thus is highly momentous (Shi et al., Citation2019). To legitimatize the bipolarity of tourism development and the protection of nature, ecological modernization theory encourages a green sacrifice for economic gains in the short-term, with a vow to justify this paranoia through technological advancement (see Mol, Citation2006). Modernization of antiquated service experience with the recent advancement in technology, particularly artificial intelligence (AI) as an extension of human agency (Bryson & Kime, Citation2011) may partly facilitate the ambition to design meaningful experiences per capita that benefit visiting areas by elevating the user-centered design process to change human collective behavior (Hassan & Ramkissoon, Citation2021; Neuhofer et al., Citation2014). In other words, AI is able to train the data and generate a powerful ex-ante estimation of visitors’ behavior which can be used to increase the propensity of pro-environmental behavior at the collective level. Encouraging collective pro-environmental behaviour has been highly recommended by researchers (e.g., Ramkissoon, Citation2020; Ramkissoon et al., Citation2018) as such adjustment may result in improvement of behaviors not only during a visit at the protected areas but also promote behavioural change long term. The use of artificial intelligence in contributing to a sustainable future has been outlined as one of the main research priorities in tourism. Researchers are invited to work on how AI in general and machine learning or deep learning in particular, can be utilized to achieve sustainable tourism (Tussyadiah, Citation2020).
Drawing on Collins' (2004) interaction ritual (IR) theory, which is an under-utilized concept in tourism literature (Sterchele, Citation2020) together with the tenets of complexity theory the present study integrates MTE and environmental passion (EP) to predict tourists’ PEB (). Although EP is known as an influential contributor to individuals’ PEB (Afsar et al., Citation2016; Robertson & Barling, Citation2013), observing its interactional effect with MTE in a nature-based context to explain visitors’ PEB is important and yet insufficiently studied. A series of configural models that simulate MTE, EP and visitors’ demographics to predict visitors’ PEB can be derived. This method enables researchers and practitioners to anticipate or manipulate visitors’ experiences based on the recipes that achieve accurate prediction of outcomes per capita to produce patterns which are generalizable to all individuals in the sample (Woodside, 2018).
The majority of existing studies in the literature have often used structural equation modelling, regression analysis and correlation testing to measure the effect of MTE on travellers’ behavioural outcomes (Chen & Rahman, Citation2018; Coudounaris & Sthapit, Citation2017; Gohary et al., Citation2020; Sthapit et al., Citation2019; Tsai, Citation2016; Wong et al., Citation2019). However, non-linearity, disorder, and instability are inherent characteristics of systems, including behavioural studies in tourism (Boavida-Portugal et al., Citation2017) which mandates the application of deeper insights to reveal non-linearity of the antecedents and their associations with combination of outcomes. The current study uses an adaptive neuro-fuzzy inference system (ANFIS) method to train and test the patterns of visitors’ PEB in a machine learning environment to come up with several compelling predictors that propel travellers’ behavior upward. Although ANFIS modelling “can detect both non-linear and linear relationship between the variables to predict higher accuracy” (Yadegaridehkordi et al., Citation2018, p.370), its application in tourism is scarce. Our study applies machine learning to assist visitor management in ecologically fragile destinations through manipulation of the design process to foster long term behavior change. This is considered as ‘the next evolutionary step in technology use and design’ (Stankov & Gretzel, Citation2020).
Our study aims to address the following research questions: a) Which aspects of MTE in case of marine protected areas are more effective to visitors’ PEB? b) Are both types of generated emotional energy (obsessive environmental passion and harmonious environmental passion) effective in the prediction of participants’ PEB? c) What are the main characteristics of those participants in interpretive marine turtle tours who engage in PEB? and d) In general, which conditions should be met to make tour participants more inclined to be environmentally friendly? These gaps are going to be elaborated utilizing newly emerged technological analysis techniques such as adaptive neuro-fuzzy inference system in the case of interpretive tours.
Nowadays, along with the enormous growth in leisure demand, a move from mass to special interest tourism such as interpretive experiences and education in marine wildlife tours has elevated in recent years where visitors expect more than only entertainment and relaxation (Lück, Citation2015; Citation2016). Interpretive experiences are complex forms of sustainable travel in which education and interpretation remain substantial segments of (eco) tours (Fennell, Citation2008). These tours chiefly aim to educate the significance of wildlife protection to visitors. Previous studies have asserted the considerable contribution of the learning with enjoyment (edutainment) through marine wildlife tourism experiences to the intended PEB (e.g., Pratt & Suntikul, Citation2016).
Theoretical discourse
Interaction ritual concept
Collins’ (2004) interaction ritual (IR) theory has the merit of supporting the link between the social construction of MTE and the transformative effect (e.g., PEB) it brings to consumers of place through emotional erosion (e.g., EP) (Sterchele, Citation2020). Scholars argue that IR theory through its emphasis on interactions that form meanings, emotions and experiences provides a suitable ground for the functioning of rituals that have similar features to tourism practices (Bargeman & Richards, Citation2020; Sterchele, Citation2020; Weenink & Spaargaren, Citation2016). In fact, significant aspects of people’s lives were treated as organized context-related activities in groups (e.g., interpretive tours) instead of occurring in the individualistic form (Bargeman & Richards, Citation2020).
The intellectual history of IR theory dates back to Durkheim’s (1912) research on how emotional arousal emerges from religious rituals (Hausmann, Jonason, & Summers‐Effler, 2011). Drawing on IR theory, future behavior and decisions are influenced by rituals (i.e. MTE) transforming individuals’ feelings into longer-term emotional energy (Sterchele, Citation2020). The generated emotional energy from the experience of the interaction in rituals, acts as a moral constraint on individual behavior (Hausmann et al., Citation2011). Using Goffman’s (1959) main characteristics for an effective interaction ritual, Collins (2004) delineates that the bodily co-presence of people is the first key ingredient which makes them become harmonized with each other. The second factor is the psychological or physical division between people in the group with outsiders. “Mutual focus of attention” and the “shared mood” are the third and fourth ingredients of a prosperous ritual interaction that altogether bring about a shared excitement that fills the participants with emotional energy (Simons, Citation2020). Collins (2004) articulates that these emotions create standards of morality and group solidarity.
Examining IR theory in case of interpretive marine turtle tours in which groups of travelers participate in the tours with the initial willingness to experience and acquire more knowledge about wildlife (Lück, Citation2015) could be expedient. These groups of visitors are often so passionate about nature that they voluntarily engage in releasing turtles into the Mediterranean Sea which is part of their tours (Olya & Akhshik, Citation2019). Moreover, promoting visitors’ PEB is vital to protect the fragile ecosystem particularly in protected areas (Ramkissoon & Mavondo, Citation2015, Citation2017). Predicting the best recipes which can lead to active participation in environmentally friendly behaviors (Ramkissoon et al., Citation2013) is one of the major desired outcomes of these tours (Akhshik et al., Citation2020).
Memorable tourism experience
Experience is said to have a considerable impact on travellers’ behavioural intention (Sthapit et al., Citation2017). On-site tourism experiences bring forth transitory feelings and have a prominent role in memory generation (Gohary et al., Citation2020). MTE is composed of several dimensions including the experience of interaction with community members (local culture), the experience of relaxation (refreshment), the experience of pleasure (hedonism), the experience of fulfilment by tourism (meaningfulness), the experience of novel information (knowledge), the experience of on-site activities (involvement), and the experience of something phenomenal (novelty) (Kim & Ritchie, Citation2014). Evidence in the literature has shown the positive influence of MTE on visitors’ behavioral intentions in various tourism settings such as local food tourism (Tsai, Citation2016), authentic tourism (Coudounaris & Sthapit, Citation2017), cultural tourism (Chen & Rahman, Citation2018), ethnic minority tourism (Wong et al., Citation2019) and eco-tourism (Gohary et al., Citation2020).
Environmental passion
Environmental passion is described as a positive emotion which leads to individuals’ willingness to participate in PEB (Robertson & Barling, Citation2013). Referring to the dualistic model of passion (Vallerand et al., Citation2003), there are two forms of passion that one may develop towards a valued activity. The first type is harmonious passion (HP) which refers to an autonomous internalisation of the favourable activity. Such a feeling generates the motivational energy to willingly participate in the task (Gousse-Lessard et al., Citation2013). The second one is obsessive passion (OP) that unlike the former is a controlled internalisation of the desired activity by which one cannot resist partaking the passionate activity (Vallerand, Citation2010). Although the empirical studies including environmental passion are limited in the literature, some evidence suggests a positive relationship between EP and people’s PEB (Afsar et al., Citation2016; Gilal et al., Citation2019; Robertson & Barling, Citation2013). Moreover, the aforementioned previous studies have only investigated the effect of harmonious passion on the outcome variable. Thus, comprehensive research considering both types of environmental passion simultaneously will be important.
The complexity of human behaviour (PEB)
The development of interpretive experiences, which involves numerous interacting factors, per se is a complex phenomenon (Olya & Akhshik, Citation2019). Additionally, travellers’ environmentally friendly behaviours in tourism destinations have always been an important discussion for managers, researchers and policymakers (Akhshik et al., Citation2020). This is evidenced by numerous studies on predictors of visitors’ PEB across settings including highly valued ecological areas (Landon et al., Citation2018;Lee et al., Citation2019; Li & Wu, Citation2019; Li et al., Citation2020; Wu et al., Citation2020). Nevertheless, the complexities of tourism and individuals’ environmental behaviours have been relatively neglected in the literature and need further elaboration (Akhshik et al., Citation2020; Akhshik et al., Citation2020; Lezak & Thibodeau, Citation2016; Olya & Akhshik, Citation2019; Ramkissoon et al., Citation2012). Although, human behaviour is best predicted when outcomes are extracted from non-linear antecedents that are not necessarily the ‘sum of the separate effects’ (Byrne, Citation1998, p. 20), often in the extant literature, visitors’ PEB has been treated based on linear relationships (Byrne, Citation1998; Mackie, Citation1974; McDonald, Citation2009) using regression analysis or structural equation modelling (Nunkoo et al., Citation2013; Nunkoo & Ramkissoon, Citation2012). Correspondingly, the authors aim to address this deficiency and discuss the complex nature of visitors’ environmentally friendly behavior. In their review paper on responsible behaviour of nature-based tourists, Lee et al. (Citation2013, p. 102) stated that “scholars have adopted various terms to describe behavior that protects the environment” such as environmentally concerned behaviour, environmentally responsible behaviour or pro-environmental behaviour. Based on the literature, this study epitomized nature-based tourists’ PEBs as behaviours that not only does not harm the environment but also benefit the environment of the destinations they visited as well. These behaviours can cover a wide range of activities such as the acquisition of knowledge about environmental protection, purchasing or donations in favour of ecological protection, picking up litter in the destination, persuading others to protect the nature, changing one’s lifestyle after visitation to minimise negative impacts on the environment (Higham & Carr, Citation2002; Smith-Sebasto & D'Costa, Citation1995; Steg & Vlek, Citation2009).
Background, materials and tools
Tourism 4.0, AI and machine learning
Human is undergoing the 4th industrial revolution characterised by the advancement of emerging technologies such as AI (Schwab, Citation2016). AI was first presented in the 1950s by John McCarthy as “the science and engineering of making intelligent machines, especially intelligent computer programs” (McCarthy, Citation2007, n.p.). Machine learning (ML) which is known as a subset of AI, uses computational algorithms that learns (pattern recognition) and improve from experience of real-world data (training sets) to predict an outcome and to make decisions on its own (Bini, Citation2018). Such technology-based transformations have influenced the tourism industry by providing interconnected and phygital systems which are known as Tourism 4.0 (Stankov & Gretzel, Citation2020).
Adaptive Neuro Fuzzy Inference System (ANFIS) which is known as a machine learning technique consists of a combination of artificial neural networks and fuzzy inference system (Karaboga & Kaya, Citation2020) which has been recently adopted in the tourism and hospitality industry to predict various variables. For example, Sun et al. (Citation2019) used ML to accurately forecast tourist arrivals for popular destinations in China. Using ANFIS, Atsalakis et al. (Citation2018) forecasted the success of a newly launched service in tourism. Recommender systems in tourism, an example of AI, have been the focus of research in recent years (Nilashi et al., Citation2017). Another recent application of ML models was to forecast hotel room prices in the Gulf Cooperation Council (GCC) countries (Al Shehhi & Karathanasopoulos, Citation2020). ML was used as a technique to predict travellers’ choice preferences of eco-friendly hotels (Nilashi et al., Citation2019) and as a means for searching query data for tourism and hospitality forecasting (Li et al., Citation2020). ML methods were also utilized to predict potential situations of over-tourism in destinations (Perles‐Ribes et al., Citation2020). In their review paper, Jiao and Chen (Citation2019) reported that AI-based models are becoming a new trend in tourism demand forecasting studies. In sum, ML and deep learning have attracted growing interest in tourism to refine predictions in recent years and especially, ML techniques have been found to be suitable for long-term and mid-term forecasting (Claveria et al., Citation2016).
There is also considerable evidence in literature suggesting how ML may contribute to environmental issues (e.g., Froemelt et al., Citation2020; Grant et al., Citation2020). ML creates a mathematical model to predict or make decisions without human interventions. The application of this approach in environmental studies has shown that it can accurately predict potential human behaviour and provide valuable contribution to tackle ecological and conservational challenges. For example, the exact tracking of the spatial distribution of fisheries’ impacts on remote areas and high seas has been a difficult task for many years for scientists (de Souza et al., Citation2016). In order to understand the global behaviour of fisheries, ML was able to locate a large fraction of the likely fishing events successfully to support the conservational management programs (de Souza et al., Citation2016). Within the context of our daily lives, ML can be used to predict households’ consumption behavior by providing a comprehensive information base for policymakers to derive environmental strategies that may reduce consumers’ ecological footprints accordingly (Froemelt et al., Citation2020). Machine learning algorithm was used in several other studies to predict individuals’ various environmental behaviours such as outdoor water conservation (Grant et al., Citation2020), electric vehicle purchase behavior (de Rubens, Citation2019) and green consumption behaviour of college students (Tang et al., Citation2020) among others.
Methodology
Study area
Alagadi Beach in Northern Cyprus is one of the few sites in the Mediterranean where two globally categorized as endangered turtles namely loggerheads (Caretta caretta) and green (Chelonia mydas) turtles nest. Sadly, human recreational activities and their consequences are among the main anthropogenic threatening factors that affect marine turtle nesting areas (Camiñas & De Málaga, Citation2004). As the economic resources generated by tourism are inevitable, the protection of these endangered species at the same time mandates the presence of strict environmental management. To promote ecological well-being, the conservational plan for the marine protected area of the study site is administrated by the “society for the protection of turtles” in Northern Cyprus. The members of the tours whose number do not exceed 20 people per visit are booked in advance and the visitors participate in a video-based educational programme followed by a comprehensive introduction about the area and turtles. The final stage in the tour is a guided activity of baby turtle release into the sea. These practices as one of the forms of alternative tourism enhance travelers’ interest in nature which is undoubtedly a significant factor in managing protected areas (Kim et al., Citation2020). Protected areas are generally highly precious for ecosystem services, tourism, preserving biodiversity, and also generating economic benefits for indigenous people (Afifi et al., Citation2017; Maldonado-Oré & Custodio, Citation2020) contributing to sustainable tourism development.
Measurement scales
Well-established scales from the relevant literature feed the data for this study. In this regard, the dualistic model of passion i.e. obsessive passion (6 items) and harmonious passion (6 items) was measured based on items adapted from Marsh et al. (Citation2013) and Vallerand et al. (Citation2003). The multidimensionality of memorable tourism experience (MTE) composed of hedonism (4 items), refreshment (4 items), local culture (3 items), meaningfulness (3 items), new knowledge (3 items), involvement (3 items), and novelty (4 items) which was adopted from Kim and Ritchie (Citation2014). In line with studies of Su et al. (Citation2018) and Thapa (Citation2010), pro-environmental behaviour has been adapted from Smith-Sebasto and D'Costa (Citation1995). All items were gauged by 5-point Likert scale ranging from ‘strongly disagree’ (1) to ‘strongly agree’ (5), except PEB, HP and OP that ranged from ‘strongly disagree’ (1) to ‘strongly agree’ (7).
Reliability, readability, timing and clarity of the items were assessed by conducting a pilot study (Karatepe et al., Citation2020a) with 20 participants and 10 academics in the field. No modifications were required. (appendix) presents the measurement tools and items.
Sampling and procedure
After obtaining the necessary permissions from the Society for the protection of turtles, the research team referred to the site during the hatchling summer season of 2018. The data was collected in two phases: pre-visit and post-visit. The latter is believed to convey a structural shift in the behaviour of marine-watching visitors (Forestell & Kaufman, 1990). In the first phase, the research team contacted the visitors face to face. The visitors who agreed to participate in the survey were provided with the consent form, purpose of the study and questions about their demographic information and their email address inquiry. Additionally, the respondents were assured about their anonymity and informed that they would be contacted via email. In total, out of 520 visitors who visited Alagadi beach during the study time, 438 people agreed to participate in this research. A follow-up questionnaire was sent by email to the participants after 4 months to capture their MTE, EP and their PEB. A total 332 (75.79%) filled questionnaires were returned for the analysis. Moreover, remedies such as temporal, proximal and methodological separation of measurement items such as counterbalancing question order and reversing coded items were considered to reduce the potential common method bias (MacKenzie & Podsakoff, Citation2012). The distribution of gender, age, education, marital status, income and nationality is presented in .
Table 1. Respondents’ profile (n = 332).
Data analysis
After the assessment of psychometric properties, correlation coefficient of the variables was calculated using cross-tabulation and Cramér’s V test to determine the extent of the relationship between the antecedent and the outcome (Olya et al., Citation2020). Cross-tabulation reveals the existence of contrarian cases in the data set. Therefore, the associations among antecedents and outcome of PEB are asymmetrical. As a result, the study pursues configural and non-linear models to address the inherent heterogeneity of PEB using fsQCA (Ragin, Citation2014). In this phase, the data were calibrated to fuzzy membership scores, and then, the fuzzy truth table was crafted using Boolean algebra by minimization of Boolean function (Quine-McCluskey method). This step produces a list of all possible conditions resulting in high and low scores of PEB. Finally, to refine the relatively important recipe from this list, counterfactual analysis was performed. Consistency and coverage are two probabilistic criteria in this step (Ragin, Citation2009). The formulas to calculate these are as follows:
Where indicates case i’s membership score in set X and
presents case i’s membership score in set Y in the outcome condition (Ragin, 2008). A threshold value beyond 1 and 8 is commonly accepted for coverage and consistency, respectively.
The study further investigates predictive validity of the model by fairly dividing the sample into two sub-samples; a causal recipe from sub-sample 1 was then compared with the holdout sample (see Gigerenzer & Brighton, Citation2009). Further, to simulate the behavior of visitors, ANFIS was used to portray the possibility of the prediction of these behaviors in a machine learning environment to identify and rank critical factors that contribute to the high PEB of the visitors. In this phase, the data was split to sub-sample (75% of the total sample), and holdout sample (35% of the total sample). Then, the subsample was used as an input to train the data while the holdout sample was an input for testing data. The in-depth clarification of each step is presented below.
Results and discussion
Psychometric properties
(appendix) reports the descriptive statistics, normality test, and exploratory factor analysis (EFA) results using the principal components analysis (PCA) and the varimax rotation method. Skewness and Kurtosis test provides evidence of normality of data distribution that falls in the commonly accepted values of (Bowen, 2006). EFA reveals the items accurately loaded under their measuring scales at an acceptable level (λ > 0.64). Moreover, the eigenvalue for all of the scales was higher than 1.00.
The reliability of the constructs was assessed using Cronbach’s alpha (>.70) (see ). Internal consistency was validated using composite reliability (CR) which is greater than the commonly accepted 0.70 cut-off level (Bagozzi & Yi, Citation1988). provides evidence of convergent validity by illustrating the average variance extracted (AVE) > .50 and greater than maximum shared squared variance (MSV) for each construct (Fornell & Larcker, Citation1981). A CFA has been performed with acceptable loadings of items under desired factors (standardized factor loading > .50; p < .001) (see ). provides evidence of discriminant validity by comparing the factor correlations with square roots of the AVEs (Fornell & Larcker, Citation1981).
Table 2. Result of CFA, CR, AVE, MSV and α.
Table 3. Cross-Tabulation Analysis of Obsessive Passion* Pro-environmental Behaviour.
Results of Cross-Tabulation analyses
To further pursue the most appropriate approach to study PEB, cross-tabulation of an antecedent (OP) and the outcome (PEB) was conducted to reveal the existence of contrarian cases in the dataset. Consequently, linear approaches may not adequately explain the behavior of these individuals that runs counter to the main effect (Woodside, 2014, 2018). As an example, the results in reveal that 70 individuals (21% of the sample) were not passionate about the environment but behaved pro-environmentally. Moreover, the negation of these relations presents 15 individuals (4.5% of the sample) who were passionate about the environment but failed to behave pro-environmentally. Consequently, despite the positive correlation between OP and PEB, there is a heterogeneity in the relationships that is hidden in the data not accurately addressed by conventional methods. Therefore, the study of PEB as a complex phenomenon demands further exploration (Ramkissoon et al., Citation2013c) which needs to consider the complex relationships using configural and non-linear modelling (Woodside, 2018).
Results of model testing
To further pursue the asymmetrical and configural model of the study (), fsQCA was applied to provide unprecedented results to determine high/low scores of PEB. Arrow A in indicates that the confluence of MTE and demographics on PEB [peb = f(hed, kno, inv, loc, mng, nov, ref, gen,mar,age,inc,edu] results in four unique recipes (A: M1-M4; solution coverage: .23, Solution consistency: .98) (see ). To clarify the recipes, an example of A: M1 (age*gen*mar*inc*∼edu*hed*mng*loc*∼nov*kno*ref) reveals that older married females with high incomes and less education who scored high on hedonism, meaningfulness, local culture, knowledge and refreshment, but lacked novelty achieved a high pro-environmental behavior score. This is in line with the studies of Olya and Akhshik (Citation2019) and Olya and Gavilyan (Citation2017) who found that these characteristics result in higher intention to behave pro-environmentally and support sustainable tourism development, respectively. The study further investigates the negation of PEB (. ∼A: M1-M4). To clarify with an example, ∼A:M1 (age*∼gen*mar*inc*∼edu*hed*mng*loc*nov*inv*∼kno*ref), reveals a recipe towards a low score of pro-environmental behavior of visitors through older married and uneducated males with higher incomes who score high on hedonism, meaningfulness, local culture, novelty, involvement and refreshment, but didn’t have sufficient knowledge of the area. Therefore, contrary to the assumption of the conventional and symmetrical approaches, a low score of PEB is not the mirror opposite of a high score in PEB. However, fsQCA facilitates crafting different recipes for the negation of the same outcome. More recipes regarding Arrow B [peb = f(hp, op, gen,mar,age,inc,edu)] and ∼ B are illustrated in .
Table 4. Configural Models of high and low scores of Peb (model A, B and their negations).
presents the configural models of high and low scores of PEB (Arrow C in ) with all the antecedents [peb and ∼ peb: f(hed, kno, inv, loc, mng, nov, ref, hp, op, gen, mar, age, inc, edu)]. Accordingly, three recipes for high score peb and two recipes for low score PEB sufficiently describe PEB and its negation (). As an example, . C: M1. (age*∼gen*mar*inc*∼edu*hed*mng*loc*nov*inv*∼kno*ref*∼op*∼hp) reveals that older, uneducated, married and wealthy males who scored high on memorable tourism experience dimensions except for knowledge but lacked obsessive and harmonious passion achieved high score of PEB. This finding is contrary to results of previous studies dominated by findings on passion having a positive effect on PEB. This is majorly due to the misinterpretations of symmetric models based on conventional methods (e.g. Afsar et al., Citation2016). However, in line with the results of the current study, Junot et al. (Citation2017) report that obsessive passion negatively correlates with environmental behaviors. What is evident, however, is that passion may not be sufficient or necessary to adjust PEB. It means that people who lack obsessive passion are not necessarily opposed to behaving pro-environmentally.
Table 5. Configural models of high and low scores of Peb with all the antecedents (model C and its negation).
Tenets of complexity theory
Woodside (2017) proposes 6 tenets to evaluate complexity theory based on the empirical data. Accordingly, a single antecedent rarely suffices to predict the desired outcome (tenet 1). Moreover, a complex interaction of the antecedents describes high/low scores of the outcome. As seen in . C: M1. (age*∼gen*mar*inc*∼edu*hed*mng*loc*nov*inv*∼kno*ref*∼op*∼hp), a complex interaction of memorable tourism experience and passion predict the high score in PEB.
Tenet 3, known as Equifinality principle proposes that a recipe that sufficiently predicts the outcome, is not necessary by itself, as there are other paths to predict the same result. Accordingly, in this study, a number of recipes described the high/low scores in PEB.
Tenet 4, known as the causal asymmetry principle, proposes that the rejected outcome is unique. In this study the negation of PEB is not the mirror opposite of the high score PEB ().
Tenet 5 propose that a single antecedent can contribute positively or negatively to predict the outcome, that depends on the other ingredients in the model. The results of the study on the other side revealed that the dualistic dimension of passion contributed to the high PEB scores both positively and negatively ().
Tenet 6 proposes that a given recipe is relevant for some, but not all cases and the coverage is less than 1. This is evident in illustrated by the fuzzy XY plot. Therefore, as predicted by Kollmuss and Agyeman (Citation2002), PEB is extremely complex and overly simplistic models may not fully predict the PEB of the visitors (Ramkissoon et al., Citation2012; Siegel et al., Citation2018).
Table 6. Evidence of predictive validity on two subsamples.
Predictive validity
The result of the predictive validity provides further insights on the ex-ante power of the proposed model and configurations. As illustrated in , we have divided the sample to a sub-sample and holdout sample. Drawing on an instance from the result of fsQCA (), a fuzzy XY plot has been portrayed with the recipes on X-axis and PEB on Y-axis with the sub-sample (Consistency: 1; Coverage: 0.09). Later, the same configural model was portrayed using the holdout sample (Consistency: 1; Coverage: 0.10). The comparisons of the models on different samples provide evidence for predictive validity of the proposed configural model (Olya et. al., Citation2020).
Simulation analysis with ANFIS
Conventional methods may oversimplify the human agency process (Yadegaridehkordi et al., Citation2018); many researchers have thus recommended soft computing techniques ( Ahani et al., Citation2017; Liébana-Cabanillas et al., Citation2017, Yadegaridehkordi et al., Citation2018 ). ANFIS integrates the cognitive dimension of human intelligence and the mechanism of reasoning with the empirical examination of the collected evidence, which allows the in-depth prediction of the future outcome. However, ANFIS was used as a complementary method in this study as it is not designed for casual relationships or conventional hypothesis testing due to its ‘Black Box’ operations (Liébana-Cabanillas et al., Citation2017). In the current study, ANFIS is performed in two ways. First, it is used to determine the most critical factor contributing to PEB. ANFIS is capable of identifying the relationships between optimal input and the output; therefore, a sensitivity analysis can identify the most relevant and critical antecedents to predict PEB (see ). Second, it is used to simulate and forecast pro-environmental behavior with the sufficient antecedents retrieved from fsQCA. In this regard, the output from fsQCA was input for ANFIS further analysis.
Table 7. ANFIS sensitivity analysis results
As an information processing system ANFIS combines the power of artificial network and fuzzy inference system with a multilayer feed-forward network. The fundamental building block of the ANFIS is a single neuron known as a ‘Perceptron’. Forward propagation of a perceptron in a neural network is illustrated in .
Each input () has a corresponding weight (
), and the inputs have an error (e) tolerance (
) as well. Multiplication of each of the inputs proceeds by membership functions that provide a sum (∑), then Fuzzy If-Then rules formulate the conditional statements that contain fuzzy logic. Then, through a normalization layer ANFIS defuzzifies (L) the weights and estimated errors and the final output (
) is produced. By comparing the errors from the actual output, the testing data predicts the best possible solution from training data in a process called “back-propagation” where the network feed backwards in each perceptron by calculating root mean square error (RMSE). To test the performance criteria, the RMSE of predicted values of ANFIS output are compared (Karaboga & Kaya, Citation2019).
Therefore, the operation of ANFIS is based on nodes that are arranged in different layers. The first layer is usually the input. Then, these inputs are merged in other nodes and layers based on the membership functions. Each node is regarded as a mathematical function that performs operations on its incoming input and processes it into and generates a corresponding output. This technique facilitates various fuzzy membership function categories. To obtain the optimal category, we generated the variables with different FIS in different categories (see , appendix). Gaussian curve membership function (gaussmf) with three categories (low, medium, and high) was deemed adequate among the existing FIS to further pursue the process (RMSE = 2.0922). In the next step, the data was split into 75:25 ratios for training and testing, respectively (Abubakar et al., Citation2019). Ten epochs with the error tolerance of 0.05 for all the 9 inputs and one output were defined. illustrates the data set index for training data (O) and FIS output (*) of PEB and all antecedents.
Figure 3. Plot representing training data (O) and FIS output (*) of outcome (Peb) and all the antecedents.
Moreover, by excluding variables from the equation, ANFIS sensitivity analysis () revealed that by eliminating meaningfulness (Model 2) from the equation, RMSE is significantly increasing. It indicates the importance of meaningfulness in predicting PEB. On the other hand, the model that lacks knowledge (Model 7) has the minimum change on RMSE. This means that knowledge contributes less to the overall prediction of PEB. The surface view of the highest and lowest contributor to PEB is displayed in .
Moreover, our comparison of different models that were extracted from fsQCA outputs demonstrates the ex-ante power of ANFIS to adequately train and predict the behaviors of visitors. It, therefore facilitates the selection of models with the best performance derived from the real-life experience in a machine learning area. To explain further, the ANFIS was tuned and run for each model derived from . The first model (C: M1) has the smallest training and testing RMSE compared to other models and overall performs better (training RMSE = 0.72415, testing RMSE = 1.5536) (See ).
Conclusion and implications
The management of human impacts on fragile and sensitive ecological resources needs a comprehensive understanding of behaviours and their influential antecedents. Meanwhile, the study of individuals’ discretionary environmentally friendly behaviours or pro-environmental behaviours continue to attract considerable attention in tourism and hospitality and other disciplines (e.g., Ari et al., Citation2020; Karatepe et al., Citation2020b; Ramkissoon, Citation2020; Rezapouraghdam et al., Citation2018). However, the majority of the studies in the tourism and hospitality literature did not use ML approach which creates a mathematical model to predict the future behavior of individuals. The trained data prepared by ML, provides a valuable information base for destination managers and policymakers to derive appropriate context-based environmental strategies that may mitigate the future environmental hazards to the environment.
Using ML to identify the combination of factors that can lead to visitors’ pro-environmentally friendly behaviours in a tourism destination, the present study brings forth several methodological, theoretical and practical implications in the context of marine protected areas. Theoretically, our proposed model was designed based on the interaction ritual concept and confirmed by tenets of complexity theory. Complexity theory asserts that the interaction of tour members’ MTE, EP and their demographics revive their PEB. This finding may further contribute to the body of knowledge. Although the interaction ritual (IR) concept along with its emotional energy notion provides a worthwhile tool to analyze and understand visitors’ behaviors affected by their travel experiences and interactions during tours, it is an underutilized framework in tourism literature. Our study advances the application of this theory by explicating tourists’ environmentally friendly behaviors in destinations and thus contributing to sustainable development goals (Ramkissoon, Citation2020; Ramkissoon et al., Citation2020). Secondly, the affirmation of our proposed conceptual model by complexity theory is an attempt to focus on the complexities of visitors’ behavior in relation with environmental phenomena which have been extensively neglected in the literature because of treating such complexities through linear approaches. Our study concludes that relying on the IR concept, the interaction of members during the interpretive marine turtle tours are effective in fostering environmental passion which may promote their responsible behavior toward the environment. However, as asserted by complexity theory, the level of such environmentally friendly behaviors varies (e.g. high, low, and medium) among the members due to the interaction between their demographics and other two variables (EP & MTE).
Our study may also contribute to the application of artificial intelligence which has been identified as one of the research priorities in sustainable tourism field (Tussyadiah, Citation2020). Our research has attempted to advance the knowledge in the field by using AI, specifically ML techniques to predict, interpret and analyze the complexity of tourists’ behaviors. Although it has been long since these technologies have been introduced (McCarthy, Citation2007), their application in the tourism and hospitality industry is still in its infancy and scholars have only recently started to utilize them for various predictive purposes (e.g. Al Shehhi & Karathanasopoulos, Citation2020; Li et al., Citation2020; Nilashi et al., Citation2019; Sun et al., Citation2019). Instead of fencing off visitors from ecologically valuable sites, alternative tourism with the aid of technology provides insights to manage visitors accordingly.
Destination managers can benefit from our findings in several ways. Our findings suggest the importance of optimizing the quality of tourists’ experiences and make them memorable. This can be achieved through increasing the meaningfulness of their travel by emphasizing their presence as a helping factor for saving the lives of baby turtles. Professional tour guides can provide further information and enhance participants’ knowledge which is an important factor in creating a memorable tourism experience. Arranging tours is a way through which tourists may experience the local culture and unique local food will be beneficial for hedonism and novelty. Moreover, the IR concept used in this study also provides implications for managers. In order to achieve a successful ritual interaction, the destination managers can increase the time participants spend together on site. Besides, the participants can be given some unique symbols such as badges, shirts or hats so that they can identify themselves as being different from other visitors. Finally, to enhance the mutual focus of attention and the shared mood the professional tour guides can be trained with a number of meditation techniques to share with the tour members before starting their turtle rescuing activities. These practices together can help the members experience more excitement and fill them with the desired emotional energy that creates standards of morality (Collins, 2004).
The technology-based prediction has the potential to reform the experience design through the information gathered from the visitors. This technique can record different aspects of an experience to offer potential benefits to managing bodies, visitors, and visiting areas. Practitioners can use ML-empowered software at destinations to predict and provide services in a way that desired and expected outcomes may best be achieved.
In other words, AI could address the heterogeneity nature of service experiences per capita to encourage predictable outcomes. Planners can produce AI-based apps to predict or calibrate pro-environmental behaviors on site. On the other hand, PEB itself is a complex phenomenon that could be reached through the interaction of different antecedents. Nevertheless, the combination of these antecedents may not be the same for different demographics. Inclusion of demographic data in the prediction of PEB unfolds unprecedented implications for managerial bodies in order to design possible personas. Moreover, an experience design with the aim of elevating PEB of visitors may be achieved through different combination of antecedents, and unless these combinations reach a certain tipping point level, self-transformation may not occur (Gladwell, Citation2006). This study addresses this issue by applying a state-of-the-art method i.e. fsQCA and ANFIS. Our study found three unique recipes (. C. M1-M3) addressing significant gaps in theory and practice. It is of the highest concern for tourism stakeholders to understand the underlying patterns shaping the high/low pro-environmental behavior of visitors, particularly at sensitive ecological sites. Additionally, the combination of memorable tourism experience and passion for the environment provides a unique solution to provoke tourists’ PEB which has rarely been investigated in the tourism literature.
Limitations and future research
The study was limited to self-report data collected from visitors; future studies may use other psychophysical approaches in managing visitors’ experience. The majority of the travellers to North Cyprus were from Britain and Germany at the time of data collection (Ataoğlu, Citation2019). Considering the importance of cultural backgrounds in shaping attitudes and behaviours, future research can consider including the influence of culture in promoting PEB.
Also, since the study site in the present study was limited to Alagadi beach, different study settings may provide a better understanding of managing tourism in protected areas. The equifinality principle emphasizes the existence of various recipes towards the desired outcome. Our study, however, was limited to two constructs (i.e. passion and memorable experiences). A combination of different antecedents provides more recipes to predict PEB. Future studies may include different antecedents of PEB. Moreover, measuring PEB using actual consumption patterns, or dividing the outcome to high-effort, low-effort PEB may add unprecedented value to future research. Our study was limited to data collected from visitors. Future studies would benefit from integrating other stakeholders. Finally, the limited spatio-temporal data used in this study opens an avenue to future studies to replicate the findings of our research.
Disclosure statement
No potential conflict of interest was reported by the authors.
Additional information
Notes on contributors
Hamed Rezapouraghdam
Hamed Rezapouraghdam is currently the director and assistant professor of the School of Tourism and Hotel Management at Bahçeşehir Cyprus University. He received his BA from Faculty of Foreign Languages, Isfahan University and his M.S. and Ph.D. from Faculty of Tourism, Eastern Mediterranean University. His research interests are in the areas of corporate sustainability, behavioral psychology and sustainable tourism.
Arash Akhshik
Arash Akhshik, PhD., is a researcher at the Institute of Environmental Science, Jagiellonian University in Krakow. his research cross cuts socio-ecological systems related to leisure activities, specifically, he is interested in the creation of experiences that provoke a profound self-change in nature-based visitors.
Haywantee Ramkissoon
Haywantee Ramkissoon is a Research Professor (Full) of Tourism Marketing at the University of Derby, UK. She leads the visitor economy research group. She is also affiliated with the School of Business & Economics, at UiT, The Arctic University of Norway and Johannesburg Business School, University of Johannesburg, South Africa. Her research has gained international significance evidenced by media coverage, prestigious awards and academic and industry keynotes. She engages in collaborative research with national and international academic and industry partners for the benefits of individuals and society. Her current research is in business innovation, sustainable tourism, behaviour change, residents’ attitudes, place attachment, pro-environmental behaviour, cultural tourism, CSR, social customer relationship management, public health and quality of life. She has published widely and serves on several editorial advisory boards and as a reviewer across disciplines. She currently serves as editor/co-editor of books and special issues in her field.
References
- Abubakar, A. M., Behravesh, E., Rezapouraghdam, H., & Yildiz, S. B. (2019). Applying artificial intelligence technique to predict knowledge hiding behavior. International Journal of Information Management, 49, 45–57. https://doi.org/10.1016/j.ijinfomgt.2019.02.006
- Afifi, E., Mavondo, F., Ramkissoon, H., & Aleti, T. (2017). The role of advertising stereotypes in destination image formation and behavioural intentions of tourists to consume indigenous tourism attractions. CAUTHE 2017: Time for Big Ideas? Re-Thinking the Field for Tomorrow, 610.
- Afsar, B., Badir, Y., & Kiani, U. S. (2016). Linking spiritual leadership and employee pro-environmental behavior: The influence of workplace spirituality, intrinsic motivation, and environmental passion. Journal of Environmental Psychology, 45, 79–88. https://doi.org/10.1016/j.jenvp.2015.11.011
- Ahani, A., Rahim, N. Z. A., & Nilashi, M. (2017). Forecasting social CRM adoption in SMEs: A combined SEM-neural network method. Computers in Human Behavior, 75, 560–578. https://doi.org/10.1016/j.chb.2017.05.032
- Akhshik, A., Ozturen, A., & Rezapouraghdam, H. (2020). A passionate travel to mind green turtles—Unpacking the complexity of visitors' green behaviour. International Journal of Tourism Research, https://doi.org/10.1002/jtr.2407
- Akhshik, A., Rezapouraghdam, H., & Ramkissoon, H. (2020). Industrialization of Nature in the Time of Complexity Unawareness: The Case of Chitgar Lake, Iran. Journal of Hospitality & Tourism Research, 1096348020964949.
- Al Shehhi, M., & Karathanasopoulos, A. (2020). Forecasting hotel room prices in selected GCC cities using deep learning. Journal of Hospitality and Tourism Management, 42, 40–50. https://doi.org/10.1016/j.jhtm.2019.11.003
- Ari, E., Karatepe, O. M., Rezapouraghdam, H., & Avci, T. (2020). A conceptual model for green human resource management: Indicators, differential pathways, and multiple pro-environmental outcomes. Sustainability, 12(17), 7089. https://doi.org/10.3390/su12177089
- Ataoğlu, F. (2019). retrieved December 19, 2020, from https://www.hurriyet.com.tr/ekonomi/kktcnin-hedefi-1-5-milyon-turist-41152796.
- Atsalakis, G. S., Atsalaki, I. G., & Zopounidis, C. (2018). Forecasting the success of a new tourism service by a neuro-fuzzy technique. European Journal of Operational Research, 268(2), 716–727. https://doi.org/10.1016/j.ejor.2018.01.044
- Bagozzi, R. P., & Yi, Y. (1988). On the evaluation of structural equation models. Journal of the academy of marketing science, 16(1), 74–94.
- Bargeman, B., & Richards, G. (2020). A new approach to understanding tourism practices. Annals of Tourism Research, 84, 102988 https://doi.org/10.1016/j.annals.2020.102988
- Bini, S. A. (2018). Artificial intelligence, machine learning, deep learning, and cognitive computing: what do these terms mean and how will they impact health care? The Journal of Arthroplasty, 33(8), 2358–2361. https://doi.org/10.1016/j.arth.2018.02.067
- Boavida-Portugal, I., Ferreira, C. C., & Rocha, J. (2017). Where to vacation? An agent-based approach to modelling tourist decision-making process. Current Issues in Tourism, 20(15), 1557–1574. https://doi.org/10.1080/13683500.2015.1041880
- Byrne, D. S. (1998). Complexity theory and the social sciences: An introduction. Psychology Press. ES.United Kingdom.
- Bryson, J. J., & Kime, P. P. (2011). Just an artifact: Why machines are perceived as moral agents [Paper presentation]. In Twenty-Second International Joint Conference on Artificial Intelligence, June.
- Camiñas, J. A., & De Málaga, C. O. (2004). Sea turtles of the Mediterranean Sea: population dynamics, sources of mortality and relative importance of fisheries impacts. FAO Fisheries Report, 738, 27–84.
- Chen, H., & Rahman, I. (2018). Cultural tourism: An analysis of engagement, cultural contact, memorable tourism experience and destination loyalty. Tourism Management Perspectives, 26, 153–163. https://doi.org/10.1016/j.tmp.2017.10.006
- Claveria, O., Monte, E., & Torra, S. (2016). Combination forecasts of tourism demand with machine learning models. Applied Economics Letters, 23(6), 428–431.
- Coudounaris, D. N., & Sthapit, E. (2017). Antecedents of memorable tourism experience related to behavioral intentions. Psychology & Marketing, 34(12), 1084–1093.
- D’Costa 1995: Smith-Sebasto, N. J., & D'Costa, A. (1995). Designing a Likert-type scale to predict environmentally responsible behavior in undergraduate students: A multistep process. The Journal of Environmental Education, 27(1), 14–20. https://doi.org/10.1080/00958964.1995.9941967
- de Rubens, G. Z. (2019). Who will buy electric vehicles after early adopters? Using machine learning to identify the electric vehicle mainstream market. Energy, 172, 243–254.
- de Souza, E. N., Boerder, K., Matwin, S., & Worm, B. (2016). Improving fishing pattern detection from satellite AIS using data mining and machine learning. PloS One, 11(7), e0158248. https://doi.org/10.1371/journal.pone.0158248
- Dewnarain, S., Ramkissoon, H., & Mavondo, F. (2019). Social customer relationship management: An integrated conceptual framework. Journal of Hospitality Marketing & Management, 28(2), 172–188. https://doi.org/10.1080/19368623.2018.1516588
- Ellis, G. D., & Rossman, J. R. (2008). Creating Value for Participants through Experience Staging: Parks, Recreation, and Tourism in the Experience Industry. Journal of Park & Recreation Administration, 26(4), 1–20.
- Fennell, D. A. (2008). Ecotourism: an Introduction., third ed. Routledge.
- Forestell, P. H., Kaufman, G. D. (1990, May). The history of whale watching in Hawaii and its role in enhancing visitor appreciation for endangered species. In Proceedings of the 1990 Congress on Coastal and Marine Tourism (Vol. 2, pp. 399–407). National Coastal Resources Research Institute.
- Fornell, C., & Larcker, D. F. (1981). Evaluating structural equation models with unobservable variables and measurement error. Journal of marketing research, 18(1), 39–50.
- Froemelt, A., Buffat, R., & Hellweg, S. (2020). Machine learning based modeling of households: A regionalized bottom‐up approach to investigate consumption‐induced environmental impacts. Journal of Industrial Ecology, 24(3), 639–652. https://doi.org/10.1111/jiec.12969
- Gigerenzer, G., & Brighton, H. (2009). Homo heuristicus: Why biased minds make better inferences. Topics in cognitive science, 1(1), 107–143.
- Gilal, F. G., Ashraf, Z., Gilal, N. G., Gilal, R. G., & Channa, N. A. (2019). Promoting environmental performance through green human resource management practices in higher education institutions: A moderated mediation model. Corporate Social Responsibility and Environmental Management, 26(6), 1579–1590.
- Gladwell, M. (2006). The tipping point: How little things can make a big difference. Little, Brown.
- Gohary, A., Pourazizi, L., Madani, F., & Chan, E. Y. (2020). Examining Iranian tourists’ memorable experiences on destination satisfaction and behavioral intentions. Current Issues in Tourism, 23(2), 131–136. https://doi.org/10.1080/13683500.2018.1560397
- Gousse-Lessard, A. S., Vallerand, R. J., Carbonneau, N., & Lafrenière, M. A. K. (2013). The role of passion in mainstream and radical behaviors: A look at environmental activism. Journal of Environmental Psychology, 35, 18–29. https://doi.org/10.1016/j.jenvp.2013.03.003
- Grant, S. B., Duong, K., Rippy, M. A., Pierce, G., Feldman, D., Zanetti, E., & McNulty, A. (2020). From yards to cities: a simple and generalizable probabilistic framework for upscaling outdoor water conservation behavior. Environmental Research Letters, 15(5), 054010. https://doi.org/10.1088/1748-9326/ab7c1e
- Hassan, A., & Ramkissoon, H. (2021). Potentials of tourism products and services in Bangladesh. In A. Hassan (Ed.), Tourism marketing in Bangladesh: An introduction (pp.317–328). Routledge.
- Hausmann, C., Jonason, A., & Summers–Effler, E. (2011). Interaction ritual theory and structural symbolic interactionism. Symbolic Interaction, 34(3), 319–329. https://doi.org/10.1525/si.2011.34.3.319
- Higham, J., & Carr, A. (2002). Ecotourism visitor experiences in Aotearoa/New Zealand: challenging the environmental values of visitors in pursuit of pro-environmental behaviour. Journal of Sustainable Tourism, 10(4), 277–294. https://doi.org/10.1080/09669580208667168
- Huang, Y. F., Zhang, Y., & Quan, H. (2019). The relationship among food perceived value, memorable tourism experiences and behaviour intention: the case of the Macao food festival. International Journal of Tourism Sciences, 19(4), 258–268.
- Jiao, E. X., & Chen, J. L. (2019). Tourism forecasting: A review of methodological developments over the last decade. Tourism Economics, 25(3), 469–492. https://doi.org/10.1177/1354816618812588
- Junot, A., Paquet, Y., & Martin-Krumm, C. (2017). Passion for outdoor activities and environmental behaviors: A look at emotions related to passionate activities. Journal of Environmental Psychology, 53, 177–184.
- Karaboga, D., & Kaya, E. (2019). Adaptive network based fuzzy inference system (ANFIS) training approaches: A comprehensive survey. Artificial Intelligence Review, 52(4), 2263–2293. https://doi.org/10.1007/s10462-017-9610-2
- Karaboga, D., & Kaya, E. (2020). Estimation of number of foreign visitors with ANFIS by using ABC algorithm. Soft Computing, 24(10), 7579–7591. https://doi.org/10.1007/s00500-019-04386-5
- Karatepe, O. M., Rezapouraghdam, H., & Hassannia, R. (2020a). Job insecurity, work engagement and their effects on hotel employees’ non-green and nonattendance behaviors. International Journal of Hospitality Management, 87, 102472. https://doi.org/10.1016/j.ijhm.2020.102472
- Karatepe, O. M., Rezapouraghdam, H., & Hassannia, R. (2020b). Does employee engagement mediate the influence of psychological contract breach on pro-environmental behaviors and intent to remain with the organization in the hotel industry? Journal of Hospitality Marketing & Management, 1–28. https://doi.org/10.1080/19368623.2020.1812142
- Kelly, C. (2020). Beyond ‘a trip to the seaside’: exploring emotions and family tourism experiences. Tourism Geographies, 1–22.
- Kim, H., & Chen, J. S. (2019). The memorable travel experience and its reminiscence functions. Journal of Travel Research, 58(4), 637–649. https://doi.org/10.1177/0047287518772366
- Kim, J. H. (2018). The impact of memorable tourism experiences on loyalty behaviors: The mediating effects of destination image and satisfaction. Journal of Travel Research, 57(7), 856–870. https://doi.org/10.1177/0047287517721369
- Kim, J. H., & Ritchie, J. B. (2014). Cross-cultural validation of a memorable tourism experience scale (MTES). Journal of Travel Research, 53(3), 323–335. https://doi.org/10.1177/0047287513496468
- Kim, J. H., Ritchie, J. B., & McCormick, B. (2012). Development of a scale to measure memorable tourism experiences. Journal of Travel Research, 51(1), 12–25. https://doi.org/10.1177/0047287510385467
- Kim, Y. J., Lee, D. K., & Kim, C. K. (2020). Spatial tradeoff between biodiversity and nature-based tourism: Considering mobile phone-driven visitation pattern. Global Ecology and Conservation, 21, e00899. https://doi.org/10.1016/j.gecco.2019.e00899
- Kollmuss, A., & Agyeman, J. (2002). Mind the gap: why do people act environmentally and what are the barriers to pro-environmental behavior? Environmental Education Research, 8(3), 239–260. https://doi.org/10.1080/13504620220145401
- Landon, A. C., Woosnam, K. M., & Boley, B. B. (2018). Modeling the psychological antecedents to tourists’ pro-sustainable behaviors: An application of the value-belief-norm model. Journal of Sustainable Tourism, 26(6), 957–972. https://doi.org/10.1080/09669582.2017.1423320
- Lee, T. H., Jan, F. H., & Huang, G. W. (2015). The influence of recreation experiences on environmentally responsible behavior: The case of Liuqiu Island, Taiwan. Journal of Sustainable Tourism, 23(6), 947–967. https://doi.org/10.1080/09669582.2015.1024257
- Lee, T. H., Jan, F. H., & Yang, C. C. (2013). Environmentally responsible behavior of nature-based tourists: A review. International Journal of Development and Sustainability, 2(1), 100–115.
- Lee, Y. K., Pei, F., Ryu, K. S., & Choi, S. (2019). Why the tripartite relationship of place attachment, loyalty, and pro-environmental behaviour matter? Asia Pacific Journal of Tourism Research, 24(3), 250–267. https://doi.org/10.1080/10941665.2018.1564344
- Lezak, S. B., & Thibodeau, P. H. (2016). Systems thinking and environmental concern. Journal of Environmental Psychology, 46, 143–153. https://doi.org/10.1016/j.jenvp.2016.04.005
- Li, Q. C., & Wu, M. Y. (2019). Rationality or morality? A comparative study of pro-environmental intentions of local and nonlocal visitors in nature-based destinations. Journal of Destination Marketing & Management, 11, 130–139. https://doi.org/10.1016/j.jdmm.2019.01.003
- Li, Q., & Wu, M. (2020). Tourists’ pro-environmental behaviour in travel destinations: benchmarking the power of social interaction and individual attitude. Journal of Sustainable Tourism, 28(9), 1371–1389. https://doi.org/10.1080/09669582.2020.1737091
- Li, X., Li, H., Pan, B., & Law, R. (2020). Machine learning in Internet search query selection for tourism forecasting. Journal of Travel Research, 0047287520934871.
- Liébana-Cabanillas, F., Marinković, V., & Kalinić, Z. (2017). A SEM-neural network approach for predicting antecedents of m-commerce acceptance. International Journal of Information Management, 37(2), 14–24. https://doi.org/10.1016/j.ijinfomgt.2016.10.008
- Loureiro, S. M. C. (2014). The role of the rural tourism experience economy in place attachment and behavioral intentions. International Journal of Hospitality Management, 40, 1–9. https://doi.org/10.1016/j.ijhm.2014.02.010
- Lück, M. (2015). Education on marine mammal tours–But what do tourists want to learn? Ocean & Coastal Management, 103, 25–33. https://doi.org/10.1016/j.ocecoaman.2014.11.002
- Lück, M. (2016). The teachable moments on marine mammal tours: Watching versus swim-with tours. Coastal Management, 44(2), 131–138. https://doi.org/10.1080/08920753.2016.1135274
- MacKenzie, S. B., & Podsakoff, P. M. (2012). Common method bias in marketing: Causes, mechanisms, and procedural remedies. Journal of Retailing, 88(4), 542–555. https://doi.org/10.1016/j.jretai.2012.08.001
- Mackie, J. L. (1974). The cement of the universe: A study of causation. Clarendon Press.
- Maldonado-Oré, E. M., & Custodio, M. (2020). Visitor environmental impact on protected natural areas: An evaluation of the Huaytapallana Regional Conservation Area in Peru. Journal of Outdoor Recreation and Tourism, 31, 100298. https://doi.org/10.1016/j.jort.2020.100298
- Marsh et al. 2013: Marsh, H. W., Vallerand, R. J., Lafrenière, M. A. K., Parker, P., Morin, A. J., Carbonneau, N., … & Paquet, Y. (2013). Passion: Does one scale fit all? Construct validity of two-factor passion scale and psychometric invariance over different activities and languages. Psychological assessment, 25(3), 796. https://doi.org/10.1037/a0032573
- McCarthy, J. (2007). What is artificial intelligence? http://jmc.stanford.edu/articles/whatisai/whatisai.pdf.
- McDonald, J. R. (2009). Complexity science: an alternative world view for understanding sustainable tourism development. Journal of Sustainable Tourism, 17(4), 455–471. https://doi.org/10.1080/09669580802495709
- Mol, A. P. (2006). Ecological modernization. In Encyclopedia of Globalization. (No. A to E, pp. 354–357). Routledge, A Taylor and Francis Company.
- Neuhofer, B., Buhalis, D., & Ladkin, A. (2014). A typology of technology‐enhanced tourism experiences. International Journal of Tourism Research, 16(4), 340–350. https://doi.org/10.1002/jtr.1958
- Nilashi, M., Ahani, A., Esfahani, M. D., Yadegaridehkordi, E., Samad, S., Ibrahim, O., Sharef, N. M., & Akbari, E. (2019). Preference learning for eco-friendly hotels recommendation: A multi-criteria collaborative filtering approach. Journal of Cleaner Production, 215, 767–783. https://doi.org/10.1016/j.jclepro.2019.01.012
- Nilashi, M., Bagherifard, K., Rahmani, M., & Rafe, V. (2017). A recommender system for tourism industry using cluster ensemble and prediction machine learning techniques. Computers & Industrial Engineering, 109, 357–368. https://doi.org/10.1016/j.cie.2017.05.016
- Nunkoo, R., & Ramkissoon, H. (2012). Structural equation modelling and regression analysis in tourism research. Current Issues in Tourism, 15(8), 777–802. https://doi.org/10.1080/13683500.2011.641947
- Nunkoo, R., Ramkissoon, H., & Gursoy, D. (2013). Use of structural equation modeling in tourism research: Past, present, and future. Journal of Travel Research, 52(6), 759–771. https://doi.org/10.1177/0047287513478503
- Olya, H. G., & Akhshik, A. (2019). Tackling the complexity of the pro-environmental behavior intentions of visitors to turtle sites. Journal of Travel Research, 58(2), 313–332. https://doi.org/10.1177/0047287517751676
- Olya, H., Altinay, L., Farmaki, A., Kenebayeva, A., & Gursoy, D. (2020). Hotels’ sustainability practices and guests’ familiarity, attitudes and behaviours. Journal of Sustainable Tourism, 1–19.
- Olya, H. G., & Gavilyan, Y. (2017). Configurational models to predict residents’ support for tourism development. Journal of Travel Research, 56(7), 893–912.
- Perles‐Ribes, J. F., Ramón‐Rodríguez, A. B., Moreno‐Izquierdo, L., & Such‐Devesa, M. J. (2020). Machine learning techniques as a tool for predicting overtourism: The case of Spain. International Journal of Tourism Research, 22(6), 825–838. https://doi.org/10.1002/jtr.2383
- Pratt, S., & Suntikul, W. (2016). Can marine wildlife tourism provide an “edutaining” experience? Journal of Travel & Tourism Marketing, 33(6), 867–884. https://doi.org/10.1080/10548408.2015.1069778
- Ragin, C. C. (2009). Redesigning social inquiry: Fuzzy sets and beyond. University of Chicago Press.
- Ragin, C. C. (2014). The comparative method: Moving beyond qualitative and quantitative strategies. University of California Press.
- Ramkissoon, H. (2020). COVID-19 Place confinement, pro-social, pro-environmental behaviors, and residents' wellbeing: A new conceptual framework. Front Psychol, 11, 2248 https://doi.org/10.3389/fpsyg.2020.02248
- Ramkissoon, H., & Mavondo, F. T. (2015). The satisfaction–place attachment relationship: Potential mediators and moderators. Journal of Business Research, 68(12), 2593–2602. https://doi.org/10.1016/j.jbusres.2015.05.002
- Ramkissoon, H., & Mavondo, F. T. (2017). Proenvironmental behavior: Critical link between satisfaction and place attachment in Australia and Canada. Tourism Analysis, 22(1), 59–73. https://doi.org/10.3727/108354217X14828625279735
- Ramkissoon, H., Mavondo, F., & Sowamber, V. (2020). Corporate social responsibility at LUX* resorts and hotels: Satisfaction and loyalty implications for employee and customer social responsibility. Sustainability, 12(22), 9745. https://doi.org/10.3390/su12229745
- Ramkissoon, H., Mavondo, F., & Uysal, M. (2018). Social involvement and park citizenship as moderators for quality-of-life in a national park. Journal of Sustainable Tourism, 26(3), 341–361. https://doi.org/10.1080/09669582.2017.1354866
- Ramkissoon, H., Smith, L. D. G., & Weiler, B. (2013). Relationships between place attachment, place satisfaction and pro-environmental behaviour in an Australian national park. Journal of Sustainable Tourism, 21(3), 434–457. https://doi.org/10.1080/09669582.2012.708042
- Ramkissoon, H., & Uysal, M. (2014). Authenticity as a value co-creator of tourism experiences. Creating experience value in tourism, 113–124.
- Ramkissoon, H., & Uysal, M. S. (2018). Authenticity as a value co-creator of tourism experiences. Creating experience value in tourism, 3, 98–109.
- Ramkissoon, H., Weiler, B., & Smith, L. D. G. (2012). Place attachment and pro-environmental behaviour in national parks: The development of a conceptual framework. Journal of Sustainable Tourism, 20(2), 257–276. https://doi.org/10.1080/09669582.2011.602194
- Rezapouraghdam, H., Alipour, H., & Darvishmotevali, M. (2018). Employee workplace spirituality and pro-environmental behavior in the hotel industry. Journal of Sustainable Tourism, 26(5), 740–758. https://doi.org/10.1080/09669582.2017.1409229
- Robertson, J. L., & Barling, J. (2013). Greening organizations through leaders' influence on employees' pro-environmental behaviors. Journal of Organizational Behavior, 34(2), 176–194. https://doi.org/10.1002/job.1820
- Schwab, K. (2016). The fourth industrial revolution. World Economic Forum/Penguin.
- Shi, F., Weaver, D., Zhao, Y., Huang, M. F., Tang, C., & Liu, Y. (2019). Toward an ecological civilization: Mass comprehensive ecotourism indications among domestic visitors to a Chinese wetland protected area. Tourism Management, 70, 59–68. https://doi.org/10.1016/j.tourman.2018.07.011
- Siegel, L., Cutter-Mackenzie-Knowles, A., & Bellert, A. (2018). Still ‘minding the gap’sixteen years later:(re) storying pro-environmental behaviour. Australian Journal of Environmental Education, 34(2), 189–203.
- Simons, I. (2020). Changing identities through collective performance at events: the case of the Redhead Days. Leisure Studies, 39(4), 568–517. https://doi.org/10.1080/02614367.2020.1768281
- Smit, B., & Melissen, F. (2018). Sustainable customer experience design: Co-creating experiences in events, tourism and hospitality. Routledge.
- Smith-Sebasto, N. J., & D'Costa, A. (1995). Designing a Likert-type scale to predict environmentally responsible behavior in undergraduate students: A multistep process. The Journal of Environmental Education, 27(1), 14–20. https://doi.org/10.1080/00958964.1995.9941967
- Stankov, U., & Gretzel, U. (2020). Tourism 4.0 technologies and tourist experiences: a human-centered design perspective. Information Technology & Tourism, 22(3), 477–488.
- Steg, L., & Vlek, C. (2009). Encouraging pro-environmental behaviour: An integrative review and research agenda. Journal of Environmental Psychology, 29(3), 309–317. https://doi.org/10.1016/j.jenvp.2008.10.004
- Sterchele, D. (2020). Memorable tourism experiences and their consequences: An interaction ritual (IR) theory approach. Annals of Tourism Research, 81, 102847. https://doi.org/10.1016/j.annals.2019.102847
- Sthapit, E., Björk, P., & Coudounaris, D. N. (2017). Emotions elicited by local food consumption, memories, place attachment and behavioural intentions. Anatolia, 28(3), 363–380. https://doi.org/10.1080/13032917.2017.1322111
- Sthapit, E., Coudounaris, D. N., & Björk, P. (2019). Extending the memorable tourism experience construct: an investigation of memories of local food experiences. Scandinavian Journal of Hospitality and Tourism, 19(4-5), 333–353. https://doi.org/10.1080/15022250.2019.1689530
- Su, L., Huang, S. S., & Pearce, J. (2018). How does destination social responsibility contribute to environmentally responsible behaviour? A destination resident perspective. Journal of Business Research, 86, 179–189.
- Sun, S., Wei, Y., Tsui, K. L., & Wang, S. (2019). Forecasting tourist arrivals with machine learning and internet search index. Tourism Management, 70, 1–10. https://doi.org/10.1016/j.tourman.2018.07.010
- Tang, H., Xu, Y., Lin, A., Heidari, A. A., Wang, M., Chen, H., Luo, Y., & Li, C. (2020). Predicting green consumption behaviors of students using efficient firefly grey wolf-assisted k-nearest neighbor classifiers. IEEE Access., 8, 35546–35562. https://doi.org/10.1109/ACCESS.2020.2973763
- Thapa, B. (2010). The mediation effect of outdoor recreation participation on environmental attitude-behavior correspondence. The Journal of environmental education, 41(3), 133–150. https://doi.org/10.1080/00958960903439989
- Triantafillidou, A., & Petala, Z. (2016). The role of sea-based adventure experiences in tourists’ satisfaction and behavioral intentions. Journal of Travel & Tourism Marketing, 33(sup1), 67–87. https://doi.org/10.1080/10548408.2015.1008667
- Tsai, C. T. (2016). Memorable tourist experiences and place attachment when consuming local food. International Journal of Tourism Research, 18(6), 536–548. https://doi.org/10.1002/jtr.2070
- Tussyadiah, I. (2020). A review of research into automation in tourism: Launching the annals of tourism research curated collection on artificial intelligence and robotics in tourism. Annals of Tourism Research, 81, 102883. https://doi.org/10.1016/j.annals.2020.102883
- Vallerand, R. J. (2010). On passion for life activities: The dualistic model of passion. In Advances in experimental social psychology (Vol. 42, pp. 97–193). Academic Press.
- Vallerand, R. J., Blanchard, C., Mageau, G. A., Koestner, R., Ratelle, C., Léonard, M., Gagné, M., & Marsolais, J. (2003). Les passions de l'ame: on obsessive and harmonious passion. Journal of Personality and Social Psychology, 85(4), 756–767. https://doi.org/10.1037/0022-3514.85.4.756
- Weenink, D., & Spaargaren, G. (2016). Emotional agency navigates a world of practices. Practice Theory and Research: Exploring the Dynamics of Social Life, 60–84.
- Wong, J. W. C., Lai, I. K. W., & Tao, Z. (2019). Memorable ethnic minority tourism experiences in China: a case study of Guangxi Zhuang Zu. Journal of Tourism and Cultural Change, 17(4), 508–525. https://doi.org/10.1080/14766825.2019.1600866
- Wu, J., Font, X., & Liu, J. (2020). Tourists’ pro-environmental behaviors: moral obligation or disengagement? Journal of Travel Research, 0047287520910787.
- Yadegaridehkordi, E., Nilashi, M., Nasir, M. H. N. B. M., & Ibrahim, O. (2018). Predicting determinants of hotel success and development using Structural Equation Modelling (SEM)-ANFIS method. Tourism Management, 66, 364–386. https://doi.org/10.1016/j.tourman.2017.11.012
Appendix
Table A.1. Results of EFA, and descriptive statistics of scale items (n = 332)
Table A2. ANFIS best configuration for Peb according to membership function type and its category.
Table A3. Result of discriminant validity analysis.