Abstract
The growing interest in intelligent automation adoption and development in tourism presents many potential solutions for sustainable tourism issues. To map the current landscape of research on intelligent automation in sustainable tourism, a systematic review was conducted of 213 scholarly articles. Five major themes were identified: intelligent automation to enhance tourist experience, preserve heritage, promote quality of life, measure tourist experience, and conserve the environment. Scholarly work on this topic has paid more attention to the economic and sociocultural aspects of sustainability but less to addressing environmental issues. To uncover further research gaps, this study outlines sustainability transition pathways using two dimensions: sustainability inclusion and tourist involvement. Twenty-three distinct AI-based innovations were mapped onto the pathways to reveal future research directions. The findings explain the lack of AI-based solutions that offer high levels of sustainability inclusion and tourist involvement. Thus, this study proposes the “AI4GoodTourism” framework, inferring that intelligent automation with high sustainability inclusion levels can scale up the marginal contributions that tourists provide collectively. This framework serves to guide future research and development for various stakeholders who are progressing sustainable tourism agendas through intelligent automation.
Introduction
The tourism sector has become increasingly automated. Furthermore, recent advancements in artificial intelligence (AI) have brought intelligence to automation. Intelligent automation broadly incorporates the use of technologies with cognitive, affective, and analytical capabilities to complete activities that humans have traditionally performed (Tuomi & Ascenção, Citation2023). It is leveraged across different sectors that support the tourism industry, such as travel, heritage, and hospitality (Tussyadiah, Citation2020). When the recent COVID-19 pandemic brought the tourism industry to an almost complete standstill (Gössling et al., Citation2021), intelligent automation-based technologies, such as robots, were adopted to mitigate the risk of infection among travellers by offering physically distant services to facilitate the restart of the industry (Pillai et al., Citation2021). However, caution towards over-reliance on intelligent automation must be observed as more adoption may lead to a lack of human touch and also impact employment opportunities (Tuomi et al., Citation2021).
Moving forward, sustainability must be at the heart of any tourism operation. Indeed, intelligent automation can be leveraged to drive sustainability and improve environmental, social, and economic performances, from reducing the use of natural resources to predicting tourist behaviour (González-Rodríguez et al., Citation2020; Pereira-Doel et al., Citation2019). Previous works have also acknowledged how technological innovation is integral to sustainable tourism development (Loureiro et al., Citation2022; Santos et al., Citation2021). More specifically, Rahmadian et al. (Citation2022) analysed how big data and AI are utilised to support sustainability in tourism. They mapped the general areas in tourism where AI techniques have been applied to facilitate big data solutions. However, little is known regarding the extent to which intelligent automation can play a crucial role in addressing sustainability issues in the tourism industry and where further research attention is needed. Since AI innovations in tourism attract scholars from various disciplines, such as tourism management (e.g. Tuomi et al., Citation2021) and information technology (e.g. Zhang et al., Citation2021), it is also pertinent to explore disciplinary contributions by mapping the current collaboration patterns from the related literature.
This paper fills these gaps by conducting a systematic review of relevant literature to address two key objectives. First, this study clarifies the current landscape of research on intelligent automation in the context of sustainable tourism to facilitate an understanding of how the power of technology can be harnessed in achieving a sustainable tourism agenda. Second, it maps sustainability transition pathways by showing how AI-based innovations would help bring changes through sustainability inclusion while embracing intelligent automation for sustainable tourism development. Recommendations for ideal future research directions are discussed under the proposed “AI4GoodTourism” framework. Acknowledging the role of tourists as the catalyst for change in the area of sustainable tourism (Juvan & Dolnicar, Citation2016), the framework suggests that intelligent automation with high sustainability inclusion levels can scale up the marginal contributions that tourists collectively provide. As such, the framework serves as a guideline for future research and development for tourism stakeholders interested in intelligent automation.
Overview of intelligent automation and sustainable tourism
Automation is widely understood as the implementation of a process or service delivery performed by either an autonomous agent or a machine with or without minimal human intervention (Ivanov et al., Citation2022). A range of automated applications has AI capabilities, referring to the extent to which automation technologies can think or act humanly and rationally (Tussyadiah, Citation2020). These capabilities include natural language processing (to communicate in a human language), knowledge representation (to store relevant information), automated reasoning (to conclude new conversations), machine learning (to extrapolate patterns and continually adapt to new situations), computer vision and speech recognition (to perceive the surroundings) or robotics (to handle objects and move about) (Russell & Norvig, Citation2021). Intelligent automation has AI capabilities, unlike other mechanical automation, such as self-service check-in kiosks at hotels (Ivanov & Webster, Citation2019).
While mechanical automation only focuses on repetitive and simple tasks, intelligent automation empowers technologies with cognitive, affective, and analytical capabilities to learn and adapt in a data-driven industry (Tuomi & Ascenção, Citation2023). Tussyadiah (Citation2020) defines intelligent automation in tourism as an integrated system of next-generation technologies designed to operate service tasks without human intervention. The applications of intelligent automation could be seen in personalisation engines, autonomous vehicles, virtual guides, and robot chefs. Furthermore, Tussyadiah (Citation2020) calls for more research on intelligent automation to promote sustainable tourism.
Sustainable tourism requires a balance between the three fundamental pillars of sustainability—environmental, social and economic (Butler, Citation1999). This is to ensure that tourism development can meet the needs of current generations without compromising those of future generations (Weaver, Citation2005). The tourism industry presents a unique challenge due to consumers’ transient and hedonic nature (Dolnicar, Citation2020; Font & McCabe, Citation2017). AI may solve this issue with its ability to manage a large amount of complex and real-world data. Therefore, addressing sustainability issues in tourism can have important implications for the broader service sectors (Roxas et al., Citation2020).
However, what should be considered sustainable tourism is unclear, and it is a stumbling block to progress. Indeed, many studies have discussed the definitional issues of sustainable tourism (Moyle et al., Citation2021). Still, academic literature has paid little attention to answering questions such as whether understanding tourism demand should be recognised as part of sustainable tourism actions (Liu, Citation2003). There are some existing frameworks, such as the Triple Bottom Line (Stoddard et al., Citation2012) and the Causal Loop Diagram (Roxas et al., Citation2020), to guide sustainable tourism development. However, these frameworks also fail to provide information on specific sustainable tourism actions, thereby posing a challenge to evaluating the tangible contribution of one technology adopted in tourism to sustainable tourism development.
To overcome this challenge, a framework introduced by UNEP and UNWTO (2005) offers a useful structure to explicate and make sense of the contribution of intelligent automation to the various aspects of sustainable tourism development. The framework contains 12 aims for the sustainable tourism agenda formulated to guide policymaking processes. Since each aim includes clear examples of sustainable tourism actions (see ), any practical benefits brought by certain technologies can be linked to the respective sustainable tourism issues that they help to address. This understanding is crucial because tourism stakeholders need clear guidelines to ascertain how the development and adoption of intelligent automation will contribute to the sustainable tourism agenda. Knowing which technology to deploy and for which purposes will allow them to identify different approaches that will enable them to mitigate the negative impacts and optimise the benefits of such technology adoption (Gössling, Citation2021; Tussyadiah, Citation2020).
Table 1. Summary of 12 aims for the sustainable tourism agenda.
Methodology
Guided by the Preferred Reporting Items for Systematic Reviews and Meta-Analyses (PRISMA) and the guidelines proposed by Kitchenham and Charters (Citation2007), a systematic literature review was conducted to map the current landscape of intelligent automation for sustainable tourism. It identified relevant keywords associated with the concepts, including “intelligent automation,” “sustainability,” “sustainable tourism areas,” and “tourism.” By combining the identified keywords (see ), a series of search strings were developed and used to retrieve scholarly articles in the following databases: Web of Science, Scopus, Science Direct, IEEE Xplore, ACM Digital Library, and Google Scholar. The searches were adjusted to yield the most targeted results based on title, abstract, and keywords. Appendix A (supplementary material) presents the adjustments in each of the searched sources. All the search processes took place in December 2021.
Table 2. Search strategy.
In terms of inclusion criteria, this systematic review included articles published after 2011, the year when the term “Industry 4.0” was introduced (Culot et al., Citation2020). Guided by the PRISMA procedure, the following criteria were applied to filter the search results:
Articles on intelligent automation technology development, deployment, or implications for its use within the tourism domain were included.
Articles had to be either empirical or conceptual research; however, review articles were excluded.
Articles published in journals and conferences were included.
Articles were written in the English language.
Using this search strategy, the initial search retrieved 6,489 articles. Thereafter, title and abstract screening helped identify 401 relevant articles. The number of articles retrieved from each of the databases are as follows: Web of Science (n = 167), Scopus (n = 40), Science Direct (n = 42), IEEE Xplore (n = 55), ACM Digital Library (n = 19) and Google Scholar (n = 78). Of these 401 articles, 95 duplicates (i.e. articles retrieved from more than one database) were found and removed. Thus, a total of 306 full-text articles were downloaded. Thereafter, 116 articles were excluded for one of three reasons: they were review papers, the technologies discussed did not have AI capabilities, or they were outside of the tourism context. This elimination resulted in the remaining 190 articles. Furthermore, the snowballing approach identified 23 relevant articles not retrieved in the previous search (Wohlin, Citation2014). Finally, a total of 213 articles were admitted for this systematic review. presents the PRISMA flow diagram of information passing through various stages of review. Appendix B (supplementary material) contains the list of all selected papers. Due to methodological limitations, there might be a few relevant papers that were not captured during the search process.
A network analysis was conducted on the keywords, disciplinary, and affiliation collaborations using an R-package for science mapping analysis called bibliometrix, and its web-based interface, biblioshiny (Aria & Cuccurullo, Citation2017). The analysis was conducted to capture general trends of research and collaboration patterns in intelligent automation for the sustainable tourism literature. Three centrality measures (i.e. degree, closeness, and betweenness) were examined to analyse the characteristics of the networks. Degree centrality measured in PageRank informs the influential nodes beyond their direct connections with other nodes in the network (Cambridge Intelligence, Citation2020). Closeness centrality measures the proximity between nodes to provide information on the span of influence each node has over the entire network (Yin et al., Citation2006). Lastly, betweenness centrality measures the extent to which a node connects with other nodes to show its influence over the entire network regarding the spread and flow of information (Kim et al., Citation2022).
Findings and discussion
shows an increasing trend of research interest in the field of intelligent automation for tourism in the last few years. Of the included 213 scholarly articles, 148 are journal articles and 65 are conference papers. The number of published journal articles spiked after 2018 (14 articles in 2019, 44 in 2020, and 65 in 2021). The rapid advancement in AI capabilities, as recently demonstrated by the large language model (LLM), ChatGPT (Carvalho & Ivanov, Citation2023), will undoubtedly attract more scholarly attention that will affect how research on intelligent automation is conducted in tourism.
Previous studies employed a computational approach (n = 78, 36.6%) to deal with the aspects associated with developing and deploying intelligent automation technologies such as robots (e.g. Duchetto et al., Citation2019). The quantitative approach (n = 52, 24.4%) involved employing various statistical techniques, such as structural equation modelling on quantitative data obtained by conducting surveys or experiments (e.g. Tussyadiah & Miller, Citation2019). Next, the conceptual approach (n = 24, 11.3%) focused on explicating concepts, developing potential hypotheses for investigations and scrutinising the impact of intelligent automation (e.g. Cohen & Hopkins, Citation2019). Qualitative methods (n = 17, 7.9%) were employed to conduct in-depth interviews and field observations to gain rich insights into a given context (e.g. Tuomi et al., Citation2021). Furthermore, a mixed-methods approach (n = 13, 6.1%) was also evident when combining quantitative and qualitative approaches in a study (e.g. Pillai & Sivathanu, Citation2020). In addition, other studies combined multiple methods, such as computational, followed by quantitative or qualitative techniques. Examples of studies with computational and quantitative approaches (n = 20, 9.4%) are evident in tourism demand forecasting research, where scholars use techniques such as deep learning (e.g. Zhang et al., Citation2021). Works such as Wu et al. (Citation2021) used computational and qualitative approaches (n = 9, 4.3%) in collecting and analysing online reviews.
A network clustering analysis (Lee et al., Citation2020) was performed on keywords in all documents to examine the most frequently used keywords and how they relate to each other. shows the keyword network analysis on the articles using the leading eigenvalue clustering algorithm based on 38 nodes. Appendix C (supplementary material) presents the corresponding centrality measure. “Artificial intelligence” has the highest betweenness, closeness, and PageRank measures, thus showing its frequent use in the domain of intelligent automation for sustainable tourism research, followed by keywords such as “service robots,” “robots,” “machine learning,” “cultural heritage,” “forecasting,” “hospitality” and “tourism.” These keywords reflect study contexts in the sphere of intelligent automation in tourism. AI-powered technologies and techniques such as “conversational agent,” “computer vision,” and “chatbot” have also been studied. This paper finds keywords such as “trust,” “acceptance,” “customer experience,” “human-robot interaction,” and “anthropomorphism,” indicating not only aspects that research considers when deploying these AI-powered technologies but also how the deployment affects their end users. Lastly, “covid-19′ is found to be connected with keywords such as “artificial intelligence” and “robots.” This signifies how research on intelligent automation in tourism is expected to increase in the post-COVID-19 era (Pillai et al., Citation2021).
Network analyses were employed at both discipline and affiliation levels to explore the disciplinary collaboration patterns. This paper adopted the disciplinary collaboration network developed by Pal and Chua (Citation2016) and identified affiliations across different disciplines. depicts the discipline-by-discipline network based on six nodes using the leading eigenvalue clustering algorithm. Meanwhile, presents the affiliation-by-affiliation collaboration network based on 35 nodes using the Louvain algorithm. The size of the nodes signifies the number of intra-disciplinary studies, while the lines connecting each node indicate the collaborations among disciplines and affiliations. Thicker lines imply stronger collaborations between the respective disciplines and affiliations. Appendix D (supplementary material) provides information on the grouping of affiliations into disciplines.
Figure 4. a) Discipline-by-discipline collaboration network; b) affiliation-by-affiliation collaboration network.
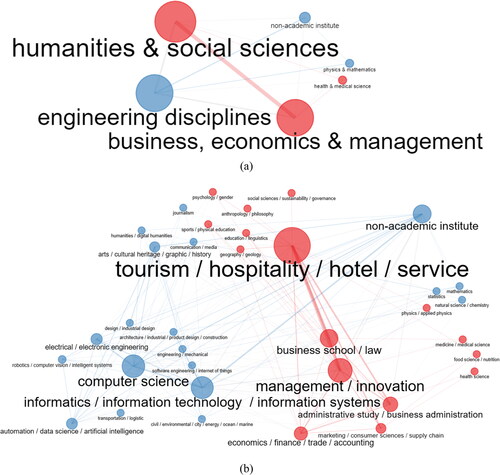
Both intra-disciplinary (n = 106) and inter-disciplinary (n = 107) scholarly collaborations have been found in the selected articles. Intra-disciplinary collaborations were more salient in Engineering disciplines (n = 52), followed by “Humanities and Social Sciences” (n = 33) and “Business, Economics, and Management” (n = 18). Of the 107 articles with interdisciplinary collaboration, scholars from “Business, Economics and Management” and “Humanities and Social Sciences” collaborated in 57. Meanwhile, 51 articles show collaborations involving Engineering disciplines with other groups, such as “Business, Economics and Management,” “Humanities and Social Sciences,” and non-academic institutes.
On the affiliation level, tourism scholars were found to have contributed to 82 articles, with most collaborations centred around the “Business and Management discipline (e.g. Zhang et al., Citation2021). Other disciplines that lead intelligent automation in tourism research include computer science and information systems, which often collaborate with non-academic institutes (e.g. Allotta et al., Citation2015) or within the same Engineering discipline (e.g. Machidon et al., Citation2020). Disciplines such as Economics (e.g. Pelau et al., Citation2021) were found to have a similar number of collaborations with research fields such as autonomous systems (e.g. Duchetto et al., Citation2019). Finally, it is evident that many disciplines under the social sciences umbrella (e.g. psychology, sustainability, and communication) still have not been fully involved in intelligent automation in tourism discourse. To this end, this affiliation-by-affiliation collaboration map provides an important indicator that will enable future researchers to build collaborations so that they are able to progress the intelligent automation for the sustainable tourism research agenda.
Current landscape of intelligent automation research in sustainable tourism context
Thematic analysis was employed to identify key themes from the selected articles. It is an effective method for examining patterns within data and reporting them in extensive detail (Braun & Clarke, Citation2006). Guided by the sustainable tourism agenda (UNEP & UNWTO, 2005), this review identified five themes of intelligent automation research: a) Intelligent automation to enhance tourist experience (n = 86, 40.4%), b) Intelligent automation to preserve heritage (n = 49, 23%), c) Intelligent automation to promote quality of life (n = 37, 17.4%), d) Intelligent automation to measure tourist experience (n = 34, 15.9%), and e) Intelligent automation to conserve the environment (n = 7, 3.3%). Each of the themes has been discussed as follows.
Intelligent automation to enhance tourist experience
This research theme focuses on the tourist experience, which relates to the economic and social aspects of sustainability. The works under this theme address three aims for the sustainable tourism agenda: Economic Viability, Visitor Fulfillment, and Local Prosperity. UNEP and UNWTO (2005) suggest that Economic Viability emphasises the importance of paying attention to the overall competitiveness of the destination and its potential to deliver tourist satisfaction. One pattern identified from the reviewed literature is that tourism stakeholders have attempted to satisfy tourist expectations by introducing intelligent automation-powered services such as bellboy robots (Rodriguez-Lizundia et al., Citation2015) and robot baristas (Hwang et al., Citation2021). Tung and Au (Citation2018) noted that such novel services could encourage guests to co-create new experiences with intelligent automation-powered services. The guests sought ways to interact with such technologies to the extent that they would even purchase products only to be delivered by the robots.
Once the intelligent automation-powered services have been deployed, managers need to see whether the given services have been well-accepted by the public. This pertains to the Visitor Fulfillment aim, which involves monitoring and addressing tourist satisfaction and the quality of their experience (UNEP & UNWTO, 2005). To do this, various theories have been considered. For instance, the Uncanny Valley Theory reveals that the right degree of anthropomorphisation of AI agents will lead to a higher willingness to use them (Yang et al., Citation2022). Concurrently, the “Computers as Social Actors” paradigm suggests that even anthropomorphic characteristics alone will not significantly lead to a higher degree of acceptance unless the computers can show empathy and interact with the users properly (Pelau et al., Citation2021). Even when the public willingly accept intelligent automation-powered services, these technologies are still bound to experience technical failures. To cope with this possibility, Lv et al. (Citation2021) found how what may be perceived to be “cuteness” in AI agents can increase customer tolerance in a service failure, with tenderness as the mediating factor.
Lastly, a growing number of works address the Local Prosperity aim as these works seek to influence the levels of tourist spending by providing more effective marketing methods (UNEP & UNWTO, 2005). Zhang et al. (Citation2019) utilised AI-empowered computer vision for analysing 35,356 Flickr photos posted by tourists to explore their behaviour. The analysis revealed preference patterns from tourists from different continents (e.g. Asian tourists were found to like shopping more), extending the attribute-holistic framework of the destination image (Echtner & Ritchie, Citation1993). Such results could provide clues for destination marketing organisations to improve their marketing promotions. To conclude, this salient research theme focuses on the tourism industry’s economic efficiency and sociocultural aspects in order to deliver tourist satisfaction.
Intelligent automation to preserve heritage
This theme is associated with preserving heritage, including cultural sites, historic houses, museums, traditions, and natural heritage (UNEP & UNWTO, 2005). Previous works on this theme have addressed two aims for the sustainable tourism agenda. The first is Cultural Richness, which includes conserving historical and cultural heritage. A piece of evidence for this exists in the study of Allotta et al. (Citation2015), who developed an autonomous vehicle to investigate underwater cultural heritage. If successful, such investigations could result in the establishment of new tourist sites. Furthermore, since Cultural Richness also refers to effective visitor management (UNEP & UNWTO, 2005), studies that capture efforts to enhance the experience in and around the cultural heritage sites fall under this research theme. For instance, Rong et al. (Citation2020) developed a route for waterborne autonomous vehicles on the Amsterdam rivers connecting museums throughout the city. Their survey also showed that tourists preferred autonomous vehicles due to their flexibility and novelty despite the longer travel time. This not only contributes to cultural richness but also to crowd management in terms of reducing urban congestion by redistributing tourists within the destination.
The other aim is Physical Integrity, covering the importance of maintaining the facades of properties such as historic buildings (UNEP & UNWTO, 2005). Works such as Mansuri and Patel (Citation2022) advanced maintenance techniques by developing an AI system capable of inspecting defects on the surface of built heritage. With an optimal accuracy for detecting defects such as cracks and spalling, the technique can cut inspection costs by up to half or more compared to the manual method. These works primarily focus on developing new intelligent automation technologies but there are very few theoretically informed studies. Of these, Machidon et al. (Citation2020) applied theoretical models, namely the Technology Acceptance Model and the Software Usability Scale, to investigate users’ acceptance of a conversational agent introduced at museums. It can be concluded that the works on this theme touch on all three sustainability aspects.
Intelligent automation to promote quality of life
This theme is associated with promoting the quality of life of the people impacted by the deployment of intelligent automation technologies. Thirty-seven papers categorised under this research theme have addressed two other aims for the sustainable tourism agenda with much focus on the sociocultural aspects of sustainability. The first aim is Employment Quality, which is related to the wellbeing of employees whose jobs are at risk due to intelligent automation and advocates for employers to provide upskilling programmes at the workplace (UNEP & UNWTO, 2005). Such concerns are well addressed in many studies, with a study by Tuomi et al. (Citation2020) being one of the best examples. Using Grounded Theory to analyse qualitative data from industry practitioners across four countries, they developed the “Decent Work through Automation” model, offering guidelines for the responsible adoption of intelligent automation. It includes a comprehensive overview of the impact of intelligent automation adoption, such as the displacement of workers. The model further advocates the importance of upskilling plans among relevant stakeholders in order to contribute toward sustainability and human development.
The other aim is Community Wellbeing, which attempts to reduce congestion at tourist destinations (UNEP & UNWTO, 2005). Camatti et al. (Citation2020) developed an AI-based system to suggest alternative destinations to avoid overtourism in order to adhere to the tourism carrying capacity limit. This aim emphasises the significance of carefully planning and managing tourism facilities and infrastructure (UNEP & UNWTO, 2005). For instance, although autonomous vehicles can be expected to offer automated on-demand 24/7 services, their lack of surveillance features might result in violent crime and antisocial behaviour, such as passengers using drugs (Cohen & Hopkins, Citation2019). The discussion on the implications of intelligent automation adoption can better inform the development of socially beneficial AI in tourism, which is part of the Good AI Society agenda (Floridi et al., Citation2018). This theme is thus central to guiding the execution of the first theme (i.e. enhancing the tourist experience) because the irresponsible implementation of intelligent automation can lead to not only the mass substitution of human employment but also the erosion of consumer privacy (González-Rodríguez et al., Citation2020).
Intelligent automation to measure tourist experience
This theme focuses on the ability of intelligent automation technologies to measure the tourist experience. These AI-powered technologies serve as ways to help either collect or process a large amount of data. Measuring tourists’ experiences is pertinent to delivering evaluations after deploying intelligent automation and guiding service improvements (Xiang et al., Citation2021). The works under this theme have addressed three aims for the sustainable tourism agenda and focused on the economic and social aspects of sustainability. Just as the Community Wellbeing aim suggests the importance of managing tourism demand and reducing seasonality (UNEP & UNWTO, 2005), so research on tourism demand forecasting can assist tourism planners in making decisions and mitigating any risk based on the forecast data. One of the most recent AI-based forecasting techniques is the decomposed deep learning method developed by Zhang et al. (Citation2021). Such contributions help create effective short-term and long-term strategies and manage potential seasonality risks with better planning.
Economic Viability is another aim that is addressed when considering this theme. UNEP and UNWTO (2005) suggest that tourism managers should always find mechanisms for checking the visitor experience and obtaining regular feedback, which nowadays appears in online reviews. Wu et al. (Citation2021) utilised machine learning to examine online customer reviews of their experiences with robotic involvement in the service encounter. The thematic analysis results were used to develop a theoretical framework based on the service role theory and consumption value theory to inform practitioners of the importance of designing robot-offered services focusing on a value-generating business concept. Last of all, Visitor Fulfillment is addressed in this research theme, with several works advancing methodologies for monitoring tourist satisfaction (UNEP & UNWTO, 2005). González-Rodríguez et al. (Citation2020) developed a facial-expression recognition system to capture tourists’ emotions during guided tours at a UNESCO heritage site. By doing so, they could measure their satisfaction levels during the experience in a non-intrusive way.
Intelligent automation to conserve the environment
This theme is associated with the conservation of the environment through intelligent automation, thus addressing two specific aims in the sustainable tourism agenda. First, the Resource Efficiency aim covers how the use of scarce and non-renewable resources should be minimised to guarantee the sustainability of the industry (UNEP & UNWTO, 2005). Tussyadiah and Miller (Citation2019) investigated the potential use of AI-powered virtual agents and robots to promote resource-efficient behaviour on the part of tourists by providing feedback on their water and energy use during their hotel stay. Another study by Pereira-Doel et al. (Citation2019) used AI-powered water-saving technology to provide feedback to hotel guests during showering. Both studies were able to elicit pro-environmental behaviour through the feedback-giving mechanism.
Second, Environmental Purity focuses on the efforts to reduce the amount of pollution generated by the tourism industry (UNEP & UNWTO, 2005). Tan and Lin (Citation2020) revealed that tourists are likely to rent electric driverless cars at tourist destinations to reduce carbon emissions. Although the environmental impacts of electric vehicles still depend on the country’s electricity generation mix, a forward-looking study of electric vehicles in 59 world regions has found that emission reductions in the subsequent years will outweigh any temporary production-related increases (Knobloch et al., Citation2020). Further empirical validations are required to gain insights into the environmental impact of autonomous electric vehicles at tourist destinations.
As a theme focusing on the environmental aspects of sustainability, the limited scholarly attention this theme has received (i.e. only 3.3%) highlights the lack of progress that has been made to tackle environmental sustainability issues through intelligent automation (Gholami et al., Citation2016). With this in mind, transition pathways promoting a better distribution of sustainability focuses are needed to guide the future development of AI-based innovations. Transition pathways are helpful in informing researchers about how radical or disruptive novel innovations are required to be in order to generate the impact that stakeholders desire from the unfolding transitions (Lindberg et al., Citation2019). The above five themes inform the general sustainability focus of current intelligent automation technologies in tourism. The discussion unveils the current dominant focus on sustainability’s economic and social aspects and the lack of attention that has been paid to the environmental dimension. However, this mapping is insufficient to recommend the types of intelligent automation that future scholars and practitioners should aim to develop. There should be further mapping that takes into account the more technical characteristics of existing AI-based innovations. For instance, what should be the drivers behind technological innovation? Who should be the users of the innovation so that its sustainability impact can be augmented? The following section will outline a framework to guide the development of AI for the sustainability transition in tourism.
Conceptualising AI for sustainability transition in tourism: AI4GoodTourism framework
Recommending the types of technological innovation that future stakeholders should develop can be challenging. Designing innovative technological solutions requires clarity in both the objectives of the technology and the identity of its beneficiaries (Stickdorn & Schneider, Citation2011). However, the impacts of technological innovation are often unpredictable. For instance, social media innovations connecting celebrities’ lives with their followers have contributed to the adoption of “frequent flier” identities among young people (Gössling, Citation2019), thereby promoting the carbon-intensive lifestyle. This phenomenon provides rebound effects showing that improved efficiency can lead to increased consumption. How tourism is being experienced is, thus, key to transitioning the industry towards sustainability. In a capitalist economy, consumers are empowered to the extent that they can voice their preferences and achieve higher levels of utility (McShane & Sabadoz, Citation2015). As consumers of services provided by the tourism industry, tourists have the power to drive changes and play a crucial role in shaping the sustainability agenda (Juvan & Dolnicar, Citation2016). Thus, it is important to recognise their role as the main users when designing technological innovations (Stickdorn & Schneider, Citation2011).
To advocate for more sustainability-focused AI-based innovations, transition pathways need to be formulated. Informed by previous works (e.g. Lindberg et al., Citation2019), this research identified two dimensions, including sustainability inclusion and tourist involvement. While the former represents the objective of the technology, the latter represents its beneficiaries, which together form two essential elements for designing technological solutions (Stickdorn & Schneider, Citation2011). The sustainability inclusion dimension (low–high) assists in analysing the extent to which existing AI-based innovations incorporate the three pillars of sustainability: economic, social, and environmental (Butler, Citation1999). The other dimension, i.e. tourist involvement (low–high), is associated with the extent to which tourists interact with AI-based innovations to pursue their goals. The constant intersection between these dimensions justifies their selection as elements of the sustainability transition pathways. For instance, any negative tourist behaviour will harm the sustainability of the tourist destinations (Juvan & Dolnicar, Citation2016). Thus, the two dimensions are pertinent to investigating how AI-based innovations would help bring changes through intelligent automation for sustainable tourism development. Lindberg et al. (Citation2019) also argued that the application of such dimensions is appropriate when seeking to capture “the characteristics of an unfolding transition” of phenomena such as technological change in a particular industry.
As shown in , the degree of sustainability was considered high when the innovations went beyond product and service innovations and require changes to be made throughout the entire system over a period of time. Conversely, it was considered low when the innovations needed the incremental improvement of existing products and services concerning their environmental and social performance (Belz & Peattie, Citation2012). Regarding tourist involvement, it was considered high when there was direct interaction between tourists and the innovations. In contrast, tourist involvement was considered low when they had no direct interaction. The collective contribution made by pro-sustainable tourists will result in a large-scale drive for change. For instance, the water-saving technology in Pereira-Doel et al. (Citation2019) study, used in a 150-room hotel with two showers per room used daily for a month, will save the equivalent of one year’s water consumption based on that of an individual living in the United Kingdom. Therefore, this paper argues that the more tourists can use AI-based innovations with a higher degree of sustainability, then the more significant the positive socio-ecological impact that is being augmented will be. This contention is the key point to take from the developed sustainability transition pathways, which correspond to the Theory of Marginal Gains, stating that small changes across a system can be aggregated to create significant improvements (Alexander et al., Citation2019).
Figure 5. AI4GoodTourism framework: four pathways for AI-based innovations. De Carlo et al. (Citation2021): AI to design collaborative strategy Neumann et al. (Citation2014): Robot waiter Shi et al. (Citation2021): AI recommendation systems Tomo et al. (Citation2015): Puppet theatre robot Zhu & Chang (Citation2020): Robot chefs
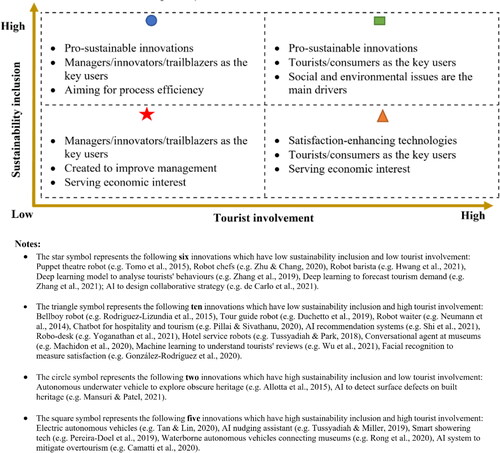
Building upon the proposed sustainability transition pathways and contextualising them for intelligent automation in tourism, further data analysis was conducted. All AI-based innovations in the retrieved articles were first coded and categorised based on their characteristics, resulting in 23 distinct AI-based innovations (see the “Notes” section above). They were further analysed and mapped onto the sustainability transition pathways. These coding processes resulted in the discovery of four quadrants with one quadrant (green square; see ) being the ideal AI-based solutions. This was developed into “AI4GoodTourism” framework, addressing the need of key stakeholders to receive guidance in the decision-making process for sustainable tourism development (Floridi et al., Citation2018). This framework encourages future applications of intelligent automation to be designed to promote sustainability inclusion through AI-based innovations in order to create large-scale impacts.
The mapping shows how current AI-based innovations are primarily considered to have low sustainability inclusion levels (i.e. 16 innovations in total). For instance, at least 42 papers discuss innovations such as service robots (e.g. Tussyadiah & Park., 2018), which amounts to nearly one-fifth of all contributions collected for this review. Such innovation has a low degree of sustainability inclusion, despite having the widespread involvement of tourists (see triangle quadrant in ). Whereas, AI-based innovations in the square quadrant are only evident in six papers, representing less than 3% of the collected literature. These innovations should be explored further if the tourism industry wants to progress the sustainable tourism agenda through intelligent automation (Gholami et al., Citation2016; Tussyadiah, Citation2020). Failure to understand how each AI-based innovation contributes to the sustainable tourism agenda will only exacerbate the paradox between pushing economic development through tourism sectors and its negative impact on environmental sustainability (Font & McCabe, Citation2017).
Conclusion and implications
A systematic review was conducted of 213 scholarly articles to map the current landscape of research on intelligent automation in the context of sustainable tourism. The review revealed five themes: intelligent automation to enhance tourist experience, preserve heritage, promote quality of life, measure tourist experience, and conserve the environment. Of these, the most dominant research focus was on using intelligent automation to enhance the tourist experience while the least dominant focus was on its use as a means to conserve the environment. After developing the sustainability transition pathways, this paper mapped selected AI-based innovations through the pathways and found different levels of engagement in terms of sustainability inclusion and tourist involvement. The finding informs our understanding of how existing AI-based innovations focus on sustainability’s economic and social aspects while neglecting environmental sustainability. It echoes the criticism aimed at the technological advancement trend in the Industry 4.0′ era, which focuses less on environmental sustainability issues (Maddikunta et al., Citation2022). Due to this oversight, scholars advocating for the upcoming Industry 5.0 have called for more sustainability-focused technological innovations (Maddikunta et al., Citation2022).
Responding to this call, this paper introduces the “AI4GoodTourism” framework to help guide the future adoption of intelligent automation in tourism. It enriches the discourse of “AI4People” put forward by Floridi et al. (Citation2018). With this framework, it is expected that future scholars will develop more theories that will help to evaluate the impact of certain AI-based innovations on the sustainability of the tourism industry, precisely the environmental aspect. For instance, technology acceptance theories such as Artificial Intelligence Device Use Acceptance (e.g. Chi et al., Citation2022) can be further expanded to include sustainability-related factors. Such inclusion could help evaluate how the technology adoption among users might impact sustainability in tourism. Currently, most studies investigating adoption levels still have not included such factors (e.g. Pillai & Sivathanu, Citation2020).
Three key future research directions can be drawn from macro, micro, and methodological perspectives. At a macro level, future research can ensure that careful impact analyses can always accompany the application of the AI4GoodTourism framework to alleviate negative consequences resulting from promoting sustainability through AI-based innovations (Hoogendoorn et al., Citation2019). The focus should be developing applications that leverage intelligent automation in a socially preferable, environmentally friendly way (Floridi et al., Citation2018) To achieve this, theories from tourism planning and governance, such as stakeholder theory (Khazaei et al., Citation2015) and the political theory of action (Jamal & Watt, Citation2011) can be explored. This is to ensure that relevant stakeholders, including the local community, can be included in designing and applying AI-based innovations. The more macro social theory, such as the strategic-relational political economy approach (Bramwell, Citation2011), might also be helpful to shed light on how the state should redirect its focus from economic to environmental concerns in order to govern the tourism industry better. This may result in more AI-based innovations with positive impacts on environmental sustainability. Should these theories be applied well, it is to be expected that the three quadrants (i.e. circle, star, and triangle) in the AI4GoodTourism framework will be improved.
From the micro perspective, tourism scholars and practitioners should strive to invent, develop, and adopt AI-based technological innovations that are not only sustainability-oriented but can involve a significant number of tourists in achieving their goals (Dolnicar, Citation2020). For instance, ChatGPT may allow scholars and practitioners to enhance the capabilities of AI-based innovations identified as having low sustainability inclusion but high tourist involvement, such as robo-desk (e.g. Yoganathan et al., Citation2021), by enabling the technologies to educate the tourists on how to become more sustainable while travelling (Loureiro et al., Citation2022). This application would employ “nudge theory” to change the tourists’ behaviour by using messages without significantly changing the economic incentives (Thaler & Sunstein, Citation2008). In addition, there is a need for exploring the implications of AI-based innovation for post-adoption behaviours and potential spillover effects of pro-environment behaviours in order to improve sustainability inclusion in line with promoting tourist involvement.
From the methodological perspective, innovative tools such as biometric research using AI-powered software like iMotions (Tussyadiah & Park, Citation2018) could help to advance efforts to make automation intelligent and influence tourist behaviour and attitudes toward sustainability. Extant research has focused on behavioural intentions and perceptions and actual behavourial outcomes need to be examined further to capture the real impact of AI-based innovation on sustainable tourism. Therefore, harnessing the power of different methodological lenses in computation, experimental design, and both quantitative and qualitative approaches is warranted to ensure the production of successful automation and users’ acceptance of new technological innovations. This will advance knowledge about the best way of developing AI-based innovations and their theoretical groundings in the field of sustainable tourism.
Furthermore, informed by the disciplinary collaborations shown in , this paper highlights the importance of identifying potential collaborations when seeking to develop and implement AI-based technologies with high levels of sustainability and tourist involvement.hows that disciplines such as psychology, sustainability, communication, and environmental sciences are still at an early stage in their involvement in intelligent automation in tourism research. The expertise of those working in these disciplines would benefit scholars and practitioners from computer science, information technology, or tourism backgrounds in enabling them to develop technology that could have substantial sustainability implications. The technology will have positive implications for the tourist involvement dimensions and significantly impact the tourists (e.g. Pereira-Doel et al., Citation2019).
Non-academic institutes (e.g. Commonwealth Bank in Herse et al., Citation2018) and scholars from disciplines such as physics, mathematics, health and medical science can be invited to advance research in this area further. The topics might include how AI can improve forecasting methods (e.g. Zhang et al., Citation2021) or how AI can enhance the tourist experience in the field of medical tourism (Viladrich & Baron-Faust, Citation2014). These topics are strongly relevant since COVID-19 has unveiled how unprepared the tourism industry was when facing the crisis (Yang et al., Citation2021). Moving forward, the ongoing energy crisis and climate change (Scott & Gössling, Citation2022) will most likely serve as the ultimate catalyst for further research on AI for sustainability in tourism and become the next research focus after COVID-19 (see ).
For example, promoting electric vehicles in tourism, whether waterborne (Rong et al., Citation2020) or driverless cars (Tan & Lin, Citation2020), would lead the industry towards environmental sustainability. Prideaux and Yin (Citation2019) emphasised the need for tourism academics to lead the sector towards low-carbon tourism mobility. Research has estimated that there will be a reduction of 4.7 billion tonnes of carbon emissions if 90% of cars are electric by 2040 (Prideaux & Yin, Citation2019). To achieve this, contributions from less represented fields of research and disciplines such as computer vision, Internet-of-Things, mechanical engineering, transportation, consumer sciences, statistics, and mathematics would be vital for helping tourism stakeholders introduce more electric vehicles. Roboat, the waterborne autonomous vehicle (Rong et al., Citation2020), is an excellent example of collaboration between scholars with diverse expertise benefitting the tourism industry. With the emergence of such technologies, further research should be carried out to investigate how tourists would adopt such new vehicle types. It will then be possible to consider the positive impact of such innovations towards environmental sustainability.
Academics with sustainable tourism expertise should lead in forming collaborations with other disciplines to address issues relating to developing sustainable tourism. Camatti et al. (Citation2020) aimed to tackle overtourism issues in cooperation with scholars from the fields of economics and geography. Undoubtedly, managing overtourism would have implications for the area’s economy (e.g. by directing tourists to other less busy areas) and need high-precision geolocation mapping to propose the best solution. In the post-COVID-19 era, overtourism can be related to health and safety concerns. AI applications could help to identify the best ways to achieve efficient crowd management and destination planning. Developing AI solutions to mitigate risks such as unpredictable viral epidemics would significantly impact the social sustainability dimensions, as the solutions would save people’s lives. Such endeavours may involve scholars from natural sciences, health and medical sciences, and even food science.
Lastly, future scholars can reflect on three unaddressed aims for the sustainable tourism agenda (UNEP & UNWTO, 2005), including Social Equity, Local Control and Biological Diversity. The Social Equity aim can be addressed with AI-based innovations created to serve disadvantaged people and marginalised communities (Khazaei et al., Citation2015) and support social tourism programmes, such as voluntourism and community-based tourism. The Local Control aim may be covered in AI-based innovations that would allow local indigenous communities more control over their resources, enabling more equitable development of communities (Jamal & Watt, Citation2011). Scholars can address the Biological Diversity aim by inventing AI-based solutions that would help safeguard biodiversity and natural areas in tourist destinations. One noticeable pattern reveals that these three issues would benefit from the expertise of less-represented disciplines, such as anthropology, gender studies, public governance, and journalism. Such interdisciplinary works, with stakeholder-inclusive and participatory design approaches, are essential for determining better-promoting solutions for sustainability problems (Zhang et al., Citation2020). To this end, the framework introduced by UNEP and UNWTO (2005) has been useful for mapping the need for AI solutions to help accomplish the proposed sustainable tourism actions in the intelligent automation era.
Overall, this paper makes two significant contributions. On the theoretical front, scholars whose research interests are in the intersection between tourism, technology, and sustainability will benefit from the sustainability transition pathways, AI4GoodTourism framework. The framework theoretically suggests the types of AI-based innovations that can be investigated in future research. Recommendations for potential disciplinary collaboration proposed in this paper will further guide scholars in pursuing the proposed research directions. On the practical front, practitioners such as destination managers, business leaders, and technology developers might use the research findings to drive the future development and adoption of AI solutions. They need to develop AI solutions strategically by focusing on their impact on sustainability and tourists. The sustainability transition pathways can act as a framework to guide the transition of the tourism industry to sustainability through intelligent automation. These contributions respond to the call for a new framework to drive technology adoption and promote sustainability in tourism (Yang et al., Citation2021). Concerning the SDGs, the overall discussion presented in this paper reflects, but is not limited to, SDG9 (Innovation), SDG13 (Climate Action), and SDG17 (Partnership for the Goals). Finally, this paper calls for future research on how intelligent automation can address sustainability challenges in the tourism industry.
Supplemental Material
Download MS Word (92.3 KB)Disclosure statement
No potential conflict of interest was reported by the authors.
Data availability statement
Data can be made available upon request.
Correction Statement
This article has been corrected with minor changes. These changes do not impact the academic content of the article.
Additional information
Funding
Notes on contributors
Gilang Maulana Majid
Gilang Maulana Majid is a PhD researcher at School of Hospitality and Tourism Management (SHTM), University of Surrey. His research interests cover technology and sustainability.
Iis Tussyadiah
Iis Tussyadiah is a Professor at SHTM, University of Surrey. Her research focuses on technology and behaviour.
Yoo Ri Kim
Yoo Ri Kim is an Assistant Professor in Hospitality Management at the Rosen College of Hospitality Management, University of Central Florida. Her research interests include applied economics and innovation.
Anjan Pal
Anjan Pal is a Lecturer at the School for Business and Society, University of York. His research interests cover digital marketing and online consumer behaviour.
References
- Alexander, P., Reddy, A., Brown, C., Henry, R., & Rounsevell, M. (2019). Transforming agricultural land use through marginal gains in the food system. Global Environmental Change, 57, 101932. https://doi.org/10.1016/j.gloenvcha.2019.101932
- Allotta, B., Baines, S., Bartolini, F., Bellavia, F., Colombo, C., Conti, R., Costanzi, R., Dede, C., Fanfani, M., Gelli, J., Gundogdu, H., Monni, N., Moroni, D., Natalini, M., Pascali, M., Pazzaglia, F., Pugi, L., Ridolfi, A., Reggiannini, M., … Tekdemir, E. (2015). Design of a modular Autonomous Underwater Vehicle for archaeological investigations [Paper presentation]. MTS/IEEE Oceans, 2015. https://doi.org/10.1109/OCEANS-Genova.2015.7271398
- Aria, M., & Cuccurullo, C. (2017). bibliometrix: An R-tool for comprehensive science mapping analysis. Journal of Informetrics, 11(4), 959–975. https://doi.org/10.1016/j.joi.2017.08.007
- Belz, F., & Peattie, K. (2012). Sustainability marketing: A global perspective (2nd ed.). Wiley.
- Bramwell, B. (2011). Governance, the state and sustainable tourism: A political economy approach. Journal of Sustainable Tourism, 19(4–5), 459–477. https://doi.org/10.1080/09669582.2011.576765
- Braun, V., & Clarke, V. (2006). Using thematic analysis in psychology. Qualitative Research in Psychology, 3(2), 77–101. https://doi.org/10.1191/1478088706qp063oa
- Butler, R. (1999). Sustainable tourism: A state-of-the-art review. Tourism Geographies, 1(1), 7–25. https://doi.org/10.1080/14616689908721291
- Camatti, N., Bertocchi, D., Carić, H., & van der Borg, J. (2020). A digital response system to mitigate overtourism. The case of Dubrovnik. Journal of Travel & Tourism Marketing, 37(8–9), 887–901. https://doi.org/10.1080/10548408.2020.1828230
- Cambridge Intelligence. (2020). Social network analysis 101: Centrality measures explained. https://cambridge-intelligence.com/keylines-faqs-social-network-analysis/
- Carvalho, I., & Ivanov, S. (2023). ChatGPT for tourism: Application, benefits and risks. Tourism Review, Vol. ahead-of-print. No. ahead-of-print. https://doi.org/10.1108/TR-02-2023-0088
- Chi, O., Gursoy, D., & Chi, C. (2022). Tourists’ attitudes toward the use of artificially intelligent (AI) devices in tourism service delivery: Moderating role of service value seeking. Journal of Travel Research, 61(1), 170–185. https://doi.org/10.1177/0047287520971054
- Cohen, S., & Hopkins, D. (2019). Autonomous vehicles and the future of urban tourism. Annals of Tourism Research, 74, 33–42. https://doi.org/10.1016/j.annals.2018.10.009
- Culot, G., Nassimbeni, G., Orzes, G., & Sartor, M. (2020). Behind the definition of Industry 4.0: Analysis and open questions. International Journal of Production Economics, 226, 107617. https://doi.org/10.1016/j.ijpe.2020.107617
- De Carlo, M., Ferilli, G., d’Angella, F., & Buscema, M. (2021). Artificial intelligence to design collaborative strategy: An application to urban destinations. Journal of Business Research, 129, 936–948. https://doi.org/10.1016/j.jbusres.2020.09.013
- Dolnicar, S. (2020). Designing for more environmentally friendly tourism. Annals of Tourism Research, 84, 102933. https://doi.org/10.1016/j.annals.2020.102933
- Duchetto, F. d., Baxter, P., & Hanheide, M. (2019). Lindsey the tour guide robot - usage patterns in a museum long-term deployment [Paper presentation]. 28th IEEE International Conference on Robot and Human Interactive Communication. https://doi.org/10.1109/RO-MAN46459.2019.8956329
- Echtner, C., & Ritchie, J. (1993). The measurement of destination image: An empirical assessment. Journal of Travel Research, 31(4), 3–13. https://doi.org/10.1177/004728759303100402
- Floridi, L., Cowls, J., Beltrametti, M., Chatila, R., Chazerand, P., Dignum, V., Luetge, C., Madelin, R., Pagallo, U., Rossi, F., Schafer, B., Valcke, P., & Vayena, E. (2018). AI4People—An ethical framework for a good AI society: Opportunities, risks, principles, and recommendations. Minds and Machines, 28(4), 689–707. https://doi.org/10.1007/s11023-018-9482-5
- Font, X., & McCabe, S. (2017). Sustainability and marketing in tourism: Its contexts, paradoxes, approaches, challenges and potential. Journal of Sustainable Tourism, 25(7), 869–883. https://doi.org/10.1080/09669582.2017.1301721
- Gholami, R., Watson, R., Hasan, H., Molla, A., & Bjorn-Andersen, N. (2016). Information systems solutions for environmental sustainability: How can we do more? Journal of the Association for Information Systems, 17(8), 521–536. https://doi.org/10.17705/1jais.00435
- González-Rodríguez, M., Díaz-Fernández, M., & Pacheco Gómez, C. (2020). Facial-expression recognition: An emergent approach to the measurement of tourist satisfaction through emotions. Telematics and Informatics, 51, 101404. https://doi.org/10.1016/j.tele.2020.101404
- Gössling, S. (2019). Celebrities, air travel and social norms. Annals of Tourism Research, 79, 102775. https://doi.org/10.1016/j.annals.2019.102775
- Gössling, S. (2021). Technology, ICT and tourism: From big data to the big picture. Journal of Sustainable Tourism, 29(5), 849–858. https://doi.org/10.1080/09669582.2020.1865387
- Gössling, S., Scott, D., & Hall, C. (2021). Pandemics, tourism and global change: A rapid assessment of COVID-19. Journal of Sustainable Tourism, 29(1), 1–20. https://doi.org/10.1080/09669582.2020.1758708
- Herse, S., Vitale, J., Tonkin, M., Ebrahimian, D., Ojha, S., Johnson, B., Judge, W., & Williams, M. (2018). Do you trust me, blindly? Factors influencing trust towards a robot recommender system. In 27th IEEE International Symposium on Robot and Human Interactive Communication. https://doi.org/10.1109/ROMAN.2018.8525581
- Hoogendoorn, G., Sütterlin, B., & Siegrist, M. (2019). When good intentions go bad: The biased perception of the environmental impact of a behavior due to reliance on an actor’s behavioral intention. Journal of Environmental Psychology, 64, 65–77. https://doi.org/10.1016/j.jenvp.2019.05.003
- Hwang, J., Choe, J., Kim, H. M., & Kim, J. (2021). The antecedents and consequences of memorable brand experience: Human baristas versus robot baristas. Journal of Hospitality and Tourism Management, 48, 561–571. https://doi.org/10.1016/j.jhtm.2021.08.013
- Ivanov, S., & Webster, C. (2019). Robots, artificial intelligence, and service automation in travel, tourism and hospitality (1st ed.). Emerald Publishing Limited.
- Ivanov, S., Webster, C., Stoilova, E., & Slobodskoy, D. (2022). Biosecurity, crisis management, automation technologies and economic performance of travel, tourism and hospitality companies – A conceptual framework. Tourism Economics, 28(1), 3–26. https://doi.org/10.1177/1354816620946541
- Jamal, T., & Watt, M. (2011). Climate change pedagogy and performative action: Toward community-based destination governance. Journal of Sustainable Tourism, 19(4–5), 571–588. https://doi.org/10.1080/09669582.2011.575227
- Juvan, E., & Dolnicar, S. (2016). Measuring environmentally sustainable tourist behaviour. Annals of Tourism Research, 59, 30–44. https://doi.org/10.1016/j.annals.2016.03.006
- Khazaei, A., Elliot, S., & Joppe, M. (2015). An application of stakeholder theory to advance community participation in tourism planning: The case for engaging immigrants as fringe stakeholders. Journal of Sustainable Tourism, 23(7), 1049–1062. https://doi.org/10.1080/09669582.2015.1042481
- Kim, Y. R., Liu, A., & Williams, A. (2022). Competitiveness in the visitor economy: A systematic literature review. Tourism Economics, 28(3), 817–842. https://doi.org/10.1177/13548166211034437
- Kitchenham, B., & Charters, S. (2007). Guidelines for performing systematic literature reviews in software engineering.
- Knobloch, F., Hanssen, S., Lam, A., Pollitt, H., Salas, P., Chewpreecha, U., Huijbregts, M., & Mercure, J. (2020). Net emission reductions from electric cars and heat pumps in 59 world regions over time. Nature Sustainability, 3(6), 437–447. https://doi.org/10.1038/s41893-020-0488-7
- Lee, Y., Lee, Y., Seong, J., Stanescu, A., & Hwang, C. (2020). A comparison of network clustering algorithms in keyword network analysis: A case study with geography conference presentations. International Journal of Geospatial and Environmental Research, 7(3), 1–14.
- Lindberg, M., Markard, J., & Andersen, A. (2019). Policies, actors and sustainability transition pathways: A study of the EU’s energy policy mix. Research Policy, 48(10), 103668. https://doi.org/10.1016/j.respol.2018.09.003
- Liu, Z. (2003). Sustainable tourism development: A critique. Journal of Sustainable Tourism, 11(6), 459–475. https://doi.org/10.1080/09669580308667216
- Loureiro, S. M. C., Guerreiro, J., & Han, H. (2022). Past, present, and future of pro-environmental behavior in tourism and hospitality: A text-mining approach. Journal of Sustainable Tourism, 30(1), 258–278. https://doi.org/10.1080/09669582.2021.1875477
- Lv, X., Liu, Y., Luo, J., Liu, Y., & Li, C. (2021). Does a cute artificial intelligence assistant soften the blow? The impact of cuteness on customer tolerance of assistant service failure. Annals of Tourism Research, 87, 103114. https://doi.org/10.1016/j.annals.2020.103114
- Machidon, O., Tavčar, A., Gams, M., & Duguleană, M. (2020). CulturalERICA: A conversational agent improving the exploration of European cultural heritage. Journal of Cultural Heritage, 41, 152–165. https://doi.org/10.1016/j.culher.2019.07.010
- Maddikunta, P. K. R., Pham, Q.-V., B, P., Deepa, N., Dev, K., Gadekallu, T. R., Ruby, R., & Liyanage, M. (2022). Industry 5.0: A survey on enabling technologies and potential applications. Journal of Industrial Information Integration, 26, 100257. https://doi.org/10.1016/j.jii.2021.100257
- Mansuri, L., & Patel, D. (2022). Artificial intelligence-based automatic visual inspection system for built heritage. Smart and Sustainable Built Environment, 11(3), 622–646. https://doi.org/10.1108/SASBE-09-2020-0139
- McShane, L., & Sabadoz, C. (2015). Rethinking the concept of consumer empowerment: Recognizing consumers as citizens. International Journal of Consumer Studies, 39(5), 544–551. https://doi.org/10.1111/ijcs.12186
- Moyle, B., Moyle, C., Ruhanen, L., Weaver, D., & Hadinejad, A. (2021). Are we really progressing sustainable tourism research? A bibliometric analysis. Journal of Sustainable Tourism, 29(1), 106–122. https://doi.org/10.1080/09669582.2020.1817048
- Neumann, B., Hotz, L., Rost, P., & Lehmann, J. (2014). A robot waiter learning from experiences. In Machine Learning and Data Mining in Pattern Recognition: 10th International Conference, MLDM 2014, St. Petersburg, Russia, pp. 285–299. https://doi.org/10.1007/978-3-319-08979-9_22
- Page, M. J., McKenzie, J. E., Bossuyt, P. M., Boutron, I., Hoffmann, T. C., Mulrow, C. D., Shamseer, L., Tetzlaff, J. M., Akl, E. A., Brennan, S. E., Chou, R., Glanville, J., Grimshaw, J. M., Hróbjartsson, A., Lalu, M. M., Li, T., Loder, E. W., Mayo-Wilson, E., McDonald, S., … Moher, D. (2021). The PRISMA 2020 statement: An updated guideline for reporting systematic reviews. Systematic Reviews, 10(89), 1–11. https://doi.org/10.1186/s13643-021-01626-4
- Pal, A., & Chua, A. (2016). Reviewing the landscape of research on the threats to the quality of user-generated content. Proceedings of the Association for Information Science and Technology, 53(1), 1–9. https://doi.org/10.1002/pra2.2016.14505301077
- Pelau, C., Dabija, D., & Ene, I. (2021). What makes an AI device human-like? The role of interaction quality, empathy and perceived psychological anthropomorphic characteristics in the acceptance of artificial intelligence in the service industry. Computers in Human Behavior, 122, 106855. https://doi.org/10.1016/j.chb.2021.106855
- Pereira-Doel, P., Font, X., Wyles, K., & Pereira-Moliner, J. (2019). Showering smartly. A field experiment using water-saving technology to foster pro-environmental behaviour among hotel guests. e-Review of Tourism Research, 17(3), 407–425. http://ertr.tamu.edu
- Pillai, R., & Sivathanu, B. (2020). Adoption of AI-based chatbots for hospitality and tourism. International Journal of Contemporary Hospitality Management, 32(10), 3199–3226. https://doi.org/10.1108/IJCHM-04-2020-0259
- Pillai, S., Haldorai, K., Seo, W., & Kim, W. (2021). COVID-19 and hospitality 5.0: Redefining hospitality operations. International Journal of Hospitality Management, 94, 102869. https://doi.org/10.1016/j.ijhm.2021.102869
- Prideaux, B., & Yin, P. (2019). The disruptive potential of autonomous vehicles (AVs) on future low-carbon tourism mobility. Asia Pacific Journal of Tourism Research, 24(5), 459–467. https://doi.org/10.1080/10941665.2019.1588138
- Rahmadian, E., Feitosa, D., & Zwitter, A. (2022). A systematic literature review on the use of big data for sustainable tourism. Current Issues in Tourism, 25(11), 1711–1730. https://doi.org/10.1080/13683500.2021.1974358
- Rodriguez-Lizundia, E., Marcos, S., Zalama, E., Gómez-García-Bermejo, J., & Gordaliza, A. (2015). A bellboy robot: Study of the effects of robot behaviour on user engagement and comfort. International Journal of Human-Computer Studies, 82, 83–95. https://doi.org/10.1016/j.ijhcs.2015.06.001
- Rong, H., Tu, W., Duarte, F., & Ratti, C. (2020). Employing waterborne autonomous vehicles for museum visits: A case study in Amsterdam. European Transport Research Review, 12(63), 1–13. https://doi.org/10.1186/s12544-020-00459-x
- Roxas, F., Rivera, J., & Gutierrez, E. (2020). Framework for creating sustainable tourism using systems thinking. Current Issues in Tourism, 23(3), 280–296. https://doi.org/10.1080/13683500.2018.1534805
- Russell, S., & Norvig, P. (2021). Artificial intelligence: A modern approach (4th ed.). Pearson Education.
- Santos, V., Sousa, M., Costa, C., & Au-Yong-Oliveira, M. (2021). Tourism towards sustainability and innovation: A systematic literature review. Sustainability, 13(20), 11440. https://doi.org/10.3390/su132011440
- Scott, D., & Gössling, S. (2022). A review of research into tourism and climate change – Launching the annals of tourism research curated collection on tourism and climate change. Annals of Tourism Research, 95, 103409. https://doi.org/10.1016/j.annals.2022.103409
- Shi, S., Gong, Y., & Gursoy, D. (2021). Antecedents of trust and adoption intention toward artificially intelligent recommendation systems in travel planning: A heuristic–systematic model. Journal of Travel Research, 60(8), 1714–1734. https://doi.org/10.1177/0047287520966395
- Stickdorn, M., & Schneider, J. (2011). This is service design thinking: Basics, tools, cases. BIS Publisher.
- Stoddard, J., Pollard, C., & Evans, M. (2012). The triple bottom line: A framework for sustainable tourism development. International Journal of Hospitality & Tourism Administration, 13(3), 233–258. https://doi.org/10.1080/15256480.2012.698173
- Tan, W., & Lin, C. (2020). Driverless car rental at tourist destinations: From the tourists’ perspective. Asia Pacific Journal of Tourism Research, 25(11), 1153–1167. https://doi.org/10.1080/10941665.2020.1825007
- Thaler, R., & Sunstein, C. (2008). Nudge: Improving decisions about health, wealth, and happiness. Yale University Press.
- Tomo, T., Enriquez, G., & Hashimoto, S. (2015). Indonesian puppet theater robot with gamelan music emotion recognition [Paper presentation]. IEEE International Conference on Robotics and Biomimetics. https://doi.org/10.1109/ROBIO.2015.7418931
- Tung, V., & Au, N. (2018). Exploring customer experiences with robotics in hospitality. International Journal of Contemporary Hospitality Management, 30(7), 2680–2697. https://doi.org/10.1108/IJCHM-06-2017-0322
- Tuomi, A., & Ascenção, M. (2023). Intelligent automation in hospitality: Exploring the relative automatability of frontline food service tasks. Journal of Hospitality and Tourism Insights, 6(1), 151–173. https://doi.org/10.1108/JHTI-07-2021-0175
- Tuomi, A., Tussyadiah, I., & Stienmetz, J. (2021). Applications and Implications of Service Robots in Hospitality. Cornell Hospitality Quarterly, 62(2), 232–247. https://doi.org/10.1177/1938965520923961
- Tuomi, A., Tussyadiah, I., Ling, E., Miller, G., & Lee, G. (2020). x=(tourism_work) y=(sdg8) while y = true: Automate(x). Annals of Tourism Research, 84, 102978. https://doi.org/10.1016/j.annals.2020.102978
- Tussyadiah, I. (2020). A review of research into automation in tourism: Launching the Annals of Tourism Research Curated Collection on Artificial Intelligence and Robotics in Tourism. Annals of Tourism Research, 81, 102883. https://doi.org/10.1016/j.annals.2020.102883
- Tussyadiah, I., & Miller, G. (2019). Nudged by a robot: Responses to agency and feedback. Annals of Tourism Research, 78, 102752. https://doi.org/10.1016/j.annals.2019.102752
- Tussyadiah, I., & Park, S. (2018, January 24–26). Consumer evaluation of hotel service robots. In Information and Communication Technologies in Tourism 2018: Proceedings of the International Conference, Jönköping, Sweden (pp. 308–320). Springer International Publishing. https://doi.org/10.1007/978-3-319-72923-7_24
- UNEP, & WTO. (2005). Making tourism more sustainable (English version). United Nations Environment Programme and World Tourism Organization. https://doi.org/10.18111/9789284408214
- Viladrich, A., & Baron-Faust, R. (2014). Medical tourism in tango paradise: The internet branding of cosmetic surgery in Argentine. Annals of Tourism Research, 45, 116–131. https://doi.org/10.1016/j.annals.2013.12.007
- Weaver, D. (2005). Sustainable tourism: Theory and practice. Elsevier.
- Wohlin, C. (2014). Guidelines for snowballing in systematic literature studies and a replication in software engineering [Paper presentation]. ACM International Conference Proceeding Series, pp. 1–10. https://doi.org/10.1145/2601248.2601268
- Wu, L., Fan, A., Yang, Y., & He, Z. (2021). Robotic involvement in the service encounter: A value-centric experience framework and empirical validation. Journal of Service Management, 32(5), 783–812. https://doi.org/10.1108/JOSM-12-2020-0448
- Xiang, Z., Stienmetz, J., & Fesenmaier, D. (2021). Smart Tourism Design: Launching the annals of tourism research curated collection on designing tourism places. Annals of Tourism Research, 86, 103154. https://doi.org/10.1016/j.annals.2021.103154
- Yang, Y., Liu, Y., Lv, X., Ai, J., & Li, Y. (2022). Anthropomorphism and customers’ willingness to use artificial intelligence service agents. Journal of Hospitality Marketing & Management, 31(1), 1–23. https://doi.org/10.1080/19368623.2021.1926037
- Yang, Y., Zhang, C., & Rickly, J. (2021). A review of early COVID-19 research in tourism: Launching Annals of Tourism Research’s Curated Collection on coronavirus and tourism. Annals of Tourism Research, 91, 103313. https://doi.org/10.1016/j.annals.2021.103313
- Yin, L., Kretschmer, H., Hanneman, R., & Liu, Z. (2006). Connection and stratification in research collaboration: An analysis of the COLLNET network. Information Processing & Management, 42(6), 1599–1613. https://doi.org/10.1016/j.ipm.2006.03.021
- Yoganathan, V., Osburg, V., Kunz, W., & Toporowski, W. (2021). Check-in at the Robo-desk: Effects of automated social presence on social cognition and service implications. Tourism Management, 85, 104309. https://doi.org/10.1016/j.tourman.2021.104309
- Zhang, J., Oh, Y., Lange, P., Yu, Z., & Fukuoka, Y. (2020). Artificial intelligence chatbot behavior change model for designing artificial intelligence chatbots to promote physical activity and a healthy diet: Viewpoint. Journal of Medical Internet Research, 22(9), e22845. https://doi.org/10.2196/22845
- Zhang, K., Chen, Y., & Li, C. (2019). Discovering the tourists’ behaviors and perceptions in a tourism destination by analyzing photos’ visual content with a computer deep learning model: The case of Beijing. Tourism Management, 75, 595–608. https://doi.org/10.1016/j.tourman.2019.07.002
- Zhang, Y., Li, G., Muskat, B., & Law, R. (2021). Tourism demand forecasting: A decomposed deep learning approach. Journal of Travel Research, 60(5), 981–997. https://doi.org/10.1177/0047287520919522
- Zhu, D., & Chang, Y. (2020). Robot with humanoid hands cooks food better?: Effect of robotic chef anthropomorphism on food quality prediction. International Journal of Contemporary Hospitality Management, 32(3), 1367–1383. https://doi.org/10.1108/IJCHM-10-2019-0904