Abstract
The effects of five ions, ,
, K+, Na+ and Cl−, on growth rates were quantified for Chlorella vulgaris (Trebouxiophyceae) and Peridinium cinctum (Dinophyceae) in batch cultures. A five-dimensional, experimental design hypervolume was defined by five-ion mixture vectors projected across a total ion concentration gradient of 1 to 30 mM to quantify growth rates in relation to N:P ratios and primary bulk solution ions. Both C. vulgaris and P. cinctum exhibited very similar, positive responses to cations and negative responses to anions. It was determined that total ion concentration for these five ions, from 1 to 30 mM, did not directly affect growth rate for either alga, although it did play an interactive role with several ions. No truly ‘optimal’ N : P ratio was identified for either alga. Instead, regions of fast growth rates were mapped within the multivariate experimental design space, or ‘hypervolumes’, that encompassed relatively broad ranges of N:P ratios. This illustrates the fact that optimality is highly contextual and directly dependent on the total ion complement of any given medium. Our results also show that pH is a poor indicator of growth medium quality and should not be considered an independent factor. This study is the first set of unconfounded, ion-specific experiments with algae that we are aware of. The data suggest that we need to reconsider the discussion surrounding ‘optimal’ ratios and concentrations of nutrients/ions in relation to growth to encompass the hyperdimensionality of media defined by multiple ion vectors. This ‘ionic context’ is extremely important to algal physiology, has broad implications for questions of nutrient requirements, and needs to be further explored.
Introduction
A central component of algal biology is ion-specific effects, e.g. nutrient ratios, osmotic potentials, salinity effects, pH, salts, total ion concentrations. Questions surrounding these issues are particularly difficult to address with aquatic algae, because many ions serve as essential nutrients and can also play roles in determining physico-chemical parameters of growth media. Algae have differing sensitivities to various ions, both in type effects such as chloride sensitivity and concentration effects such as salinity tolerance. These sensitivities affect many fundamental concerns in nutrient acquisition and primary productivity, and consequently play driving roles in issues of ‘bottom-up’ control of community ecology.
Our understanding of the importance of nutrient ratios, primarily (N) and
(P), in relation to microalgal growth and growth rate (primary production and productivity, respectively) can be traced directly to Redfield's (Citation1934) seminal work when he quantified the elemental ratios of marine phytoplankton in relation to the prevailing stoichiometry of the world's oceans. The N:P ratio of 16:1, the ‘Redfield ratio’, is often used as a benchmark for differentiating N-limited growth from P-limited growth, and is thought to set an upper limit on the nitrate:phosphate ratio in the ocean (Geider & La Roche, Citation2002), with N-limitation occurring at N : P < 16 and P-limitation occurring at N : P > 16 (Tyrrell & Taylor, Citation1996; Falkowski, Citation1997).
The question of nutrient ratio effects on growth rates has been examined in laboratory and field settings; the primary methodologies employed are (1) correlational analyses based on field observations of species composition and water chemistry and (2) laboratory/field-based experiments where nitrogen and/or phosphate salts are employed to manipulate the relative concentrations of N and/or P (e.g. Rhee, Citation1978; Leonardos & Geider, Citation2005; Schindler et al., Citation2008). In these manipulative experiments, growth rates are measured and plotted against their respective N:P values and conclusions concerning ‘optimal N:P ratios’ are drawn. There are two primary reasons why interpreting such data is difficult: (1) the standard N:P ratio experimental designs do not separate the effects of N:P ratio from [N + P] (i.e. proportionality is confounded with concentration) and (2) the bulk solution ions (e.g. Na+, K+, ) that provide an ‘ionic context’ are also varied but not accounted for in the experimental design (i.e. all ion effects are confounded).
The second reason is of particular concern as it assumes that these bulk solution ions have little or no effect on nutrient requirements and uptake kinetics, and thus growth rate. Does the basal medium formulation really not matter when quantifying optimal N:P ratios–i.e. the optimal N:P ratio is the same in Bold's Basal Medium, Chu's #10 Medium, or in water from an agricultural retention pond? If the ions that drive the bulk solution properties do have a substantial effect, is the optimal N:P ratio for growth of any given alga the same for any given combination of ions? Is it possible to compare results between studies that use different bulk solution ion blends but do not account for their effects? We think that the type and concentration of bulk solution ions could have a substantial effect on growth and therefore must be accounted for in any experiment aiming to determine the effect of N:P ratios. However, we are not aware of any studies that have addressed these issues from a mathematically valid, empirical perspective.
We present a series of experiments that determines the main and interaction effects of five ions, ,
, K+, Na+ and Cl−, on the growth of two algal species, Chlorella vulgaris and Peridinium cinctum. The experiments were designed to quantify the effects of ion ratios as distinct from ion concentrations and are representative of a wide range of ratio and amount combinations of these ions. Such experiments required the construction of a hyperspatial experimental geometry (see Experimental design below), identification of sufficient points for a representative sampling of the hypervolume, and measuring the algal growth at each of these points. We report the relationships between growth rates, N:P ratios, ion concentrations and other bulk solution parameters on the growth of these two algae.
Materials and methods
Experimental design
Every factor-based experimental design can be thought of in geometric terms. A single-factor design is defined by a finite vector, x, which is varied over some given range. This one-dimensional experimental design is usually coupled with a response vector, y, and presented graphically in the standard ‘x–y’ graph. If we wish to quantify the effects of two independent factors, factor x and factor y, then the experimental design space is now defined as a plane bounded by two normal, finite vectors, and any measured response would be plotted, in dimension zxy , as a response surface superimposed on this design space. Three factors translate to a cubic experimental design volume with vectors x, y and z, and a response volume, axyz , mapped within this 3-dimensional space. This reasoning can be extended to n-dimensions, although it becomes more difficult to depict dimensionality beyond 3-D. A 4-D+ volume is often referred to as a ‘hypervolume’ as it exists in ‘hyperspatial’ Euclidean space. The geometry of mixture-based experimental designs, defined as any experiment in which the responses are affected by factor proportionalities, presents a special case (cf. Cornell, Citation2002). Since proportionalities are inherently relational, in practice they require a minimum of two components. Thus, mixture experimental design geometries have n-1 dimensions, where n is the number of components in the mixture. A 3-component mixture design space is 2-D and describes an equilateral triangle if the component vectors are unconstrained (). An unconstrained, 4-component mixture design space describes a tetrahedron (), while a 5-component mixture, as used in the study presented here, describes a ‘4-D tetrahedron’, or a ‘pentatope’ ().
Fig. 1. The geometries for (A) 2-D, 3-component, (B) 3-D, 4-component, and (C) 4-D, 5-component mixture-based experimental designs. (A) is referred to as a ‘tri-linear’ graph and bears some explanation for those unfamiliar with interpreting these types of presentations. The vertices, labelled x, y and z, represent a ‘pure blend’ or 100% of that component. The relative proportion of each component decreases as you move away from its vertex. The proportionalities of point (a) are 50% x, 25% y, and 25% z. The edges of the tri-linear graph are all blends of the two components that define each edge, e.g. y–z, or x–y. Thus, the proportionalities of point (b) are 0% x, 50% y and 50% z.
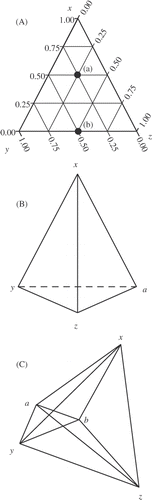
For this study a ‘mixture-amount’ design (Cornell, Citation2002) was chosen in order to isolate nutrient/ion proportionality effects from concentration effects. This type of design describes a (n−1)-dimensional hypervolume where each dimension is the relative proportion of each ion, i.e. ‘mixture’; this ‘mixture’ hypervolume is then projected across a total ion concentration vector, i.e. ‘amount’. D-optimality criteria were used to minimize the number of factor/component combinations necessary to provide accurate estimates of the model coefficients of a crossed, i.e. ‘cubic × cubic’, Scheffé polynomial experimental design volume (Box & Draper, Citation1971; Cornell, Citation2002). Specifically, sufficient treatments or ‘design points’ were selected with Design Expert® software (v7.0.3, Stat-Ease, Inc. Minneapolis, MN) such that the determinant of the (X′X)−1 matrix was minimized, which has the net result of minimizing the volume of the confidence ellipsoid for the coefficients of the selected model (Box & Draper, Citation1971; Piepel, Citation1988). There were 140 unique ion proportion/total ion concentration treatment points distributed throughout the 5-D experimental design hypervolume to estimate model coefficients. Forty treatment points were added to estimate the lack of fit (LOF) between the response surface models and design points not used to generate the model fits (Weisberg, Citation1985). Another 33 treatments were added to the experimental design in order to: (1) attain sufficient degrees of freedom (df) to estimate pure error across the design space, (2) provide estimates of block effects and (3) to reduce the potential effect(s) of high leverage points. In all, there were 213 design points distributed among three blocks ().
Fig. 2.
Two methods for visualizing the experimental design used in this study. (A) A 2-D perspective projection of a 4-D, 5-vertex pentatope projected across a total ion concentration (1–30 mM) dimension. Geometrically, there are no differences between the three pentatopes, but the total ion concentration that the proportions of ions in each pentatope sum to varies from left to right. (B) A 2-D, non-metric multidimensional scaling (MDS) plot of the 5-ion treatments chosen for this study. The symmetry of the 5-dimensional experimental design hypervolume remains visible even after collapsing the experimental design pentatope to two dimensions. Ion vertex points, type and concentration (mM), are indicated by the stars (N = and P =
). The stress of the 2-D MDS projection was 0.19.
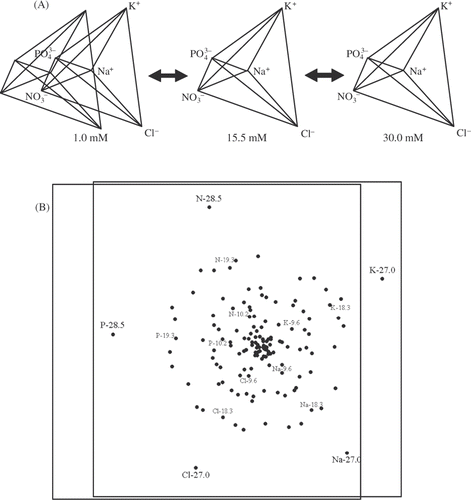
Culture conditions
Chlorella vulgaris Beijerinck (UTEX 1809) and Peridinium cinctum (O.F. Müller) Ehrenberg (CCAP 1140/1) were obtained from the Culture Collection of Algae at the University of Texas at Austin, USA, and the Culture Collection of Algae and Protozoa at Dunstaffnage Marine Laboratory, Argyll, Scotland. Cultures were grown in modified Bold's Basal Medium (mBBM) made from a combination of autoclaved and filter-sterilized stock solutions. The mBBM constituents kept constant were: 185 µM , 170 µM Ca2+, 1.7 µM Co2+, 6.3 µM Cu2+, 171 µM EDTA4−, 17.9 µM Fe2+, 304 µM Mg2+, 7.3 µM Mn2+, 4.9 µM Mo2+, 344 µM
and 30.7 µM Zn2+. All values are total ionic concentrations within the bulk medium and do not reflect any speciation that may occur in solution. Total ion concentrations of mixtures of
,
, K+, Na+ and Cl− were varied from 1 to 30 mM.
and
proportions were varied from 5 to 95% and K+, Na+ and Cl− were varied from 0 to 90% of the mixtures for these five ions. Thus, N:P ratios ranged from 0.05 to 19, N-P concentrations ranged from 50 µM to 28.5 mM and K-Na-Cl concentrations ranged from 0 to 27 mM. All media recipes were derived using the linear programming approach described by Niedz & Evens (Citation2006). Calculations were performed with ARS-Media (Ver. 1.0.1) ion solution calculation software (available as a free download at: http://www.ars.usda.gov/services/software/download.htm?softwareid=148). All media formulations were calculated and manipulated based on the parent ions and not on possible speciation products.
All flasks were initially inoculated to c. 104 cells ml−1 from a maintenance culture in standard BBM. Cultures were acclimated to experimental conditions for a minimum of 5–6 generations before dilution with fresh media and the commencement of the experiments. Batch cultures were grown in 75 ml of medium in 150 ml Erlenmeyer flasks in a temperature-controlled incubator at 25°C under an 18 : 6 light : dark irradiance regime. Incubator temperature was monitored by an internal digital temperature probe and by a thermometer placed in a 150 ml flask filled with 75 ml of water. Illumination was provided by cool-white fluorescent lights at an irradiance level of 125 µmol photons m−2 s−1. Scalar PAR irradiance (Eo) levels were determined with a 4π spherical micro quantum sensor (Heinz-Walz US-SQS/B) placed within a stoppered 150 ml flask and immersed in 50 ml of H2O. Flasks were swirled/shaken by hand at least twice daily and their positions on the incubator shelves were rearranged randomly at least once each day to mitigate any discontinuities in irradiance or temperature within the incubator. Cell counts were carried out microscopically using an Improved Neubauer haemocytometer. The correlation between cell numbers and optical density at 750 nm (OD750) was determined for both species (data not shown). OD750 was determined daily for each culture and used to estimate cell numbers; these estimates were occasionally checked with haemocytometer counts and were found to deviate no more than ± 5% from the predicted values.
Media formulations that did not exhibit growth were periodically re-inoculated with cells from their nearest neighbour(s) in Euclidean space that exhibited a positive growth rate. This resulted in the methodical acclimation of the cultures to increasingly extreme ionic conditions and allowed us to find the vertices of the growth hypervolume for each alga. Growth rates were calculated during early exponential growth.
Statistical analyses
A detailed description of the statistical methods used to analyse the data can be found in Evens et al. (Citation2008). Briefly, all possible models from the mean to cubic polynomial were calculated with Design Expert®. Initial model selection was based on a battery of adequacy tests as described by Anderson & Whitcomb (Citation2005). Normality and constant variance were determined graphically; a Box–Cox plot was used to choose the correct transformations (Box & Cox, Citation1964). Overly influential data points were identified with DFFITS and DFBETAS plots (cf. Belsley et al., Citation1980 for a definition of DFFITS and DFBETAS). Adequate precision of the model was determined by comparing the range of the predicted values at the design points (ŷ) to the average variance (V-bar) of the prediction (Anderson & Whitcomb, Citation2005). Potential outlier points were checked with externally studentized ‘outlier-t’ (Weisberg, Citation1985; Myers, Citation1990) and Cook's Distance (Cook & Weisberg, Citation1982) graphical plots. R2, adjusted-R2 () and predicted-R2 (
) were estimated for each selected model (cf. Myers & Montgomery, Citation2002).
Model validation
In order to empirically assess the usefulness of the predictive capabilities of the proposed RSM models, a variety of validation treatments were grown in regions of the design space not included in the initial experiments. In addition, a multivariate optimization technique (Nelder & Mead, Citation1965; Derringer & Suich, Citation1980) was used to identify the region of optimal growth for C. vulgaris and P. cinctum by simultaneously maximizing μ and maximum cell density; three validation treatments were grown in and near this region. Cultures were grown at all of these points and the measured responses were compared with model predictions (data not shown). All responses falling within the 95% prediction interval (Hahn & Meeker, Citation1991) were considered successful.
pH calculations/measurements
All pH calculations were performed with MINEQL+ (Ver. 4.5; Schecher & McAvoy, Citation1998), a chemical equilibrium model capable of calculating aqueous speciation, solid phase saturation states, precipitation-dissolution and adsorption. Calculations were based on all ions in each medium (i.e. 14 to 17 in total), temperature corrected, and assumed to be open to the atmosphere with a ppCO2 at sea level of 0.04%. The pH of each medium before addition of algal cells was measured with an accumet™ AR25 pH meter fitted with Ag/AgCl gel-filled, temperature-corrected probe.
Results
Model fitting
No deviation from normality was detected for any of the measured responses. The variances appeared constant as all points fell within ± 3σ and the scatter of residuals did not reveal any obvious distortions. The predicted versus measured plots indicated very close correlations between modelled and measured data points. Outlier-t and Cook's distance plots did not reveal treatments considered as outliers and therefore suspect. DFFITS and DFBETAS plots did not indicate treatments with overly large influences on predictions or regression coefficients, respectively.
A summary of ANOVA data, model diagnostics and coded regression coefficients for Chlorella vulgaris and Peridinium cinctum is presented in and , respectively. Model reduction by backward elimination (Peixoto, Citation1990; Nelder, Citation1998) improved the model fits. Therefore, the models are referred to as ‘reduced’. The models for all responses were highly significant (P < 0.0001), and, with the exception of lack of fit, all diagnostics indicated very close agreement between models and data.
Table 1. Scheffé polynomial model terms and coefficients, and ANOVA P-/F-values for Chlorella vulgaris growth rates in relation to
,
, Na+, K+ and Cl− ratios and total ion concentrations ([Conc]).
Table 2. Scheffé polynomial model terms and coefficients, and ANOVA P-/F-values for Peridinium cinctum growth rates in relation to
,
, Na+, K+ and Cl− ratios and total ion concentrations ([Conc]).
Specific growth rates
At the lowest total ion concentrations both C. vulgaris and P. cinctum exhibited ion-specific, as opposed to cation/anion-specific, growth rate responses. These ion-specific responses were more pronounced in P. cinctum and resulted in this alga growing in relatively limited regions of the ion space that appear to be inaccessible to C. vulgaris. Ion-specificity was replaced by a more general anion-/cation-specificity at total ion concentrations ≥ 15 mM for both algae. While there was significant overlap between the hypervolumes where each alga exhibited positive growth, C. vulgaris grew much faster than P. cinctum over most of this common ion-space.
Chlorella vulgaris exhibited sustained positive growth in 64 of the 174 unique treatment points (c. 37%), while P. cinctum was able to grow in 70 of the 174 points (c. 40%). The largest region of the 5-ion hypervolume accessible to P. cinctum but not to C. vulgaris was at 1 mM total ion concentration with high proportions of NO3 and/or PO4 (Figs ). Positive specific growth rates ranged from 0.06 to 0.91 d−1 for C. vulgaris and from 0.03 to 0.59 d−1 for P. cinctum. Overall, C. vulgaris had a greater average growth rate than P. cinctum (0.29 vs. 0.21 d−1, respectively) for the treatment points where each alga exhibited positive growth. Regression coefficients for the individual ions are indicative of the main effect of each ion at its vertex in the experimental design hypervolume, which is a geometric term defined as the medium formulation with the maximum proportion of that ion. There was virtually no difference in regression coefficient values between the three anions or between the two cations for C. vulgaris and only slight differences for P. cinctum. Peridinium cinctum exhibited a slightly more positive response to K+ than Na+ for growth rate, while C. vulgaris showed an equally positive response to both cations. One or both cations were in every significant model term for both C. vulgaris and P. cinctum. Total ion concentration was only significant in ≥ third order model terms for both algae. It was much more important for P. cinctum as evidenced by the marked differences between 1 and 30 mM responses (, ).
Fig. 3.
A tetrahedron net projection (i.e. an ‘unfolded and flattened’ tetrahedron) of inverse square root-transformed, specific growth rate, µ (d−1), in relation to a range of proportions of ,
, K+ and Na+ for Chlorella vulgaris at (A) 1 mM and (B) 30 mM total [N + P + K + Na + Cl] ion concentration. The colour scale is indicated by the labelled contour lines. A cartoon describing how a tetrahedron is ‘unfolded and flattened’ to produce a ‘net’ is provided in (C).
![Fig. 3. A tetrahedron net projection (i.e. an ‘unfolded and flattened’ tetrahedron) of inverse square root-transformed, specific growth rate, µ (d−1), in relation to a range of proportions of , , K+ and Na+ for Chlorella vulgaris at (A) 1 mM and (B) 30 mM total [N + P + K + Na + Cl] ion concentration. The colour scale is indicated by the labelled contour lines. A cartoon describing how a tetrahedron is ‘unfolded and flattened’ to produce a ‘net’ is provided in (C).](/cms/asset/b939c1e0-1a92-431d-934f-9be8d948600e/tejp_a_475979_o_f0003g.gif)
Fig. 4.
A tetrahedron net projection (i.e. an ‘unfolded and flattened’ tetrahedron) of inverse square root-transformed, specific growth rate, µ (d−1), in relation to a range of proportions of ,
, K+, and Na+ for Peridinium cinctum at 1 mM (A) and 30 mM (B) total [N + P + K + Na + Cl] ion concentration. The colour scale is indicated by the labelled contour lines.
![Fig. 4. A tetrahedron net projection (i.e. an ‘unfolded and flattened’ tetrahedron) of inverse square root-transformed, specific growth rate, µ (d−1), in relation to a range of proportions of , , K+, and Na+ for Peridinium cinctum at 1 mM (A) and 30 mM (B) total [N + P + K + Na + Cl] ion concentration. The colour scale is indicated by the labelled contour lines.](/cms/asset/d16bfab2-c9bd-4c1f-bb29-0e6cf3b6c8c0/tejp_a_475979_o_f0004g.gif)
Fig. 5.
A tetrahedron net projection (i.e. an ‘unfolded and flattened’ tetrahedron) of inverse square root-transformed, specific growth rate, µ (d−1), in relation to a range of proportions of ,
, Cl−, and Na+ for Chlorella vulgaris at 1 mM (A) and 30 mM (B) total [N + P + K + Na + Cl] ion concentration. The colour scale is indicated by the labelled contour lines.
![Fig. 5. A tetrahedron net projection (i.e. an ‘unfolded and flattened’ tetrahedron) of inverse square root-transformed, specific growth rate, µ (d−1), in relation to a range of proportions of , , Cl−, and Na+ for Chlorella vulgaris at 1 mM (A) and 30 mM (B) total [N + P + K + Na + Cl] ion concentration. The colour scale is indicated by the labelled contour lines.](/cms/asset/6d12eb45-b974-4ce5-83f5-5e18278ec120/tejp_a_475979_o_f0005g.gif)
Fig. 6.
A tetrahedron net projection (i.e. an ‘unfolded and flattened’ tetrahedron) of inverse square root-transformed, specific growth rate, µ (d−1), in relation to a range of proportions of ,
, Cl−, and Na+ for Peridinium cinctum at 1 mM (A) and 30 mM (B) total [N + P + K + Na + Cl] ion concentration. The colour scale is indicated by the labelled contour lines.
![Fig. 6. A tetrahedron net projection (i.e. an ‘unfolded and flattened’ tetrahedron) of inverse square root-transformed, specific growth rate, µ (d−1), in relation to a range of proportions of , , Cl−, and Na+ for Peridinium cinctum at 1 mM (A) and 30 mM (B) total [N + P + K + Na + Cl] ion concentration. The colour scale is indicated by the labelled contour lines.](/cms/asset/0ba96c3c-98ae-4e81-902d-8259bc37a2f2/tejp_a_475979_o_f0006g.gif)
Discussion
In this study our principal goal was to quantify the main and interaction effects of several primary nutrient and bulk solution ions. Because of logistical constraints we were limited to batch cultures, which dictated that we focus on maximal, nutrient-replete growth rates that occur in early exponential growth phase when issues of biologically induced media modification and self-shading are minimal. Although there is a great deal of interest in microalgal responses under nutrient limitation, batch culturing is not the best method to explore this issue. Thus, we initiated our cultures with a minimum of 50 µM for and
, i.e. 100 µM [N + P], with the assumption that this concentration was not limiting for either C. vulgaris or P. cinctum during early log phase of growth when the growth rates were measured. However, the conceptual approach and experimental design methodology described here are directly applicable to any ion-based study if coupled with proper culturing techniques.
The total five-ion concentration range chosen spans fresh to slightly brackish waters (1–30 mM) and explores most of the hypervolume delineated by the five ion/concentration vectors, i.e. all possible combinations of these five ions (given the imposed constraints) are encompassed within the experimental hypervolume. Some regions of this hypervolume will resemble ‘natural’ waters and some will not. There are two compelling reasons for exploring these ‘unnatural’ regions of the design space. (1) We can ask physico-biological questions such as ‘are negatively-charged ion effects anion-specific or ion-specific’, i.e. are bulk solution effects on microalgal growth rates the same regardless of the anion? (2) It is mathematically favourable to minimize constraints on the experimental design space (cf. Piepel, Citation1982).
From a mixture-amount perspective two results of particular interest are that ‘optimal’ N:P ratios are highly contextual and dependent upon the species and the proportions/concentrations of the other ions in solution; and that the interactions between total ion concentration (from 1–30 mM) and ion proportionalities are more prevalent for P. cinctum than C. vulgaris. It is immediately obvious that ionic effects, as opposed to nutritional effects, of and
are paramount in the absence of a significant concentration of a cation favourable for growth, e.g. Na+ and/or K+ (). As can be seen in Figs , the
–
axis covers a wide range of N:P ratios, but there is no growth of either C. vulgaris or P. cinctum until the proportions of Na+ and/or K+ are increased to some threshold value. The required cation proportion is relatively constant for C. vulgaris over the concentration range of 1–30 mM, but is more variable for P. cinctum. In it can be seen that at 1 mM total ion concentration P. cinctum maximal growth rates can be achieved at a variety of N:P ratios, from 0.10–13.00, if 0.30 ≤ [Na+] ≤ 0.75 (mM) and [K+] + [Cl−] = 0.00. At 30 mM total ion concentration P. cinctum maximal growth rates can be achieved at N:P ratios of 0.15–6.00 if 0.65 ≤ [Na+] ≤ 0.90 (mM) and [K+] + [Cl−] = 0.00 (note that these conditions are an oversimplification of the information contained in the equation describing the growth rate response volume as presented in Tables ). Thus, maximal growth rates can be achieved with media N:P ratios that vary over an order of magnitude if the bulk solution conditions are amenable. This means that from a nutrient-replete growth perspective, there is no truly ‘optimal’ N:P ratio as there are multiple regions within the ionic hypervolume, with the exact same N:P ratio, where each alga will grow well or not at all. These regions are a multivariate function of the proportion, type and concentration of each ion/nutrient present. With the conceptual approach and experimental methodologies outlined in this paper we are now able to map these regions and describe them mathematically. Thus, we can quantify not only the main effects of each ion of interest, but also the interactions between them.
Fig. 7. Response-trace plots of the effects of each of the five ions examined in this study. Response-trace plots are used to simultaneously compare the effects of all the components in the mixture design space. The lines represent the effect of changing each mixture component along a line from a reference blend (i.e. all five components at standard BBM proportions; indicated by the black dots) to a single component's vertex while holding all other components in relatively constant and equal ratios, i.e. as the amount of the labelled component increases, the amounts of all the other components decrease, but their relative ratios remain equal to each other.
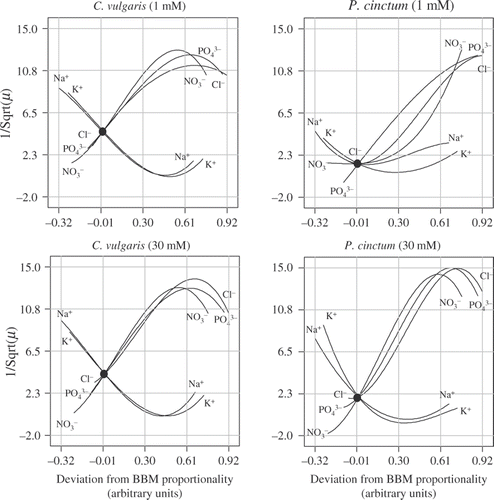
It is tempting to assign pH as a/the driver behind these responses. However, if we consider the relationship between the initial pH values of the media used in this study and growth rate (), it is obvious that initial pH is a poor predictor of growth rate for either C. vulgaris or P. cinctum. We did measure daily, presumably CO2-mediated, pH fluctuations; values predictably increased during the photoperiod and decreased in the dark. However, we saw no large shifts in pH during the early exponential phase of growth when the growth rates presented here were calculated. Some of the cultures that were able to achieve relatively high cell densities experienced permanent pH shifts, tending to increased pH, but this occurred only as they approached stationary phase. Thus, we find little evidence to directly correlate pH with growth rates.
Fig. 8. A correlational plot of initial pH of growth media versus maximum specific growth rates for Peridinium cinctum and Chlorella vulgaris.
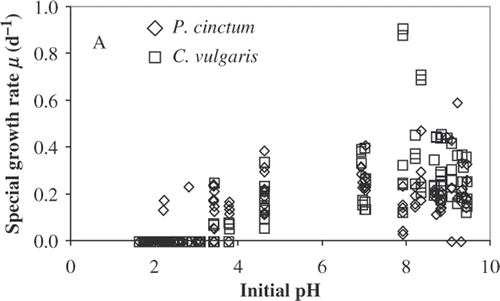
The multivariate, ionic hypervolume approach presented here is decidedly complex. The richness of the data generated by this type of experimental design argues alone for its adoption over traditional, salt-based, one-factor-at-a-time (OFAT) methodologies. However, there are also compelling mechanistic/procedural difficulties associated with the standard methodologies used to quantify nutrient/ion effects that recommend the multivariate approach used in this study: (1) the co-ion confounding introduced as a result of using salts to manipulate ion proportions/concentrations, (2) the confounding of nutrient and ion ratio effects with total ion concentration effects and (3) the difficulty in distinguishing interactions between nutrient ions and ‘bulk solution’ ions.
Co-ion confounding
Most, if not all, nutrient ratio studies are based on some type of manipulative experiment wherein the ion(s) of interest are covaried with the counter-ion of the salt used, e.g. using NaNO3 to manipulate []. If the proportion and/or concentration of an ion are not varied in an independent manner, then the main effect(s) associated with that ion can be mathematically indistinguishable from, or confounded with, the effect(s) of the covaried ions (Niedz & Evens, Citation2006). While it is true that the presence of a covariate does not a priori result in confounding, the two primary ways to avoid confounding are to eliminate the covariate or to show that the main effect and/or interactions of the covariate are constant and/or negligible between all independent factors. This type of confounding is of most concern in low salinity (‘freshwater’) studies where the covaried ion can introduce a significant change in ion ratios and concentrations, e.g. introducing µM levels of NaNO3 to manipulate [
] is not as much of a concern in seawater, although this can still be confounded. The import of this is that the only conclusion that is mathematically supported in a salt-based experiment is the combined effect of the covaried ions at the proportionality dictated by the parent salt at multiple concentrations, or in mixture-amount terms, the mean effect of the salt's component ions at a fixed proportionality over a range of total ion concentrations (cf. Evens & Niedz, Citation2008).
Ion ratio–total ion concentration confounding
There is general agreement that the effects of media nutrient ratios may be important for understanding microalgal physiology and ecology. It is also generally agreed that total ion concentration is important as well. However, the confounding of nutrient ratio effects with total ion concentration effects is, to the best of our knowledge, common to all nutrient ratio studies (). This insidious confounding occurs whenever nutrient ratios are manipulated without regard to the total concentration of the ions of interest. By disregarding the effect of total ion concentration there is an a priori assumption that this has minimal or no effect, which is counterintuitive. The net, unequivocal result of this type of confounding is that there can be no quantification of the main effects or interactions of nutrient ratios and/or total ion concentrations from such studies (cf. Fisher, Citation1935). Note that this confounding is not ameliorated by the high ion concentration of seawater as with co-ion confounding. The only approach that we are aware of that can be used to quantify the effects of ion proportionality independent of the effects of total ion concentration is the type of ‘mixture-amount’ experimental design (Cornell, 1992) employed in the present study.
Table 3. The proportions and total N + P concentrations of a typical 2 × 2 factorial approach to quantifying the effects of nutrient ratios. The covariance and confounding of proportion and [N + P] is obvious. No ion-specific, N : P proportionality, or total [N + P] main effects or interactions can be quantified with this type of experimental design.
The study presented here is the first set of unconfounded, ion-specific experiments with algae that we are aware of. We have shown that ‘optimal’ N:P ratios are contextual and highly dependent on the bulk solution properties of the medium. There are broad ranges of N:P ratios that are capable of supporting similar growth rates and conversely, there are broad ranges of growth rates associated with any given N:P ratio, depending on the bulk solution, ionic milieu. These data suggest that we need to reconsider the discussion surrounding ‘optimal’ ratios and concentrations of nutrients/ions in relation to growth to encompass the hyperdimensionality of media defined by multiple ion vectors. This ‘ionic context’ is extremely important to algal physiology, has broad implications for questions of nutrient requirements, and needs to be further explored.
References
- Anderson , MJ and Whitcomb , PJ . 2005 . RSM Simplified: Optimizing Processes Using Response Surface Methods for Design of Experiments , New York : Productivity Press .
- Belsley , DA , Kuh , E and Welsch , RE . 1980 . Regression Diagnostics: Identifying Influential Data and Sources of Collinearity , New York : Wiley & Sons, Inc. .
- Box , GEP and Cox , DR . 1964 . An analysis of transformations (with discussion) . J. Royal Statistical Soc. Ser. B , 26 : 211 – 246 .
- Box , GEP and Draper , NR . 1971 . Factorial designs, the X’X criterion, and some related matters . Technometrics , 13 : 731 – 741 .
- Cook , RD and Weisberg , S . 1982 . Residuals and Influence in Regression , New York : Chapman and Hall .
- Cornell , JA . 2002 . Experiments with Mixtures: Designs, Models and the Analysis of Mixture Data, , 3rd , New York : Wiley & Sons .
- Derringer , G and Suich , R . 1980 . Simultaneous optimization of several response variables . J. Qual. Technol. , 12 : 214 – 219 .
- Evens, T.J. & Niedz, R.P. (2008). Are Hofmeister series relevant to modern ion-specific effects research? Schol. Res. Exch. Vol. 2008, Article ID 818461, doi:10.3814/2008/818461.
- Evens , TJ , Niedz , RP and Kirkpatrick , GJ . 2008 . Temperature and irradiance impacts on the growth, pigmentation and Photosystem II quantum yields of Haematococcus pluvialis (Chlorophyceae) . J. Appl. Phycol. , 20 : 411 – 422 .
- Falkowski , PG . 1997 . Evaluation of the nitrogen cycle and its influence of the biological sequestration of CO2 in the ocean . Nature , 387 : 272 – 275 .
- Fisher , RA . 1935 . The Design of Experiments , Edinburgh : Oliver & Boyd .
- Geider , RJ and La Roche , J . 2002 . Redfield revisited: variability of C:N:P in marine microalgae and its biochemical basis . Eur. J. Phycol. , 37 : 1 – 17 .
- Hahn , GJ and Meeker , WQ . 1991 . Statistical Intervals: A Guide for Practitioners , New York : Wiley & Sons .
- Leonardos , N and Geider , RJ . 2005 . Elemental and biochemical composition of Rhinomonas reticulata (Cryptophyta) in relation to light and nitrate-to-phosphate supply ratios . J. Phycol. , 41 : 567 – 576 .
- Myers , RH . 1990 . Classical and Modern Regression with Applications., , 2nd , Boston : PWS-KENT Publishing Co. .
- Myers , RH and Montgomery , DC . 2002 . Response Surface Methodology: Process and Product Optimization using Designed Experiments., , 2nd , New York : Wiley & Sons .
- Nelder , JA . 1998 . The selection of terms in response surface models–how strong is the weak heredity principle? . Am. Statist. , 52 : 315 – 318 .
- Nelder , JA and Mead , R . 1965 . A simplex method for function minimization . Comput. J. , 7 : 308 – 313 .
- Niedz , RP and Evens , TJ . 2006 . A solution to the problem of ion confounding in experimental biology . Nature Methods , 3 : 417
- Peixoto , J . 1990 . A property of well-formulated polynomial regression models . Am. Statist. , 44 : 26 – 30 .
- Piepel , GF . 1982 . Measuring component effects in constrained mixture experiments . Technometrics , 24 : 29 – 39 .
- Piepel , GF . 1988 . Programs for generating extreme vertices and centroids of linearly constrained experimental regions . J. Qual. Technol. , 20 : 125 – 139 .
- Redfield , AC . 1934 . “ On the proportions of organic derivatives in sea water and their relation to the composition of plankton ” . In James Johnstone Memorial Volume , Edited by: Daniel , RJ . 176 – 192 . Liverpool : Liverpool University Press .
- Rhee , G-Y . 1978 . Effects of N:P atomic ratios and nitrate limitation on algal growth, cell composition, and nitrate uptake . Limnol. Oceanogr. , 23 : 10 – 25 .
- Schecher, W.D. & Mcavoy, D.C. (1998). MINEQL+ A Chemical Equilibrium Modeling System: Version 4.0 for Windows. User's Manual, Environmental Research Software, Hallowell, Maine.
- Schindler , DW , Hecky , RE , Findlay , DL , Stainton , MP , Parker , BR , Paterson , MJ , Beaty , KG , Lyng , M and Kasian , SEM . 2008 . Eutrophication of lakes cannot be controlled by reducing nitrogen input: results of a 37-year whole-ecosystem experiment . Proc. Nat. Acad. Sci. , 105 : 11254 – 11258 .
- Tyrrell , T and Taylor , AH . 1996 . A modeling study of Emiliania huxleyi in the NE Atlantic . J. Mar. Syst. , 9 : 83 – 112 .
- Weisberg , S . 1985 . Applied Linear Regression., , 2nd , New York : Wiley & Sons .