Abstract
This study was undertaken to compare and evaluate the parameters of 10 different mathematical models for their predictive ability in describing the lactation curves for daily milk yield of different genotypes under cooperative dairying in Bangladesh. A database consisting of 7340 herd-test records from 738 cows over the period 1999–2001 was assembled. Breed combinations included Pabna cattle, Australian-Friesian-Sahiwal×Pabna, Holstein×Pabna, Jersey×Pabna and Sahiwal×Pabna. The estimated parameters of the mathematical models, and the predicted lactation milk yields differed significantly between genotypes. The models were evaluated by four fit statistics: Akaike information criteria, coefficient of determination (R 2), root mean square prediction error (RMSPE) and concordance correlation coefficient (CCC). The AIC values indicated that the Ali model provided a good fit for all genotypes. The R 2 value suggested that the Legendre polynomial and Ali models were the best fit for all genotypes. CCC and RMSPE values indicated that the best models for all genotypes were Nelder and Wood. Since the CCC value was considered the most informative of the four fit statistics, the Nelder model was the best model to predict the full lactation profile based on test-day records for all genotypes. Using the Nelder model, the predicted 270 day milk yield of the Australian-Friesian-Sahiwal×Pabna genotype was higher (1823 kg) than the other genotypes (1509, 1650, 1531 and 1627 kg for Pabna, Australian-Friesian–Sahiwhal×Pabna, Jersey×Pabna and Sahiwal×Pabna, respectively).
Introduction
The members of the primary dairy cooperatives of the Bangladesh Milk Producer's Cooperative Union Limited (BMPCUL) milk mostly Pabna cattle, which were developed through the natural mating of local cows with Hariana, Red Sindhi and Sahiwal bulls. The Pabna cattle are regularly crossed with Sahiwal, Holstein-Friesian and Jersey breeds.
In Bangladesh, there is no well-developed recording system for dairy cattle genetic evaluation and improvement. However, non-governmental organisations and private farms maintain milk and health records. The Bangladesh Agricultural University, Bangladesh Livestock Research Institute (BLRI) and BMPCUL also maintain milk, pedigree and health records of their herds. But in the BMPCUL milk-shed area cows’ management and rearing conditions vary throughout the year. During the wet season, all dairy cattle are kept in unplanned semi-intensive housing, where they are fed mainly paddy straw. In the dry season, animals are grazed on pasture land, popularly known as Bathan. Throughout the year, lactating cows are fed 2–3 kg/day/cow of concentrates. For various nutritional experiments, genetic evaluations and other research projects, BMPCUL and BLRI sometimes keep individual cow productive and reproductive performance records of the different genotypes.
Mathematical models are required to predict the total amount of milk yield throughout the entire milking period using regularly recorded daily yields. It is also normal to standardise the number of days in the milking period for all cows. Mathematical models of lactation yields were developed in the 1960s when Wood (Wood Citation1967) proposed the incomplete gamma function. Before Wood, other workers (Gaines Citation1927; Nelder Citation1966), predicted lactation yield with simple mathematical models, which had limited accuracy. Since Wood, various models describing the lactation curve in dairy cows have been reported in the literature, but their applicability under tropical conditions has received limited investigation (Val-Arreola et al. Citation2004). In Bangladesh, no such studies have been undertaken.
The objectives of this study were to: (1) examine the lactation curves for Pabna and crossbred cows, (2) compare a number of mathematical models for simulating lactation curves and (3) determine the most suitable mathematical model(s), for predicting milk yield under cooperative dairying in Bangladesh.
Materials and methods
Data sources
Measurements of milk production traits of Pabna cattle, Australian-Friesian-Sahiwal×Pabna, Holstein×Pabna, Jersey×Pabna and Sahiwal×Pabna were obtained using records from the Animal Breeding Section of BMPCUL. The data covered the calving years from 1999 to 2001, with each year being divided into two calving seasons: dry (November to May) and wet (June to October). Milk yield data were recorded within 15 days of calving, and collected continuously until the end of lactation at an interval of 30 days for each individual cow. Occasionally, due to unavoidable circumstances, the milk recorder failed to record milk data on the desired day resulting in a small number of cows having records not taken exactly on the 30-day time schedule. Cows with less than four herd test records were deleted from the data, leaving 7340 records for analysis. In this study, nine test-day milk yield records on 738 cows were used to predict lactation milk yields (PLMY) over 270 days. In addition, lactation milk yields were calculated by BMPCUL staff as 15 days times the 15 day milk yield plus 30 days times 45 day milk yield etc. up to the end of lactation. This calculation takes no account of the shape of the lactation curve, nevertheless it will give a modestly accurate estimate of the total yield due to the regular recording of the milk yield. The averages of lactation milk yield and lactation length of all the genotypes were estimated and differences between means were performed using the least significant difference test (Steel et al. Citation1997).
Lactation equations
Ten mathematical models were used to model the lactation curve of daily milk yields recorded at 30 day intervals (). For the equations (), parameter a is always the y-intercept; it controls the vertical position of the curves when plotting daily milk yield against days in lactation. Generally, parameters b and c control the height of the maximum yield and parameters d and k shift the curve up or down the y-axis. The parameters a, b, c, d and k, together with days in milk define the shape and position of each curve.
Table 1. Mathematical models used to describe the lactation curve of dairy cows.
Statistical analysis
The Ganies and Wood model functions were fitted using PROC NLMIXED of SAS (SAS Citation2000). The fifth-order polynomial, Legendre polynomial and Ali polynomial regression, were fitted using PROC MIXED of SAS to estimate their parameters. All other models were fitted using PROC NLIN of SAS.
The goodness-of-fit of predicted values to actual test-day records was tested using four model statistics: the Akaike information criteria (AIC); coefficient of determination (R 2); concordance correlation coefficient (CCC) and root mean square prediction error (RMSPE). The AIC combines the maximum likelihood (data-fitting) and the number of parameters of model, by penalising the (log) maximum likelihood with a term related to model complexity (Val-Arreola et al. Citation2004). Smaller values of AIC indicate a better fit when comparing the models.
The CCC is a comprehensive statistic used to test simultaneously and jointly for accuracy and precision of the models (Runze and Chow Citation2005) and assesses the systematic error of actual and predicted values (Pierre Citation2005), with high values indicating a superior fit. The CCC (Lin Citation1989) was calculated as:
A i is the actual milk yield at day i and P i is the predicted milk yield at day i.
The following statistical model was used to obtain the least square means (±standard error) for each parameter (a, b, c, d and k), estimated from the 10 mathematical models, the predicted 270 day lactation milk yields and for each of the fitness measures.
where Y ijkl represents the parameter (predicted milk yield, a, b, c, d, k and fit statistics); µ is the overall mean; βi is the effect of ith genotype; γj is the effect of jth year of calving; S k is the effect of kth calving season; e ijkl is the random residual effect distributed as N(0, σ2).
The model was fitted considering only the effects of genotype, year and season without interactions as the interaction of the year and calving season was found to be non-significant. To select the best model(s) for each breed(s), the fit statistics values were used.
Results and discussion
The number of cows, total lactation milk yield estimated by BMPCUL staff and lactation length of different genotypes varied (). There were only small numbers of cows for Australasian-Friesian-Sahiwal×Pabna and Jersey×Pabna genotypes. The calculation of lactation yields by BMPCUL technicians showed that the Australasian-Friesian-Sahiwal×Pabna and Holstein×Pabna genotypes produced higher lactation yields and had longer lactation lengths than other genotypes. Similar findings were reported by several authors (e.g. Bhuiyan et al. Citation1998; Khan and Khatun Citation1998; Hossain Citation2006). However, a study by Talukder et al. (Citation2001) reported lower milk yields for Sahiwal×Local and Jersey×Local genotypes than in the current study, which might be due to the use of Deshi rather than Pabna cattle in their study. Furthermore, many findings (e.g. Cunningham and Syrstad Citation1987; Syrstad Citation1989; Madalena et al. Citation1990) from other tropical countries have also shown that the first cross of temperate breeds with tropical breeds produce more milk than pure breeds in a tropical environment.
Table 2. The number of cows, lactation yield calculated by BMPCUL technicians and lactation length of different breeds, estimated from test-day records.
Model performance and model parameters
The 10 mathematical models fitting lactation curves to the test-day data were evaluated based on the four different fit statistics (). The Rook and Diskstra models were not considered further due to their unsuitable model parameters and also poor fit statistics value. The Gaines model was also excluded from further consideration, as it did not allow for the shape of the lactation curve. Four fit statistics (AIC, R 2, CCC and RMSPE) values were used to evaluate the performance of the remaining seven models. Smaller AIC and RMSPE values indicated a better fit (Motulsky and Christopoulos Citation2003; Val-Arreola et al. Citation2004), but for R 2 and CCC higher values indicated superior models (Motulsky and Christopoulos Citation2003; Pierre Citation2005). Within each model, the fit statistics were fairly consistent between breeds. Among the four fit statistics, the CCC value was considered the most informative due to its accuracy, precision and the possibility of assessing the systematic error for actual and predicted values (Runze and Chow Citation2005; Pierre Citation2005).
Table 3. Comparison of model performance using different fit statistics (±standard error).
The poorest models were: AIC values – Legendre polynomials and Wilmink; R 2 values-Sikka and Wilmink; RMSPE values – Legendre polynomials and Sikka and for CCC – Legendre Polynomial and Sikka models. The best models were: AIC values – Ali and Nelder; R 2 – Legendre polynomials and Ali; RMSPE – Nelder and Wood and for CCC – Nelder and Wood models (). Therefore, according to the four fit statistics the best four (Nelder, Ali, Wood and Polynomials) models for five different genotypes were presented for the shape of the lactation curve, model performance and model parameters.
The variation of fit of the models between breeds may have arisen from the differences in breeds, mathematical functions of the models, differences of test-day yield and the amount of data. The differences in the lactation curves are a combination of environmental and genetic factors (Pérochon et al. Citation1996). The numbers of test-day records and the intervals between tests have effects on the estimation of fit statistics, and also the model fitting ability (Tekerli et al. Citation2000; Wiggans et al. Citation2002).
Results of this study indicated that the Nelder model was the most suitable function to model test-day milk yields for all genotypes based on higher CCC and RMSPE and they also performed well enough for the other two criteria. The Ali and Wood models were the next two best models.
Shape of the lactation curves
The raw test day milk yields of the five genotypes and the best fit lactation curve generated by the Nelder model were plotted (). The figures showed that the time of peak milk yield calculated from the raw data was about 75 days in milk (DIM) for all genotypes, followed by a gradual decline. The Nelder model gave a peak at 45 DIM for the Pabna, Holstein×Pabna and Sahiwal×Pabna genotypes, 15 DIM for the Australian-Friesian-Sahiwal×Pabna genotype and 73 DIM for the Jersey×Pabna genotype.
Figure 1. Lactation curves for all the cows of different genotypes obtained from the test-day data and by fitting the Nelder equation.
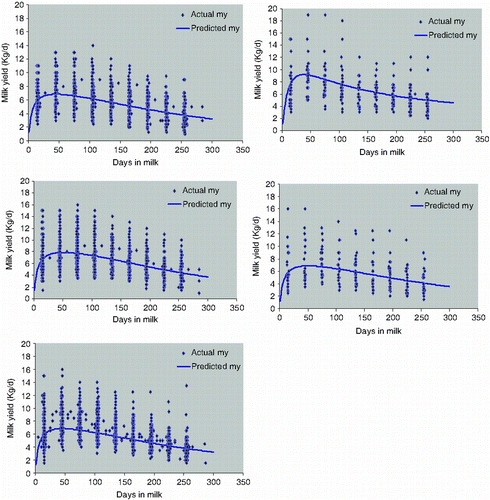
The gradual decline in the lactation curve has been reported in previous studies (Tekerli et al. Citation2000; Val-Arreola et al. Citation2004). The most common shape was a rapid increase, followed by a gradual decline until the cow is dried off. The other shape is a gradual decline from parturition. Several studies (e.g. Macciotta et al. Citation2005; Fathi Nasri et al. Citation2008), have shown that the differences in the general shape and the differences of model parameters are associated with yield at the beginning of lactation, the degree of incline and decline before and after peak yield, days in milk at peak and peak and lactation yields of the lactation curves.
The total lactation milk yields calculated from the raw data () were similar to the total lactation yields predicted by the Nelder model (), except for the Holstein×Pabna genotype. The reasons for the similar milk yield between calculated and predicted were due to the regular milk testing by the BMPCUL staff. For the Holstein×Pabna genotype the Nelder model predicted 204 kg of milk more than that from the raw data. The predicted milk yield of Holstein×Pabna was significantly higher due to the breed effects and the predicted ability of the model parameters. Similar factors were reported for the differences in predicted milk yield from lactation curve studies from elsewhere (Pérochon et al. Citation1996; Vargas et al. Citation2000; Brown et al. Citation2001).
Table 4. Parameter estimates (±standard error) and other measures when Nelder model was fitted to the different breed groups in Bangladesh.
Effects of genotype
Differences in the shape of the lactation curve between different genotypes were observed, with the differences in day of peak yield, the steepness and also the flatness of the curves and these are the results of the differences for predicted model parameters of different models. These differences for predicted model parameters were also reported by other investigators (Pérochon et al. Citation1996; Vargas et al. Citation2000). In addition, the differences of curve shape could be attributed to few test-day records prior to the peak, and also to a low number of available records. Other studies (Rekik and Gara Citation2004; Silvestre et al. Citation2006) identified similar results for the estimation of lactation curve shape.
As estimated by the Nelder model (), the intercept (a) for Australian-Friesian-Sahiwal×Pabna, Holstein×Pabna and Sahiwal×Pabna, the curve shape (b) for Holstein×Pabna and Jersey×Pabna Sahiwal×Pabna and Pabna genotypes and the placement of the parabola (c and d) for the Australian-Friesian-Sahiwal×Pabna genotypes were significantly higher than other genotypes.
Conclusion
Results of this study indicate that the Nelder model was the most suitable to transform test-day milk yields into a 270-day predicted milk yield for all genotypes, based on higher CCC and lower RMSPE and AIC values. The Wood and Ali models were the next best models. The predicted lactation milk yield differed between genotypes, mathematical functions of the model and also the model parameters. The Australian-Friesian-Sahiwal×Pabna and Holstein×Pabna genotypes fitted better than the other genotypes for all models. There were some limitations in this study, such as the low number of records per genotype representing only three years. Confidence that the correct mathematical model has been chosen would be increased if additional data were to be collected and added to the current analyses.
Acknowledgements
The first author is funded by NZAID: Postgraduate scholarship for PhD studies sponsored by The New Zealand Ministry of Foreign Affairs and Trade. The authors are grateful to the Ministry for their financial support and they would like to thank the Institute of Veterinary, Animal and Biomedical Sciences, Massey University, Palmerston North, New Zealand for their active cooperation and support to pursue this study. The authors are also very much grateful to Mr Shakhawat Hussen, Manager, Animal Breeding Section, Bangladesh Milk producer's Cooperative Union Limited for providing the data.
References
- Ali , TE and Schaeffer , LR . 1987 . Accounting for co variances among test day milk yields in dairy cows . Canadian Journal of Animal Science , 67 : 637 – 644 .
- Bhuiyan , AKFH , Hossain , SS and Hussen , MS . 1998 . Effect of Sahiwalisation on the performance of graded cattle at a rural area of Bangladesh. Proceedings of the 6th World Congress on Genetics Applied to Livestock Production, Armidale . Australia , 25 : 201 – 204 .
- Brotherstone , SI , White , MS and Meyer , K . 2000 . Genetic modelling of daily milk yield using orthogonal polynomials and parametric curves . Animal Science , 70 : 407 – 415 .
- Brown , MA , Brown , AH Jr , Jackson , WG and Miesner , JR . 2001 . Genotype×environment interactions in milk yield and quality in Angus, Brahman and reciprocal cross cows on different forage systems . Journal of Animal Science , 79 : 1643 – 1649 .
- Cunningham EP , Syrstad O. 1987 . Crossbreeding Bos indicus and Bos taurus for milk production in the tropics . FAO Animal Health and Production paper 68 , Rome Italy .
- Dijkstra , J , France , J , Dhanoa , MS , Maas , JA , Hanigan , MD , Rook , AJ and Beever , DE . 1997 . A model to describe growth patterns of the mammary gland during pregnancy and lactation . Journal of Dairy Science , 80 : 2340 – 2354 .
- Fathi Nasri , MH , France , J , Odongo , NE , Lopez , S , Bannink , A and Kebreab , E . 2008 . Modelling the lactation curve of dairy cows using the differentials of growth functions . Journal of Agricultural Science , 146 : 633 – 641 .
- Gaines WL. 1927 . Persistency of lactation in dairy cows . Agricultural Extension Standard Bulletin 288 .
- Hossain MS. 2006 . Genetic evaluation of fertility and milk production traits of crossbred cows at Baghabarighat milk shed area [PhD thesis] . Mymensingh : Department of Animal Breeding & Genetics, Bangladesh Agricultural University .
- Khan , MKI and Khatun , MJ . 1998 . Performance of F1 crossbred cows at Baghabarighat milk shed area . Bangladesh Journal of Animal Science , 27 : 183 – 186 .
- Lin , LIK . 1989 . A concordance correlation coefficient to evaluate reproducibility . Biometrics , 45 : 255 – 268 .
- Macciotta , NPP , Vicario , D and Cappio-Borlino , A . 2005 . Detection of different shapes of lactation curve for milk yield in dairy cattle by empirical mathematical models . Journal of Dairy Science , 88 : 1178 – 1191 .
- Madalena , FE , Teodoro , RL , Lemos , AM , Monteiro , JBN and Barbosa , RT . 1990 . Evaluation of strategies for crossbreeding of dairy cattle in Brazil . Journal of Dairy Science , 73 : 1887 – 1901 .
- Motulsky HJ , Christopoulos A. 2003 . Fitting models to biological data using linear and nonlinear regression. A practical guide to curve fitting [Internet] . San Diego , CA , GraphPad, Software Inc . 13 – 57 . Available from: www.graphpad.com/manuals/prism4/regressionbook.pdf .
- Nelder , JA . 1966 . Inverse polynomials, a useful group of multi-factor response functions . Biometry , 22 : 128 – 141 .
- Pérochon , L , Coulon , JB and Lescourreet , F . 1996 . Modelling lactation curves of dairy cows with emphasis on individual variability . Animal Production , 63 : 189 – 200 .
- Pierre NR. 2005 . Validating mathematical models of biological systems: application of the concordance correlation coefficient [Internet] . Sensitivity Analysis of Model Output , 341 – 350 . Available from: http://library.lanl.gov/cgi-bin/getdoc?event=SAMO2004&document=samo04-41.pdf
- Rekik , B and Gara , AB . 2004 . Factors affecting the occurrence of atypical lactations for Holstein-Friesian cows . Livestock Production Science , 87 : 245 – 250 .
- Rook , AJ , France , J and Dhanoa , MS . 1993 . On the mathematical description of lactation curves . Journal of Agricultural Science , 121 : 97 – 102 .
- Runze , L and Chow , M . 2005 . Evaluation of reproducibility for paired functional data . Journal of Multivariate Analysis , 93 : 81 – 101 .
- SAS® , User's Guide . 2000 . Statistics , 8th ed . Cary , NC : SAS Institute Inc .
- Silvestre , AM , Petim-Batista , F and Colaso , J . 2006 . The accuracy of seven mathematical functions in modelling dairy cattle lactation curves based on test-day records from varying sample schemes . Journal of Dairy Science , 89 : 1813 – 1821 .
- Sikka , LC . 1950 . A study of lactation as affected by heredity and environment . Journal of Dairy Research , 17 : 231 – 252 .
- Steel , RGD , Torrie , JH and Dickey , DA . 1997 . Principles and procedure of statistics- a biometrical approach , 3rd ed , 139 – 177 . New York and London : McGraw-Hill Co., Inc .
- Syrstad , O . 1989 . Dairy cattle crossbreeding in the tropics: performance of secondary crossbred populations . Livestock Production Science , 23 : 97 – 106 .
- Talukder , MSU , Haque , MN , Hossain , MI , Aziz , SA and Rahman , MR . 2001 . Effect of different genotypes on milk and reproductive performance of cows . Pakistan Journal of Biological Sciences , 4 : 1421 – 1424 .
- Tekerli , M , Akinci , Z , Dogan , I and Akcan , A . 2000 . Factors affecting the shape of lactation curves of Holstein cows from the Balikesir province of Turkey . Journal of Dairy Science , 83 : 1381 – 1386 .
- Val-Arreola , D , Kebreab , E , Dijkstra , J and France , J . 2004 . Study of the lactation curve in dairy cattle on farms in central Mexico . Journal of Dairy Science , 87 : 3789 – 3799 .
- Vargas , B , Koops , WJ , Herrero , M and Van Arendonk , JAM . 2000 . Modelling extended lactation of dairy cows . Journal of Dairy Science , 83 : 1371 – 1380 .
- Wiggans , GR , VanRaden , PM , Bormann , J , Philpot , JC , Druet , T and Gengler , N . 2002 . Deriving lactation yields from test-day yields adjusted for lactation stage, age, pregnancy and herd test date . Journal of Dairy Science , 85 : 264 – 274 .
- Wilmink , JBM . 1987 . Adjustment of test day milk, fat and protein yield for age, season and stage of lactation . Livestock Production Science , 16 : 335 – 348 .
- Wood , PDP . 1967 . Algebraic model of the lactation curve in cattle . Nature , 216 : 164 – 165 .