Abstract
Multi-sensor and multi-resolution source images consisting of optical and long-wave infrared (LWIR) images are analyzed separately and then combined for urban mapping in this study. The framework of its methodology is based on a two-level classification approach. In the first level, contributions of these two data sources in urban mapping are examined extensively by four types of classifications, i.e. spectral-based, spectral-spatial-based, joint classification, and multiple feature classification. In the second level, an objected-based approach is applied to decline the boundaries. The specificity of our proposed framework not only lies in the combination of two different images, but also the exploration of the LWIR image as one complementary spectral information for urban mapping. To verify the effectiveness of the presented classification framework and to confirm the LWIR’s complementary role in the urban mapping task, experiment results are evaluated by the grss_dfc_2014 data-set.
1. Introduction
In recent decades, remote sensing images are provided with increasingly finer resolutions in both spectral and spatial domains. Improvements in spatial resolution for optical sensors are specifically prominent, which can support the more detailed and accurate mapping. Data from these sensors enable advanced applications, such as urban mapping, precision agriculture, environmental monitoring, and military applications. Besides, the optical sensors of high spatial resolutions also challenge the image analysis, since high spatial resolution can lead to high interclass spectral confusion (Huang and Zhang Citation2009). Consequently, spectral-based methods are not appropriate solutions to deal with very high-resolution (VHR) spatial images.
To overcome this inadequacy, various attempts have been made to improve VHR classifications. One of the most widely used methods is to extract spatial features to provide discriminate information, such as gray level co-occurrence matrix (GLCM) textures (Puissant, Hirsch, and Weber Citation2005; Pacifici, Chini, and Emery Citation2009), shape information (Zhang et al. Citation2006), object-based approach (Huang, Zhang, and Li Citation2008), morphological profiles (Tuia et al. Citation2009), and Markov random field (Li, Bioucas-Dias, and Plaza Citation2012). Meanwhile, the emergence of new sensors and advanced processing techniques has made the utility of multi-source data increasingly feasible. Consequently, a different approach to compensate the spectral deficiency of VIR imagery is to integrate different data sources into applications, such as LIDAR data (Huang, Zhang, and Gong Citation2011) and SAR data (Waske and Benediktsson Citation2007).Since information provided by single sensor might be incomplete, inconsistent or imprecise, information fusion of multi-sensors is definitely a better approach in remote sensing classification, comparing with the single-source image classification.
The objective of this study is to examine the utility of the long-wave infrared (LWIR) information as a complementary spectral source for VHR classification in urban mapping. There are several studies reporting the use of thermal bands for land cover mapping. Lu and Weng (Citation2005) converted the thermal infrared image into a surface temperature map and incorporated it in the classification process. Gao et al. (Citation2006) used thermal infrared (TIR) bands to distinguish earth objects that have spectral confusion in the visible and near-infrared bands, their experiments also revealed that TIR bands contained useful information to distinguish different types of rock. Bischof, Schneider, and Pinz (Citation1992) stacked thermal data as spectral feature into the neural networks classification for the multi-spectral classification. Their experiments revealed that temperature information is helpful for the land cover class identification. Segl et al. (Citation2003) treated thermal data as spectral features in the classification and their results showed that thermal data played a key role for improving identification of non-vegetated urban surfaces. Keuchel et al. (Citation2003) selected a temperature threshold level on the thermal data to remove cool clouds from the warmer ground surface. Then the thermal band after preprocessing was combined with spectral bands for the classification. Their results showed that the thermal information indirectly provided helpful information for the land cover classification.
In order to exploit the utility of LWIR information in urban mapping, a novel two-level-based analytical approach is proposed in this paper. In the first level, optical image is firstly down-sampled to fit the size of the LWIR image. Afterward, four classification strategies are applied to investigate the contributions of the optical and LWIR image in urban mapping. Then, in the second level, an objected-based approach is adopted to project the low-resolution classification maps into the original size. The experiments in the first level are conducted on the 1 m optical image and 1 m LWIR image, and are conducted on the 0.2 m optical image in the second level.
2. Methodology
2.1. The classification framework
Different from the traditional optical image that records sunlight reflectance of the earth surface, the LWIR image responds to the varied temperature and emissivity of the ground. In our classification framework (Figure ), in order to investigate the utility of the optical/LWIR image and their combined applications in urban mapping, experiments are carried on in the following two levels:
(1) | First, the bilinear down-sampling process is implemented on the optical image as a preprocessing procedure. There are three main points accounting for the down-sampling process. For more details, it can adjust the optical image’s resolution to match the LWIR image’s coarse resolution, while simplify the complexity of the feature extraction and computation process. In addition, the down-sampling process can decrease the VHR image’s local variation and variance, thus, the pepper-salt effect which is commonly appeared in VHR classifications can be effectively reduced. Then contributions of these two data sources in the classification are extensively discussed by four types of classification:
| ||||||||||||||||||||||||||||
(2) | Second, the preferable classification maps from the first level are projected into the high-resolution boundaries which are generated by multi-resolution segmentation. |
2.2. Classification strategies
2.2.1. Spectral-based classification
Optical and LWIR images are utilized as two individual spectral data sources to investigate their validity in classifications, respectively. Thus, only spectral features are input for classification. It is adventurous to apply classification directly on the LWIR image. The classification performance is intriguing. Support vector machine (SVM) classifier, ENVI, is adopted in our study for all the classifications, with penalty coefficient = 100, kernel = RBF (radial basis function), and band width σ = 1/n (n is the dimension of the input features).
2.2.2. Spectral-spatial classification
For the spectral-spatial classification process, we exploit the spatial features as complementary information in the spectral feature space. Improvements in mapping accuracies can be expected, when shape, texture, and spatial coherence are integrated with the spectral features. Spectral-spatial classifications are performed separately for these two data sources with their corresponding spatial features involved in the classifications. To be innovative, spectral-spatial classification is firstly implemented on the LWIR images. For these two data sources, the 3D DWT features are calculated based on the original spectral features, while the other four spatial features are built from the first principal component (PC1) of the original spectral features.
2.2.3. Opt-LWIR joint classification
The Opt-LWIR joint classification is achieved by stacking the optical images (1 m), the spatial features and three PCs (most significant) of the LWIR images into the classifiers. The corresponding spatial features of these two data sources are utilized separately for comparison. This process aims to examine the practicability of the combined imagery of optical and LWIR images for urban mapping, and to verify the respective effectiveness of spatial features generated from each data source in the urban mapping.
2.2.4. Multiple feature classification
Since there are so many spatial features concerned in the spectral-spatial and joint classification process, it is interesting to explore whether their combined application will further improve the accuracy of urban mapping. Stacking different features is a conventional way to integrate various features. Unfortunately, feature stacking can also lead to high dimensionality and is not so effective in certain cases. It is a promising solution to fuse the classification outputs generated by various spatial features according to some decision rules. Accordingly, the decision rules used in this paper are majority voting, posterior probability, and uncertainty, respectively. In our experiments, the stacking is to stack all the spatial features with the optical and the first three principal components analysis (PCA) of the LWIR image for the classification process. However, for the decision fusion process, all the classification outputs of the spectral-spatial and joint classification are taken into consideration.
2.2.5. Objected-based approach
According to practical application, it is necessary to recover the low-resolution classification maps generated by the first level into the original size. Up-sampling the low-resolution maps directly into the original size can lead to very serious blurry edges. To overcome this inadequacy, objected-based approach provides a nice possibility. In this study, a segmentation algorithm, which is implemented in the commercial software eCognition®, is adopted to acquire boundaries at different scales by dividing the image into a series of non-overlapping objects. Afterward, for the up-sampled classification map, class labels for each object are relearned by majority voting of all the classification labels among this object.
2.3. Spatial features
2.3.1. GLCM
GLCM-based textural feature extraction has been proved to be the most effective statistical texture measures for land cover classification (Pacifici, Chini, and Emery Citation2009). In our study, the following two commonly used measures are chosen for the co-occurrence matrix:(1)
(2)
where (i, j) are coordinates in the co-occurrence matrix space; p(i, j) is the co-occurrence matrix value at (i, j); N is the dimension of co-occurrence matrix. In order to obtain multi-scale textural features, in our experiments, four window sizes are used: 3 × 3, 5 × 5, 7 × 7, and 9 × 9. Afterward, to suppress the directionality of GLCM, extracted textural features of each window size are averaged over four directions.
2.3.2. EMP
The opening and closing operators, which are defined on dilation and erosion by reconstruction, were firstly proposed to analyze panchromatic images by Pesaresi and Benediktsson (Citation2001). It has been proven to be effective tools for extracting spatial features from images and widely applied in remote sensing images analysis (Benediktsson, Palmason, and Sveinsson Citation2005).
Let and
be the morphological opening and closing with a structuring element (SE) for an image I, respectively. MPs are defined by a series of SEs with increasing sizes:
(3)
where λ is the radius of a disk-shaped SE. EMPs have been proposed for morphological feature extraction from hyperspectral imagery and can be written as (Liao et al. Citation2012):(4)
where f comprises a set of the n-dimensional base images with f(1) is the first band and f(n) is the nth band of image f. Similarly, to obtain multi-scale features, in this study, the EMPs are calculated based on a disk SE which is set to SE = [1, 3, 5, 7], with four openings and four closings.
2.3.3. DMP
DMPs (Pesaresi and Benediktsson Citation2001), another set of opening- and closing-based morphological features, were used to calculate the morphological profiles value differences at different scales. It has been proved out to be the state-of-the-art spatial feature extraction approach and is widely applied in the urban mapping (Huang and Zhang Citation2009) and automatic information extraction (Jin and Davis Citation2005). It can be expressed within the framework of MPs defined in Equation (Equation3(3) )
(5)
The signal recorded in the DMPs gives information about the size and the type of the structures in the image. Similar with EMPs, DMPs can also be built on base images of the hyperspectral images to avoid high-dimensional feature space. In our experiments, the SE setting for the DMP is the same as that of the EMP’s.
2.3.4. EAP
The third morphological profile involved in this paper is attribute profiles (AP). AP represents adaptive analysis by implementing a series of attribute thickening and thinning operators on the connect components according to various criteria (Dalla Mura et al. Citation2010). It has been proved to be effective spatial analysis tools to enhance the structures that presented in an image and is widely applied in urban mapping (Dalla Mura et al. Citation2011; Huang et al. Citation2014).
APs can also be expressed within the framework of MPs defined in Equation (Equation3(3) ), by replacing the opening and closing operators by a series of morphological attributes. Let us denote
and
by the attribute thinning and thickening operators, respectively. With a criterion Tλ, the APs can be written as:
(6)
The criteria considered in this paper consist of the area of the regions and the standard deviation. Similarly, for the multi/hyperspectral imagery, the EAPs can be represented by:(7)
In this study, the parameters of the morphological attribute profiles were defined according to the works of Dalla Mura et al. (Citation2010): (1) the area of the regions (λa = [100, 500, 1000, 5000]), (2) the standard deviation of the gray-level values of the pixels in the regions (λs = [20, 30, 40, 50]).
2.3.5. 3D DWT
Wavelet-transform-based feature extraction method is achieved by firstly over-complete wavelet decomposing of a square local area around each pixel. Afterward, different statistical measures of each sub-image are calculated and assigned to the components of the feature vector of the central pixel in the area (Fukuda and Hirosawa Citation1999). On account of the ability of examining the signal at different resolutions and desired scales, it was found to be a promising tool in image analysis of both spatial and frequency domain (Khare et al. Citation2013). In our research, a recently proposed object-based 3D discrete wavelet transform texture (Guo, Huang, and Zhang Citation2014) was adopted, which considers the local imagery (object) patch as a cube, and decomposes it into a set of spectral-spatial components. The 3D DWT feature is subsequently obtained by measuring the energy function of the wavelet coefficients, and providing the representation of the imagery information in both the spectral and spatial domains. Accordingly, for the multi/hyperspectral imagery, 3D DWT is constructed by a tensor product and can be written as follows:(8)
where ⊕ and ⊗ are the space direct sum and tensor product, respectively; L and H denote the low and high filters; superscripts of x and y denote the spatial coordinates of an image; z is the spectral axis. Afterward, the energy statistic is used to characterize the texture property and it can be written as follows:(9)
where W is a B × B × N local cube; B and N are the dimensions in the spatial and spectral domains, respectively; P(i, j, k) is the wavelet coefficient in the cube centered by the pixel (i, j, k). In our experiments, the so called “local imagery” is non-overlapping blocks that are generated by multi-scale segmentation of eCognition® software. For the segmentation criterion: scale parameter is set to 100, with shape and compactness is set to 0.1 and 0.5, respectively.
3. Experiments and analysis
3.1. Data-set
Experiments are carried on the grss_dfc_2014 data-sets which are provided for the 2014 Data Fusion Contest by Telops Inc. (Canada). Thegrss_dfc_2014 data-sets consist of two different data-sets acquired at different spectral ranges and spatial resolutions, which are integrated to the same airborne platform − a coarse-resolution LWIR hyperspectral data-set and a fine-resolution visible data-set, covering an urban area near Thetford Mines in Quebec, Canada. The thermal hyperspectral image which was acquired using an airborne LWIR hyperspectral imager (Hyper-Can), contains 84 spectral bands in the range of 7.8–11.5 μm at a spatial resolution of approximately 1 m. While the visible image was collected by a digital color camera, it contains RGB uncalibrated digital data at a spatial resolution of 0.2 m. The two data-sets were collected simultaneously on 21 May 2013, between 22:27:36 and 23:46:01 UTC with the average height of both sensors was 807 m, and they are georeferenced.
A training map was provided along with the data-sets, and the spatial resolution equals to that of the airborne color data-set. Numbers for the training and testing set in our experiments are listed in Table , and the training samples (200 for each class) used in the classification are generated randomly from the training set.
Table 1. Number of training and testing samples.
3.2. Experiments
3.2.1. Spectral-based classification
The SVM classification results for the spectral-based classification are listed in Table . It can be learned from the global accuracies that the optical image can roughly discriminate the main classes of the image, while the LWIR image has difficulty in recognizing them. Regarding the class-specific accuracies of optical images, some classes, such as road and vegetation, have rather low accuracies due to their spectral similarity with some other classes in high-resolution images. For the LWIR image, the classification performance of road achieves a markedly good accuracy of 90.9%. This can be attributed to the fact that the class road exhibits unique thermal radiation characteristics in the LWIR image.
Table 2. Class-specific accuracies of spectral classification for optical and LWIR image.
3.2.2. Spectral-spatial classification
The SVM classification results for the spectral-spatial classification are listed in Table and AAs over spectral-based classification are shown in Figure . As shown in Figure , the integration of spatial features allows significant improvements for both the optical and LWIR image. For the optical image, the textural features (GLCM and 3D DWT) outperformed the other spatial features, according to the average accuracies. For the LWIR image, all the spatial features have shown comparable capabilities for the classification improvement.
Table 3. Class-specific accuracies of spectral-spatial classifications for both optical and LWIR image.
Figure 2. Percentage of improvement for the AA obtained by the spectral-spatial classification methods, compared to the raw spectral-based method.
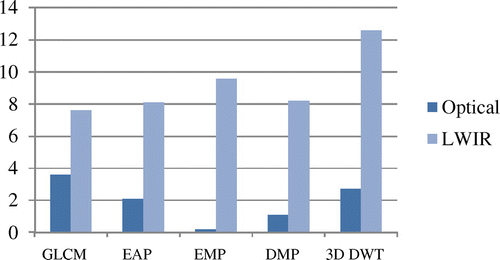
When a careful analysis is done on the class-specific accuracies, excellent class accuracies are shown. Particularly, in Table , the classification performance of class road reaches an accuracy of 96.5% using EAP, trees 92.7% using EMP, red roof 86.5% and gray roof 93.6% using GLCM, concrete roof 87.1% using EMP, and finally, bare soil 89.0% using EAP. Accordingly, it can be concluded that for each information class there are specific spatial features that are helpful for their identification.
3.2.3. Opt-LWIR joint classification
The SVM classification results for the joint classification are shown in Tables and , with the corresponding spatial features calculated based on the first PCA of the optical and LWIR image, respectively. The “spectral” denotes the spectral-joint classification of this two data sources, while the “GLCM”, “EAP”, “EMP”, “DMP”, and “3D DWT” represent the corresponding spatial features involved in the joint classification.
Table 4. Class-specific accuracies for the joint classification optical and LWIR image (with spatial features calculated on the first PC of optical image).
Table 5. Class-specific accuracies for the joint classification optical and LWIR Image (with spatial features calculated on the first PC of LWIR image).
It can be learned from Table that the integration of LWIR information leads to better global results, compared with the optical spectral and spectral-spatial classifications. And when we use the spatial features generated from the first PC of optical image, the improvements are more obvious. Regarding the class-specific accuracies, the classification performance of trees, red roof, concrete roof, and vegetation achieve the highest accuracies than ever before. Table shows when spatial features are calculated based on LWIR information, the overall performance of the joint classification is not as satisfactory as the former. An exception is the DMP features calculated based on LWIR information which promotes the optical spectral classification. Surprisingly, in this case, concrete roof achieves the highest accuracy. To sum up, spatial features based on optical information are more effective than on LWIR information for the majority class discrimination.
3.2.4. Multiple features classification
The classification accuracies for the multiple features classification are listed in Table . It can be seen from the global classification that the decision fusion shows the superiority in implementing multiple feature classification over the feature stacking.
Table 6. Class-specific accuracies for the multiple features classification.
It is also obvious that multiple feature classification has average increments of 2% in terms of AA, compared with the best results in spectral-spatial classification. Surprisingly, the classification of stacked features gives trees and vegetation wonderful recognition accuracies, especially for the vegetation, showing an unprecedented good result for all of the classifications results in our research.
3.2.5. Object-based classification
Accuracies for the object-based classification are listed in Table , and preferable classification maps are displayed in Figure . As expected, the effectiveness of our classification framework is demonstrated by the far more accurate class recognitions. And the GLCM textures are proved to be the most helpful spatial features for the classification of this data-set, as AA being equally matched with the multiple features classification. However, the good accuracy of trees and vegetation that generated by stacking is weakened by object-based classification. Thus, object-based approach is more adapted to classes that have regular shapes such as road and roofs.
Table 7. Class-specific accuracies for the object-based classification.
Figure 3. Classification maps for the object-based classification: (a) Multiple features classification map using majority voting; (b) Multiple features classification map using posterior probability; (c) Multiple features classification map using uncertainty; (d) Spectral-spatial classification map using GLCM textures; (e) Joint classification map using GLCM textures.
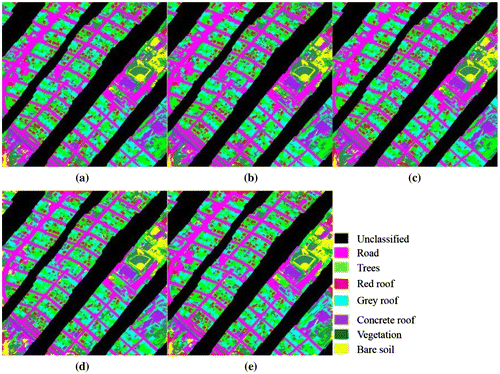
4. Conclusions
In this paper, we addressed the challenge of using VHR data in urban mapping, and proposed a combined imagery methodology framework which is based on the optical and LWIR image. In which, the spectral, spatial, and multi-senor information are simultaneously taken into account. Experiments are conducted on a two levels classification framework using the 0.2 m spatial-resolution optical image and 1 m resolution LWIR image.
Level 1: Classifications at low resolution.
The optical image is down-sampled firstly to fit the size of LWIR image. To examine the contribution of the optical and LWIR image in the classification, four types of classifications are applied on the two data sources. We have illustrated the performance of the framework in both the global accuracies and the class-specific accuracies. Some important observations resulting from this level can be summarized as follows:
(1) | Spectral-based classification only. Experiments prove that the two data sources do not show preferable performances according to the global accuracy. But the LWIR image has the potential value for better identifying of the Road class. | ||||
(2) | Spectral-spatial classification. Spatial features such as GLCM texture, EAP, EMP, DMP, and 3D DWT texture are compared in the spectral-spatial process for the optical and LWIR image, respectively. Experiments show that both the two data sources exhibit better classification accuracies, while taking spatial features into consideration. And particularly, textural features are more effective than the MPs for the optical image accuracy increments. Further, for each class, there exist the specific spatial features that are best suitable for the recognition. | ||||
(3) | Joint classification. To overcome drawbacks brought by single data source, optical and LWIR image with their respective spatial features are classified together. Experiments show that joint classification can greatly improve the optical classification (some classes reaching best accuracies). Furthermore, according to the global accuracy, the spatial features calculated based on optical information are proved to be more effective than that of LWIR information. But the LWIR-based spatial features are suitable for the identification of some specific class, such as the class concrete roof for this data-set. | ||||
(4) | Multiple features classification. To have a combined usage of various spatial features, multiple feature classification is implemented in our experiments, and the results show that far more accurate accuracy are obtained. Besides, the decision fusion displays the superiority in the global classification over the feature stacking. But stacking all the spatial features for the classification gives great accuracies for some specific classes, such as the class trees and vegetation in our experiments. |
Level 2: Projecting classification maps to the high resolution.
To recover the original size of the classification map, the object-based approach is implemented to retain the edge information with multi-resolution segmentation map regarding as the boundary reference. Experiments show good result of the object-based up-sampling methods, in terms of the global accuracy. However, the accuracies proved that classes with regular shapes are more adapted to the object-based approach.
The proposed urban mapping framework demonstrates that the LWIR data are effective complementary information for land cover task. It is evident that thermal remote sensing is potentially a powerful tool for examining land cover and is particularly suited for some specific class recognition. This is essential within the research area of global change where the current land cover must be accurately monitored in order to better determine the potential future change. As a result, the thermal data are an increasingly important component in remote sensing research. It still remains necessary to be analyzed extensively.
Funding
This study is supported by the National Key Research and Development Program of China [grant number 2016YFC080310909].
Notes on contributors
Xuehua Guan is an assistant research engineer with Twenty-First Century Aerospace Technology Co., Ltd (21AT). Her research interests include deep learning, multi/hyperspectral image classification and applications.
Shuai Liao is an assistant engineer of Beijing Remote Sensing Information Institute. His research interests include remote sensing and geographic information integration, radar signal recognition.
Jie Bai is senior engineer of China TOPRS Technology Co., Ltd. Her research interest includes resource, environment monitoring with multi-sources satellite data, application of aerospace data.
Fei Wang is an assistant engineer of Chinese Academy of Surveying and Mapping. Her research interests include image information extraction, 3D data engine design and visualization.
Zhixin Li has the bachelor’s degree of Engineering (remote sensing) in Wuhan University and now is pursuing the master’s degree in machine learning at Purdue University, USA.
Qiang Wen is a vice-general manager and senior project manager with 21AT. He is responsible for remote sensing research, application and service activities. He is mainly engaged in data delivery and management service based on remote sensing and geographic information system.
Jianjun He is a chief technology officer with 21AT. He is responsible for enterprise technology activities; contribute to research and development remote sensing and geospatial service technologies.
Ting Chen is a senior research engineer with 21AT. She is actively involved in the research and development of remote sensing applications and programs.
Acknowledgment
The authors would like to thank Telops Inc. (Quebec, Canada) for acquiring and providing the data used in this study, the IEEE GRSS Image Analysis and Data Fusion Technical Committee and Dr. Michal Shimoni (Signal and Image Centre, Royal Military Academy, Belgium) for organizing the 2014 Data Fusion Contest, the Centre de Recherche Public Gabriel Lippmann (CRPGL, Luxembourg) and Dr. Martin Schlerf (CRPGL) for their contribution of the Hyper-Cam LWIR sensor, and Dr. Michaela De Martino (University of Genoa, Italy) for her contribution to data preparation.
References
- Benediktsson, J. A., J. A. Palmason, and J. R. Sveinsson. 2005. “Classification of Hyperspectral Data from Urban Areas Based on Extended Morphological Profiles.” IEEE Transactions on Geoscience & Remote Sensing 43 (3): 480–491.10.1109/TGRS.2004.842478
- Bischof, H., W. Schneider, and A. J. Pinz. 1992. “Multispectral Classification of Landsat-Images Using Neural Networks.” IEEE Transactions on Geoscience & Remote Sensing 30 (3): 482–490.10.1109/36.142926
- Dalla Mura, M., J. A. Benediktsson, B. Waske, and L. Bruzzone. 2010. “Morphological Attribute Profiles for the Analysis of Very High Resolution Images.” IEEE Transactions on Geoscience & Remote Sensing 48 (10): 3747–3762.10.1109/TGRS.2010.2048116
- Dalla Mura, M., A. Villa, J. A. Benediktsson, J. Chanussot, and L. Bruzzone. 2011. “Classification of Hyperspectral Images by Using Extended Morphological Attribute Profiles and Independent Component Analysis.” IEEE Geoscience & Remote Sensing Letters 8 (3): 542–546.10.1109/LGRS.2010.2091253
- Fukuda, S., and H. Hirosawa. 1999. “A Wavelet-Based Texture Feature Set Applied to Classification of Multifrequency Polarimetric SAR Images.” IEEE Transactions on Geoscience & Remote Sensing 37 (5): 2282–2286.10.1109/36.789624
- Gao, F., J. Masek, M. Schwaller, and F. Hall. 2006. “On the Blending of the Landsat and MODIS Surface Reflectance: Predicting Daily Landsat Surface Reflectance.” IEEE Transactions on Geoscience & Remote Sensing 44 (8): 2207–2218.
- Guo, X., X. Huang, and L. Zhang. 2014. “Three-Dimensional Wavelet Texture Feature Extraction and Classification for Multi/Hyperspectral Imagery.” IEEE Geoscience & Remote Sensing Letters 11 (12): 2183–2187.
- Huang, X., L. Zhang, and P. Li. 2008. “A Multiscale Feature Fusion Approach for Classification of Very High Resolution Satellite Imagery Based on Wavelet Transform.” International Journal of Remote Sensing 29 (20): 5923–5941.10.1080/01431160802139922
- Huang, X., and L. Zhang. 2009. “A Comparative Study of Spatial Approaches for Urban Mapping Using Hyperspectral ROSIS Images over Pavia City, Northern Italy.” International Journal of Remote Sensing 30 (12): 3205–3221.10.1080/01431160802559046
- Huang, X., L. Zhang, and W. Gong. 2011. “Information Fusion of Aerial Images and LIDAR Data in Urban Areas: Vector-Stacking, Re-Classification and Post-Processing Approaches.” International Journal of Remote Sensing 32 (1): 69–84.10.1080/01431160903439882
- Huang, X., X. Guan, J. A. Benediktsson, L. Zhang, J. Li, A. Plaza, and M. Dalla Mura. 2014. “Multiple Morphological Profiles from Multicomponent-Base Images for Hyperspectral Image Classification.” IEEE Journal of Selected Topics in Applied Earth Observations & Remote Sensing 7 (12): 4653–4669.10.1109/JSTARS.2014.2342281
- Jin, X., and C. H. Davis. 2005. “Automated Building Extraction from High-Resolution Satellite Imagery in Urban Areas Using Structural, Contextual, and Spectral Information.” EURASIP Journal on Advances in Signal Processing 2005 (14): 2196–2206.10.1155/ASP.2005.2196
- Keuchel, J., S. Naumann, M. Heiler, and A. Siegmund. 2003. “Automatic Land Cover Analysis for Tenerife by Supervised Classification Using Remotely Sensed Data.” Remote Sensing of Environment 86 (4): 530–541.10.1016/S0034-4257(03)00130-5
- Khare, M., A. K. S. Kushwaha, R. K. Srivastava, and A. Khare. 2013. “An Approach towards Wavelet Transform Based Multiclass Object Classification.” The IEEE International Conference on Contemporary Computing (IC3), Nodia, India, August 8–10.
- Li, J., J. M. Bioucas-Dias, and A. Plaza. 2012. “Spectral-spatial Hyperspectral Image Segmentation Using Subspace Multinomial Logistic Regression and Markov Random Fields.” IEEE Transactions on Geoscience & Remote Sensing 50 (3): 809–823.10.1109/TGRS.2011.2162649
- Liao, W., R. Bellens, A. Pizurica, W. Philips, and Y. Pi. 2012. “Classification of Hyperspectral Data over Urban Areas Using Directional Morphological Profiles and Semi-Supervised Feature Extraction.” IEEE Journal of Selected Topics in Applied Earth Observations & Remote Sensing 5 (4): 1177–1190.10.1109/JSTARS.2012.2190045
- Lu, D., and Q. Weng. 2005. “Urban Classification Using Full Spectral Information of Landsat ETM+ Imagery in Marion County, Indiana.” Photogrammetric Engineering & Remote Sensing 71 (11): 1275–1284.10.14358/PERS.71.11.1275
- Pacifici, F., M. Chini, and W. J. Emery. 2009. “A Neural Network Approach Using Multi-Scale Textural Metrics from Very High-Resolution Panchromatic Imagery for Urban Land-Use Classification.” Remote Sensing of Environment 113 (6): 1276–1292.10.1016/j.rse.2009.02.014
- Pesaresi, M., and J. A. Benediktsson. 2001. “A New Approach for the Morphological Segmentation of High-Resolution Satellite Imagery.” IEEE Transactions on Geoscience & Remote Sensing 39 (2): 309–320.10.1109/36.905239
- Puissant, A., J. Hirsch, and C. Weber. 2005. “The Utility of Texture Analysis to Improve Per‐pixel Classification for High to Very High Spatial Resolution Imagery.” International Journal of Remote Sensing 26 (4): 733–745.10.1080/01431160512331316838
- Segl, K., S. Roessner, U. Heiden, and H. Kaufmann. 2003. “Fusion of Spectral and Shape Features for Identification of Urban Surface Cover Types Using Reflective and Thermal Hyperspectral Data.” ISPRS Journal of Photogrammetry & Remote Sensing 58 (1–2): 99–112.10.1016/S0924-2716(03)00020-0
- Tuia, D., F. Pacifici, M. Kanevski, and W. J. Emery. 2009. “Classification of Very High Spatial Resolution Imagery Using Mathematical Morphology and Support Vector Machines.” IEEE Transactions on Geoscience & Remote Sensing 47 (11): 3866–3879.10.1109/TGRS.2009.2027895
- Waske, B., and J. A. Benediktsson. 2007. “Fusion of Support Vector Machines for Classification of Multisensor Data.” IEEE Transactions on Geoscience & Remote Sensing 45 (12): 3858–3866.10.1109/TGRS.2007.898446
- Zhang, L., X. Huang, B. Huang, and P. Li. 2006. “A Pixel Shape Index Coupled with Spectral Information for Classification of High Spatial Resolution Remotely Sensed Imagery.” IEEE Transactions on Geoscience & Remote Sensing 44 (10): 2950–2961.10.1109/TGRS.2006.876704