Abstract
The study investigates the potential of UAV-based remote sensing technique for monitoring of Norway spruce health condition in the affected forest areas. The objectives are: (1) to test the applicability of UAV visible an near-infrared (VNIR) and geometrical data based on Z values of point dense cloud (PDC) raster to separate forest species and dead trees in the study area; (2) to explore the relationship between UAV VNIR data and individual spruce health indicators from field sampling; and (3) to explore the possibility of the qualitative classification of spruce health indicators. Analysis based on NDVI and PDC raster was successfully applied for separation of spruce and silver fir, and for identification of dead tree category. Separation between common beech and fir was distinguished by the object-oriented image analysis. NDVI was able to identify the presence of key indicators of spruce health, such as mechanical damage on stems and stem resin exudation linked to honey fungus infestation, while stem damage by peeling was identified at the significance margin. The results contributed to improving separation of coniferous (spruce and fir) tree species based on VNIR and PDC raster UAV data, and newly demonstrated the potential of NDVI for qualitative classification of spruce trees. The proposed methodology can be applicable for monitoring of spruce health condition in the local forest sites.
1. Introduction
Unmanned aerial vehicles (UAV) remote sensing technology has been involved in civil and military industry and the market of services to end users for the last 15 years in Europe. Europe became the world leader in the civil sector, where a significant part of the studies was focused on forestry applications (Foster Citation2015). Torresan et al. (Citation2016) reported that estimation of dendrometric parameters based on UAV data concerns 36% of forestry studies, forest health monitoring and diseases mapping represents 21% of applications, followed by tree species classification (14%), post-fire recovery monitoring and fire measuring (14%), quantification of spatial gaps (7%), and the estimation of post-harvest soil displacement (7%).
Forest ecosystems in Central Europe are frequently hit by disturbances that are attributed mainly to natural factors, such as weather extremes and infestations by insects and fungi (Lorenz and Mues Citation2007; Näsi et al. Citation2015; Seidl, Schelhaas, and Lexer Citation2011). Therefore, the accurate and timely monitoring of stand and tree conditions for forest management is needed. Generally, forest health monitoring and diseases mapping is based on time-consuming field sampling and observations of symptoms on the trunk and foliage (Gothlin, Schroeder, and Lindelow Citation2000; Lausch et al. Citation2013). UAVs have several benefits that make them suitable for forest health monitoring: high-intensity data collection and low operational costs (Tang and Shao Citation2015). Several studies used UAV for forest damage monitoring in Central Europe. Lehmann et al. (Citation2015) detected forest insect damage in German oak stands (Quercus sp.) based on UAV multispectral data. Infested and dead oaks were identified by means of object-based image analysis (OBIA) with the accuracy of 82−85%. Näsi et al. (Citation2015) identified single diseased spruce (Picea abies L. Karst) trees from UAV hyperspectral data in Finland, where the promising results were obtained in spruce classification into healthy, infested and dead trees. A UAV-borne thermal sensor (Smigaj et al. Citation2015) was used to monitor canopy temperature in ailing pine plots (Pinus sylvestris L. and Pinus contorta Dougl.) in Scotland. A moderate positive correlation was obtained between the tree temperature and disease progress. In only one case (Minarik and Langhammer Citation2016), UAV multispectral images were applied for the assessment of spatial and qualitative aspects of forest disturbance in the Czech Republic, in the specific spruce forest area with an extensive forest disturbance and decay. Typically, decline of spruce trees in the Czech forests represents a spotted and uneven pattern. Forest area is fragmented by mosaic of individual stands with varying extent of infestation. Decline in health condition of spruce-dominated stands became more pronounced after extreme drought events in 2003 and 2007, followed by rising temperature during growth season (Jonard et al. Citation2012; Kolar et al. Citation2015). This also applies to Beskydy Mts, SE of the Czech Republic, the domain of the current study. The area is situated east from the large industrial regions of Ostrava, the Czech Republic, and Upper Silesia, Poland, which was historically a significant source of air pollutions during the twentieth century. Although the emission load has decreased considerably during the recent decades, the long-term effects on soils such as acidification and nutrient degradation are notable even today, which contributes to the unfavorable health status and resilience of Norway spruce grown in the region (Cienciala et al. Citation2017; Grodzki Citation2010; Rybníček et al. Citation2010). To conduct monitoring of such forests is difficult using field sampling method and expensive using airborne data acquisition (for the high spatial resolution required for monitoring of individual trees). The UAV methods with a very high spatial and temporal resolution can potentially be a solution for effective monitoring of the spot forest areas and qualitative classification of tree health status.
The aim of this study was to investigate the potential of the UAV-based remote sensing technique for monitoring of spruce trees characterized by spotted and uneven health decline pattern. Specifically, study objectives were: (1) to test the applicability of UAV visible and infrared (VNIR) and geometrical data based on Z values of point dense cloud (PDC raster) to separate forest species in the study area; (2) to explore the relationship between UAV VNIR data and individual spruce health indicators from field observations; and (3) to explore the possibility of the qualitative classification of spruce health indicators.
2. Material and methods
To distinguish the study objectives photogrammetric processing, the classification of trees and tree health indicators identification were performed based on the UAV and field survey data (Figure ).
Figure 1. Framework of the study.
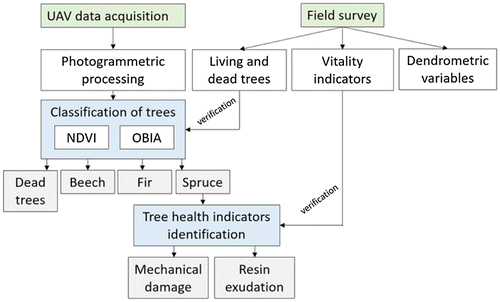
2.1. Study area
The forest sites used in this project were located in the Forest management unit (FMU) Jablunkov, Beskids Mts, NE Czech Republic. The unit includes 12.7 thousand ha of forests and elevation gradient from 350−1050 m a.s.l. (Figure ). Based on the local forest management inventory as of 2008, Norway spruce was a dominant tree species (64%), accompanied mainly with beech (28%), fir (1.5%), and oak (0.6%).
2.2. Data
2.2.1. Field survey
The specific sites selected for this study were two squared plots 50 m × 50 m established at elevation of 700 and 790 m a.s.l (Plot A and Plot B in Figure , respectively) . Both plots represent the typical managed forest stands in the area established by planting. Similarly as elsewhere in FMU Jablunkov, these spruce-dominated forest stands are susceptible to fungal pathogen and bark beetle infestation and their management has recently become problematic (Cienciala et al. Citation2017). As of 2016, the age of these stands were 43 and 54 years, respectively, according to the actual forest management plan. The plots were surveyed in 2010, 2013, and 2016 using Field-Map technology (www.fieldmap.cz) using the laser rangemeter and inclinometer (TruPulse 360, Laser technology, Inc., USA). The surveyed variables included the assessment of living and dead standing trees, fundamental dendrometric variables such diameter at breast height (DBH), tree height, social categorization of trees, and an array of tree vitality indicators. These included those observed at tree stems such as mechanical damage, peeling, resin exudation and its classification in relation to presence of Armillaria ostoyae. Other indicators observed at tree crowns such as foliage discoloration, dead tree top, and reduced tree top shoot increment were noticed, too. The dendrometric characteristics at the plot level are summarized in Table . As it can be observed, both plots were similarly composed with dominant Norway spruce (Picea abies L. Karst.) and significant shares of silver fir (Abies alba Mill.) and European beech (Fagus sylvatica L.).
Table 1. The basic stand dendrometric characteristics including mean tree stem diameter at breast height (DBHm), mean tree height H, stand basal area (G), and stand tree density (N) for the studied plots.
For the purpose of UAV-assisted analysis, the trees of the two plots were merged into one tree sample and treated jointly. This was possible due to the equal variance in tree dimensions in these two plots (DBH, t-test p = 0.523).
2.2.2. UAV survey
Prior UAV survey, the plot was identified in the field and contrast markers were placed on the points visible from the air. These ground control points (GCP) were measured with the real-time kinematics GPS allowing for accuracy approximately in interval of 10 cm mostly due to the mountain (relief) conditions. The images were acquired using commercial Unmanned Aerial System (UAS) the DJI Spreading Wings S-800 equipped with two cameras Sony NEX-5R simultaneously taking pictures. One camera was modified. Its inner IR filter placed on CCD sensor was removed and additional visible light filter HOYA R72 with 720 nm cutoff was placed on the lens. The UAS was driven by computer-based ground station (DJI Ground Station) for autonomous flight in the height of 100 m above terrain level, the constant forward speed of 4 m/s and flight side overlapping 70%. The take-off and landing was done manually for security reasons. Once in the air the autopilot was switched on remotely from the computer for autonomous flying. The pilot position was chosen so as to keep the vehicle constantly in the line of sight. After the flight the images were transformed to the computer from the memory card.
2.3. Photogrammetric processing of UAV data
Photo processing included in the first step the manual evaluation of photo quality when the images with obvious artifacts, high level of blurring or too close to the ground (during take-off and landing) were removed from further processing. The correct images were loaded in the software Agisoft Photoscan©, where they were aligned using default settings for alignment with quality set to high. Next, the resulting model was analyzed again for inconsistent alignments and outlier points. The outlier points were manually removed and the cameras were optimized again for their position estimation using camera optimization process. After this step the most computationally demanding procedure, namely the dense cloud calculation, was executed. This resulted in digital elevation model (DEM) and digital terrain model (DTM) that were extracted from the imager. Both models required an orthorectification of the cloud using the established ground control points. These points were identified using the dense cloud and manually precisely positioned inside each image. The dense cloud was automatically analyzed for ground and other (vegetation) points based on Z values, and DEM and DTM were exported. Finally, the DEM was textured from the imagery and exported as common aerial orthophoto map. We used the mosaicking option for texturing, which ensured no spectral changes in the individual pixels (Panagiotidis et al. Citation2016). The orthophoto was exported with a ground resolution of approximately 5 cm and processed in ArcGIS software using menu Extract values to points, where the individual tree positions measured on the ground were used as the point data. The dense cloud was also imported into ArcGIS environment as PDC raster and processed with the available tool Las Data-set to Raster, where Z value was used as its variation.
2.4. Tree species and dead trees identification from UAV data
2.4.1. Tree species and dead trees identification using vegetation index and PDC raster
NDVI was calculated as the value of (NIR + Red) / (NIR – Red) from VNIR UAV data. The two databases were merged (ground truth data and UAV remote sensed data). NDVI and PDC raster were used as UAV datasets in merged databases for analysis. We used a classical ANOVA followed by pairwise comparison for identifying the significant differences among individual tree categories. The equality of variance was tested prior selecting either Tukey post hoc test for equal variance, or Games–Howell test for unequal variance. All statistical procedures were run using Systat v. 13.1 (Systat Inc., USA).
2.4.2. Tree species and dead trees identification using object-oriented classification
Tree species classification using object-oriented image analysis was performed in two steps in eCognition Developer-6 software (Trimble). In the first step, the object-oriented image analysis approach delineated segments of homogeneous image areas using a multiresolution segmentation algorithm − Fractal Net Evolution Approach (FNEA) (Yu et al. Citation2006). In the second step, the delineation segments were classified to real-world objects based on spectral, textural, neighborhood- and object-specific shape parameters.
FNEA starts with single-pixel objects. The small adjacent objects are merged into the bigger ones based on the smallest growth of heterogeneity, which is defined through both spectral and spatial differences within the object. The merging process stops when the smallest growth exceeds user-defined parameters. The scale parameter defines the maximum allowed heterogeneity for the resulting image objects; color determines the percentage contribution of the spectral values to the homogeneity criterion, as opposed to the percentage of the shape homogeneity defined by the shape parameter. Compactness defines the weight of the compactness criterion, the higher the value, the more compact image objects. The homogeneity criterion for multiresolution segmentation in study area was: shape 0.1, compactness 0.5, and scale parameter 85.
The second step was a standard nearest neighbor classification. Brightness, mean layer, standard deviation, and maximum difference were used as a feature for object classification based on layer values. Objects were classified into 9 classes: “beech”, “beech shadow”, “dead tree”, “fir”, “fir shadow”, “spruce”, “spruce shadow”, “artefacts”, and “surface”. Further, four aggregated classes were used to calculate species classification accuracy metrics: spruce (classes “spruce” and “spruce shadow”), beech (classes “beech” and “beech shadow”), fir (classes “fir” and “fir shadow”) and dead tree.
The species classification was performed two times using different sets of UAV data as inputs: VNIR image (inputted for the first classification), and VNIR image with PDC raster (inputted for the second one). An effect of PDC raster to classification results was estimated by comparison of errors of commission and errors of omission of the classified objects. The errors of commission represent the fraction of values that were predicted to be in a class but do not belong to that class. The errors of omission represent the fraction of values that belong to a class but were predicted to be in a different class. The overall accuracy was calculated by summing the number of correctly classified values and dividing by the total number of values.
2.5. Tree health indicators identification from UAV data
The observed data on tree health including mechanical damage and resin exudation associated with presence of Honey fungus were examined by UVA data. NDVI was calculated (see Section 2.4) the dense point cloud was converted into raster with cell size of ca. 7 cm (depended on the canopy height) using Agisoft Photoscan Create Mesh and Orthophotomap options. The referenced orthophotomap was imported into ESRI Arcmap version 10.4 and overlaid with the terrestrial data containing the positions of individual trees. In ArcGIS, the tool Extract by Points was used to extract the individual spectral information at the position of each tree top. The data included near infrared (NIR) and RGB values, subsequently used for calculation of NDVI.
The complete point clouds from Agisoft (Agisoft Company Citation2016) including the geometrical information, e.g. coordinates X, Y, and Z, were imported to ESRI Arcmap using 3D Analyst Tool – LAS Data-set and processed LAS Point Statistics by Area tool. This tool creates raster file with information about Z variance for each cell.
The statistical tests used to assess significant differences included t-test as implemented in Systat v 13.1 (Systat Inc., USA). Dead trees were excluded from the tested sample.
3. Results
3.1. Tree species and dead trees identification using vegetation index and PDC raster
The tree species identification including dead trees as a specific category using NDVI from UAV resulted in significant differences among these categories (ANOVA, p < 0.001). NDVI proved to be robust enough to separate the dead standing trees from the living trees for all tree species, and captured well the differences between spruce and fir. However, NDVI was not successful in identifying differences between beech and the two present alive coniferous species (spruce and fir) (ANOVA, pairwise comparison). Using PDC raster for detecting tree species category resulted in identifying well spruce from fir and fir from dead trees (ANOVA, pairwise comparison), but the separation of dead standing trees from other living trees was not that robust as in the case of NDVI (Figure ).
Figure 3. Box-and-Whisker plots of NDVI (left) and PDC raster (right) for tree species category including alive specimen of Norway spruce, Silver fir and European beech, and standing dead trees.
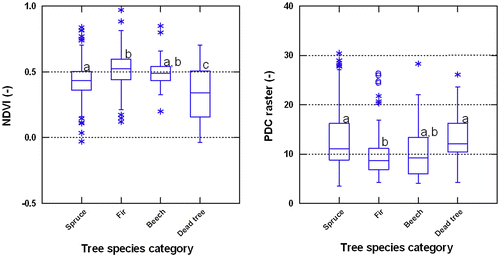
3.2. Tree species and dead trees identification using object-oriented classification
The confusion matrices were calculated based on number of objects demonstrates correct, omission, and commission classifications for each class (Table ). The accuracy metrics are presented in Table .
Table 2. Confusion matrixes of species classification.
Table 3. Species classification accuracy metrics.
3.3. Tree health indicators identification
The UAV data products PDC raster and NDVI were tested against tree health indicators observed on tree stems (Figure ). First, the presence of peeling, sole mechanical damage, and resin exudation on living trees was tested individually using t-test. While PDC raster was showing a significant difference only for mechanical damage (p = 0.020), NDVI was able to identify the presence of both mechanical damage and resin exudation (both at p < 0.050), while the stem damage by peeling was identified at the significance margin (p = 0.054). Similar results were obtained when the presence of any health indicator was used against the trees with no indicator occurring. Also here, NDVI data showed a significant difference between the groups (p = 0.001), whereas PDC raster did not detect any significant difference (p = 0.338).
4. Discussion
4.1. Tree species and dead trees identification
The approach of tree species identification based on NDVI separated well spruce and fir, but was not successful in identifying differences between the two conifers and beech (Figure , left). Separation between spruce and fir was also distinguished based on PDC raster analysis (Figure , right), what could be an advancement of our study in comparison with the previous coniferous tree species identification based on VNIR UAV data. Kuzmin et al. (Citation2016) reported the difficulty to identify coniferous species (spruce and pine) based on VNIR images (2 cm). To succeed with identification between fir and beech using this approach more spectral information is required (Näsi et al. Citation2015). Other reason explaining the difficulties in distinguishing beech from other living tree species, was a limited representation of beech, which was only 3−6% by tree density in the studied stands (Table ).
NDVI is robust enough to separate dead trees from living trees (Figure , left). It proves the potential of NDVI from UAV data to monitor tree health status (Lehmann et al. Citation2015; Näsi et al. Citation2015). Minarik and Langhammer (Citation2016) showed the NDVI ability to separate even the different categories of tree decline (dead, infested, and healthy) using multispectral imaging UAV camera (550, 650, 700, 800, 850, and 900 nm spectral ranges) in the spruce forest with a massive back beetle infestation. PDC raster was successful only in separation of dead trees from fir. Identification of dead tree category from living spruce and beech was not robust (Figure , right).
Tree species classification from the object-oriented analysis separated beech, spruce, fir, and the dead trees on study site (Figure ). Our results (kappa is 0.74, overall accuracies are 0.745 and 0.784) are comparable with the results of the recent studies using object-based classification. The overall accuracy of the classification in five deciduous species composition classes was 79.5% from VNIR UAV data (Michez et al. Citation2016). Species composition of a boreal forest was automatically recognized by the object-oriented image processing from RGB data (spatial resolution of 2 cm) (Kuzmin et al. Citation2016) separating spruce, pine and birch with overall accuracy of 81.9%. The study site (Kuzmin et al. Citation2016) was more homogenous and less dense than our forest area, which could reasonably explain the stable segmentation and classification results and higher overall accuracy. Lehmann et al. (Citation2015) classified oak forest stands to healthy, infested, and dead using object-oriented image analysis with VNIR UAV data (flight altitude was between 20 and 80 m above surface). The overall accuracy and kappa were 0.85 and 0.81, respectively, where the dead trees were partly misclassified to canopy gap class. The classes of healthy, infested, and dead trees were identified by Näsi et al. (Citation2015) for urban spruce-dominated forest using UAV hyperspectral data (spatial resolution was 9 cm). The best overall accuracy was 0.76. In our study, the dead trees were classified without errors of commission and omission (Table ). The inclusion of PDC raster to VNIR images did not improve the classification accuracy (0.745) in comparison with the overall classification accuracy using only VNIR data (0.784). It revealed a marginal improvement in terms of errors of commission for spruce and beech, and errors of omission for fir (Table ). The dead trees were identified without errors for both input sets of data.
4.2. Tree health indicators identification
NDVI was found robust enough in qualitative classification of spruce health. The presence of both mechanical damage and resin exudation was identified based on NDVI (Figure ). The PDC raster did not show any significant difference between the groups of damaged and undamaged trees. Despite numerous studies about tree health monitoring and disease mapping from UAV data, only two papers using qualitative classification of vegetation were identified (Felderhof and Gillieson Citation2011; Lelong et al. Citation2008). In these studies, the mean reflectance of different bands and vegetation indices from UAV VNIR data were compared with field ‘treatments’ of vegetation. Felderhof and Gillieson (Citation2011) reported about the relationship between NDVI values and variability of growth and health status of macadamia trees. Lelong et al. (Citation2008) reported a strong relationship between the conditions of wheat crop and NDVI from UAV images. Our results newly demonstrate the potential of NDVI from VNIR UAV data for qualitative classification of spruce trees to identify mechanical damage and resin exudation indicators and hence extend the use of UAV data for forest health monitoring in the spot forest areas.
5. Conclusions
The study demonstrates the extended potential of UAV data for forest monitoring. Analysis based on NDVI and PDC raster (Z values of point dense cloud) was successfully applied for the separation of spruce and fir, and for identification of dead tree category. The object-oriented image analysis classified confidently all categories of trees: spruce, beech, fir, and dead trees. Qualitative classification of spruce trees based on NDVI values resulted in identification of mechanical damage and resin exudation indicators. The results contributed to improved separation of coniferous tree species (spruce and fir) based on VNIR and PDC raster UAV data, and newly demonstrated the potential of NDVI for qualitative classification of spruce trees. The proposed methodology can be applicable for monitoring of spruce health indicators in the local forest sites.
Funding
This work was supported by the Ministry of Education, Youth and Sports of the Czech Republic within the National Programme for Sustainability I [grant number LO1415]; partly by EEA Grants if Iceland, Liechtenstein and Norway [grant number EHP-CZ02-OV-1-019-2014].
Notes on contributors
Olga Brovkina is a researcher in the Remote Sensing Department at Global Change Research Institute CAS. She is interested in estimation of forest ecosystem/stand/trees characteristics from satellite and airborne data. More specifically, her work examines forest decline, aboveground biomass, and inventory parameters.
Emil Cienciala is a senior scientist at IFER − Institute of Forest Ecosystem Research. His research interests include forest ecology, ecophysiology, forest management, and forest inventory methods. Recently, he has been working mainly on issues related to GHG emission inventories for the LULUCF sector.
Peter Surový is a researcher in Department of Forest Management in Faculty of Forestry in Czech University of Life Sciences Prague. His research aims include photogrammetry and 3D measurements and modeling of forest ecosystems. Recently, he has been focused on utilization of unmanned aerial vehicles in forestry.
Přemysl Janata is a research assistant in the Department of Forest Management and Applied Geoinformatics at Mendel University in Brno. He is interested in unmanned aerial vehicles and their usage in forestry. He is also interested in photogrammetric reconstruction of forest stands.
References
- Agisoft Company. 2016. Accessed May 15, 2017. www.agisoft.com/
- Cienciala, E., J. Tumajer, V. Zatloukal, J. Beranová, Š. Holá, I. Hůnová, and R. Russ. 2017. “Recent Spruce Decline with Biotic Pathogen Infestation as a Result of Interacting Climate, Deposition and Soil Variables.” European Journal of Forest Research 136 (2): 307–317. doi:10.1007/s10342-017-1032-9.
- Felderhof, L., and D. Gillieson. 2011. “Near-infrared Imagery from Unmanned Aerial Systems and Satellites can be Used to Specify Fertilizer Application Rates in Tree Crops.” Canadian Journal of Remote Sensing 37 (4): 376–386.
- Foster, J. 2015. Draft Report on Safe Use of Remotely Piloted Aircraft Systems (RPAS), Commonly Known as Unmanned Aerial Vehicles (UAVs), in the Field of Civil Aviation (2014/2243(INI)). Committee on Transport and Tourism, European Parliament, 2014−2019.
- Gothlin, E., L. M. Schroeder, and A. Lindelow. 2000. “Attacks by Ips typographus and Pityogenes chalcographus on Windthrown Spruce Spruces (Picea abies) during the Two Years following a Storm Felling.” Scandinavian Journal of Forest Research 15: 542–549.10.1080/028275800750173492
- Grodzki, W. 2010. “The Decline of Norway Spruce Stands in Beskid Slaski and Zywiecki: Theoretical Concept and Reality.” Beskydy 3 (1): 19–26.
- Jonard, M., A. Legout, M. Nikolas, M. Dambrine, C. Nys, E. Ulrich, R. Perre, and Q. Ponette. 2012. “Deterioration of Norway Spruce Vitality Despite a Sharp Decline in Acid Deposition: A Long-term Integrated Perspective.” Global Change Biology 18 (2): 711–725.
- Kolar, T., P. Cermak, F. Oulehle, M. Trnka, P. Stepanek, P. Cudlin, J. Hruska, U. Buntgen, and M. Rybnicek. 2015. “Pollution Control Enchanced Spruce Growth in the ‘Black Triangle’ Near the Czech-Polish Border.” Science of the Total Environment 538: 703–711.
- Kuzmin, A., L. Korhonen, T. Manninen, and M. Maltamo. 2016. “Automatic Segment-level Tree Species Recognition Using High Resolution Aerial Winter Imagery.” European Journal of Remote Sensing 49: 239–259.10.5721/EuJRS20164914
- Lausch, A., M. Heurich, D. Gordalla, H.-J. Dobner, S. Gwillym-Margianto, and C. Salbach. 2013. “Forecasting Potential Bark Beetle Outbreaks Based on Spruce Forest Vitality Using Hyperspectral Remote-sensing Techniques at Different Scales.” Forest Ecology and Management 308: 36–89.
- Lehmann, J. R., F. Nieberding, T. Prinz, and C. Knoth. 2015. “Analysis of Unmanned Aerial System-based CIR Images in Forestry – A New Perspective to Monitor Pest Infestation Levels.” Forests 6 (3): 594–612.10.3390/f6030594
- Lelong, C. C. D., F. Burger, G. Jubelin, B. Roux, S. Labbe, and F. Baret. 2008. “Assessment of Unmanned Aerial Vehicles Imagery for Quantitative Monitoring of Wheat Crop in Small Plots.” Sensors 8 (5): 3557–3585.10.3390/s8053557
- Lorenz, M., and V. Mues. 2007. “Forest Health Status in Europe.” The Scientific World Journal 7: 22–27.10.1100/tsw.2007.17
- Michez, A., H. Piegay, J. Lisein, H. Claessens, and P. Lejeune. 2016. “Classification of Riparian Forest Species and Health Conditions Using Muti-temporal and Hyperspectral Imagery from Unmanned Aerial System.” Environmental Monitoring and Assessment 188: 146–343165.10.1007/s10661-015-4996-2
- Minarik, R., and J. Langhammer. 2016. “Use of a Multispectral UAV Photogrammetry for Detection and Tracking of Forest Disturbance Dynamics.” The International Archives of Photogrammetry, RS and Spatial Informational Science. Vol. XLI-B8, XXIII ISPRS Congress, Prague. 1−8.
- Näsi, R., E. Honkavaara, P. Lyytikanen-Saarenmaa, M. Blomqvist, P. Litkey, T. Hakala, N. Viljanen, T. Kantola, T. Tanhuanpää, and M. Holopainen. 2015. “Using UAV-based Photogrammetry and Hyperspectral Imaging for Mapping Bark Beetle Damage at Tree-level.” Remote Sensing 7 (12): 15467–15493.10.3390/rs71115467
- Panagiotidis, D., A. Abdollahnejad, P. Surový, and V. Chiteculo. 2016. “Determining Tree Height and Crown Diameter from High-resolution UAV Imagery.” International Journal of Remote Sensing 38 (8–10): 2392–2410.
- Rybníček, M., P. Cermak, T. Zid, and T. Kolar. 2010. “Radial Growth and Health Condition of Norway Spruce Stands in Relation to Climate (Silesian Beskids, Czech Republic).” Geochronometria 36: 9–16.
- Seidl, R., M. J. Schelhaas, and M. Lexer. 2011. “Unraveling the Drivers of Intensifying Forest Disturbance Regimes in Europe.” Global Change Biology 17 (9): 2842–2852.10.1111/gcb.v17.9
- Smigaj, M., R. Gaulton, S. L. Barr, and J. C. Suarez. 2015. “UAV-borne Thermal Imaging for Forest Health Monitoring: Detection of Disease-induced Canopy Temperature Increase.” The International Archives of Photogrammetry, Remote Sensing and Spatial Information Sciences XL-3/W3: 349–354.
- Tang, L., and G. Shao. 2015. “Drone Remote Sensing for Forestry Research and Practices.” Journal of Forestry Research 26 (4): 791–797.10.1007/s11676-015-0088-y
- Torresan, Ch, A. Berton, F. Carotenuto, S. Filippo, S. F. Gennaro, B. Gioli, A. Matese, et al. 2016. “Forestry Applications of UAVs in Europe: A Review.” International Journal of Remote Sensing 38 (8–10): 2427–2447.
- Yu, Q., P. Gong, N. Clinton, G. Biging, M. Kelly, and D. Schironkauer. 2006. “Object-based Detailed Vegetation Classification with Airborne High Spatial Resolution Remote Sensing Imagery.” Photogrammetric Engineering and Remote Sensing 72 (7): 799–812.10.14358/PERS.72.7.799