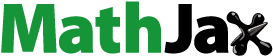
ABSTRACT
Previous studies generally used land use and travel flow to investigate the efficiency of the railway system in Transit-Oriented Development (TOD) cities. Furthermore, to study the association of land use and jobs–housing distribution of commuters, we can find out the potential development of rail commuting. In this research, four railway lines in Wuhan, China, were selected to explore the land use in promoting practical commuting population according to the smart-card data obtained. For land use issues, except the road density and building density, a Normalized Location-Weighted Landscape Index (NLWLI) based on the source – sink theory in landscape ecology was established to assess the jobs–housing land use around rail transit stations. Meanwhile, employment and housing details of commuters around rail transit stations were identified using smart-card data. We found that the generation of commuting flow was affected by building density and the land use of employment in the immediate vicinity of rail transit stations. The distribution of building density and commuters in a mature rail line was roughly a normal distribution. However, due to the inconsistency of land use and jobs – housing distribution around stations, the requirement of the balance of land use and jobs – housing distribution should be reduced at the scale of rail stations in the TOD planning systems. This study is the application of massive smart-card data in the field of urban research. It identifies land use issues that affect rail transit commuting flow, and can help urban planners improve the efficiency of rail transit through planning and design.
1. Introduction
Due to extremely large sizes and populations of urban areas, there is a serious shortage of urban public transport in many places around the world, which has contributed to the increase in private cars usage (Guerra et al. Citation2018; Mouratidis, Ettema, and Næss Citation2019). Alongside the expansion of the travel range of urban residents by vehicles, traffic congestion has reduced the travel efficiency (Engelfriet, and Koomen Citation2018). Furthermore, vehicles have been and continue to be one of the main sources of air pollutants (Gately et al. Citation2017). For these reasons, clean and expanded heavy-duty public rail transport is urgently needed. In the road network system, railways are the main axes for the development of cities which follow a Transit-Oriented Development (TOD) plan (hereafter referred to as “TOD cities”) (Loo, Chen, and Chan Citation2010; Papa, and Bertolini Citation2015; Vale Citation2015; Lyu, Bertolini, and Pfeffer Citation2016; Ma et al. Citation2018; Staricco, and Brovarone Citation2018). Mustafa et al. (Citation2018) found that the developments of medium- and high-density built-up areas are likely to be located near train stations. The original purpose of TOD aimed at facilitate people to live near transit services, then reduces their dependence on driving (Carlton Citation2009). Numerous studies have proved that development of railway lines and stations can help to reduce the number of cars on the road, thereby reducing the environmental impact of cars, slowing down the growth of traffic congestion, and advancing sustainable urban development (Nasri, and Zhang Citation2014; Ahmad et al. Citation2016; Ma et al. Citation2017; Huang et al. Citation2019). With the recognition of the importance of TOD, there were 41 cities running rail transit in China’s mainland as of December 2019 according to Citation2019 China Urban Construction Statistical Yearbook.
TOD schemes involve multiple scales and elements of a city. According to “TOD implementation resources and tools”, the scales of TOD are city-region, corridor, station area, and site level (Ollivier, Kalra, and Ghate Citation2018). Generally, station areas (i.e. the areas around transit nodes) are the most critical areas to provide concentrated, diversified, walkable, and bicycle-friendly services of living, working, shopping, entertainment, etc. (Higgins, and Kanaroglou Citation2016; Lyu, Bertolini, and Pfeffer Citation2016; Zhao, and Li Citation2017; Zuo, Wei, and Rohne Citation2018). However, sustainable implementation of such integration remains to challenge (Staricco, and Brovarone Citation2018; Zhao et al. Citation2018; Nigro, Bertolini, and Moccia Citation2019). Land use is the carrier of varied functions and the most fundamental factor affecting the development of rail transit (Zhao et al. Citation2018; Shen et al. Citation2020). Although there is no standard paradigm for urban design around railway lines and stations, studies have encouraged the high-density development of mixed land use for the purposes of employment, business, education, residence, and culture, in order to increase the vitality and efficiency of railway stations (Lyu, Bertolini, and Pfeffer Citation2016; Ewing et al. Citation2017). It is believed that the greater the diversity of land use, the better the utilization of transit (Sahu Citation2018). Together with land use factor, social population, and public transport services are also the most important ones in the land use design model of station-level planning (Ma et al. Citation2018; Sahu Citation2018). Generally, land use indices established mainly include three aspects: land use area, building density, and land use diversity (Lin, and Gau Citation2006; Vale Citation2015). At present, the land diversity is often indicated by the degree of mixed land use (Sahu Citation2018), which ignores the functional orientation of land use, and there would be a variety of uncertain combination of land use. Population indices include residential density and employment density (Canepa Citation2007); and public transport services include the intersection density, dead-end road density, and accessibility (Kamruzzaman et al. Citation2014; Tasic, Zhou, and Zlatkovic Citation2014). Meanwhile, descriptive and quantifiable indices based on TOD planning factors are also used to evaluate, classify, or sort stations in terms of pedestrian mobility, safety, convenience, comfort, and environmental friendliness (Higgins, and Kanaroglou Citation2016; Lyu, Bertolini, and Pfeffer Citation2016).
Rail transit station areas should gather a variety of functions, and one of their important roles is for commuting. For the spatial restructuring of mega cities has led to the distribution of jobs and housing separated (Zhang, Zhou, and Zhang Citation2017), residents tend to preferentially choose high-efficiency rail transit for commuting in TOD cities. Since land use and transportation influence commuting patterns (Guerra et al. Citation2018), jobs and housing surrounding railways and railway stations will greatly improve the urban commuting efficiency (Zhou, Chen, and Zhang Citation2016). Zamir et al. (Citation2014) found that residents of TOD cities tend to make shorter trips by all kinds of transportation and have a higher probability of choosing employment closer to transit hubs. Then, locating housing and jobs near the railway network promotes the accessibility of railways (Papa, and Bertolini Citation2015). Meanwhile, the presence of railway lines promotes the growth of commercial land (Lavery, and Kanaroglou Citation2012; Bhattacharjee, and Goetz Citation2016), and the cost of housing and leasing along rail lines will increase. However, this increase of cost will be offset by lower transportation costs (Renne et al. Citation2016). Therefore, the areas around rail stations are a great attraction for large investment of housing projects. For example, in Hong Kong, China, the “Rail + Property” development project has greatly increased the ridership of rail transit and housing prices (Cervero, and Murakami Citation2009). Furthermore, successful TOD planning at the regional and corridor levels, transit-oriented housing projects in Washington, D.C., and Portland, Oregon have particularly brought about low rates of vehicle trips (Cervero, and Arrington Citation2008). Therefore, housing density, employment density, and urban design along railway lines have significant impact on the transit efficiency and future urban growth (Canepa Citation2007).
For the distribution of jobs and housing in the city, jobs – housing balance refers to a situation where there is a similar number of jobs and housing. This is an important concept that has been proposed to assist in the reduction of travel flow and increase the continued vitality of area throughout the day, which partly coincides with the goal of TOD. The jobs – housing ratio is usually used to measure the jobs – housing balance in traffic analysis zones, neighborhoods, and administrative boundaries, such as streets, districts, and counties (Yan et al. Citation2018). The larger the scale of the research unit, the easier it is to achieve complete jobs – housing balance. However, Wu, Zhang, and Yang (Citation2015) considered that micro-level measurement is more likely to reflect the relationship between travel behavior and land use pattern. In terms of the definition of the jobs – housing ratio, the “housing” part in the ratio may be defined as households, housing units, employed residents, and working-age population. The definition of housing should be changed according to the problem being studied. Generally, the ratio of jobs to employed residents is the most accurate way to quantify the jobs – housing balance (Wu, Zhang, and Yang Citation2015). And the value of jobs – housing ratio of balanced jobs – housing distribution is generally considered to lie between 0.8 and 1.2 (Park, and Kwon Citation2018). However, jobs – housing ratio is prone to extreme values, and its range needs to be adjusted based on the mean jobs – housing ratio of the whole city. Therefore, some advanced indices have been proposed to determine the jobs – housing balance, such as the D metric of Charron (Citation2007) and the zero-one index of Song et al. (Citation2019). Along with the increasing availability of jobs and housing data and clear application scenarios, an increasing number of indices of the jobs – housing balance are likely to be developed and play an important role in urban planning.
Exploring the association of land use with jobs – housing distribution could assist in the development of TOD cities and improve the efficiency of rail transit commuting by land use planning. Previous studies have shown that the variation of passenger flow in rail transit stations with time is closely related to land use type around station (Loo, Chen, and Chan Citation2010; Sung, and Oh Citation2011; Gong, Lin, and Duan Citation2017). Rail traffic volume is an important criterion for the planning, design, and management of rail traffic in turn (Feng et al. Citation2017; Kim, Lim, and Kim Citation2018; Tu et al. Citation2018). According to the driving force of ridership generation, source – sink landscape theory provides a useful theoretical framework for the analysis of transportation (Brady, and Richardson Citation2017; Wu et al. Citation2017). Traffic source – sink areas is related to residential land use, commercial land use, industrial land use, and others, and the source or sink area transforms dynamically throughout a day (Liu et al. Citation2012). For commuters by rail transit, it is certain that the place of residence related to residential land use (R) is the “source” and workplace related to the type of land used for employment is the “sink”. The “sink” landscape in this research includes land used for public management and public service facilities (A), land used for commercial service facilities (B), and land used for industry (M). Meanwhile, Smart-card data can provide to an extent to identify the rail commuters, and the number of jobs and housing near rail transit stations.
Therefore, to study how to play the commuting role of rail transit through the land use layout at the station level, this study takes Wuhan, China, as an example, aiming to (1) analyze the distribution of employment and residential land use from the viewpoint of landscape ecology, (2) use large quantity of smart-card data to reflect the overall characters of rail commuting, and (3) explore how the land use affects the number of jobs and residents around the rail transit stations. The primary contribution of this study is: Normalized Location-Weighted Landscape Index (NLWLI) is proposed to evaluate jobs – housing land use around rail stations based on the source – sink theory, and the index would be an option in the absence of working and housing population data to calculate jobs – housing ratio, which would be suitable to similar problems on other scales. The remainder of this paper is organized as follows: Section 2 outlines Wuhan’s transit-oriented development; Section 3 describes the methods of land use issues and commuters by rail transit identified from smart-card data; Section 4 presents the results; Section 5 presents some discussion; and Section 6 presents a conclusion.
2. Wuhan’s transit-oriented development
Wuhan, a mega city in central China with a population of more than 10 million, has experienced a period of centralized land expansion (Lu et al. Citation2014). With territory of 8569.15 km2, it is in urgent need of development of public transport of rail transit. In fact, the construction of rail transit in Wuhan was first formally proposed in 1992, with one light rail. After that, it is gradually proposed to build systematic rail transit networks, and comprehensively improve the proportion of rail transit in public transportation and the coverage rate of population around stations. By the end of 2019, there were nine rail transit lines in operation with the length of 335.2 km (China Tansport Statistical Yearbook Citation2019). Line 1 was first opened in 2004, followed by Line 2 in 2012, Line 4 in 2013, Line 3 in 2015, Line 6 in 2016, Line 8 and Yangluo in 2017, and Line 7 and 11 in 2018. Line 1 to Line 4 were selected for this study according to smart-card data of August 2016 (). Line 1 was from Dongwu Avenue to Zhuyehai, Line 2 was from Jinyintan to Optics Valley Square, Line 3 was from Zhuanyang Avenue to Hongtu Avenue, and Line 4 was from Huangjingkou to Wuhan Railway Station (Appendix A). And Line 1 and Line 4 have no interchange stations. Relevant government departments and scholars put forward that Wuhan’s transportation system would be coordinated with intensive land development, so a set of comprehensive rail transit planning system has been formed for the planning, development, and management of rail transit. Planning usually puts forward strategies and conceptual schemes, but its implementation is considered to have a gap. There are many reasons for this gap, the main reasons are restrictions on the development of land along the rail transit such as the severe shortage of land supply in the central city, and not sufficient research on the reasonable structure and intensity of land use around the stations. Other factors including culture, architecture, design, and so on. In the actual case of TOD in Wuhan, the pattern of “Rail + Property” has been introduced, and it is still in the initial stage.
Wuhan has a multi-center urban spatial form (Jiao et al. Citation2017). Due to historical and geographical reasons, local people habitually divide Wuhan into three towns of Hankou, Wuchang, and Hanyang (). Compared with private transportation, public transport is closely related to the dispersion or concentration of urban areas (Loo, Chen, and Chan Citation2010; Staricco, and Brovarone Citation2018; Vale, Viana, and Pereira Citation2018). The single-center or multi-center urban form affects the population distribution and employment density (Dadashpoor, and Alidadi Citation2017; Huang et al. Citation2017; Moghadam et al. Citation2018), and then affects commuting choices (Marcińczak, and Bartosiewicz Citation2018). Generally, no matter single-center city or multi-center city, employment opportunities are concentrated in the main urban areas, and new residential areas locate in the suburbs. Connecting employment centers and suburban residential areas by rail transit will save workers’ commuting time (Han et al. Citation2015; Lin, Allan, and Cui Citation2016). And developing employment centers around rail stations is essential to improve urban productivity (Angel, and Blei Citation2016; Bothe, Hansen, and Winther Citation2018).
3. Methods
The study of the association between land use and jobs – housing distribution mainly includes two parts (). On the one hand, the density and structure indices are used to evaluate the land use within the vicinity of the rail transit stations. On the other hand, commuters are identified from smart-card data, and the characteristics of the jobs – housing distribution and commuting flow are analyzed. According to the above results, Pearson correlation coefficient is used to analyze the correlation between land use and jobs – housing distribution.
3.1. Land use issues
TOD guidelines not only contain factors such as density, diversity, distance, design, but also can be enriched in more research (Sahu Citation2018). Density and structure are two aspects selected to evaluate the land use around rail transit stations in commuting, where density refers to the intensity of land use and structure refers to the diversity of land use types and their combinations as follows in this study.
Density. Firstly, TOD encourages orderly connections between rail transit and other public transportation. The higher the density of road network, the smaller the block, and the more conducive to other public transportation and pedestrian accessibility (Kamruzzaman et al. Citation2014) Secondly, building density could represent the compactness and efficiency of land use, and the urban centers and traffic arteries tend to have higher building density (Lin, and Gau Citation2006; Jiao et al. Citation2017). Therefore, for the assessment of density of land use, two indices including road density and building density are selected in this study.
Structure. As for the structure of land use, it is generally believed that the higher mixed degree, the greater diversity of land use (Sahu Citation2018). We believe that there are differences between mixed land use and diversity. With the same type of land use, the mixed degree and dominant function of land use may be different. In this study, land use of residence and employment affecting rail commuting is clear. Therefore, a proposed index based on “source – sink” theory is adopted in this study to assess the structure of land use. The newly developed NLWLI in this study refers to the ratio of difference and sum of influence index of “sink” landscape and influence index of “source” landscape.
Chen et al. (Citation2009) put forward the Location-Weighted Landscape Index (LWLI) to quantitatively calculate the influence of the “source” landscape and “sink” landscape during ecological processes, such as air pollution, soil erosion, and the generation of heat islands. The LWLI has a value between 0 and 1 (Equation (Equation1(1)
(1) )). In order to determine the relative dominance of “source” and “sink” landscapes, this study normalized the LWLI to [−1, 1] using Equation (Equation2
(2)
(2) ). This index is termed the normalized LWLI (NLWLI), which helps to compare with the jobs – housing ratio. If the NLWLI is greater than 0, the “sink” landscape is dominant; if it is less than 0, the “source” landscape is dominant; and if it is close to 0, the influence of “source” and “sink” landscapes are relatively balanced.
where is the influence index of the “sink” landscape;
is the influence index of the “source” landscape; A_Sinki represents the area of irregular triangular formed by the curve of accumulated area ratio, and the normalized accumulated land area from 0 to 1 as the vertical axis, and the normalized distance of service radius from rail transit station as the horizontal axis of “sink” landscape i (). Accordingly, A_Sourcej represents the area of irregular triangular of “source” landscape j. Since the service radius of Wuhan’s rail transit stations are usually 500–800 m, here we choose a small scale of 500 m for land use analysis. Wi and Wj represent the weights of “sink” landscape i and “source” landscape j, respectively. In this study, in the process of analyzing land use for residential and employment, the residential land use is considered as the “source” landscape, then Wj is 1, the number of types of “source” landscapes n is 1. Meanwhile, there are three types of “sink” landscapes, including public management and public service facilities (A), commercial service facilities (B), and industry (M), then the number of types of “source” landscapes m is 3. As there is per capita index of individual land use in the city, the weights of the A, M, and B land use types are determined by the ratios of the average employment densities in these land use types. According to the Citation2017 China Statistical Yearbook and economic census data of 2013, in Wuhan city, there are about 441,700 public service practitioners, including medical, governmental, and educational, 1 million factory practitioners, and 3.56 million commercial service practitioners. Accordingly, the weights of the A, M, and B land use types are 0.05, 0.05, and 0.90, respectively. Pi and Pj represent the percentage of the area of “sink” landscape i and “source” landscape j in the area of the circle with a radius of 500 m.
3.2. Commuters by rail transit identified from smart-card data
Smart-card data not only helps to analyze day-to-day variability of transit usage, but also discover commuting use through periodic changes (Sari Aslam, Cheng, and Cheshire Citation2019; Egu, and Bonnel Citation2020), and predict potential trip purpose (Sari Aslam et al. Citation2021; Zhang, Zhang, and He Citation2021). In order to reflect character of rail commuting in Wuhan, we collected more than six million transit records during five workdays from 1 to 5 August 2016 of smart-card data of Line 1, Line 2, Line 3, and Line 4 from the government agency. The records contained the following variables: (1) card ID number—“Cardid”; (2) the station number of the boarding and alighting stations—“BoardID” and “AlightID”, respectively; (3) the year, date, and day of travel—“Year”, “Date”, “Weekday”; and (4) the hour and second of boarding and alighting—“BoardHour” and “BoardSecond”, and “AlightHour” and “AlightSecond”, respectively. In addition to the repeated records from “BoardID” to “AlightID” of commuters in a fixed interval, the original database contained large number of irregular records, such as one-way trips, trips with multiple “BoardID” and “AlightID” variables, etc. Therefore, in order to identify the stations that commuters used to travel to and from work among the smart-card records, the original database was cleared and converted. This was achieved as follows:
For the trip records with “BoardID” and “AlightID”, cardholders who completed a certain trip more than three times within five days are considered as potential commuters. Although 1 August 2016 was the Army Day, and Active military personnels have half a day off, this event didn’t impact on this study. The filtered records mainly include the three trip cases shown in . The scenario shown in involves multiple trips with short stops at intermediate stations, which makes it difficult to determine the residence and workplace of the cardholders. Therefore, only the trip scenarios in are used to identify commuters and their residence and workplace.
Some cardholders completing the one-way trip type shown in have two or more different travel intervals, and it is therefore impossible to determine where they live and work without any auxiliary information. Thus, these records are removed. The remaining records of one-way trips are assumed to represent commuting to and from work. Commuters who travel in the morning are assumed to live near station 1—i.e. that represented by “BoardID”—and work near station 2—i.e. that represented by “AlightID”—while commuters who travel after 16:00 are assumed to live near station 2 and work near station 1.
The scenario shown in is the most typical type of commuter trip. For this scenario, the first “BoardID” of one day is also the last “AlightID” of the day, and there are two cases: (1) the cardholder leaves in the morning, returns in the evening, stays at their workplace for more than 6–8 h, and there is a round trip per day; and (2) the cardholder makes multiple round trips throughout the day from station 1 to station 2. For the scenario shown in , it can be determined that the cardholders’ place of residence is near station 1 and their workplace is near station 2.
It is assumed that the identified commuters live and work near the rail transit stations, and therefore that jobs – housing ratio can reflect the jobs – housing balance of the areas around rail transit stations. To reflect jobs – housing balance objectively, we choose one of the jobs – housing ratios, namely the zero-one index of Song et al. (Citation2019).
where JRNI is a normalized jobs – housing ratio into [−1, 1]. J represents the number of jobs at stations; and R represents the number of employed residents at stations. If the value of JRNI is close to 0, there is balance between jobs and housing; it is greater than 0, the area is oriented toward employment; and it is less than 0, the area is oriented toward housing.
4. Results
4.1. Values of land use indices
The maximum value of road density is 15.48, the minimum is 0.61, and the mean is 8.02 (). In the stations of Line 1, the mean value of road density is 8.10; for Line 2, the mean value is 9.46; for Line 3, the mean value is 7.60; and for Line 4, the mean value is 7.19.
Table 1. Statistics for the land use indices.
The maximum value of building density is 20.77, the minimum is 0.00, and the mean is 3.57 (). For Line 1, the mean value of building density is 4.30; for Line 2, the mean value is 4.10; for Line 3, the mean value is 2.83; and for Line 4, the mean value is 2.93.
Within the 500 m buffer zone of Yangchun Lake and Wuhan Railway stations, the area of “source” and “sink” landscapes are zero according to the data source. Then, the NLWLI of the two stations cannot be calculated. The mean values of the influence index of “source” landscape, influence index of “sink” landscape, and the NLWLI of the remaining 94 rail transit stations are 0.11, 0.03, and −0.48, respectively (). Based on the influence index, only Optics Valley Square station achieved land use balance, i.e. with a value of 0.00. Among the stations, there are three stations in the [−0.10, 0) interval and five stations in the (0, 0.10] interval, that is, close to balanced land use. A total of 78 stations have an influence index of less than 0.00 and 15 stations have an influence index greater than 0.00. For Line 1, the mean influence index of the “source” landscape is 0.11, the mean influence index of the “sink” landscape is 0.03 ± 0.03, and the mean NLWLI is −0.59. For Line 2, the mean influence index of the “source” landscape is 0.10, the mean of influence index of the “sink” landscape is 0.04, and the mean NLWLI is −0.33. For Line 3, the mean influence index of the “source” landscape is 0.10, the mean influence index of the “sink” landscape is 0.05 ± 0.03, and the mean NLWLI is −0.27. For Line 4, the mean influence index of the “source” landscape is 0.11, the mean influence index of the “sink” landscape is 0.03, and the mean NLWLI is −0.64.
According to values of coefficient of variation (), the dispersion degree of all the land use indices is high, but the degree of road density (Coefficient of variation is 39.78%) and the influence index of “source” landscape (Coefficient of variation is 45.45%) is relatively lower than other indices.
4.2. Commuting characteristics of stations
Using the above method, smart-card data was collected for a total of 232,950 commuters, accounting for 9.78% of cardholders. There are 134,802 commuters for the case in and 98,148 commuters for the case in . The mean commuting time of one-way trip was found to be 24 min. Additionally, it was determined that there is a significant difference in the number of residents and jobs at rail transit stations (, ). A total of seven stations had more than 5001 residents in , namely Optics Valley Square, Jiyuqiao, Jinyintan, Yangjiawan, Changgang Road, Xunlimen, and Huquan. Meanwhile, a total of 11 stations had more than 5001 jobs in , namely Chuhehan Street, Optics Valley Square, Zhongshan Park, Zhongnan Road, Jianghan Road, Jiedaokou, Xunlimen, Hongshan Square, Wangjiadun East, North Liji Road, and Jiyuqiao. These high-volume stations are mostly concentrated on Line 2, and Xunlimen, Zhongnan Road, and Hongshan Square are interchange stations.
Table 2. Numbers of stations according to residents and jobs around the station.
The results suggest that there are six travel flows with more than 1000 people, namely (1) Yangjiawan to Optics Valley Square, (2) Tieji Road to Chuhehan Street, (3) Jinyintan to Zhongshan Park, (4) Optics Valley Square to Jiedaokou, (5) Jiyuqiao to Jianghan Road, and (6) Jiyuqiao to Zhongshan Park (). Among them, five travel flows are on Line 2 and one is on Line 4. In addition, there are 22 travel flows of 501–1000 people, 53 flows of 300–500 people. Of the four metro lines, Line 2 has the highest commuting capacity, followed by Line 4 and Line 1, and the commuting capacity of Line 3 is the lowest. There are significant differences between the three towns of Hankou, Wuchang, and Hanyang. Commuters who use the large rail travel flow in Hankou live in the periphery of Hankou and are employed in the commercial centers of Zhongshan Park and Jianghan Road, and major of the commuters live in Wuchang. For Wuchang town, there is two-way travel flow along Line 2, and the jobs are mostly concentrated in the Jiedaokou, Optics Valley Square, and Chuhehan Street stations. The employment attraction of Hanyang is weakest and jobs there are concentrated around Wangjiawan station.
The maximum calculated value of JRNI for the stations is 0.87, the minimum is −0.77, and the mean is −0.10. Three stations achieved jobs – housing balance, i.e. a JRNI of 0.00, namely Gutian 1st Road, Huiji 2nd Road, and Citizens Home. A total of 58 stations have a JRNI of less than 0.00 and 35 have a JRNI of greater than 0.00. An additional eight stations are in the [−0.10, 0) interval and an additional 10 stations are in the (0, 0.10] interval, that is, close to jobs – housing balance. In the stations of Line 1, the mean value of JRNI is −0.10; for Line 2, the mean value is 0.04; for Line 3, the mean value is −0.04; and for Line 4, the mean value is −0.27.
4.3. Association between land use and jobs – housing distribution around stations
According to the NLWLI, the influence index of “source” landscape and “sink” landscape could be individually used to indicate the land use of employment and residence. By the correlation analysis shown in , road density and influence index of “source” landscape have a weak correlation with the number of residents. The influence index of the “sink” landscape is positively correlated with the number of jobs with correlation coefficient of 0.52, and building density and road density also have a certain correlation with the number of jobs (). For a pair of normalized indices of NLWLI and JRNI, the correlation coefficient is 0.57, which is significant at the level of 0.01 ().
Table 3. Pearson correlation coefficients between residents, jobs, and land use indices at rail transit stations.
5. Discussion
According to the results, the distribution of residents and jobs of rail commuting is correlated with building density. In , the number of residents or jobs at some stations is consistent with the fluctuation of building density. From the distribution of each line, values of Line 1 peak in middle stations and are lower at both sides. The trend of Line 1 is close to normal distribution, and the other three lines are disordered. Line 1 was opened at the earliest time in 2004 and is a mature rail line. Whether other lines will develop in normal distribution still needs to be continuously tested. Line 2 has the largest travel flow and multiple employment center, and it is the key line for development. The variation of the supporting road network around the stations is small, and there is a weak correlation with commuters (). The reasons maybe as follows: Firstly, although smart-card data was obtained for a relatively large number of passengers, it could not be used to obtain data on people using other modes of transportation (e.g. walking, private cars, buses, and bicycles) along the railway lines. Secondly, land use indices were calculated based on the 500 m service radius of the station, and it was also assumed that the commuters analyzed in this study lived and worked within 500 m of the stations. In cases where this assumption is not consistent, the correlation weakens. But this does not affect the general understanding that a more developed road network would help to the use of rail transit (Kamruzzaman et al. Citation2014).
Figure 7. Residents, jobs and building density of four lines (station names are in Appendix A).
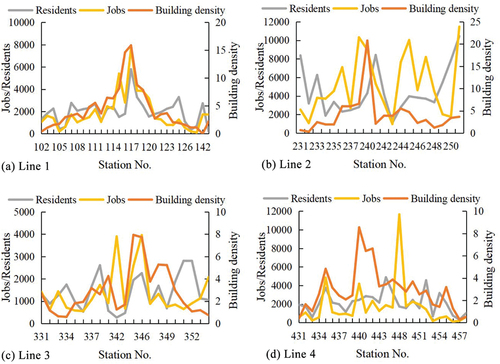
The land use around stations is dominated by residential land use, and the distribution of residential land use is relatively concentrated. Although the area around the railway lines is mainly used for residential functions, the jobs provided by “sink” landscape are more attractive for commuting by rail. At present, the average proportion of residential land within the 500 m service radius of the stations is 32.3%, the commercial land is 9.6%, and the public facilities land is 11.5%. These represent the common scale of a rail station with about 30% residential land, 10% commercial land, 10% public facilities land. For example, the proportion of residential land along Indian railway lines should be no less than 30%, and should include about 10% commercial land and 10% public facilities land (Ollivier, Kalra, and Ghate Citation2018). Land use planning for the area around rail transit stations is a long-term development framework. Observing the centralized distribution of travel flow, large traffic stations are characterized by commercial centers close to rail transit stations (), which is an important reason to promote employment. However, the formation of commercial center should also comply with objective laws. Generally, enhancing the efficiency of rail transit stations should be supported by the connected facilities, open space, building density, housing value, and urban design. But different development strategies should be adopted for different functions of rail stations. There are several stations (Sixin Avenue, Yunfei Road, Shuangdun, Taojialing, Dongfeng Motor Company) with a large scale of land use for employment but low commuting flow, reasons in addition to land use factor need to be further analyzed.
Table 4. Land use indices and JRNI of six vital stations.
In theory, NLWLI is greater than 0 and JRNI is greater than 0, or NLWLI is smaller than 0 and JRNI is smaller than 0, that means the jobs – housing orientation of rail stations is consistent. However, there are 32 stations of the NLWLI and the JRNI are opposite signs (). The area of commercial land use is small, but the employment attraction is great; within 500 m radius of the rail station, the large area of residential land use contributes to the negative value of NLWLI (). The contradiction between NLWLI and JRNI reminds two aspects: Firstly, whether it is feasible to adjust the land use strategy, such as reducing the residential land, and further increasing the land use for employment, to make the employment attraction of the station stronger. Secondly, the evaluation of rail station should not only rely on land use indices, but also combine with the real travel data to reflect the rail transit usage. Since travelers by rail are dynamical changing, and the non-commuters account for the majority by rail. Meanwhile, the index of land use is a constant. For the trend of three-dimensional development of the rail station, the evaluation of land use needs to be constantly developed from a two-dimensional plane into a three-dimensional space.
Although the jobs – housing balance is an ideal state, it was found difficult to achieve the balance of jobs – housing land use and commuters around the rail stations. The reason may be the station-level leads to the functional orientation prominently. Even on a larger scale along the rail lines, there is also a difference between residence and employment. Of the four lines, Line 2 is the closest to achieving both jobs – housing balance and land use balance, while Line 4 is dominated by residential function. At present, the balance of jobs – housing land use does not necessarily bring about the balance of jobs – housing ratio, and the balance of jobs – housing ratio does not necessarily bring about large commuting flow. We should not overemphasize the jobs – housing balance at the small scale of rail stations. Instead, according to the existing jobs and housing distribution, we should improve the coordinated land planning and facilities, and strengthen the potential employment attraction of rail stations. In the future, it is planned for Wuhan to have a total of about 13 railway lines, and as such the rail system will have a greater impact on the production and life of the city. Therefore, it is necessary to develop TOD planning systems to cope with rail travel flow and land use.
In addition to the difference between the job – housing distribution identified by the smart-card data and their actual distribution, there are still other limitations. First of all, there may be dynamic changes in commuters, and different groups would be identified in different time periods. Despite the large quantity of smart-card data used in the study to reflect the overall characters of rail commuting, it lacks comparative analysis with other periods due to the limited study period of smart-card data used. Secondly, without the personal information of commuters, the diversity of commuters’ socioeconomic characteristics has increased the difficulty of attribution. A previous study showed that secondary industry workers are more likely to achieve jobs – housing balance, while tertiary industry workers are more likely to commute long distances (Zhou, Yeh, and Yue Citation2018). Another study found that rich areas have less demand for public transport, while poor neighborhoods have more demand for public transport (Forouhar Citation2016). Therefore, if the sociodemographic characteristics of commuters could be obtained, such as gender, age, occupation, family details, and income, more useful information to assist in the development of rail transit could be extracted (Long, and Thill Citation2015). To promote the commuting use of Wuhan rail transit, our project will further investigate social characteristics of Wuhan’s population.
6. Conclusion
Land use and jobs – housing distribution around rail transit stations are important aspects of TOD city. To study the associations of land use with distribution of residents and jobs of rail commuters in Wuhan, China, the NLWLI index was constructed based on source – sink landscape theory together with road density and building density to evaluate the land use around the rail transit stations. Meanwhile, the character of rail commuting was identified with the aid of smart-card data. Through the associations between land use and commuters, we found that residential land was dominant around most rail transit stations, but the generation of commuting flow was mainly affected by building density and the land use of employment. At present, the land use balance around rail transit stations does not necessarily lead to jobs – housing balance and large travel flow, and some high-traffic stations have opposite dominant functions under the two indices. Although it is correlated between land use and commuters, the quantitative relation is relatively weak. When evaluating the efficiency of rail stations, both land use indices and real travel data should be used. The rail commuting time and distance were relatively short in Wuhan, and the employment center of Wuhan had been quite dispersed. In this regard, we advocate that balance of jobs – housing land use and jobs – housing ratio around rail stations should be used as inconclusive references at the scale of rail stations. Functional positioning of the rail transit stations should be based to further optimize the land use to promote the TOD of Wuhan. Since TOD in Chinese cities is still in an exploratory stage, this study provides a methodological reference for evaluating jobs – housing land use around rail transit stations. Meanwhile, a series of comprehensive designs are still needed to improve the traffic attraction of rail transit stations based on a deeper investigation.
Disclosure statement
No potential conflict of interest was reported by the author(s).
Data availability statement
The data that support the findings of this study are available from the Wuhan Land Use and Urban Spatial Planning Research Center, https://doi.org/10.1080/10095020.2022.2100286 with permission or from the corresponding author [email protected] upon reasonable request.
Additional information
Funding
Notes on contributors
Qingming Zhan
Qingming Zhan is a professor at Wuhan University. His research interests are urban spatial information theory and application of urban spatial information in urban planning and urban governance.
Yuqiu Jia
Yuqiu Jia completed her postdoctoral work at Wuhan Land Use and Urban Spatial Planning Research Center and is currently a lecture at Jilin Normal University. Her research interests are urban ecology and environmental effects of urban spatial form.
Zhenhua Zheng
Zhenhua Zheng is working at Wuhan Land Use and Urban Spatial Planning Research Center. He is committed to the practice of urban planning and management.
Qi Zhang
Qi Zhang received his PhD degree at Hong Kong Polytechnic University and is currently a postdoctoral fellow at Wuhan Land Use and Urban Spatial Planning Research Center. His research interests are urban governance and sustainability.
Lei Luo
Lei Luo completed her postdoctoral work at Wuhan Land Use and Urban Spatial Planning Research Center and is currently a lecture at Hubei University. Her research interests are urban infrastructure equalization and industrial geography.
References
- Ahmad, S., R. Avtar, M. Sethi, and A. Surjan. 2016. “Delhi’s Land Cover Change in Post Transit Era.” Cities 50: 111–118. doi:10.1016/j.cities.2015.09.003.
- Angel, S., and A. M. Blei. 2016. “The Spatial Structure of American Cities: The Great Majority of Workplaces are No Longer in Cbds, Employment Sub-Centers, or Live-Work Communities.” Cities 51: 21–35. doi:10.1016/j.cities.2015.11.031.
- Bhattacharjee, S., and A. R. Goetz. 2016. “The Rail Transit System and Land Use Change in the Denver Metro Region.” Journal of Transport Geography 54: 440–450. doi:10.1016/j.jtrangeo.2016.02.004.
- Bothe, K., H. K. Hansen, and L. Winther. 2018. “Spatial Restructuring and Uneven Intra-Urban Employment Growth in Metro-And Non-Metro-Served Areas in Copenhagen.” Journal of Transport Geography 70: 21–30. doi:10.1016/j.jtrangeo.2018.05.014.
- Brady, S. P., and J. L. Richardson. 2017. “Road Ecology: Shifting Gears Toward Evolutionary Perspectives.” Frontiers in Ecology and the Environment 15 (2): 91–98. doi:10.1002/fee.1458.
- Canepa, B. 2007. “Bursting the Bubble: Determining the Transit-Oriented Development’s Walkable Limits.” Transportation Research Record 1992 (1): 28–34. doi:10.3141/1992-04.
- Carlton, I. 2009. “Histories of Transit-Oriented Development: Perspectives on the Development of the TOD Concept.” Institute of Urban and Regional Development, University of California. Accessed September 16, 2019. https://www.econstor.eu/handle/10419/59412.
- Cervero, R., and G. B. Arrington. 2008. “Vehicle Trip Reduction Impacts of Transit-Oriented Housing.” Journal of Public Transportation 11 (3): 1. doi:10.5038/2375-0901.11.3.1.
- Cervero, R., and J. Murakami. 2009. “Rail and Property Development in Hong Kong: Experiences and Extensions.” Urban Studies 46 (10): 2019–2043. doi:10.1177/2F0042098009339431.
- Charron, M. 2007. “From Excess Commuting to Commuting Possibilities: More Extension to the Concept of Excess Commuting.” Environment & Planning A 39 (5): 1238–1254. doi:10.1068/2Fa3897.
- Chen, L., H. Tian, B. Fu, and X. Zhao. 2009. “Development of a New Index for Integrating Landscape Patterns with Ecological Processes at Watershed Scale.” China Geography Science 19 (1): 37–45. doi:10.1007/s11769-009-0037-9.
- Dadashpoor, H., and M. Alidadi. 2017. “Towards Decentralization: Spatial Changes of Employment and Population in Tehran Metropolitan Region, Iran.” Applied Geography 85: 51–61. doi:10.1016/j.apgeog.2017.05.004.
- Egu, O., and P. Bonnel. 2020. “Investigating Day-to-Day Variability of Transit Usage on a Multimonth Scale with Smart Card Data. A Case Study in Lyon.” Travel Behaviour and Society 19: 112–123. doi:10.1016/j.tbs.2019.12.003.
- Engelfriet, L., and E. Koomen. 2018. “The Impact of Urban Form on Commuting in Large Chinese Cities.” Transportation 45 (5): 1269–1295. doi:10.1007/s11116-017-9762-6.
- Ewing, R., G. Tian, T. Lyons, and K. Terzano. 2017. “Trip and Parking Generation at Transit-Oriented Developments: Five US Case Studies.” Landscape and Urban Planning 160: 69–78. doi:10.1016/j.landurbplan.2016.12.002.
- Feng, J., X. Li, B. Mao, Q. Xu, and Y. Bai. 2017. “Weighted Complex Network Analysis of the Beijing Subway System: Train and Passenger Flows.” Physica A 474: 213–223. doi:10.1016/j.physa.2017.01.085.
- Forouhar, A. 2016. “Estimating the Impact of Metro Rail Stations on Residential Property Values: Evidence from Tehran.” Public Transport 8 (3): 427–451. doi:10.1007/s12469-016-0144-9.
- Gately, C. K., L. R. Hutyra, S. Peterson, and I. S. Wing. 2017. “Urban Emissions Hotspots: Quantifying Vehicle Congestion and Air Pollution Using Mobile Phone GPS Data.” Environmental Pollution 229: 496–504. doi:10.1016/j.envpol.2017.05.091.
- Gong, Y., Y. Lin, and Z. Duan. 2017. “Exploring the Spatiotemporal Structure of Dynamic Urban Space Using Metro Smart-Card Records.” Computers, Environment and Urban Systems 64: 169–183. doi:10.1016/j.compenvurbsys.2017.02.003.
- Guerra, E., C. Caudillo, P. Monkkonen, and J. Montejano. 2018. “Urban Form, Transit Supply, and Travel Behavior in Latin America: Evidence from Mexico’s 100 Largest Urban Areas.” Transport Policy 69: 98–105. doi:10.1016/j.tranpol.2018.06.001.
- Han, H., C. Yang, E. Wang, J. Song, and M. Zhang. 2015. “Evolution of Jobs-Housing Spatial Relationship in Beijing Metropolitan Area: A Job Accessibility Perspective.” Chinese Geographical Science 25 (3): 375–388. doi:10.1007/s11769-015-0738-1.
- Higgins, C. D., and P. S. Kanaroglou. 2016. “A Latent Class Method for Classifying and Evaluating the Performance of Station Area Transit-Oriented Development in the Toronto Region.” Journal of Transport Geography 52: 61–72. doi:10.1016/j.jtrangeo.2016.02.012.
- Huang, D., Z. Liu, X. Zhao, and P. Zhao. 2017. “Emerging Polycentric Megacity in China: An Examination of Employment Subcenters and Their Influence on Population Distribution in Beijing.” Cities 69: 36–45. doi:10.1016/j.cities.2017.05.013.
- Huang, X., X. Cao, J. Yin, and X. Cao. 2019. “Can Metro Transit Reduce Driving? Evidence from Xi’An, China.” Transport Policy 81: 350–359. doi:10.1016/j.tranpol.2018.03.006.
- Jiao, L., Z. Li, G. Xu, B. Zhang, T. Dong, and Y. Gu. 2017. “The Characteristics and Patterns of Spatially Aggregated Elements in Urban Areas of Wuhan.” Acta Geographica Sinica 72 (8): 1432–1443. doi:10.11821/dlxb201708008.
- Kamruzzaman, M., D. Baker, S. Washington, and G. Turrell. 2014. “Advance Transit Oriented Development Typology: Case Study in Brisbane, Australia.” Journal of Transport Geography 34: 54–70. doi:10.1016/j.jtrangeo.2013.11.002.
- Kim, K. Y., C. Y. Lim, and E. J. Kim. 2018. “A New Approach to the Space-Time Analysis of Big Data: Application to Subway Traffic Data in Seoul.” Journal of Big Data 5 (1): 5. doi:10.1186/s40537-018-0116-9.
- Lavery, T., and P. Kanaroglou. 2012. “Rediscovering Light Rail: Assessing the Potential Impacts of a Light Rail Transit Line on Transit Oriented Development and Transit Ridership.” Transportation Letters 4 (4): 211–226. doi:10.3328/TL.2012.04.04.211-226.
- Lin, J. J., and C. C. Gau. 2006. “A TOD Planning Model to Review the Regulation of Allowable Development Densities Around Subway Stations.” Land Use Policy 23 (3): 353–360. doi:10.1016/j.landusepol.2004.11.003.
- Lin, D., A. Allan, and J. Cui. 2016. “The Influence of Jobs–housing Balance and Socio-Economic Characteristics on Commuting in a Polycentric City: New Evidence from China.” Environment and Urbanization ASIA 7 (2): 157–176. doi:10.1177/2F0975425316654802.
- Liu, Y., F. Wang, Y. Xiao, and S. Gao. 2012. “Urban Land Uses and Traffic ‘Source-Sink Areas’: Evidence from GPS-Enabled Taxi Data in Shanghai.” Landscape and Urban Planning 106 (1): 73–87. doi:10.1016/j.landurbplan.2012.02.012.
- Long, Y., and J. C. Thill. 2015. “Combining Smart-Card Data and Household Travel Survey to Analyze Jobs–housing Relationships in Beijing.” Computers, Environment and Urban Systems 53: 19–35. doi:10.1016/j.compenvurbsys.2015.02.005.
- Loo, B. P., C. Chen, and E. T. Chan. 2010. “Rail-Based Transit-Oriented Development: Lessons from New York City and Hong Kong.” Landscape and Urban Planning 97 (3): 202–212. doi:10.1016/j.landurbplan.2010.06.002.
- Lu, S., X. Guan, C. He, and J. Zhang. 2014. “Spatio-Temporal Patterns and Policy Implications of Urban Land Expansion in Metropolitan Areas: A Case Study of Wuhan Urban Agglomeration, Central China.” Sustainability 6 (8): 4723–4748. doi:10.3390/su6084723.
- Lyu, G., L. Bertolini, and K. Pfeffer. 2016. “Developing a TOD Typology for Beijing Metro Station Areas.” Journal of Transport Geography 55: 40–50. doi:10.1016/j.jtrangeo.2016.07.002.
- Ma, X., C. Liu, H. Wen, Y. Wang, and Y. J. Wu. 2017. “Understanding Commuting Patterns Using Transit Smart-Card Data.” Journal of Transport Geography 58: 135–145. doi:10.1016/j.jtrangeo.2016.12.001.
- Ma, X., X. Chen, X. Li, C. Ding, and Y. Wang. 2018. “Sustainable Station-Level Planning: An Integrated Transport and Land Use Design Model for Transit-Oriented Development.” Journal of Cleaner Production 170: 1052–1063. doi:10.1016/j.jclepro.2017.09.182.
- Marcińczak, S., and B. Bartosiewicz. 2018. “Commuting Patterns and Urban Form: Evidence from Poland.” Journal of Transport Geography 70: 31–39. doi:10.1016/j.jtrangeo.2018.05.019.
- Ministry of Housing and Urban-Rural Development of the People’s Republic of China. 2019. China Urban Construction Statistical Yearbook. Beijing: China Statistical Press.
- Ministry of Transport of the People’s Republic of China. 2019. China Tansport Statistical Yearbook. Beijing: China Communication Press Co., Ltd.
- Moghadam, A. S., A. Soltani, B. Parolin, and M. Alidadi. 2018. “Analysing the Space-Time Dynamics of Urban Structure Change Using Employment Density and Distribution Data.” Cities 81: 203–213. doi:10.1016/j.cities.2018.04.009.
- Mouratidis, K., D. Ettema, and P. Næss. 2019. “Urban Form, Travel Behavior, and Travel Satisfaction.” Transportation Research Part A: Policy and Practice 129: 306–320. doi:10.1016/j.tra.2019.09.002.
- Mustafa, A., A. V. Rompaey, M. Cools, I. Saadi, and J. Teller. 2018. “Addressing the Determinants of Built-Up Expansion and Densification Processes at the Regional Scale.” Urban Studies 55 (15): 3279–3298. doi:10.1177/2F0042098017749176.
- Nasri, A., and L. Zhang. 2014. “The Analysis of Transit-Oriented Development (TOD) in Washington, DC and Baltimore Metropolitan Areas.” Transport Policy 32: 172–179. doi:10.1016/j.tranpol.2013.12.009.
- National Bureau of Statistics of China. 2017. China Statistical Yearbook. Accessed September 16, 2019. http://www.stats.gov.cn/tjsj/ndsj/.
- Nigro, A., L. Bertolini, and F. D. Moccia. 2019. “Land Use and Public Transport Integration in Small Cities and Towns: Assessment Methodology and Application.” Journal of Transport Geography 74: 110–124. doi:10.1016/j.jtrangeo.2018.11.004.
- Ollivier, G. P., B. Kalra, and A. Ghate. 2018. “TOD Implementation Resources and Tools (English).” Global Platform for Sustainable Cities, World Bank. Accessed September 16, 2019. http://documents.worldbank.org/curated/en/261041545071842767/TOD-Implementation-Resources-and-Tools.
- Papa, E., and L. Bertolini. 2015. “Accessibility and Transit-Oriented Development in European Metropolitan Areas.” Journal of Transport Geography 47: 70–83. doi:10.1016/j.jtrangeo.2015.07.003.
- Park, G. H., and S. M. Kwon. 2018. “The Ameliorated Trade-Off Between Commuting Efficiency and Jobs–housing Balance in a Green-Belted City: Lessons from Seoul.” Socio-Economic Planning Sciences 63: 47–59. doi:10.1016/j.seps.2017.06.005.
- Renne, J. L., T. Tolford, S. Hamidi, and R. Ewing. 2016. “The Cost and Affordability Paradox of Transit-Oriented Development: A Comparison of Housing and Transportation Costs Across Transit-Oriented Development, Hybrid and Transit-Adjacent Development Station Typologies.” Housing Policy Debate 26 (4–5): 819–834. doi:10.1080/10511482.2016.1193038.
- Sahu, A. 2018. “A Methodology to Modify Land Uses in a Transit Oriented Development Scenario.” Journal of Environmental Management 213: 467–477. doi:10.1016/j.jenvman.2017.12.004.
- Sari Aslam, N., T. Cheng, and J. Cheshire. 2019. “A High-Precision Heuristic Model to Detect Home and Work Locations from Smart Card Data.” Geo-Spatial Information Science 22 (1): 1–11. doi:10.1080/10095020.2018.1545884.
- Sari Aslam, N., M. R. Ibrahim, T. Cheng, H. Chen, and Y. Zhang. 2021. “ActivityNet: Neural Networks to Predict Public Transport Trip Purposes from Individual Smart Card Data and Pois.” Geo-Spatial Information Science 24 (4): 711–721. doi:10.1080/10095020.2021.1985943.
- Shen, P., L. Ouyang, C. Wang, Y. Shi, and Y. Su. 2020. “Cluster and Characteristic Analysis of Shanghai Metro Stations Based on Metro Card and Land-Use Data.” Geo-Spatial Information Science 23 (4): 352–361. doi:10.1080/10095020.2020.1846463.
- Song, X., Y. Wang, Y. Yang, K. Zhang, and X. Niu. 2019. “Statistical Verification of Home-Work Separation Based on Commuting Distance.” Journal of Geo-Information Science 21 (11): 1699–1709. doi:10.12082/dqxxkx.2019.180606.
- Staricco, L., and E. V. Brovarone. 2018. “Promoting TOD Through Regional Planning: A Comparative Analysis of Two European Approaches.” Journal of Transport Geography 66: 45–52. doi:10.1016/j.jtrangeo.2017.11.011.
- Sung, H., and J. T. Oh. 2011. “Transit-Oriented Development in a High-Density City: Identifying Its Association with Transit Ridership in Seoul, Korea.” Cities 28 (1): 70–82. doi:10.1016/j.cities.2010.09.004.
- Tasic, I., X. Zhou, and M. Zlatkovic. 2014. “Use of Spatiotemporal Constraints to Quantify Transit Accessibility: Case Study of Potential Transit-Oriented Development in West Valley City, Utah.” Transportation Research Record 2417 (1): 130–138. doi:10.3141/2F2417-14.
- Tu, W., R. Cao, Y. Yue, B. Zhou, Q. Li, and Q. Li. 2018. “Spatial Variations in Urban Public Ridership Derived from GPS Trajectories and Smart-Card Data.” Journal of Transport Geography 69: 45–57. doi:10.1016/j.jtrangeo.2018.04.013.
- Vale, D. S. 2015. “Transit-Oriented Development, Integration of Land Use and Transport, and Pedestrian Accessibility: Combining Node-Place Model with Pedestrian Shed Ratio to Evaluate and Classify Station Areas in Lisbon.” Journal of Transport Geography 45: 70–80. doi:10.1016/j.jtrangeo.2015.04.009.
- Vale, D. S., C. M. Viana, and M. Pereira. 2018. “The Extended Node-Place Model at the Local Scale: Evaluating the Integration of Land Use and Transport for Lisbon’s Subway Network.” Journal of Transport Geography 69: 282–293. doi:10.1016/j.jtrangeo.2018.05.004.
- Wu, Q., M. Zhang, and D. Yang. 2015. Recent Developments in Chinese Urban Planning: Jobs–housing Balance: The Right Ratio for the Right Place. Cham: Springer.
- Wu, T., L. Tang, H. Chen, Z. Wang, and Q. Qiu. 2017. “Application of Source–sink Landscape Influence Values to Commuter Traffic: A Case Study of Xiamen Island.” Sustainability 9 (12): 2366. doi:10.3390/su9122366.
- Yan, L., D. Wang, S. Zhang, and D. Xie. 2018. “Evaluating the Multi-Scale Patterns of Jobs-Residence Balance and Commuting Time-Cost Using Cellular Signaling Data: A Case Study in Shanghai.” Transportation 46 (3): 777–792. doi:10.1007/s11116-018-9894-3.
- Zamir, K. R., A. Nasri, B. Baghaei, S. Mahapatra, and L. Zhang. 2014. “Effects of Transit-Oriented Development on Trip Generation, Distribution, and Mode Share in Washington, DC, and Baltimore, Maryland.” Transportation Research Record 2413 (1): 45–53. doi:10.3141/2F2413-05.
- Zhang, P., J. Zhou, and T. Zhang. 2017. “Quantifying and Visualizing Jobs–housing Balance with Big Data: A Case Study of Shanghai.” Cities 66: 10–22. doi:10.1016/j.cities.2017.03.004.
- Zhang, T., W. Zhang, and Z. He. 2021. “Measuring Positive Public Transit Accessibility Using Big Transit Data.” Geo-Spatial Information Science 24 (4): 722–741. doi:10.1080/10095020.2021.1993754.
- Zhao, P., and S. Li. 2017. “Bicycle-Metro Integration in a Growing City: The Determinants of Cycling as a Transfer Mode in Metro Station Areas in Beijing.” Transportation Research Part A: Policy and Practice 99: 46–60. doi:10.1016/j.tra.2017.03.003.
- Zhao, P., H. Yang, L. Kong, Y. Liu, and D. Liu. 2018. “Disintegration of Metro and Land Development in Transition China: A Dynamic Analysis in Beijing.” Transportation Research Part A: Policy and Practice 116: 290–307. doi:10.1016/j.tra.2018.06.017.
- Zhou, X., X. Chen, and T. Zhang. 2016. “Impact of Megacity Jobs–housing Spatial Mismatch on Commuting Behaviors: A Case Study on Central Districts of Shanghai, China.” Sustainability 8 (2): 122. doi:10.1016/j.tra.2018.06.017.
- Zhou, X., A. G. O. Yeh, and Y. Yue. 2018. “Spatial Variation of Self-Containment and Jobs–housing Balance in Shenzhen Using Cellphone Big Data.” Journal of Transport Geography 68: 102–108. doi:10.1016/j.jtrangeo.2017.12.006.
- Zuo, T., H. Wei, and A. Rohne. 2018. “Determining Transit Service Coverage by Non-Motorized Accessibility to Transit: Case Study of Applying GPS Data in Cincinnati Metropolitan Area.” Journal of Transport Geography 67: 1–11. doi:10.1016/j.jtrangeo.2017.12.006.