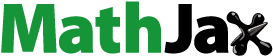
ABSTRACT
Recent decades have witnessed several infectious disease outbreaks, including the coronavirus disease (COVID-19) pandemic, which had catastrophic impacts on societies around the globe. At the same time, the twenty-first century has experienced an unprecedented era of technological development and demographic changes: exploding population growth, increased airline flights, and increased rural-to-urban migration, with an estimated 281 million international migrants worldwide in 2020, despite COVID-19 movement restrictions. In this review, we synthesized 195 research articles that examined the association between human movement and infectious disease outbreaks to understand the extent to which human mobility has increased the risk of infectious disease outbreaks. This article covers eight infectious diseases, ranging from respiratory illnesses to sexually transmitted and vector-borne diseases. The review revealed a strong association between human mobility and infectious disease spread, particularly strong for respiratory illnesses like COVID-19 and Influenza. Despite significant research into the relationship between infectious diseases and human mobility, four knowledge gaps were identified based on reviewed literature in this study: 1) although some studies have used big data in investigating infectious diseases, the efforts are limited (with the exception of COVID-19 disease), 2) while some research has explored the use of multiple data sources, there has been limited focus on fully integrating these data into comprehensive analyses, 3) limited research on the global impact of mobility on the spread of infectious disease with most studies focusing on local or regional outbreaks, and 4) lack of standardization in the methodology for measuring the impacts of human mobility on infectious disease spread. By tackling the recognized knowledge gaps and adopting holistic, interdisciplinary methods, forthcoming research has the potential to substantially enhance our comprehension of the intricate interplay between human mobility and infectious diseases.
1. Introduction
Human movement, as a critical component of human contact and connectivity, is one of key drivers of the infectious diseases transmissions, particularly during a pandemic. Human mobility is widely defined as individuals’ or groups’ movement in space and time, including displacement, rural to urban, household fluidity, domestic and international migrations, and involuntary mobility like human trafficking for sexual exploitation, migration associated with armed conflicts, and forced labor. With the increase of individual and collective human movement due to globalization and massive transportation networks, the spread of infectious diseases has also grown on an unprecedented scale, consequently leading to an epidemic or a pandemic. Humans have witnessed several pandemics throughout history. For example, Black Death in the 14th Century is believed to have triggered quarantine in Europe. Despite the undeveloped state of transportation systems, mobility was considered a key element in the widespread transmission of the Black Death (Duncan and Scott Citation2005). The 1918 Spanish influenza infected hundreds of millions and led to the death of approximately fifty million people (Johnson and Juergen Citation2002); the 1968 flu pandemic, also known as Hong Kong flu that originated in China and lasted till 1970, is estimated to have caused between 0.5 to 2 million deaths worldwide (Saunders-Hastings and Krewski Citation2016). The 2009 HIN1 outbreak emerged in Mexico, and approximately simultaneous outbreaks started in Southern United States (Saunders-Hastings and Krewski Citation2016). In December 2019, a pneumonia was reported in Wuhan, China (Zhu et al. Citation2020), caused by a new type of coronavirus named SARS-CoV-2. To date, over 663 million confirmed cases and almost 6.72 million deaths have been reported worldwide. During these pandemics, various responses targeted restricting the spread of the virus. In the COVID-19 crisis, human mobility has been regarded as one of the major drivers of outbreaks worldwide. To curb human movement, a large number of countries instituted full or partial lockdown ranging from days to months, including domestic travel bans, international travel restriction, border closure, stay-at-home orders, prohibited public gatherings, as well as implementing closures of educational institutions, public transportation systems, and workplaces (Barbieri et al. Citation2021; Kharel et al. Citation2022).
Throughout various stages of pandemics, researchers have devoted significant resources to explore the relationship between infectious diseases and human mobility, seeking to uncover the patterns that link movement and virus spread. They have utilized an extensive array of simulation, mathematical, and prediction models on a diverse set of datasets. The use of human movement models to predict the spread of viruses has demonstrated that human mobility plays a crucial role in the transmission of infectious diseases, particularly during COVID-19 (Lee et al. Citation2023; Perrotta et al. Citation2022). Analogously, numerous models have been proposed for the transmission of Human Immunodeficiency Virus (HIV) (Saravanakumar et al. Citation2020), Ebola virus (Li Citation2017), and vector-borne diseases such as malaria persistence (Gomes et al. Citation2020), dengue cases (Heidrich et al. Citation2021) and Zika virus (Perrotta et al. Citation2022) among others. These studies consistently show that human movement plays a critical role in the transmission of infectious diseases. However, it is crucial to specify that mobility restrictions have shown to be effective primarily in the early stages of an epidemic when there is no available effective vaccine or medication. For instance, movement restrictions have been successful in slowing down transmission rates, as observed during the COVID-19 pandemic, they have not been sufficient in halting the spread entirely. On the other hand, such measures come with considerable economic, social, and psychological costs, particularly affecting marginalized communities (Bonal and Gonzalez Citation2020; Panda et al. Citation2021; Roques et al. Citation2020).
In this review, the authors comprehensively examined literature from the past two decades that focuses on the interplay between human mobility and the spread of infectious diseases. Specifically, our investigation is structured along four key dimensions to provide a coherent analysis of existing research. First, we investigated the types of data sources predominantly employed in these studies. We evaluated the extent to which various data types, such as medical records, travel statistics, or social media feeds, have been harnessed to explore the relationship between human movement and disease transmission. Second, we turned our attention to methodological approaches, highlighting the range of techniques used in the literature. This includes an in-depth look at mathematical models, regression analyses, and predictive algorithms, among other methods, to assess their effectiveness and limitations in capturing the complexities of disease spread related to human mobility. Third, we examined the scalability of the studies, categorizing them based on their geographical focus. This segmentation allows us to assess the breadth and limitations of existing research, particularly in terms of its applicability to broader contexts. Lastly, we focused on the degree of methodological standardization across studies. We assessed whether uniform approaches are used, and if not, how these variances impact the reliability and comparability of research findings. Based on these results, we synthesized and discussed the association between human mobility and infectious diseases. We then identified key areas for improvement in the field of public health and infectious diseases, including underutilized data sources and methodological diversity. By offering a roadmap for scalable research and advocating for methodological standardization, we provide actionable insights that build upon and refine earlier studies, ultimately aiming to enhance public health interventions and open new avenue of research for future studies.
To achieve these objectives, we searched relevant literature by querying human mobility and the chosen infectious diseases in two databases (Web of Science & PubMed). Web of Science is a comprehensive, multidisciplinary database that covers numerous subject areas and provides access to a vast array of high-quality, peer-reviewed articles, with PubMed specializing in life sciences and biomedical literature, ensuring a comprehensive and in-depth review of health research topics (Kulkarni et al. Citation2009; Sayers et al. Citation2023).
2. Methodology
2.1. Search strategy and eligibility criteria
The authors, experts in the fields of geography and public health, meticulously devised a search protocol. Furthermore, the review article adheres to the guidelines prescribed by the Preferred Reporting Items for Systematic Reviews and Meta-Analyses (PRISMA) (Moher et al. Citation2009). To identify relevant articles, two databases were systematically reviewed: Web of Science (WOS) Core Collection and PubMed. Both WOS and PubMed are reputable databases with a high degree of credibility and reliability, providing access to a wide range of peer-reviewed journals. These databases are thoroughly curated, and their content is typically more rigorous and reliable than other sources. WOS is a paid-access platform that enables researchers to access multiple databases in various academic disciplines from more than 1.9 billion cited references, mainly focusing on medicine and natural science (Ellegaard and Wallin Citation2015). PubMed, however, is an unpaid search engine allowing primarily over 35 million citations in the field of biomedical literature (Ossom Williamson and Minter Citation2019). Following the research protocol, we designed, we successfully retrieved a substantial number of papers from the two databases, which adequately support this review article.
The search terms used for downloading articles included “human mobility”, “infectious diseases”, and their synonyms. The keywords for “human mobility” and “infectious diseases” were collected based on previous studies (Jing et al. Citation2023) because no official lists exist for these two terms that we could refer to. Furthermore, Boolean operators, hyphens, and truncations were utilized to make the search as comprehensive as possible. To further broaden the coverage of the review, the references of reviewed articles were searched for additional literature. Ultimately, sources that clearly discussed the association between human mobility and infectious diseases were retained.
Articles were chosen when they were (1) peer-reviewed original research, (2) published in English journals, (3) quantitatively assessed the association between human mobility and selected infectious diseases, and (4) published between January 2000 and December 2022, as the twenty-first century has been characterized by rapid technological and demographic changes, increased global connectivity, and urbanization, which coincide with a surge in infectious disease outbreaks. These articles reported observational studies, including cohort, cross-sectional, and models for prediction, to examine the association between human mobility and infectious diseases. Articles were excluded when they (1) were literature reviews, (2) conference proceedings, and (3) related to vaccination.
2.2. Screening and information extraction
The initial search obtained 2458 articles from the selected databases; of these results, 2129 articles were selected after eliminating duplicates. Since the initial search could include irrelevant articles based on the appearance of search terms in titles, abstracts, or content, a combination of unsupervised machine learning and manual screening was applied to identify relevant articles from the initial search pool.
First, the Lbl2Vec algorithm, an unsupervised document classification and retrieval method, was used to select literature that appeared relevant to the topic of interest based on titles and abstracts (Schopf, Daniel, and Florian Citation2022). The algorithm automatically produces jointly embedded labels, word vectors, and documents and then returns categories of documents based on predefined keywords. Once the representation of input documents and words is generated in a shared vector-space, the algorithm learns label vectors from predefined keywords, representing varieties of categories. Finally, the algorithm predicts the connection of documents to each category based on the similarity of documents vector with generated label vectors. For calculating the centroid of embedding, the below equation is used, where
represents the number of words.
Two hundred sixty-two articles were obtained after implementing the algorithm, and of these results, 192 distinctive papers were finalized after the manual screening. In addition, to ensure that the algorithm detected relevant articles, the authors randomly selected 500 papers with a higher score of similarity from unselected publications. After reading their titles and abstracts, only three of them was deemed relevant and included in the review. Next, full-text versions were obtained for those articles that were recognized to be relevant. Following this, the articles were summarized, and information was extracted pertaining to the study purpose, data types, methodological approaches, country, and main findings. In this review, the focus was solely on the relationship between human mobility and the selected infectious diseases and the relevant outcomes, even though some studies may have explored additional relationships. illustrates the article identification and selection process.
Figure 1. The flow diagram illustrates the process of article identification, screening, and selection. While the diagram shows the selection of 192 articles, three additional papers was manually included after examining 500 random articles from the unselected pool via unsupervised machine learning, bringing the total to 195.
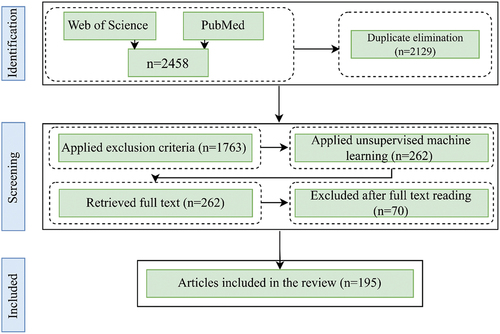
3. Result
3.1. Overview
The number of articles explicitly discussed the association between human mobility and infectious diseases varied based on the type of disease. Some infectious diseases, for example, have gained more attention compared to others. Since there are more than 27 infectious diseases based on (Jing et al. Citation2023), discussing human mobility and all of these diseases is beyond the scope of this paper. Consequently, this review paper only includes infectious diseases for which we were able to retrieve more than 10 relevant articles from WoS and PubMed databases using our search terms. These articles must have clearly discussed the connection between human mobility and the transmission of infectious diseases. The final included diseases are vector-borne illnesses (Zika virus, dengue and malaria), Ebola, HIV, influenza, hepatitis, and COVID-19, as presented in .
Figure 2. A total of 195 articles were selected for review: the distribution of these articles by disease type is presented in (a); (b) visualizes the percentage of selected studies by region, providing insights into the geographic focus of research on infectious diseases in this review.
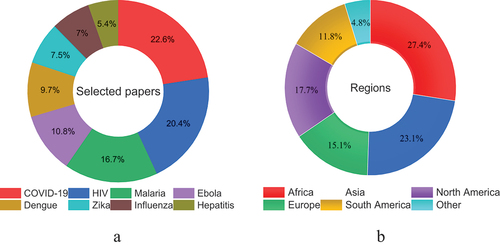
Before delving into the discussion regarding the chosen articles for review, we illustrate the transmission of infectious diseases via human mobility in . The figure illustrates the spread of selected infectious disease outbreaks (Cox Citation2010; De Cock, Jaffe, and Curran Citation2012; Patel et al. Citation2010), although it is important to note that it does not necessarily indicate the origin of the virus as there may be some controversy regarding the starting location of certain diseases due to the lack of solid evidence.
Figure 3. Illustrates the outbreak of each disease and its spread via human movement to other places. Seven types of infectious diseases are presented in this figure, and there has not been any outbreak of hepatitis in recent decades (Cox Citation2010; De Cock, Jaffe, and Curran Citation2012; Patel et al. Citation2010).
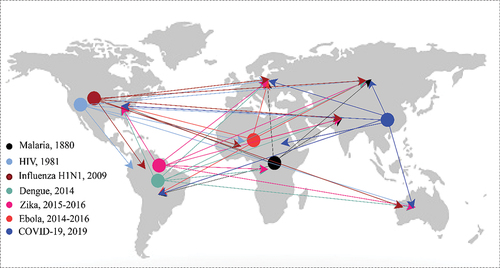
3.2. Vector-borne diseases
Vector-borne diseases (VBD) caused by parasites, bacteria, and viruses are transmitted to humans by vectors (). Each year, there are over 700,000 deaths from vector-borne diseases, such as malaria, dengue, Zika, yellow fever, and other VBDs. The burden of such diseases is disproportionately high in tropical and subtropical regions and excessively affects the poorest people. In recent years, major outbreaks of Zika, dengue, and malaria have afflicted populations in various regions of the world and claimed many lives. The virus is transmitted by the bite of infected mosquitoes to humans, and then humans spread the disease via movement into new areas (Wilson et al. Citation2020). In this category of viruses, Zika, dengue, and malaria have garnered significant attention from researchers, while other vector-borne diseases (VBDs) have received comparatively less focus. Subsequently, few studies have explored the association between the spread of such viruses and human mobility. A summary of the articles chosen for this study, focusing on Zika, dengue, and malaria diseases, is provided in . This disparity is evident in our literature search, as we were able to retrieve more than 10 relevant articles solely for Zika, dengue, and malaria based on the defined search terms.
Figure 4. The life cycle of malaria, Zika, and dengue viruses is depicted for enhanced comprehension.
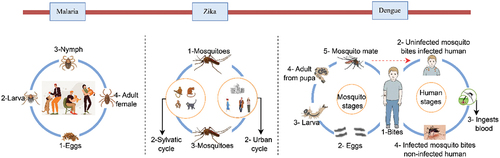
Table 1. Summary of studies related to human mobility and vector-borne diseases.
3.2.1. Zika virus
Zika virus is a mosquito-borne disease that was first isolated in 1947 from the Zika forest in Uganda (Dick, Stuart F, and Alexander Citation1952). Since its inception, several outbreaks have been recorded in various parts of the world. The primary mode of transmission for this disease is through the bite of Aedes spp. mosquitoes. However, the virus can also be transmitted via sexual practices, blood transfusions, and from mother to fetus during pregnancy. Of particular concern, infants whose mothers contract the virus during pregnancy may be born with microcephaly, a serious condition that affects brain development.
Over decades of studies, many precautionary measures are regarded to be effective in controlling the Zika virus, including the human mobility factor (Wesolowski et al. Citation2016); moving within and between regions with Zika virus increases the probability of carrying the virus between places by the infected individuals. The Zika virus outbreak between 2015 and 2016 in America prompted the World Health Organization to announce an emergency situation. Numerous studies have explored the impact of human mobility on the transmission of the Zika virus, considering a variety of factors. For example, some studies aimed to identify which demographic groups are more likely to travel to affected areas (Teich et al. Citation2018) and how the virus has influenced travelers’ plans (Widmar et al. Citation2017). Other studies have assessed public awareness of the Zika virus, especially among pregnant women during their travels (Guo et al. Citation2017; Squiers et al. Citation2018), as well as the perceptions of pregnant women in Zika-affected regions regarding travel and mobility (Chandrasekaran et al. Citation2017). Further studies have investigated the importation and exportation of the Zika virus across different regions, such as its importation into Australia by travelers (Pyke et al. Citation2014; Quam and Wilder-Smith Citation2016) and imported cases identified at a travel center in Paris (Sokal et al. Citation2016). One study focused on strategies to minimize the risk of Zika virus outbreaks caused by international travelers (Angelo et al. Citation2020). Infectious disease prediction based on human mobility is another important area of research aimed at curbing virus outbreaks. For instance, stochastic models utilizing human mobility data were employed to forecast the 2015–2016 Zika virus outbreak in Colombia (Perrotta et al. Citation2022), emphasizing the significance of human movement in disease outbreaks. Reconstructing past Zika outbreaks and projecting future spread using mobility and demographic data can help public health officials prepare for and prevent future outbreaks (Zhang et al. Citation2017). The reviewed studies largely applied regression models, mathematical models, and stochastic approaches to primarily survey data and occasionally other sources like mobile phone and air travel data, with most research conducted in the Americas.
3.2.2. Dengue virus
Dengue virus (DENV) is another viral infectious disease transmitted to humans through female mosquito bites, and it has rapidly spread in most regions, largely in tropical and subtropical areas. At present, the geographical distribution of DENV includes approximately 141 countries. One study indicated that more than 390 million infections cause by DENV per year, and Asian countries carry over 70% of the actual burden (Bhatt et al. Citation2013), and nearly 3.9 billion individuals are at risk of infection with DENV globally (Brady et al. Citation2012). The virus is transmitted to mosquitoes from humans who are viremic with DENV, and vice versa (Duong et al. Citation2015).
Numerous studies have revealed that human mobility can play an integral role in the dengue virus spark by characterizing people’s potential contact with the infected sources. Human movement noticeably expands the spatial scale of spreading and causes contact to happen throughout an infected individual’s activity space (Schaber et al. Citation2021). The dengue pathogen is transmitted by invasive mosquitoes associated with the Aedes in various regions. Several studies have investigated the role of environmental factors in the transmission of the dengue virus (DENV). Among these, several found that human mobility, as tracked by mobile phone data, is a critical driver in the spatial spread of the virus (Kiang et al. Citation2021; Wesolowski et al. Citation2015). Other research highlighted the importance of understanding mobility patterns and seasonality for predicting dengue outbreaks at the neighborhood level (Bomfim et al. Citation2020; Heidrich et al. Citation2021). The influence of human movement on DENV transmission varies depending on the scale of endemic regions, making this knowledge essential for implementing effective control measures (Falcon-Lezama et al. Citation2017). Additionally, factors such as symptom severity and the scale of infection can impact daily human mobility (Schaber et al. Citation2019). The extent of human movement and the regions traversed also play crucial roles in the severity of dengue outbreaks (Tsuzuki et al. Citation2014). Using stochastic models, some studies have demonstrated that human movement may indeed be the primary driving force behind the dynamics of diseases like DENV (Barmak, Dorso, and Otero Citation2016; Zhu et al. Citation2019). Social media data has also proven to be a valuable source in studying the spread of the dengue virus. For example, researchers have used this data to predict the intra-urban spread of dengue (Ramadona et al. Citation2019) and analyze spatiotemporal patterns based on human mobility extracted from geolocated tweets (Castro et al. Citation2021; Kraemer et al. Citation2018). In fact, understanding the factors that contribute to transmission is crucial for containing the virus. This includes further investigation into the influence of human mobility patterns on dengue virus outbreaks.
3.2.3. Malaria virus
Malaria is an acute illness caused by parasites that are transmitted to humans via female Anopheles mosquito bites, and It is a preventable and curable disease. African countries carry a disproportionately high percentage of the global malaria burden. Many western nations, however, succeeded in eliminating the disease during the first half of the twentieth century (Greenwood and Theonest Citation2002).
Human mobility plays a significant role in the persistence of malaria, even though the disease is not contagious and cannot spread directly from one person to another. Extensive research has been conducted on malaria elimination worldwide, with a focus on human mobility characteristics. For example, studies have investigated how to effectively target mobile and migrant populations at high risk of malaria and implement efficient intervention strategies (Khamsiriwatchara et al. Citation2011; Pongvongsa et al. Citation2019; Smith et al. Citation2019; Volkman et al. Citation2020). Other researchers have examined the contribution of foreign migrant workers and their mobility patterns on malaria transmission, as well as their risk of contracting the disease (Hlaing et al. Citation2015; Martins et al. Citation2020; Schicker et al. Citation2015; Wai et al. Citation2014). The connectivity of endemic regions through mobile populations has also been investigated, as understanding how these regions contribute to malaria spread is crucial (Guerra et al. Citation2019; Pindolia et al. Citation2013), as depicts domestic migration patterns in 2015, during a period when malaria was considered an endemic disease. Some studies have focused on providing malaria prevention recommendations and precautionary measures for travelers visiting high-risk regions to reduce the global burden of the disease and limit its spread to non-endemic areas (Cave et al. Citation2003; Shellvarajah, Hatz, and Schlagenhauf Citation2017). Additionally, one study involved mapping the travel patterns of infected individuals (Sinha et al. Citation2020), and other studies have examined the contribution of population flow to malaria persistence (Das et al. Citation2022; Gomes et al. Citation2020). Moreover, studies have been conducted to identify the environmental risk factors associated with malaria transmission among nonimmune travelers (Texier et al. Citation2013). and identified key traveler groups relevant to malaria transmission (Marshall et al. Citation2016). In addition to studies examining the association between human movement and malaria transmission, scholars proposed mathematical and simulation models to predict malaria spread with regard to mobility patterns (Chen et al. Citation2018; Li et al. Citation2022; Marshall et al. Citation2018). A number of studies constructed mobility patterns based on Global Positioning System (GPS) data and then investigated malaria disease (Hast et al. Citation2019, Citation2022).
Figure 5. Estimated domestic human migration patterns between subnational administrative divisions of countries where malaria was endemic in 2015 (WorldPop).
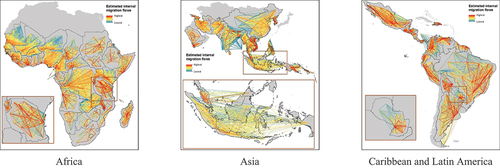
Although African and Asian countries carry the highest burden of malaria cases (Talapko et al. Citation2019), few studies have been conducted in these two regions. Additionally, a large body of studies on malaria in African counties utilized survey data. In contrast, in other regions, cellphone data and GPS logger devices (Hast et al. Citation2019) have been used in addition to survey data. Incorporating big data (e.g. mobile phones and social media platforms) into research can reduce bias in outcomes, enable real-time data collection, and provide a larger sample size than traditional data collection methods (Garattini et al. Citation2019).
3.3. Ebola virus
Ebola Virus Disease (EVD), also known as Ebola hemorrhagic fever, is a rare infectious disease resulting in a severe and mostly fatal illness in humans. Ebola outbreaks typically begin from a single case of zoonotic transmission, subsequently human-to-human transmission through direct contact or contact with contaminated bodily fluids or infected fomites (Jacob et al. Citation2020). Among several sources of infection, human-to-human transmission has been considered a major source of Ebola outbreaks worldwide. For example, health-care professionals and workers have frequently been diagnosed with the Ebola virus since they are in close contact with infected individuals and traveling into endemic regions.
Migration of contaminated animals and human are determined as the source of introducing the virus into regions. The Ebola virus has been extensively studied over decades of research based on utilizing human mobility factors on top of other determinants, including developing prediction models, implementing simple and advanced regression models, and examining the effectiveness of mobility control on the disease. Notable studies on this topic include Vogel et al. (Citation2015), who applied stochastic simulation based on cell phone data to monitor movement and travel patterns in West African countries to shed light on Ebola propagation. Additionally, Djiomba Njankou and Nyabadza (Citation2022) considered applying a mathematical model to examine the potential influence of migrants on the Ebola virus dynamic and investigating how mobility causes to circulate of the virus within communities by utilizing Susceptible-Infected-Isolated-Removed (SIIR) model (Rebaza Citation2021). Valdez et al. (Citation2015) investigated the propagation of the Ebola virus between countries caused by travel and forecasting Ebola epidemic paths (Kramer et al. Citation2016). Other studies considered regression analysis to explore the association between different forms of human mobility and Ebola transmission. For instance, factors influencing US travelers domestic travel avoidance due to the confirmed cases of the Ebola virus in late 2014 were described (Cahyanto et al. Citation2016). In response to the 2014 Ebola outbreak, several countries implemented travel bans in areas with an Ebola outbreak in 2014, aiming to limit the spread of the virus to new regions (Peak et al. Citation2018; Poletto et al. Citation2014). On the other hand, there were people with relatively high mobility despite the existence of the Ebola virus in 2014–2016 (Fallah et al. Citation2022). The US Centers for Disease Control and Prevention (CDC) implemented a monitoring program for travelers who visited West Africa during 2014–2016 (Prue et al. Citation2019), aiming to prevent the spread of Ebola in the US. These studies showed that human mobility regulation played a pivotal role in curbing the spread of the Ebola virus, as detailed further in . EVD research, especially in West Africa, has used diverse data like surveys, flight itineraries, and cellphone data to study human movement and virus transmission, but the use of geolocation information from social media platforms for Ebola virus forecasting remains underexplored.
Table 2. Summary of studies pertaining to human mobility and Ebola infectious disease.
3.4. Human Immunodeficiency Virus (HIV)
HIV remains a significant global public health issue, with over 40 million lives lost to the disease so far. The virus can be transmitted through the exchange of body fluids from infected individuals, including semen, vaginal secretions, blood, breast milk, and from mother to child. Although there is no specific cure for HIV infections (Cohen Citation1998; Shaw and Eric Citation2012), effective prevention programs, early diagnosis, and access to treatment and care have transformed HIV into a manageable chronic health condition, allowing people to live longer, healthier lives.
Over many years of research, it has been proven that human mobility plays a critical role in the prevention and treatment of HIV. In recent years, the world has faced significant challenges caused by human mobility; the rate of domestic and international displaced populations is at a record high, and pressures caused by migration are increasing, particularly for those who live in the lowest-resource settings. According to the literature review, three possible scenarios can be considered during the migration in terms of HIV spread: (1) migrants travel to destinations from regions with a high risk of HIV infections. In this case, the risk of HIV transmission potentially increases in the host destinations (von Wyl et al. Citation2011); (2) migrants travel from low-risk regions to high-risk areas of HIV. In this case, migrants are possibly infected and carry the virus to their own regions (Rai et al. Citation2014), and (3) both the origins and destinations are regarded as high-risk areas (Agadjanian Citation2016). Factors such as travel conditions, accommodations, and work environments can increase migrants’ risk of exposure to HIV. Separation from their families and spouses may lead a significant portion of migrants to engage in sexual behaviors that increase their risk of contracting HIV (Wang et al. Citation2013). HIV prevalence varies among different mobile groups. For example, studies show that HIV risk is significantly high among the drivers whose trip is longer and who spend nights on their trip (Delany-Moretlwe et al. Citation2014), and female sex workers who experienced forced sex and violence (Pannetier et al. Citation2018). In addition, knowledge regarding HIV and migrants’ perceptions and practices vary across different mobile groups (Agadjanian, Carlos, and Boaventura Citation2011). Furthermore, some mobile populations may face barriers to accessing HIV prevention and treatment services, including a lack of HIV treatment centers (Vignier et al. Citation2018). Human mobility facilitates the geographic transmission of HIV infectious disease at the local and global scale, as illustrated in , based on the relevant literature. Thus, understanding mobility patterns will greatly improve HIV prevention programs, manage HIV, and decrease the spread of the HIV virus. Predominantly based in Africa and Asia, the selected HIV studies primarily employed various regression models and relied heavily on survey data. Fewer studies considered mathematical and stochastic models or alternative data sources like mobile location and social media geolocation data for forecasting HIV transmission.
Table 3. A brief summary of studies related to HIV and human mobility.
3.5. Influenza virus
Influenza is an acute respiratory infection and a global threat to human health, and it caused four pandemics in the last century. As the existing knowledge regarding the influenza virus evolution, the transmission and distribution of this virus have increased, and avenues to future pandemics have become apparent (Harrington, Kackos, and Webby Citation2021). The influenza virus spreads rapidly, particularly in crowded places such as nursing homes and educational centers. This demonstrates the pivotal role human movement plays in transporting the virus to new regions.
When an infected individual coughs or sneezes, infectious droplets are dispersed into the environment and can spread up to a meter; if another person visits the contaminated environment, the individual will likely be infected and move the virus to a new area. Studies confirmed that human mobility is a source of transmission. For instance, during the 2016–2017 influenza season, the proportion of mobility significantly reduced among female populations and individuals with higher socioeconomic conditions in order to minimize the risk of influenza virus spread (Eum and Yoo Citation2022). The virus can also be transmitted by infected passengers on international flights (Foxwell et al. Citation2011), and then transmitted to new communities. In 2007, a significant outbreak of influenza occurred in Australia, prompting the government to implement movement restrictions and quarantine for infected people to contain the virus (Taylor et al. Citation2008). It is also evident that traveling by jet aircraft is a great vector for influenza transmission, and potentially can be reduced if travelers consider precautionary measures (Hanley and Birthe Citation2010; Masuet-Aumatell, Toovey, and Zuckerman Citation2013; Yanni et al. Citation2010). Furthermore, the behaviors of international travelers also play a key role in the transmission of the influenza virus, including attitudes and perceptions of travelers (Sharangpani et al. Citation2011). More recently, another wave of the influenza epidemic was documented in New South Wales in 2018, with overseas and local travel identified as the primary drivers of the epidemic (Marsh et al. Citation2022). There is growing evidence to suggest that human mobility plays a role in the transmission of the virus. Yet, only one study indicated that there was little evidence to support the association between influenza and human mobility in Hawaii and the United States-affiliated Pacific Islands (USAPI), in 2009 (Miller et al. Citation2012). Nevertheless, understanding human mobility patterns is widely regarded as an essential element in efforts to reduce influenza transmission and ultimately eradicate the virus (Wesolowski et al. Citation2012). The selected influenza studies, largely from the Americas and Australia, commonly used statistical methods and occasionally neural networks for estimating transmission pathways, as presented in .
Table 4. Summary of influenza virus and human mobility patterns.
3.6. Hepatitis virus
Hepatitis is a general term referring to inflammation of the liver and is caused by five main hepatitis viruses: A, B, C, D, and E. Hepatitis A and E are typically caused by the ingestion of contaminated water or food, while the other types mainly result from parenteral contact with contaminated body fluids. Common modes of transmission include receiving infected blood, using contaminated equipment, transmission between family members, through sexual contact, and from mother to baby (Razavi Citation2020).
Studies show that those who travel to hepatitis-endemic countries are at great risk of being infected, which then leads to the spread of the virus in their home country (Lu et al. Citation2018). Specific guidance is constituted by many developed countries for in- and out travelers who are from hepatitis endemic regions or visiting areas with a high risk of hepatitis virus, and provide awareness programs for these groups of population (Frew et al. Citation2017; Nielsen et al. Citation2012; Nielsen, Petersen, and Larsen Citation2009). For example, in 2009, East Asia and Southeast Asia were considered highly endemic zones for hepatitis B and included many tourist destinations for Australians (Leggat et al. Citation2009), and travelers were encouraged to take precautionary measures to reduce the possibility of virus transmission back to their home countries. Similarly, travelers, especially those visiting endemic regions, have been identified as a primary cause of hepatitis A and B virus transmission in European countries (Pedersini et al. Citation2016; Zuckerman and Hoet Citation2008). Likewise, countries that accept refugees and migrants from war-torn countries have witnessed significant increases in the incidence of hepatitis, as observed in Australia (Sievert et al. Citation2018). Upon relocation to host countries, these groups often face barriers such as limited access to healthcare services and challenges related to their socioeconomic status (Kowdley et al. Citation2012; Tiittala et al. Citation2018). As human mobility has been recognized as a factor in the transmission of hepatitis viruses between countries, regions with a high risk of hepatitis infection may require more stringent monitoring strategies regarding human movement. The literature review reveals a concentration of studies on Europe and Australia, using various regression analyses for methodology similar to other diseases, as it can be observed in .
Table 5. Summary of studies pertaining to hepatitis virus and human mobility.
3.7. Coronavirus disease of 2019 (COVID-19)
COVID-19 is an infectious disease related to the SARS-CoV-2 virus, and it was first reported in Wuhan, China (Li et al. Citation2020). The global spread of COVID-19 and the thousands of deaths in the early stage of the outbreak caused by COVID-19 led the World Health Organization to announce a pandemic on the 12th of March 2020. The health impact of COVID-19 vary across different groups. Often, infected populations develop mild to moderate illness, and they recover without hospitalization (Ciotti et al. Citation2020). Coronavirus can be transmitted through direct contact (human-to-human or droplet) and indirect contact (infected objects and airborne contagion). The transmission of COVID-19 has been facilitated by human interactions, which are often associated with movement. Studies have shown that both local and long-distance movements play a significant role in the spread of the virus within and among communities (Alessandretti Citation2022). To prevent transmission, many countries have implemented restriction policies on human movement (Gao et al. Citation2020; Her et al. Citation2022, Li et al. Citation2020), and global mobility patterns have profoundly changed (Bao et al. Citation2022; Dobbie et al. Citation2022; Sigler et al. Citation2021). Researchers have put substantial efforts into exploring the relationship between human mobility and coronavirus transmission (Lee et al. Citation2020).
The COVID-19 outbreak stands out as one of the largest pandemics ever recorded in modern human history, and it significantly impacted global human mobility patterns during the pandemic (Sigler et al. Citation2021). Research indicates that the interconnectedness fostered by globalization significantly expedited the dissemination of COVID-19, despite many countries implemented travel and mobility restrictions. This suggests that the global flow of goods, services, and people inherent to globalization can undermine containment strategies, making the virus more difficult to control (Bickley et al. Citation2021; Vannoni et al. Citation2020). Additionally, a study analyzed cross-border mobility data revealed that containment measures implemented in the destination country, coupled with school closures in the country of origin, were more successful in reducing cross-border movements than either international travel bans or fears related to COVID-19 case numbers and deaths (Docquier et al. Citation2022). Nonetheless, while numerous countries enacted early movement restrictions to mitigate the spread of COVID-19, tackling such a global pandemic effectively calls for international collaboration to adopt unified and effective measures (Assefa et al. Citation2022).
In an effort to decelerate the spread of the virus, the majority of countries constituted movement restrictions, resulting in significant declines in almost all transport modes. Public transport, in particular, experienced the biggest drop in ridership (Hasselwander et al. Citation2021). Similar research conducted in Australia suggests that public transport ridership has returned but at considerably lower levels compared to pre-pandemic (Currie, Jain, and Aston Citation2021). The impact of COVID-19 on human mobility varied across different regions, with low-income populations in sub-Saharan Africa have experienced a reduction in travel and accessibility due to the decline of welfare and transport services (Behrens and Newlands Citation2022). However, the pandemic also offered some individuals the opportunity to explore alternative daily routines that may persist post-COVID-19. As such, transportation planners need to understand these alterations and effectively design future travel scheduling to gain public trust in using public transportation (Dianat, Hawkins, and Habib Citation2022; Zheng, Luo, and Ritchie Citation2021). These findings underscore the urgent need to develop a more robust public transportation system that can cope with future infectious disease outbreaks and be more resilient in the face of disruptions.
Studies revealed that the impact of COVID-19 on transportation modes varied significantly. Active modes of transportation, such as motorcycles, biking, and personal cars, were perceived as the least risky mode of transportation during the pandemic (Dingil and Esztergár-Kiss Citation2021). As walking and biking increased during the pandemic in Spain, the majority of people agreed to increase space for pedestrians and cyclists and recommended more restrictions on vehicle mobility (Awad-Nunez et al. Citation2021). As personal cars were perceived as a safer mode of transportation, people became more interested in purchasing cars for short trips rather than relying on public transportation (Luan et al. Citation2021). The pandemic had a particularly significant impact on those who relied on transit services, resulting in many individuals shifting to walking as their primary mode of transportation (Parker et al. Citation2021). Although transit services were considerably influenced during the early stages of the outbreak, public transportation gradually regained some trust after the easing of lockdowns (Ferreira et al. Citation2022). In Southeast Asian countries where personal car ownership is limited, public transportation continued to be the primary mode of transportation even during the pandemic, a significant portion of the population utilized non-motorized modes of transportation (Abdullah et al. Citation2021; Paul et al. Citation2021). In fact, travelers underwent considerable changes in their transportation modes during the pandemic, as compared to the pre- and post-pandemic periods (Nikiforiadis et al. Citation2022). Comprehending these changing patterns can assist policymakers in turning the COVID-19 pandemic into an opportunity to invest in sustainable and flexible public transportation infrastructure (Ehsani et al. Citation2021). By addressing the evolving transportation needs of individuals and communities, countries can ensure that their transportation systems are better prepared to withstand future pandemics and other potential disruptions. The pandemic underscores the significance of having flexible and adaptable transportation systems that can cater to the changing needs of individuals and communities during infectious disease outbreaks.
The COVID-19 pandemic significantly reshaped human mobility from various aspects and shed light on existing societal challenges, including how the impacts of pandemics can vary across diverse population groups based on their socioeconomic status (SES), ethnicity, and age groups (Gauvin et al. Citation2021; Zeng et al. Citation2022). Numerous studies have confirmed that people in low SES regions continued to move even during the pandemic, particularly those employed in retail occupations (Brough, Freedman, and Phillips Citation2021; Levin et al. Citation2021; Roman et al. Citation2021; Wang et al. Citation2022). Similarly, evidence strongly suggests that COVID-19 incidence was unevenly distributed, possibly due to higher mobility rates, particularly among those individuals with lower SES (Lamb, Kandula, and Shaman Citation2021; Yechezkel et al. Citation2021). Moreover, mobility data revealed that nonwhite populations encountered higher exposure risks in the US (Coleman et al. Citation2022). Researchers also investigated the environmental factors influencing the daily mobility of older adults during COVID-19 and discovered that the duration of active travel significantly decreased for this demographic (from 174.72 to 76.92 minutes per week) (Choe, Du, and Sun Citation2022). The pandemic’s impacts also varied across gender groups (Mejía-Dorantes, Montero, and Barceló Citation2021). For further studies, please refer to . These findings illustrate that the impacts of infectious disease outbreaks can be different across various population groups in terms of their mobility patterns, with higher mobility observed among less-educated people, lower-income populations, and migrants. These insights highlight the need for policymakers to address these disparities and ensure that transportation systems can meet the needs of all individuals and communities, especially during pandemics. Implementing such policies can assist in ensuring that everyone has access to safe and reliable transportation options, irrespective of their socioeconomic status, ethnicity, age, or gender.
Table 6. Summary of studies related to COVID-19 infectious disease and human mobility patterns.
Robust prediction models can effectively forecast the outbreak of the disease based on the availability of mobility data (Li et al. Citation2021) and other historical data; governments can then implement restriction policies to reduce the transmission of infectious diseases. Over the course of the COVID-19 pandemic, studies used various prediction approaches, for instance, proposing prediction models based on connectedness, mobility patterns, and digital proximity tracing for predicting new cases of COVID-19 (Cencetti et al. Citation2021; Vahedi, Karimzadeh, and Zoraghein Citation2021). Moreover, statistical models were deployed to effectively forecast new cases based on public mobility data (Guan et al. Citation2021; Ilin et al. Citation2021; Kuniya Citation2020) and predict international mobility that allowed the model to detect disease transmission (Luca et al. Citation2022). Other scholars considered developing mathematical models for simulating COVID-19 transmission and examined how transmission varied over time (Chen et al. Citation2020; Giordano et al. Citation2020; Ivorra et al. Citation2020; Kucharski et al. Citation2020) and then combined stochastic models with data recorded cases of COVID-19. Furthermore, graph modeling was also deployed, and it was able to identify undetected cases of infections based on mobility patterns and social distancing measures (Alguliyev, Aliguliyev, and Yusifov Citation2021). Examining international and domestic mobility trends during the COVID-19 pandemic has been crucial for understanding the spread of the virus (Rowe et al. Citation2023; Shepherd et al. Citation2021). Identifying regions with a higher risk of infection and analyzing the influence of human mobility on potential future COVID-19 outbreaks can provide valuable insights for policymakers and public health officials (Chang et al. Citation2021). Similarly, the Long Short Term Memory neural network model was implemented to forecast the rate of new cases, and the model was first trained based on factual data related to virus transmission (Banerjee and Lian Citation2022). All in all, comprehensive predictive approaches can significantly reduce virus transmission and accurately identify regions with higher vulnerability using mobility data and other clinical data.
4. Discussion
Key findings of published articles discussing the association between human mobility and eight types of infectious diseases are summarized in this review paper. In an era of pandemics, mobility can indeed disrupt the efforts to isolate and treat infectious diseases. Early and timely responses are essential to prevent a pandemic crisis. In light of the significant impact of human mobility on the spread of various infectious diseases, many countries have formalized preventive measures for travelers, including travel restrictions during an infectious disease pandemic. For instance, the early implementation of the lockdown in Wuhan considerably decelerated the spread of COVID-19 at the initial stage of the pandemic (Lau et al. Citation2020). Similarly, mobility restrictions were implemented in Sierra Leone due to an unprecedented scale of Ebola virus cases in 2015 (Peak et al. Citation2018). In 2009, public health authorities identified East Asia and Southeast Asia as regions with high endemic rates of hepatitis B, which are also popular tourist destinations. As a result, travelers were advised to take preventive measures to reduce the risk of bringing the virus back to their home countries (Leggat et al. Citation2009; Sievert et al. Citation2018). Similarly, government health agencies have issued recommendations for travelers to take precautions when visiting areas known for other infectious diseases, such as influenza and vector-borne illnesses (Falcon-Lezama et al. Citation2017; Foxwell et al. Citation2011; Prue et al. Citation2019; Shellvarajah, Hatz, and Schlagenhauf Citation2017).
Although a considerable body of research suggests that mobile populations are key contributors to the transmission of infectious diseases, some studies challenge this view. For instance, a study indicated that geographical features had a greater impact on the spread of Ebola than human mobility (Kramer et al. Citation2016). Likewise, the type of mobility, such as rural-rural versus rural-urban, has been shown to have varying effects on the transmission of HIV (Coffee et al. Citation2005). Additionally, studies have found that mobile populations may even exhibit lower risk behaviors compared to local populations in the context of HIV (Camlin et al. Citation2017; Mantell et al. Citation2011). Human mobility was found to have a lesser role in the spread of influenza in specific U.S. regions (Miller et al. Citation2012). According to the literature reviewed, the relationship between human mobility and the spread of infectious diseases varies depending on the specific illness. For example, mobility was a significant factor in the spread of COVID-19, whereas its influence appears to be less pronounced in the cases of HIV and influenza. These divergent findings suggest that the relationship between human mobility and infectious disease transmission is complex and not universally applicable, warranting a nuanced approach in public health strategies.
Given the complexities and varying evidence surrounding the topic, we next focus on critical gaps that require further exploration to refine our understanding of this topic. Upcoming sections will specifically address issues related to data quality, methodological advancements, the resulting implications for public health, and policy guidance based on the reviewed articles.
4.1. Data quality
Data is the main body of research, and its reliability, accuracy, consistency, and completeness must be considered in a study. Numerous studies probing the connection between human mobility and infectious diseases have relied heavily on survey questionnaires. These can be administered through various channels such as paper, online, mobile, or a combination of these methods. Mobile and online surveys are often the most cost-effective, yet their reach may be limited, potentially excluding participants who can only respond through alternate methods. Traditional face-to-face interviews or paper surveys serve as viable alternatives in such scenarios.
Nonetheless, survey-based studies have challenges. For instance, capturing hard-to-reach respondents can be a significant hurdle. Besides, surveys are prone to response and nonresponse errors, their reach may be limited to specific geographical regions, and they often yield a relatively small sample size (Dillman and Bowker Citation2001). Health professionals express particular concern about these errors, especially in oral health surveys (Locker Citation2000). Moreover, some studies rely on preexisting datasets from previous research, potentially leading to data that is outdated or lacks comprehensive information. Parameters borrowed from studies conducted in different geographical locations might also skew results due to regional disparities (Zhu et al. Citation2019). The use of varied data collection methods within a single study can result in a sample that is not accurately representative (Squiers et al. Citation2018). During the early stages of a disease outbreak, data scarcity can hamper the accuracy and effectiveness of public health interventions and policy decisions. Surveys conducted post-surge may fail to provide an accurate picture of the initial spread. Furthermore, inconsistencies between surveillance data and epidemiological reports can complicate accurate assessments and impede the development of effective public health strategies (Perrotta et al. Citation2022). Most studies are local, focusing on specific geographical regions. None of the selected articles examined the association of mobility and infectious disease based on regional or global survey data, likely due to the prohibitive costs of large-scale surveys. The tendency to use small sample sizes is a significant limitation, often exacerbated when study areas are remote and challenging to access (Li et al. Citation2022). Therefore, to surmount these challenges, it is crucial to devise comprehensive survey questionnaires that are representative, reliable, and can be accurately administered across diverse geographical regions (Hill Citation1998).
Apart from COVID-19, a relatively small proportion of literature considered big data (e.g. mobile phones, GPS, social media) to examine the linkage between mobility and infectious disease transmission. Using mobile and GPS data sources considerably increases the sample size within a certain border and enables monitoring the effectiveness of mobility restrictions, contact tracing, and identifying potential sources of spread. However, while these data sources offer many advantages, they are not without drawbacks. Privacy concerns abound, and capturing cross-border travel patterns can prove challenging. For instance, some countries host a large population of undocumented migrants, and possibly they regularly travel in and out of the host countries; yet, this group is probably reluctant to participate, and lack of their participation may be led to bias (Wesolowski et al. Citation2015). Likewise, GPS data contain certain errors caused by GPS logger devices. Likely, participants forget to carry the logger or allow others to carry the GPS loggers (Hast et al. Citation2022). Nonetheless, mobile and GPS data enable researchers to study the connection between human mobility and infectious diseases at a larger scale compared to questionnaires and also offer more comprehensive insights into the dynamics between mobility and infectious diseases. However, given the sensitive nature of such data, it is vital to exercise caution in its collection and analysis.
Social media data sources, such as Twitter, Facebook, Instagram, and Weibo, are valuable sources of mobility data, which allows researchers to conduct insightful studies (Li et al. Citation2021; Souza et al. Citation2019). Mobility data extracted from social media platforms have been extensively applied in investigating the association between COVID-19 transmission and human movement patterns, especially Twitter, Facebook, and Weibo (Hu et al. Citation2021). Such rich resources, however, have not been widely utilized in other infectious diseases, including malaria, Ebola, Zika, HIV, and influenza. Analyzing social media data enable scholars to track human behaviors and activities in addition to extracting mobility patterns. Yet, there has been limited attention given to utilizing these data sources in exploring the connection between human mobility and infectious diseases beyond COVID-19. Future studies can consider integrating various data sources, including large-scale survey data, mobile phone data, and social media (e.g. Facebook, Twitter, Instagram, and Weibo), to conduct research at regional and global levels. While the value of data and information sharing for managing infectious diseases is well-acknowledged, recent public health crises have heightened the need for effective cross-border communication, yet there is a lack of empirical research exploring the factors that influence the transfer and utilization of public health data and expertise (Liverani et al. Citation2018).
4.2. Methodological enhancement
Outbreaks of COVID-19 and pandemic flu or other types of infectious diseases have raised concerns regarding how governments utilize modeling to forecast a disease’s course. Models do not solely assist decision-makers in implementing disease control policies and efficiently administering resources but also inform conceptual frameworks for future research on infectious diseases; if designed models are unsound, they possibly do not generate the reliable predictions required to make good decisions (Grassly and Fraser Citation2008). Various types of models have been established throughout the course of studies, including statistical approaches, mechanistic state-space models (e.g. predictions, stochastic), and simplified arithmetic approaches (Zhang et al. Citation2022). Statistical analysis, prediction models, and stochastic simulations are mainly applied in the selected articles for this review.
Approximately 80% of the selected articles utilized statistical analyses – ranging from exploratory analysis to simple and complex regression models – to investigate the relationship between human mobility and infectious diseases and evaluate the effectiveness of social restrictions and interactions. While these analyses typically outline patterns and correlations inherent in the data, they often fall short in determining causal relationships. This limitation is particularly pronounced when multiple factors contribute to an outcome, as not all of these factors may be captured by the available data (Chambers and Trevor Citation2017). Furthermore, the majority of studies did not develop a robust methodology for handling missing data, which can have significant implications for the accuracy and validity of the research findings (Prue et al. Citation2019; Wai et al. Citation2014). Missing data can arise from various sources, such as non-response, measurement errors, or data collection challenges. When not properly addressed in the process of analyses, missing data can lead to biased estimates, reduced statistical power, and incorrect conclusions. In contrast, the majority of studies didn’t acknowledge this challenge in their research. It is essential for researchers to acknowledge the presence of missing data in their analyses, adopt suitable techniques to address this issue and assess the potential impact of missing data on the overall findings of their studies. For instance, to achieve a meaningful result from applying a multivariate analysis, the input data must be relatively large, but most studies only considered a small dataset (Fonner et al. Citation2021; Pongvongsa et al. Citation2019) while using this model. Hence, considering a proper model based on the dataset requires full attention for the analyses; otherwise, it is challenging to draw a generalized conclusion.
Nearly 10% of the publications included in this review developed mathematical models to predict the spread of infectious diseases. Major limitations of prediction models are to rely on a set of assumptions, like uniform distribution of cases over the course of the study, assuming equal distribution of cases in the entire study area, and setting constant parameters in the experiments (Kramer et al. Citation2016; Quam and Wilder-Smith Citation2016). Modeling approaches, particularly those aimed at understanding complex phenomena such as the spread of infectious diseases and the impact of human mobility, often face challenges in incorporating all relevant factors. Two primary reasons for this limitation are computational complexity and data scarcity (Zhu et al. Citation2019). This potentially can be addressed by leveraging a hybrid approach that combines data-driven machine learning techniques and mechanistic models with simplified, domain-specific models, allowing for efficient integration of available data and reduced computational demands. Adding measures of non-pharmaceutical interventions (e.g. rate of mobility change, social distancing, isolation), as well as pharmaceutical factors, including vaccine availability, accessibility to health care centers, resource availability, and various types of viral mutations, can enhance the performance of the developed models (Djiomba Njankou and Nyabadza Citation2022). The characteristics of each type of infectious disease need to be thoroughly reviewed first, to guide the model design. In sexually transmitted diseases, for example, the characteristics of mobility (e.g. short or long distance, domestic or cross-border), socio-demographics of mobile populations (e.g. age, gender, education level, income) (Zhang et al. Citation2020), and the legal migration status (legal or undocumented) play a fundamental role in disease transmission. The incorporation of all of these characteristics into forecasting models could potentially enhance the accuracy of prediction models. However, the majority of these features were not considered in the selected articles in this review. Likewise, for vector-borne diseases such as Zika, malaria, and dengue, one approach is to identify regions with a higher likelihood of infectious transmission using satellite imagery. This imagery can reveal critical environmental factors such as seasonal changes, vegetation cover, and water bodies, which are known to influence the habitats of disease vectors. After identifying these potential high-risk areas, researchers can then integrate this information with mathematical models. This combined approach provides a more comprehensive understanding of disease transmission patterns, allowing for more effective disease control and prevention strategies (Sinha et al. Citation2020), while less focus has been given to exploring such data sources. Another promising approach could be the integration of multi-source data based on a hybrid of models across disciplines. Additional challenges for prediction models are the computational complexity and storage, particularly if the given data is huge; considering high-performance computing techniques are promising directions to overcome such challenges (Wang et al. Citation2021). Long-standing infectious diseases continue to pose challenges to global health infrastructure. Thus, it is important to continually refine and enhance these models, as they play a vital role in developing more effective public health strategies and facilitating a better understanding of the complex dynamics underlying infectious disease transmission and human mobility.
4.3. Public health implications
This review paper transcends academic discussion to offer actionable insights crucial for improving public health policy and infectious disease control. By identifying key research gaps and vulnerabilities in existing systems, we highlight the urgent need for targeted action. These findings have considerable implications in informing effective disease control interventions and improving public health outcomes. This review serves not just as academic inquiry but as a vital framework for health professionals and healthcare providers. With this context in mind, we will now delve into the distinct public health ramifications identified by our review.
First, the impact of mobility on infectious diseases varies based on the characteristics of mobility. Migrants and long-distance travelers often face substantial challenges due to factors such as language barriers, discrimination, difficulties in accessing healthcare services, and uncertain legal status in their host countries (Desgrees-du-Lou et al. Citation2016). Beyond transporting communicable diseases to their destinations, these groups can also become particularly vulnerable to infection. This vulnerability may arise from their destinations being high-risk areas for infectious diseases or from their marginalization and exclusion within the communities they join. Consequently, it’s essential that public health initiatives account for these unique challenges and vulnerabilities to ensure effective disease prevention and control among these groups (Coffee et al. Citation2005). To successfully promote prevention measures and enhance timely treatment, healthcare services require to recognize this critical challenge and must consider the unique challenges faced by these groups, ensuring that they have access to appropriate healthcare services and preventative measures to protect both themselves and the communities in which they reside. Second, the public plays an essential role in containing a communicable disease if they are well informed regarding infectious disease threats. However, the reviewed articles suggest that public awareness is significantly low among mobile populations regarding infectious diseases (Guo et al. Citation2017; Teich et al. Citation2018). Similarly, public perception is critically important toward infectious diseases to decelerate transmission. For instance, the Ebola outbreak in early 2013 rapidly spread beyond the original geographical location due to the lack of precautionary measures by public in the infected communities (Coltart et al. Citation2017). Therefore, it is considerably important for public health professionals to pay more attention and invest in awareness programs. Third, massive global travel, urbanization, and population growth (Bloom and Cadarette Citation2019) have intensified the need for more comprehensive research in this area. To address these concerns, public health professionals require to closely conduct research with transportation sectors and implementing effective surveillance systems. Likewise, urban planning and public health intervention must be closely integrated, with an emphasis on creating healthy living environments that minimize the risk of disease transmission while ensuring that healthcare services are accessible and effective for all residents. Subsequently, through interdisciplinary collaboration, researchers can develop more effective strategies to mitigate the spread of infectious diseases. Finally, in an era marked by rapid globalization, both domestic and international travel have increased considerably, profoundly impacting the spread of diseases on local and global scales. This underscores the necessity for health professionals to broaden their research scope beyond local contexts. Such wide-ranging investigations could shed light on how globalization exacerbates global health challenges and help pinpoint why certain regions struggle to stave off the threat of infectious diseases. For instance, African nations bear an excessively high percentage of the global malaria burden, while Asian countries shoulder over 70% of the global dengue virus burden (Bhatt et al. Citation2013). Understanding the factors contributing to these disparities is vital for crafting effective public health strategies and interventions.
4.4. Policy amendment
Recent years have witnessed outbreaks of previously unknown infectious diseases and the rapid spread of known infectious diseases beyond geographic regions where they initially emerged and typically exist, including reemerging diseases that significantly declined before. More recently COVID-19 pandemic (Wu, Chen, and Chan Citation2020), despite significant scientific and medical advancements, the global impact of the virus underscores the ongoing risk of outbreaks escalating into future epidemics and pandemics (Ciotti et al. Citation2019; Coltart et al. Citation2017). The next COVID-19-like pandemic could hit any time in the future now; it is, therefore, critical that countries establish a worldwide policy to rapidly and effectively respond to any future pandemics before it disrupts health systems and the economy. Policy interventions, in fact, have been proven to be effective in containing diseases and lowering the spread of viruses (Glover et al. Citation2020; van de Pas et al. Citation2019).
Research indicates that countries with higher domestic cases of infectious diseases are less likely to implement travel restriction policies (Smith Citation2006). Interestingly, countries with a larger proportion of older residents are less inclined to enact travel restrictions; moreover, contrary to expectations, countries with more robust healthcare systems are more likely to put travel restriction policies in place (Bickley et al. Citation2021). Studies also indicate that while countries with high social globalization are quicker to implement travel restrictions (Cash and Narasimhan Citation2000), those with effective governments and international political ties are less likely to do so. This presents a policy challenge of balancing international cooperation with national public health goals. Policymakers should thus consider the multifaceted impacts of globalization and human mobility when devising strategies to control infectious diseases, potentially through multilateral agreements that address these complexities. Studies also show that marginalized groups are more at risk of vulnerability in a pandemic (Holder-Dixon et al. Citation2022). Studies indicate that undocumented migrants are at higher risk for HIV and STDs due to factors like sexual behavior, lack of treatment, forced sex, and socioeconomic status (Pannetier et al. Citation2018; Swain et al. Citation2011). Certain groups, such as men who have sex with men, are particularly vulnerable (Garcia et al. Citation2017; Ramesh et al. Citation2014; Vanden Berghe et al. Citation2013; Zhang et al. Citation2017). These findings call for urgent policy adjustments in countries with high rates of infectious diseases, including easier access to treatment for migrants and measures to reduce violence against them.
4.5. Limitation
This study has a number of limitations that are important to acknowledge. First, the review was confined to two databases, Web of Science and PubMed, which do not encompass all journals. There may be a delay of several months before articles from other indexed journals are added to these databases, potentially excluding recent relevant literature. Secondly, the use of unsupervised machine learning in the initial screening stage may have inadvertently excluded some pertinent articles from the primary search pool. Thirdly, this review was restricted to only original, peer-reviewed papers, excluding high-quality articles that are under review and conference proceedings, potentially introducing a selection bias. Furthermore, the distribution of diseases studied was skewed, with approximately 43% of the reviewed papers focusing on COVID-19 and HIV, while the remaining 57% discussed Zika, Ebola, malaria, dengue, influenza, and hepatitis. This could have influenced the search results and the conclusions drawn from them. Given these limitations, future research should aim for a more expansive scope, encompassing a broader array of infectious diseases and human mobility, and consider other databases for a more comprehensive review.
5. Conclusion
This systematic review enriches the existing knowledge in the realms of public health and geography. The forces of globalization, urbanization, and population growth are increasingly interconnecting the world, thereby heightening the potential for the rapid spread of infectious diseases across geographical borders and within densely populated regions. With the continuous evolution of these global trends, it is paramount to create innovative, cross-disciplinary strategies in public health that address the intricate nexus between human mobility, environmental factors, and infectious disease transmission dynamics.
Our review suggests that the relationship between human mobility and the transmission of infectious diseases is intricate and not universally applicable. The impact of human mobility exhibits spatial and temporal disparities: spatially, it holds more significance in urban areas compared to rural ones, in regions with higher connectivity as opposed to those with lower connectivity, and in certain geographic areas over others; temporally, it manifests more prominently during the initial stages of outbreaks rather than the later phases. Furthermore, its influence varies across different types of diseases – it is more pronounced in respiratory infectious diseases than in sexually transmitted ones.
Despite an extensive body of research, certain knowledge gaps persist. We have identified four areas that warrant further exploration to deepen our understanding of this field. Research gaps in the existing studies of human mobility and infectious diseases include a limited focus on diseases other than COVID-19 and HIV, reliance on survey data with its inherent limitations, a predominant use of statistical analyses over mathematical modeling, and a local rather than global perspective. These gaps hinder our understanding and the development of comprehensive strategies. Furthermore, this review highlights critical gaps in public health policy and infectious disease control, emphasizing the unique vulnerabilities of migrants and long-distance travelers. It underscores the need for tailored public health initiatives and better public awareness programs, particularly for mobile populations. The review also calls for interdisciplinary collaboration to address the challenges of globalization, urbanization, and varying disease burdens across regions. These actionable insights aim to guide public health professionals in crafting more effective strategies for improved health outcomes.
Additionally, in this era, despite scientific and medical advancements, the threat of infectious disease outbreaks evolving into global pandemics remains. Countries exhibit various approaches to public health measures like travel restrictions, often influenced by factors such as domestic case rates, age demographics, healthcare capacity, and international political engagements. Policymakers face the challenge of balancing international cooperation with national public health imperatives. Special attention needs to be given to vulnerable populations, who are at higher risk of infection due to various socio-economic factors and lack of accessibility to medical facilities. The findings underscore the need for a coordinated, multifaceted, and globally minded approach to public health policy to better prepare for and manage future outbreaks.
By addressing these identified areas of knowledge gaps and embracing a holistic, interdisciplinary approach, future research can substantially enhance our comprehension of the complex interplay between human mobility and infectious diseases. This, in turn, will guide the formulation of more precise and effective public health strategies to mitigate the impacts of these diseases, catering to the needs of various populations across different geographical regions.
Disclosure statement
No potential conflict of interest was reported by the author(s).
Data availability statement
Data sharing is not applicable to this article as no new data were created or analyzed in this study.
Additional information
Funding
Notes on contributors
M. Naser Lessani
M. Naser Lessani is currently a PhD student in the Department of Geography at the University of South Carolina. He received his B.S from Kabul Polytechnique University, Kabul, Afghanistan, and MSc from Central South University, Changsha, China. His primary research interests are geospatial big data analytics, human mobility, parallel spatial computing and GIS.
Zhenlong Li
Zhenlong Li is an Associate Professor in the Department of Geography and Director of the Center for GIScience and Geospatial Big Data at the University of South Carolina. Dr. Li received his PhD in Earth Systems and Geoinformation Sciences from George Mason University in 2015. His primary research field is GIScience with a focus on geospatial big data analytics, spatial computing, and deep learning/GeoAI with applications to disaster management, human mobility, and public health.
Fengrui Jing
Fengrui Jing is a Postdoctoral Fellow in the Department of Geography at the University of South Carolina and an upcoming Assistant Professor in the Department of Public Health and Preventive Medicine at Jinan University. Dr. Jing received his Ph.D. in Cartography and Geographic Information Systems from Sun Yat-sen University in 2021. His research interests focus on spatial statistics and spatial data science, spatial epidemiology, and environmental health.
Shan Qiao
Shan Qiao is an Associate Professor in the Department of Health Promotion Education and Behavior in Arnold School of Public Health at the University of South Carolina. Dr. Qiao received her Ph.D. in International Health from Bloomberg School of Public Health at Johns Hopkins University in 2012. Her primary research field is HIV prevention, treatment and care with a focus on mental health, behavior changes and interventions, health disparities and implementation science.
Jiajia Zhang
Jiajia Zhang is a Professor in the Department of Epidemiology and Biostatistics at the University of South Carolina. Dr. Zhang’s main research and teaching interests focus on investigating the association between the mortality/incidence of a certain disease with its potential risk factors using survival analysis.
Bankole Olatosi
Bankole Olatosi is an Assistant Professor in the Department of Health Services Policy and Management at the University of South Carolina. Dr. Olatosi’s research interests are in the fields of Health Analytics, HIV/AIDS, and COVID-19.
Xiaoming Li
Xiaoming Li is Professor in the Department of Health Promotion, Education and Behavior in Arnold School of Public Health at the University of South Carolina, SC SmartState Endowed Chair for Clinical and Translational Research, Director of SC SmartState Center for Healthcare Quality (CHQ), and Director of USC Big Data Health Science Center (BDHSC). He received his Ph.D. in Psychology from University of Minnesota in 1992. As a social science methodologist with a very strong background in health disparity, intervention clinical trial design, biostatistics, and psychometrics, Dr. Li has been working with an interdisciplinary team of clinicians, psychologists, and other health care professionals for 30 years in the areas of social and psychological aspects of HIV/AIDS, HIV treatment cascade, mental health, child development, data sciences, and research training in both domestic and international settings.
References
- Abdullah, M., N. Ali, S. Arif Hussain, A. Bilal Aslam, and M. Ashraf Javid. 2021. “Measuring Changes in Travel Behavior Pattern Due to COVID-19 in a Developing Country: A Case Study of Pakistan.” Transport Policy 108: 21–33. https://doi.org/10.1016/j.tranpol.2021.04.023.
- Agadjanian, V. 2016. “Research on International Migration within Sub-Saharan Africa: Foci, Approaches, and Challenges.” The Sociological Quarterly 49 (3): 407–421. https://doi.org/10.1111/j.1533-8525.2008.00121.x.
- Agadjanian, V., A. Carlos, and C. Boaventura. 2011. “Health Costs of Wealth Gains: Labor Migration and Perceptions of HIV/AIDS Risks in Mozambique.” Social Forces 89 (4): 1097–1117.
- Alessandretti, L. 2022. “What Human Mobility Data Tell Us About COVID-19 Spread.” Nature Reviews Physics 4 (1): 12–13. https://doi.org/10.1038/s42254-021-00407-1.
- Alguliyev, R., R. Aliguliyev, and F. Yusifov. 2021. “Graph Modelling for Tracking the COVID-19 Pandemic Spread.” Infectious Disease Models 6:112–122. https://doi.org/10.1016/j.idm.2020.12.002.
- Angelo, K. M., R. J. Stoney, G. Brun-Cottan, K. Leder, M. P. Grobusch, N. Hochberg, S. Kuhn, et al. 2020. “Zika among international travellers presenting to GeoSentinel sites, 2012-2019: implications for clinical practice.” Journal of Travel Medicine 27 (4). https://doi.org/10.1093/jtm/taaa061.
- Arisco, N. J., C. Peterka, and M. C. Castro. 2021. “Cross-Border Malaria in Northern Brazil.” Malaria Journal 20 (1): 135. https://doi.org/10.1186/s12936-021-03668-4.
- Assefa, Y., C. F. Gilks, S. Reid, R. van de Pas, D. G. Gete, and W. Van Damme. 2022. “Analysis of the COVID-19 Pandemic: Lessons Towards a More Effective Response to Public Health Emergencies.” Globalization and Health 18 (1): 10. https://doi.org/10.1186/s12992-022-00805-9.
- Awad-Nunez, S., R. Julio, B. Moya-Gomez, J. Gomez, and J. Sastre Gonzalez. 2021. “Acceptability of sustainable mobility policies under a post-COVID-19 scenario. Evidence from Spain.” Transport Policy (Oxf) 106:205–214. https://doi.org/10.1016/j.tranpol.2021.04.010.
- Banerjee, S., and Y. Lian. 2022. “Data Driven COVID-19 Spread Prediction Based on Mobility and Mask Mandate Information.” Applied Intelligence (Dordr) 52 (2): 1969–1978. https://doi.org/10.1007/s10489-021-02381-8.
- Bao, H., X. Zhou, Y. Xie, Y. Zhang, and L. Yanhua. 2022. “COVID-GAN+: Estimating Human Mobility Responses to COVID-19 Through Spatio-Temporal Generative Adversarial Networks with Enhanced Features.” ACM Transactions on Intelligent Systems and Technology 13 (2): 1–23. https://doi.org/10.1145/3481617.
- Barbieri, D. M., B. Lou, M. Passavanti, C. Hui, I. Hoff, D. A. Lessa, G. Sikka, et al. 2021. “Impact of COVID-19 Pandemic on Mobility in ten Countries and Associated Perceived Risk for All Transport Modes.” PloS One 16 (2): e0245886. https://doi.org/10.1371/journal.pone.0245886.
- Barmak, D. H., C. O. Dorso, and M. Otero. 2016. “Modelling Dengue Epidemic Spreading with Human Mobility.” Physica A Statistical Mechanics & Its Applications 447:129–140. https://doi.org/10.1016/j.physa.2015.12.015.
- Behrens, R., and A. Newlands. 2022. “Revealed and Future Travel Impacts of COVID-19 in Sub-Saharan Africa: Results of Big Data Analysis and a Delphi Panel Survey.” Journal of Transport and Supply Chain Management 16. https://doi.org/10.4102/jtscm.v16i0.758.
- Bhatt, S., P. W. Gething, O. J. Brady, J. P. Messina, A. W. Farlow, C. L. Moyes, J. M. Drake, et al. 2013. “The global distribution and burden of dengue.” Nature 496 (7446): 504–507. https://doi.org/10.1038/nature12060.
- Bickley, S. J., H. F. Chan, A. Skali, D. Stadelmann, and B. Torgler. 2021. “How Does Globalization Affect COVID-19 Responses?” Globalization and Health 17 (1): 57. https://doi.org/10.1186/s12992-021-00677-5.
- Bloom, D. E., and D. Cadarette. 2019. “Infectious Disease Threats in the Twenty-First Century: Strengthening the Global Response.” Frontiers in Immunology 10:549. https://doi.org/10.3389/fimmu.2019.00549.
- Bomfim, R., S. Pei, J. Shaman, T. Yamana, H. A. Makse, J. S. Andrade Jr., A. S. Lima Neto, and V. Furtado. 2020. “Predicting Dengue Outbreaks at Neighbourhood Level Using Human Mobility in Urban Areas.” Journal of the Royal Society Interface 17 (171): 20200691. https://doi.org/10.1098/rsif.2020.0691.
- Bonal, X., and S. Gonzalez. 2020. “The Impact of Lockdown on the Learning Gap: Family and School Divisions in Times of Crisis.” International Review of Education 66 (5–6): 635–655. https://doi.org/10.1007/s11159-020-09860-z.
- Brady, O. J., P. W. Gething, S. Bhatt, J. P. Messina, J. S. Brownstein, A. G. Hoen, C. L. Moyes, A. W. Farlow, T. W. Scott, and S. I. Hay. 2012. “Refining the Global Spatial Limits of Dengue Virus Transmission by Evidence-Based Consensus.” PLoS Neglected Tropical Diseases 6 (8): e1760. https://doi.org/10.1371/journal.pntd.0001760.
- Brough, R., M. Freedman, and D. C. Phillips. 2021. “Understanding Socioeconomic Disparities in Travel Behavior During the COVID-19 Pandemic.” Journal of Regional Science 61 (4): 753–774. https://doi.org/10.1111/jors.12527.
- Cahyanto, I., M. Wiblishauser, L. Pennington-Gray, and A. Schroeder. 2016. “The Dynamics of Travel Avoidance: The Case of Ebola in the U.S.” Tourism Management Perspectives 20:195–203. https://doi.org/10.1016/j.tmp.2016.09.004.
- Camlin, C. S., A. Akullian, T. B. Neilands, M. Getahun, A. Bershteyn, S. Ssali, E. Geng, et al. 2019. “Gendered Dimensions of Population Mobility Associated with HIV Across Three Epidemics in Rural Eastern Africa.” Health & Place 57:339–351. https://doi.org/10.1016/j.healthplace.2019.05.002.
- Camlin, C. S., A. M. El Ayadi, Z. A. Kwena, W. McFarland, M. O. Johnson, T. B. Neilands, E. A. Bukusi, and C. R. Cohen. 2017. “High Mobility and HIV Prevalence Among Female Market Traders in East Africa in 2014.” Journal of Acquired Immune Deficiency Syndromes 74 (5): e121–e128. https://doi.org/10.1097/QAI.0000000000001252.
- Cash, R. A., and V. Narasimhan. 2000. “Impediments to Global Surveillance of Infectious Diseases: Consequences of Open Reporting in a Global Economy.” Bulletin of the World Health Organization 78:1358–1387.
- Castro, L. A., N. Generous, W. Luo, Y. Piontti, A. Pastore, K. Martinez, M. F. C. Gomes, et al. 2021. “Using heterogeneous data to identify signatures of dengue outbreaks at fine spatio-temporal scales across Brazil.” PLoS Neglected Tropical Diseases 15 (5): e0009392. https://doi.org/10.1371/journal.pntd.0009392.
- Cave, W., P. Pandey, D. Osrin, and D. R. Shlim. 2003. “Chemoprophylaxis Use and the Risk of Malaria in Travelers to Nepal.” Journal of Travel Medicine 10 (2): 100–105.
- Cencetti, G., G. Santin, A. Longa, E. Pigani, A. Barrat, C. Cattuto, S. Lehmann, M. Salathe, and B. Lepri. 2021. “Digital Proximity Tracing on Empirical Contact Networks for Pandemic Control.” Nature Communications 12 (1): 1655. https://doi.org/10.1038/s41467-021-21809-w.
- Chambers, J. M., and J. H. Trevor. 2017. “Statistical Models.” In Statistical Models In S, 13–44.
- Chandrasekaran, N., M. Marotta, S. Taldone, and C. Curry. 2017. “Perceptions of Community Risk and Travel During Pregnancy in an Area of Zika Transmission.” Cureus 9 (7): e1516. https://doi.org/10.7759/cureus.1516.
- Chang, H. H., A. Wesolowski, I. Sinha, C. G. Jacob, A. Mahmud, D. Uddin, S. I. Zaman, et al. 2019. “Mapping imported malaria in Bangladesh using parasite genetic and human mobility data.” Elife 8. https://doi.org/10.7554/eLife.43481.
- Chang, M. C., R. Kahn, Y. A. Li, C. S. Lee, C. O. Buckee, and H. H. Chang. 2021. “Variation in Human Mobility and Its Impact on the Risk of Future COVID-19 Outbreaks in Taiwan.” BMC Public Health 21 (1): 226. https://doi.org/10.1186/s12889-021-10260-7.
- Chen, Q., M. Tsubaki, Y. Minami, K. Fujibayashi, T. Yumoto, J. Kamei, Y. Yamada, H. Kominato, H. Oono, and T. Naito. 2021. “Using Mobile Phone Data to Estimate the Relationship Between Population Flow and Influenza Infection Pathways.” International Journal of Environmental Research and Public Health 18 (14). https://doi.org/10.3390/ijerph18147439.
- Chen, T. M., J. Rui, Q. P. Wang, Z. Y. Zhao, J. A. Cui, and L. Yin. 2020. “A Mathematical Model for Simulating the Phase-Based Transmissibility of a Novel Coronavirus.” IInfectious Diseases of Poverty 9 (1): 24. https://doi.org/10.1186/s40249-020-00640-3.
- Chen, T. M., S. S. Zhang, J. Feng, Z. G. Xia, C. H. Luo, X. C. Zeng, X. R. Guo, Z. R. Lin, H. N. Zhou, and S. S. Zhou. 2018. “Mobile Population Dynamics and Malaria Vulnerability: A Modelling Study in the China-Myanmar Border Region of Yunnan Province, China.” Infectious Diseases of Poverty 7 (1): 36. https://doi.org/10.1186/s40249-018-0423-6.
- Choe, E. Y., Y. Du, and G. Sun. 2022. “Decline in Older adults’ Daily Mobility During the COVID-19 Pandemic: The Role of Individual and Built Environment Factors.” BMC Public Health 22 (1): 2317. https://doi.org/10.1186/s12889-022-14780-8.
- Ciotti, M., S. Angeletti, M. Minieri, M. Giovannetti, D. Benvenuto, S. Pascarella, C. Sagnelli, M. Bianchi, S. Bernardini, and M. Ciccozzi. 2019. “COVID-19 Outbreak: An Overview.” Chemotherapy 64 (5–6): 215–223. https://doi.org/10.1159/000507423.
- Ciotti, M., C. Massimo, T. Alessandro, J. Wen-Can, W. Cheng-Bin, and B. Sergio. 2020. “The COVID-19 Pandemic.” Critical Reviews in Clinical Laboratory Sciences 57 (6): 365–388.
- Coffee, M. P., Garnett G. P., Mlilo, M., Voeten, H.A., Chandiwana, S. and Gregson, S. 2005. “Patterns of Movement and Risk of HIV Infection in Rural Zimbabwe.” The Journal of Infectious Diseases 191 (Supplement_1): S159–S167.
- Cohen, M. S. 1998. “Sexually Transmitted Diseases Enhance HIV Transmission: No Longer a Hypothesis.” Lancet 351 (Suppl 3): 5–7. https://doi.org/10.1016/s0140-6736(98)90002-2.
- Coleman, N., X. Gao, J. DeLeon, and A. Mostafavi. 2022. “Human Activity and Mobility Data Reveal Disparities in Exposure Risk Reduction Indicators Among Socially Vulnerable Populations During COVID-19 for Five US Metropolitan Cities.” Scientific Reports 12 (1): 15814. https://doi.org/10.1038/s41598-022-18857-7.
- Coltart, C. E., B. Lindsey, I. Ghinai, A. M. Johnson, and D. L. Heymann. 2017. “The Ebola Outbreak, 2013-2016: Old Lessons for New Epidemics.” Philosophical Transactions of the Royal Society of London Series B, Biological Sciences 372 (1721). https://doi.org/10.1098/rstb.2016.0297.
- Cox, F. E. 2010. “History of the Discovery of the Malaria Parasites and Their Vectors.” Parasites & Vectors 3 (1): 1–9.
- Currie, G., T. Jain, and L. Aston. 2021. “Evidence of a Post-COVID Change in Travel Behaviour – Self-Reported Expectations of Commuting in Melbourne.” Transportation Research Part A: Policy and Practice 153:218–234. https://doi.org/10.1016/j.tra.2021.09.009.
- Das, A. M., M. W. Hetzel, J. O. Yukich, L. Stuck, B. S. Fakih, A. H. Al-Mafazy, A. Ali, and N. Chitnis. 2022. “The Impact of Reactive Case Detection on Malaria Transmission in Zanzibar in the Presence of Human Mobility.” Epidemics 41:100639. https://doi.org/10.1016/j.epidem.2022.100639.
- De Cock, K. M., H. W. Jaffe, and J. W. Curran. 2012. “The Evolving Epidemiology of HIV/AIDS.” AIDS 26 (10): 1205–1213. https://doi.org/10.1097/QAD.0b013e328354622a.
- Delany-Moretlwe, S., B. Bello, P. Kinross, M. Oliff, M. Chersich, I. Kleinschmidt, and H. Rees. 2014. “HIV Prevalence and Risk in Long-Distance Truck Drivers in South Africa: A National Cross-Sectional Survey.” International Journal of STD & AIDS 25 (6): 428–438. https://doi.org/10.1177/0956462413512803.
- Desgrees-du-Lou, A., J. Pannetier, A. Ravalihasy, M. Le Guen, A. Gosselin, H. Panjo, N. Bajos, et al. 2016. “Is Hardship During Migration a Determinant of HIV Infection? Results from the ANRS PARCOURS Study of Sub-Saharan African Migrants in France.” AIDS 30 (4): 645–656. https://doi.org/10.1097/QAD.0000000000000957.
- Desjardins, M. R., I. Casas, A. M. Victoria, D. Carbonell, D. M. Davalos, and E. M. Delmelle. 2020. “Knowledge, attitudes, and practices regarding dengue, chikungunya, and Zika in Cali, Colombia.” Health & Place 63:102339. https://doi.org/10.1016/j.healthplace.2020.102339.
- Dianat, A., J. Hawkins, and K. N. Habib. 2022. “Assessing the Impacts of COVID-19 on Activity-Travel Scheduling: A Survey in the Greater Toronto Area.” Transportation Research Part A: Policy and Practice 162:296–314. https://doi.org/10.1016/j.tra.2022.06.008.
- Dick, G. W., Kitchen S. F, and Haddow A. J. 1952. “Zika Virus (I). Isolations and Serological Specificity.” Transactions of the Royal Society of Tropical Medicine and Hygiene 46 (5): 509–520.
- Dillman, D. A., and Bowker D. K. 2001. “The Web Questionnaire Challenge to Survey Methodolog.” Online Social Sciences 7:53–71. https://doi.org/10.1186/1475-2875-12-397.
- Dingil, A. E., and D. Esztergár-Kiss. 2021. “The Influence of the COVID-19 Pandemic on Mobility Patterns: The First Wave’s Results.” Transportation Letters 13 (5–6): 434–446. https://doi.org/10.1080/19427867.2021.1901011.
- Djiomba Njankou, S. D., and F. Nyabadza. 2022. “Modelling the Potential Influence of Human Migration and Two Strains on Ebola Virus Disease Dynamics.” Infectious Disease Models 7 (4): 645–659. https://doi.org/10.1016/j.idm.2022.10.002.
- Dobbie, L. J., T. J. Hydes, U. Alam, A. Tahrani, and D. J. Cuthbertson. 2022. “The Impact of the COVID-19 Pandemic on Mobility Trends and the Associated Rise in Population-Level Physical Inactivity: Insights from International Mobile Phone and National Survey Data.” Frontiers in Sports and Active Living 4:773742. https://doi.org/10.3389/fspor.2022.773742.
- Docquier, F., N. Golenvaux, S. Nijssen, P. Schaus, and F. Stips. 2022. “Cross-Border Mobility Responses to COVID-19 in Europe: New Evidence from Facebook Data.” Globalization and Health 18 (1): 41. https://doi.org/10.1186/s12992-022-00832-6.
- Duncan, C. J., and S. Scott. 2005. “What Caused the Black Death?.” Postgraduate Medical Journal 81 (955): 315–320. https://doi.org/10.1136/pgmj.2004.024075.
- Duong, V., L. Lambrechts, R. E. Paul, S. Ly, R. S. Lay, K. C. Long, R. Huy, et al. 2015. “Asymptomatic humans transmit dengue virus to mosquitoes.” Proceedings of the National Academy of Sciences of the United States of America 112 (47): 14688–14693. https://doi.org/10.1073/pnas.1508114112.
- Ehsani, J. P., J. P. Michael, M. L. Duren, Y. Mui, and K. M. P. Porter. 2021. “Mobility Patterns Before, During, and Anticipated After the COVID-19 Pandemic: An Opportunity to Nurture Bicycling.” American Journal of Preventive Medicine 60 (6): e277–e279. https://doi.org/10.1016/j.amepre.2021.01.011.
- Ellegaard, O., and J. A. Wallin. 2015. “The Bibliometric Analysis of Scholarly Production: How Great is the Impact?” Scientometrics 105 (3): 1809–1831. https://doi.org/10.1007/s11192-015-1645-z.
- Eum, Y., and E. H. Yoo. 2022. “Using GPS-Enabled Mobile Phones to Evaluate the Associations Between Human Mobility Changes and the Onset of Influenza Illness.” Spatial and Spatio-Temporal Epidemiology 40:100458. https://doi.org/10.1016/j.sste.2021.100458.
- Falcon-Lezama, J. A., R. Santos-Luna, S. Roman-Perez, R. A. Martinez-Vega, M. A. Herrera-Valdez, A. F. Kuri-Morales, B. Adams, et al. 2017. “Analysis of spatial mobility in subjects from a Dengue endemic urban locality in Morelos State, Mexico.” PloS One 12 (2): e0172313. https://doi.org/10.1371/journal.pone.0172313.
- Fallah, M., S. Lavalah, T. Gbelia, M. Zondo, M. Kromah, L. Tantum, G. Nallo, et al. 2022. “Contextualizing mobility during the Ebola epidemic in Liberia.” PLoS Neglected Tropical Diseases 16 (4): e0010370. https://doi.org/10.1371/journal.pntd.0010370.
- Ferreira, S., M. Amorim, A. Lobo, M. Kern, N. Fanderl, and A. Couto. 2022. “Travel Mode Preferences Among German Commuters Over the Course of COVID-19 Pandemic.” Transport Policy 126: 55–64. https://doi.org/10.1016/j.tranpol.2022.07.011.
- Fonner, V. A., D. Geurkink, F. Chiwanga, I. Amiri, and S. Likindikoki. 2021. “Long-Distance Travel for HIV-Related Care-Burden or Choice?: A Mixed Methods Study in Tanzania.” AIDS and Behavior 25 (7): 2071–2083. https://doi.org/10.1007/s10461-020-03136-1.
- Foxwell, A. R., L. Roberts, K. Lokuge, and P. M. Kelly. 2011. “Transmission of influenza on international flights, may 2009.” Emerging Infectious Diseases 17 (7): 1188–1194. https://doi.org/10.3201/eid1707.101135.
- Frew, G., E. McGeorge, S. Grant, and G. de Wildt. 2017. “Hepatitis B: A Cross-Sectional Survey of Knowledge, Attitudes and Practices Amongst Backpackers in Thailand.” Travel Medicine and Infectious Disease 15:57–62. https://doi.org/10.1016/j.tmaid.2016.11.008.
- Gao, S., J. Rao, Y. Kang, Y. Liang, J. Kruse, D. Doepfer, A. K. Sethi, J. F. M. Reyes, J. Patz, and B. S. Yandell 2020. “Association of Mobile Phone Location Data Indications of Travel and Stay-At-Home Mandates with COVID-19 Infection Rates in the US.” JAMA Network 3 (9): e2020485.
- Garattini, C., J. Raffle, D. N. Aisyah, F. Sartain, and Z. Kozlakidis. 2019. “Big Data Analytics, Infectious Diseases and Associated Ethical Impacts.” Philosophy & Technology 32 (1): 69–85. https://doi.org/10.1007/s13347-017-0278-y.
- Garcia, P. J., B. Fazio, A. M. Bayer, A. G. Lizarraga, M. Chiappe, S. La Rosa, M. Lazo, L. Lopez, M. Valderrama, and C. P. Carcamo. 2017. “Sexual Health Knowledge and Practices and STI/HIV Prevalence Among Long-Distance Truck Drivers in Peru.” SAGE Open Med 5:2050312117746308. https://doi.org/10.1177/2050312117746308.
- Gareau, E., and K. P. Phillips. 2022. “Sexual Behaviors at Home and Abroad: An Online Survey of Canadian Young Adult Travelers.” BMC Public Health 22 (1): 967. https://doi.org/10.1186/s12889-022-13383-7.
- Gauvin, L., P. Bajardi, E. Pepe, B. Lake, F. Privitera, and M. Tizzoni. 2021. “Socio-Economic Determinants of Mobility Responses During the First Wave of COVID-19 in Italy: From Provinces to Neighbourhoods.” Journal of the Royal Society Interface 18 (181): 20210092. https://doi.org/10.1098/rsif.2021.0092.
- Giordano, G., F. Blanchini, R. Bruno, P. Colaneri, A. Di Filippo, A. Di Matteo, and M. Colaneri. 2020. “Modelling the COVID-19 Epidemic and Implementation of Population-Wide Interventions in Italy.” Nature Medicine 26 (6): 855–860. https://doi.org/10.1038/s41591-020-0883-7.
- Glover, R. E., M. C. I. van Schalkwyk, E. A. Akl, E. Kristjannson, T. Lotfi, J. Petkovic, M. P. Petticrew, K. Pottie, P. Tugwell, and V. Welch. 2020. “A Framework for Identifying and Mitigating the Equity Harms of COVID-19 Policy Interventions.” Journal of Clinical Epidemiology 128:35–48. https://doi.org/10.1016/j.jclinepi.2020.06.004.
- Gomes, M. F. C., C. T. Codeco, L. S. Bastos, and R. M. Lana. 2020. “Measuring the Contribution of Human Mobility to Malaria Persistence.” Malaria journal 19 (1): 404. https://doi.org/10.1186/s12936-020-03474-4.
- Grassly, N. C., and C. Fraser. 2008. “Mathematical Models of Infectious Disease Transmission.” Nature Reviews Microbiology 6 (6): 477–487. https://doi.org/10.1038/nrmicro1845.
- Greenwood, B., and M. Theonest. 2002. “Malaria in 2002.” Nature 415 (6872): 670.
- Guan, G., Y. Dery, M. Yechezkel, I. Ben-Gal, D. Yamin, and M. L. Brandeau. 2021. “Early Detection of COVID-19 Outbreaks Using Human Mobility Data.” PloS One 16 (7): e0253865. https://doi.org/10.1371/journal.pone.0253865.
- Guerra, C. A., D. T. Citron, G. A. Garcia, and D. L. Smith. 2019. “Characterising Malaria Connectivity Using Malaria Indicator Survey Data.” Malaria Journal 18 (1): 440. https://doi.org/10.1186/s12936-019-3078-2.
- Guo, F., A. R. Norton, E. L. Fuchs, J. M. Hirth, M. A. Garcia-Blanco, and A. B. Berenson. 2017. “Provider-patient communication about Zika during prenatal visits.” Preventive Medicine Reports 7:26–29. https://doi.org/10.1016/j.pmedr.2017.05.003.
- Hanley, B. P., and B. Birthe. 2010. “Aerosol Influenza Transmission Risk Contours: A Study of Humid Tropics versus Winter Temperate Zone.” Virology Journal 7 (1): 1–18.
- Harrington, W. N., C. M. Kackos, and R. J. Webby. 2021. “The evolution and future of influenza pandemic preparedness.” Experimental & Molecular Medicine 53 (5): 737–749. https://doi.org/10.1038/s12276-021-00603-0.
- Hasselwander, M., T. Tamagusko, J. F. Bigotte, A. Ferreira, A. Mejia, and E. J. S. Ferranti. 2021. “Building Back Better: The COVID-19 Pandemic and Transport Policy Implications for a Developing Megacity.” Sustainable Cities and Society 69:102864. https://doi.org/10.1016/j.scs.2021.102864.
- Hast, M., S. Mharakurwa, T. M. Shields, J. Lubinda, K. Searle, L. Gwanzura, S. Munyati, and W. J. Moss. 2022. “Characterizing Human Movement Patterns Using GPS Data Loggers in an Area of Persistent Malaria in Zimbabwe Along the Mozambique Border.” BMC Infectious Diseases 22 (1): 942. https://doi.org/10.1186/s12879-022-07903-4.
- Hast, M., K. M. Searle, M. Chaponda, J. Lupiya, J. Lubinda, J. Sikalima, T. Kobayashi, et al. 2019. “The Use of GPS Data Loggers to Describe the Impact of Spatio-Temporal Movement Patterns on Malaria Control in a High-Transmission Area of Northern Zambia.” International Journal of Health Geographics 18 (1): 19. https://doi.org/10.1186/s12942-019-0183-y.
- Heidrich, P., Y. Jayathunga, W. Bock, and T. Götz. 2021. “Prediction of Dengue Cases Based on Human Mobility and Seasonality—An Example for the City of Jakarta.” Mathematical Methods in the Applied Sciences 44 (17): 13633–13658. https://doi.org/10.1002/mma.7648.
- Her, P. H., S. Saeed, K. Hoan Tram, and S. R. Bhatnagar. 2022. “Novel Mobility Index Tracks COVID-19 Transmission Following Stay-At-Home Orders.” Scientific Reports 12 (1): 7654. https://doi.org/10.1038/s41598-022-10941-2.
- Hill, R. 1998. “What Sample Size is “Enough” in Internet Survey Research.” Interpersonal Computing and Technology: An Electronic Journal for the 21st Century 6 (3–4): 1–12.
- Hlaing, T., K. T. Wai, T. Oo, N. Sint, T. Min, S. Myar, K. N. Lon, et al. 2015. “Mobility Dynamics of Migrant Workers and Their Socio-Behavioral Parameters Related to Malaria in Tier II, Artemisinin Resistance Containment Zone, Myanmar.” BMC Public Health 15:886. https://doi.org/10.1186/s12889-015-2241-0.
- Holder-Dixon, A. R., O. R. Adams, T. L. Cobb, A. J. Goldberg, R. A. Fikslin, M. A. Reinka, A. N. Gesselman, and D. M. Price. 2022. “Medical Avoidance Among Marginalized Groups: The Impact of the COVID-19 Pandemic.” Journal of Behavioral Medicine 45 (5): 760–770. https://doi.org/10.1007/s10865-022-00332-3.
- Hu, T., S. Wang, B. She, M. Zhang, X. Huang, Y. Cui, J. Khuri, et al. 2021. “Human Mobility Data in the COVID-19 Pandemic: Characteristics, Applications, and Challenges.” International Journal of Digital Earth 14 (9): 1126–1147. https://doi.org/10.1080/17538947.2021.1952324.
- Ikeda, J. M., J. R. Schaffer, M. L. Sac Ixcot, K. Page, and N. Hearst. 2014. “Risk of Sexually Transmitted Infections Among Mayan Women in Rural Guatemala Whose Partners are Migrant Workers.” AIDS and Behavior 18 (Suppl 1): S32–6. https://doi.org/10.1007/s10461-013-0592-z.
- Ilin, C., S. Annan-Phan, X. H. Tai, S. Mehra, S. Hsiang, and J. E. Blumenstock. 2021. “Public mobility data enables COVID-19 forecasting and management at local and global scales.” Scientific Reports 11 (1): 13531. https://doi.org/10.1038/s41598-021-92892-8.
- Ivorra, B., M. R. Ferrandez, M. Vela-Perez, and A. M. Ramos. 2020. “Mathematical Modeling of the Spread of the Coronavirus Disease 2019 (COVID-19) Taking into Account the Undetected Infections. The Case of China.” Communications in Nonlinear Science & Numerical Simulation 88:105303. https://doi.org/10.1016/j.cnsns.2020.105303.
- Jacob, S. T., I. Crozier, W. A. Fischer 2nd, A. Hewlett, C. S. Kraft, M. A. Vega, M. J. Soka, et al. 2020. “Ebola virus disease.” Nature Reviews Disease Primers 6 (1): 13. https://doi.org/10.1038/s41572-020-0147-3.
- Jing, F., L. Zhenlong, S. Qiao, J. Zhang, B. Olatosi, and L. Xiaoming. 2023. “Using geospatial social media data for infectious disease studies: a systematic review.” International Journal of Digital Earth 16 (1): 130–157. https://doi.org/10.1080/17538947.2022.2161652.
- Johnson, N. P., and M. Juergen. 2002. “Updating the Accounts: Global Mortality of the 1918-1920 ”Spanish” Influenza Pandemic.” Bulletin of the History of Medicine 76:105–115. https://doi.org/10.1186/1471-2458-11-357.
- Khamsiriwatchara, A., W. Piyaporn, T. Julie, E. James, S. Wichai, C. Delacollette, and J. Kaewkungwal. 2011. “Respondent-Driven Sampling on the ThailandCambodia Border. I. Can Malaria Cases Be Contained in Mobile Migrant Workers?” Malaria Journal 10 (1): 1–11.
- Kharel, M., J. L. Sakamoto, R. R. Carandang, S. Ulambayar, A. Shibanuma, E. Yarotskaya, M. Basargina, and M. Jimba. 2022. “Impact of COVID-19 Pandemic Lockdown on Movement Behaviours of Children and Adolescents: A Systematic Review.” BMJ Global Health 7 (1). https://doi.org/10.1136/bmjgh-2021-007190.
- Kiang, M. V., M. Santillana, J. T. Chen, J. P. Onnela, N. Krieger, K. Engo-Monsen, N. Ekapirat, et al. 2021. “Incorporating Human Mobility Data Improves Forecasts of Dengue Fever in Thailand.” Scientific Reports 11 (1): 923. https://doi.org/10.1038/s41598-020-79438-0.
- Kowdley, K. V., C. C. Wang, S. Welch, H. Roberts, and C. L. Brosgart. 2012. “Prevalence of Chronic Hepatitis B Among Foreign-Born Persons Living in the United States by Country of Origin.” Hepatology 56 (2): 422–433. https://doi.org/10.1002/hep.24804.
- Kraemer, M. U. G., D. Bisanzio, R. C. Reiner, R. Zakar, J. B. Hawkins, C. C. Freifeld, D. L. Smith, S. I. Hay, J. S. Brownstein, and T. A. Perkins. 2018. “Inferences About Spatiotemporal Variation in Dengue Virus Transmission are Sensitive to Assumptions About Human Mobility: A Case Study Using Geolocated Tweets from Lahore, Pakistan.” European Physical Journal 7 (1): 16. https://doi.org/10.1140/epjds/s13688-018-0144-x.
- Kramer, A. M., J. T. Pulliam, L. W. Alexander, A. W. Park, P. Rohani, and J. M. Drake. 2016. “Spatial Spread of the West Africa Ebola Epidemic.” Royal Society Open Science 3 (8): 160294. https://doi.org/10.1098/rsos.160294.
- Kucharski, A. J., T. W. Russell, C. Diamond, Y. Liu, J. Edmunds, S. Funk, R. M. Eggo, andCovid-working group Centre for Mathematical Modelling of Infectious Diseases. 2020. “Early Dynamics of Transmission and Control of COVID-19: A Mathematical Modelling Study.” The Lancet Infectious Diseases 20 (5): 553–558. https://doi.org/10.1016/S1473-3099(20)30144-4.
- Kulkarni, A. V., A. Brittany, S. Iffat, and J. W. Busse. 2009. “Comparisons of Citations in Web of Science, Scopus, and Google Scholar for Articles Published in General Medical Journals.” Journal of the American Medical Association 302 (10): 1092–1096.
- Kumanan, T., V. Sujanitha, and R. Nadarajah. 2019. “The Impact of Population Mobility on Dengue: An Experience from Northern Sri Lanka.” Sri Lankan Journal of Infectious Diseases 9 (2). https://doi.org/10.4038/sljid.v9i2.8252.
- Kuniya, T. 2020. “Prediction of the Epidemic Peak of Coronavirus Disease in Japan, 2020.” Journal of Clinical Medicine 9 (3). https://doi.org/10.3390/jcm9030789.
- Lamb, M. R., S. Kandula, and J. Shaman. 2021. “Differential COVID-19 Case Positivity in New York City Neighborhoods: Socioeconomic Factors and Mobility.” Influenza and Other Respiratory Viruses 15 (2): 209–217. https://doi.org/10.1111/irv.12816.
- Lau, H., V. Khosrawipour, P. Kocbach, A. Mikolajczyk, J. Schubert, J. Bania, and T. Khosrawipour. 2020. “The Positive Impact of Lockdown in Wuhan on Containing the COVID-19 Outbreak in China.” Journal of Travel Medicine 27:3. https://doi.org/10.1093/jtm/taaa037.
- Lee, H., S. Kim, M. Jeong, E. Choi, H. Ahn, and J. Lee. 2023. “Mathematical Modeling of COVID-19 Transmission and Intervention in South Korea: A Review of Literature.” Yonsei Medical Journal 64 (1): 1–10. https://doi.org/10.3349/ymj.2022.0471.
- Lee, M., J. Zhao, Q. Sun, Y. Pan, W. Zhou, C. Xiong, and L. Zhang. 2020. “Human Mobility Trends During the Early Stage of the COVID-19 Pandemic in the United States.” PloS One 15 (11): e0241468. https://doi.org/10.1371/journal.pone.0241468.
- Leggat, P. A., N. A. Zwar, B. J. Hudson, and Australia Travel Health Advisory Group. 2009. “Hepatitis B Risks and Immunisation Coverage Amongst Australians Travelling to Southeast Asia and East Asia.” Travel Medicine and Infectious Disease 7 (6): 344–349. https://doi.org/10.1016/j.tmaid.2009.03.008.
- Levin, R., D. L. Chao, E. A. Wenger, and J. L. Proctor. 2021. “Insights into Population Behavior During the COVID-19 Pandemic from Cell Phone Mobility Data and Manifold Learning.” Nature Computational Science 1 (9): 588–597. https://doi.org/10.1038/s43588-021-00125-9.
- Li, L. 2017. “Transmission Dynamics of Ebola Virus Disease with Human Mobility in Sierra Leone.” Chaos, Solitons & Fractals 104:575–579. https://doi.org/10.1016/j.chaos.2017.09.022.
- Li, Q., X. Guan, P. Wu, X. Wang, L. Zhou, Y. Tong, R. Ren, et al. 2020. “Early Transmission Dynamics in Wuhan, China, of Novel Coronavirus-Infected Pneumonia.” The New England Journal of Medicine 382 (13): 1199–1207. https://doi.org/10.1056/NEJMoa2001316.
- Li, Y., K. Stewart, K. T. Han, Z. Y. Han, P. P. Aung, Z. W. Thein, T. Htay, D. Chen, M. M. Nyunt, and C. V. Plowe. 2022. “Understanding Spatio-Temporal Human Mobility Patterns for Malaria Control Using a Multi-Agent Mobility Simulation Model.” Clinical Infectious Diseases. https://doi.org/10.1093/cid/ciac568.
- Li, Z., X. Huang, T. Hu, H. Ning, X. Ye, B. Huang, and X. Li. 2021. “ODT FLOW: Extracting, Analyzing, and Sharing Multi-Source Multi-Scale Human Mobility.” Public Library of Science One 16 (8): e0255259. https://doi.org/10.1371/journal.pone.0255259.
- Liverani, M., S. Teng, M. S. Le, and R. Coker. 2018. “Sharing Public Health Data and Information Across Borders: Lessons from Southeast Asia.” Globalization and Health 14 (1): 94. https://doi.org/10.1186/s12992-018-0415-0.
- Locker, D. 2000. “Response and Nonresponse Bias in Oral Health Surveys.” Journal of Public Health Dentistry 60 (2): 72–81. https://doi.org/10.1111/j.1752-7325.2000.tb03298.x.
- Lu, P. J., A. C. O’Halloran, W. W. Williams, and N. P. Nelson. 2018. “Hepatitis B Vaccination Coverage Among Adults aged >/= 18 years Traveling to a Country of High or Intermediate Endemicity, United States, 2015.” Vaccine 36 (18): 2471–2479. https://doi.org/10.1016/j.vaccine.2018.03.030.
- Luan, S., Q. Yang, Z. Jiang, and W. Wang. 2021. “Exploring the Impact of COVID-19 on Individual's Travel Mode Choice in China.” Transport Policy 106: 271–280. https://doi.org/10.1016/j.tranpol.2021.04.011.
- Luca, M., B. Lepri, E. Frias-Martinez, and A. Lutu. 2022. “Modeling International Mobility Using Roaming Cell Phone Traces During COVID-19 Pandemic.” European Physical Journal 11 (1): 22. https://doi.org/10.1140/epjds/s13688-022-00335-9.
- Mantell, J. E., E. A. Kelvin, X. Sun, J. Zhou, T. M. Exner, S. Hoffman, F. Zhou, T. G. Sandfort, and C. S. Leu. 2011. “HIV/STI Risk by Migrant Status Among Workers in an Urban High-End Entertainment Centre in Eastern China.” Health Education Research 26 (2): 283–295. https://doi.org/10.1093/her/cyr002.
- Marsh, C. K., V. Sheppeard, S. Tobin, R. Gilmour, and R. M. Andrews. 2022. “Drivers of the Summer Influenza Epidemic in New South Wales, 2018-19.” The Medical Journal of Australia 216 (1): 33–38. https://doi.org/10.5694/mja2.51266.
- Marshall, J. M., M. Toure, A. L. Ouedraogo, M. Ndhlovu, S. S. Kiware, A. Rezai, E. Nkhama, et al. 2016. “Key Traveller Groups of Relevance to Spatial Malaria Transmission: A Survey of Movement Patterns in Four Sub-Saharan African Countries.” Malaria Journal 15:200. https://doi.org/10.1186/s12936-016-1252-3.
- Marshall, J. M., S. L. Wu, C. Hm Sanchez, S. S. Kiware, M. Ndhlovu, A. L. Ouedraogo, M. B. Toure, H. J. Sturrock, A. C. Ghani, and N. M. Ferguson. 2018. “Mathematical Models of Human Mobility of Relevance to Malaria Transmission in Africa.” Scientific Reports 8 (1): 7713. https://doi.org/10.1038/s41598-018-26023-1.
- Martins, J. F., C. Marques, B. Nieto-Andrade, J. Kelley, D. Patel, D. Nace, C. Herman, et al. 2020. “Malaria Risk and Prevention in Asian Migrants to Angola.” The American Journal of Tropical Medicine and Hygiene 103 (5): 1918–1926. https://doi.org/10.4269/ajtmh.20-0706.
- Masuet-Aumatell, C., S. Toovey, and J. N. Zuckerman. 2013. “Prevention of influenza among travellers attending at a UK travel clinic: beliefs and perceptions. A cross-sectional study.” Influenza Other Respir Viruses 7 (4): 574–583. https://doi.org/10.1111/irv.12010.
- Mejía-Dorantes, L., L. Montero, and J. Barceló. 2021. “Mobility Trends Before and After the Pandemic Outbreak: Analyzing the Metropolitan Area of Barcelona Through the Lens of Equality and Sustainability.” Sustainability 13 (14). https://doi.org/10.3390/su13147908.
- Miller, H. B., R. B. Gose, M. T. Nagata, R. H. Sciulli, and A. C. Whelen. 2012. “Pacific region influenza surveillance for oseltamivir resistance.” Journal of Clinical Virology 54 (1): 73–75. https://doi.org/10.1016/j.jcv.2012.01.007.
- Moher, D., A. Liberati, J. Tetzlaff, D. G. Altman, and P. Group. 2009. “Preferred Reporting Items for Systematic Reviews and Meta-Analyses: The PRISMA Statement.” Public Library of Science Medicine 6 (7): e1000097. https://doi.org/10.1371/journal.pmed.1000097.
- Nielsen, U. S., E. Petersen, and C. S. Larsen. 2009. “Hepatitis B Immunization Coverage and Risk Behaviour Among Danish Travellers: Are Immunization Strategies Based on Single Journey Itineraries Rational?” The Journal of Infection 59 (5): 353–359. https://doi.org/10.1016/j.jinf.2009.08.018.
- Nielsen, U. S., R. W. Thomsen, S. Cowan, C. S. Larsen, and E. Petersen. 2012. “Predictors of Travel-Related Hepatitis a and B Among Native Adult Danes: A Nationwide Case-Control Study.” The Journal of Infection 64 (4): 399–408. https://doi.org/10.1016/j.jinf.2011.12.013.
- Nikiforiadis, A., L. Mitropoulos, P. Kopelias, S. Basbas, N. Stamatiadis, and S. Kroustali. 2022. “Exploring Mobility Pattern Changes Between Before, During and After COVID-19 Lockdown Periods for Young Adults.” Cities 125:103662. https://doi.org/10.1016/j.cities.2022.103662.
- Norris, A. H., Y. Loewenberg Weisband, M. Wiles, and J. R. Ickovics. 2017. “Prevalence of Sexually Transmitted Infections Among Tanzanian Migrants: A Cross-Sectional Study.” International Journal of STD & AIDS 28 (10): 991–1000. https://doi.org/10.1177/0956462416685486.
- Ossom Williamson, P., and C. I. J. Minter. 2019. “Exploring PubMed as a Reliable Resource for Scholarly Communications Services.” Journal of the Medical Library Association 107 (1): 16–29. https://doi.org/10.5195/jmla.2019.433.
- Panda, P. K., J. Gupta, S. R. Chowdhury, R. Kumar, A. K. Meena, P. Madaan, I. K. Sharawat, and S. Gulati. 2021. “Psychological and Behavioral Impact of Lockdown and Quarantine Measures for COVID-19 Pandemic on Children, Adolescents and Caregivers: A Systematic Review and Meta-Analysis.” Journal of Tropical Pediatrics 67 (1). https://doi.org/10.1093/tropej/fmaa122.
- Pannetier, J., A. Ravalihasy, N. Lydie, F. Lert, A. D. du Loû, and Desgrees du Lou, and group Parcours study. 2018. “Prevalence and Circumstances of Forced Sex and Post-Migration HIV Acquisition in Sub-Saharan African Migrant Women in France: An Analysis of the ANRS-PARCOURS Retrospective Population-Based Study.” The Lancet Public Health 3 (1): e16–e23. https://doi.org/10.1016/S2468-2667(17)30211-6.
- Parker, M. E., L. Meiqing, M. Amine Bouzaghrane, H. Obeid, D. Hayes, K. Trapenberg Frick, D. A. Rodríguez, R. Sengupta, J. Walker, and D. G. Chatman. 2021. “Public Transit Use in the United States in the Era of COVID-19: Transit riders’ Travel Behavior in the COVID-19 Impact and Recovery Period.” Transport Policy 111: 53–62. https://doi.org/10.1016/j.tranpol.2021.07.005.
- Patel, M., A. Dennis, C. Flutter, and Z. Khan. 2010. “Pandemic (H1N1) 2009 influenza.” British Journal of Anaesthesia 104 (2): 128–142.
- Paul, T., A. B. S. Ornob, R. Chakraborty, and N. Anwari. 2021. “Assessment of COVID-19 Induced Travel Pattern Changes in Dhaka City.” Case Studies on Transport Policy 9 (4): 1943–1955. https://doi.org/10.1016/j.cstp.2021.11.003.
- Peak, C. M., A. Wesolowski, E. Zu Erbach-Schoenberg, A. J. Tatem, E. Wetter, X. Lu, D. Power, et al. 2018. “Population Mobility Reductions Associated with Travel Restrictions During the Ebola Epidemic in Sierra Leone: Use of Mobile Phone Data.” International Journal of Epidemiology 47 (5): 1562–1570. https://doi.org/10.1093/ije/dyy095.
- Pedersini, R., C. Marano, L. De Moerlooze, L. Chen, and J. Vietri. 2016. “HAV & HBV Vaccination Among Travellers Participating in the National Health and Wellness Survey in Five European Countries.” Travel Medicine and Infectious Disease 14 (3): 221–232. https://doi.org/10.1016/j.tmaid.2016.03.008.
- Perrotta, D., E. Frias-Martinez, Y. Piontti, A. Pastore, Q. Zhang, M. Luengo-Oroz, D. Paolotti, M. Tizzoni, and A. Vespignani. 2022. “Comparing Sources of Mobility for Modelling the Epidemic Spread of Zika Virus in Colombia.” Public Library of Science Neglected Tropical Diseases 16 (7): e0010565. https://doi.org/10.1371/journal.pntd.0010565.
- Pindolia, D. K., Garcia A. J., Huang Z., Smith D. L., Alegana V. A., Noor A. M., Snow R. W., and Tatem A. J. 2013. “The Demographics of Human and Malaria Movement and Migration Patterns in East Africa.” Malaria Journal 12 (1): 1–12.
- Poletto, C., M. F. Gomes, A. Pastore y Piontti, L. Rossi, L. Bioglio, D. L. Chao, I. M. Longini Jr., M. E. Halloran, V. Colizza, and A. Vespignani. 2014. “Assessing the Impact of Travel Restrictions on International Spread of the 2014 West African Ebola Epidemic.” Eurosurveillance 19 (42). https://doi.org/10.1186/1475-2875-12-397.
- Pongvongsa, T., D. Nonaka, M. Iwagami, P. Soundala, P. Khattignavong, P. Xangsayarath, F. Nishimoto, et al. 2019. “Malaria Among Foreign Migrant Workers in Savannakhet Province, Lao People’s Democratic Republic.” Tropical Medicine and Health 47:10. https://doi.org/10.1186/s41182-019-0140-2.
- Prue, C. E., P. N. Williams, H. A. Joseph, M. Johnson, A. E. Wojno, B. A. Zulkiewicz, J. Macom, J. P. Alexander, S. E. Ray, and B. G. Southwell. 2019. “Factors That Mattered in Helping Travelers from Countries with Ebola Outbreaks Participate in Post-Arrival Monitoring During the 2014-2016 Ebola Epidemic.” Inquiry 56:46958019894795. https://doi.org/10.1177/0046958019894795.
- Pyke, A. T., Daly M. T., Cameron, J.N., Moore, P.R., Taylor, C.T., Hewitson, G.R., Humphreys, J.L. and Gair, R. 2014. “Imported Zika Virus Infection from the Cook Islands into Australia, 2014.” Public Library of Science Currents 6. https://doi.org/10.1371/currents.outbreaks.4635a54dbffba2156fb2fd76dc49f65e.
- Quam, M. B., and A. Wilder-Smith. 2016. “Estimated Global Exportations of Zika Virus Infections via Travellers from Brazil from 2014 to 2015.” Journal of Travel Medicine 23 (6). https://doi.org/10.1093/jtm/taw059.
- Rai, T., H. S. Lambert, A. B. Borquez, N. Saggurti, B. Mahapatra, and H. Ward. 2014. “Circular Labor Migration and HIV in India: Exploring Heterogeneity in Bridge Populations Connecting Areas of High and Low HIV Infection Prevalence.” The Journal of Infectious Diseases 210 (Suppl 2): S556–61. https://doi.org/10.1093/infdis/jiu432.
- Ramadona, A. L., Y. Tozan, L. Lazuardi, and J. Rocklov. 2019. “A Combination of Incidence Data and Mobility Proxies from Social Media Predicts the Intra-Urban Spread of Dengue in Yogyakarta, Indonesia.” Public Library of Science Neglected Tropical Diseases 13 (4): e0007298. https://doi.org/10.1371/journal.pntd.0007298.
- Ramesh, S., P. Mehrotra, B. Mahapatra, D. Ganju, K. Nagarajan, and N. Saggurti. 2014. “The Effect of Mobility on Sexual Risk Behaviour and HIV Infection: A Cross-Sectional Study of Men Who Have Sex with Men in Southern India.” Sexually Transmitted Infections 90 (6): 491–497. https://doi.org/10.1136/sextrans-2013-051350.
- Razavi, H. 2020. “Global Epidemiology of Viral Hepatitis.” Gastroenterology Clinics 49 (2): 179–189. https://doi.org/10.1016/j.gtc.2020.01.001.
- Rebaza, J. 2021. “A General Multipatch Model of Ebola Dynamics.” Nonautonomous Dynamical Systems 8 (1): 125–135. https://doi.org/10.1515/msds-2020-0129.
- Reed, E., J. Gupta, M. Biradavolu, and K. M. Blankenship. 2012. “Migration/Mobility and Risk Factors for HIV Among Female Sex Workers in Andhra Pradesh, India: Implications for HIV Prevention.” International Journal of STD & AIDS 23 (4): e7–e13. https://doi.org/10.1258/ijsa.2009.009421.
- Roman, M., K. Bhatta, M. Roman, and P. Gautam. 2021. “Socio-Economic Factors Influencing Travel Decision-Making of Poles and Nepalis During the COVID-19 Pandemic.” Sustainability 13 (20): 11468. https://doi.org/10.3390/su132011468.
- Roques, L., E. K. Klein, J. Papaix, A. Sar, and S. Soubeyrand. 2020. “Impact of Lockdown on the Epidemic Dynamics of COVID-19 in France.” Frontiers in Medicine (Lausanne) 7:274. https://doi.org/10.3389/fmed.2020.00274.
- Rowe, F., A. Calafiore, D. Arribas-Bel, K. Samardzhiev, and M. Fleischmann. 2023. “Urban Exodus? Understanding Human Mobility in Britain During the COVID-19 Pandemic Using Meta-Facebook Data.” Population, Space and Place 29 (1): e2637. https://doi.org/10.1002/psp.2637.
- Saravanakumar, S., A. Eswari, L. Rajendran, and M. Abukhaled. 2020. “A Mathematical Model of Risk Factors in HIV/AIDS Transmission Dynamics: Observational Study of Female Sexual Network in India.” Applied Mathematics and Information Sciences 14 (6): 967–976. https://doi.org/10.18576/amis/140603.
- Saunders-Hastings, P. R., and D. Krewski. 2016. “Reviewing the History of Pandemic Influenza: Understanding Patterns of Emergence and Transmission.” Pathogens 5 (4). https://doi.org/10.3390/pathogens5040066.
- Sayers, E. W., E. E. Bolton, J. R. Brister, K. Canese, J. Chan, D. C. Comeau, C. M. Farrell, et al. 2023. “Database Resources of the National Center for Biotechnology Information in 2023.” Nucleic Acids Research 51 (D1): D29–D38. https://doi.org/10.1093/nar/gkac1032.
- Schaber, K. L., V. A. Paz-Soldan, A. C. Morrison, W. H. D. Elson, A. L. Rothman, C. N. Mores, H. Astete-Vega, et al. 2019. “Dengue illness impacts daily human mobility patterns in Iquitos, Peru.” PLoS Neglected Tropical Diseases 13 (9): e0007756. https://doi.org/10.1371/journal.pntd.0007756.
- Schaber, K. L., T. A. Perkins, A. L. Lloyd, L. A. Waller, U. Kitron, V. A. Paz-Soldan, J. P. Elder, et al. 2021. “Disease-Driven Reduction in Human Mobility Influences Human-Mosquito Contacts and Dengue Transmission Dynamics.” PLoS Computational Biology 17 (1): e1008627. https://doi.org/10.1371/journal.pcbi.1008627.
- Schicker, R. S., N. Hiruy, B. Melak, W. Gelaye, B. Bezabih, R. Stephenson, A. E. Patterson, et al. 2015. “A Venue-Based Survey of Malaria, Anemia and Mobility Patterns Among Migrant Farm Workers in Amhara Region, Ethiopia.” Public Library of Science One 10 (11): e0143829. https://doi.org/10.1371/journal.pone.0143829.
- Schopf, T., B. Daniel, and M. Florian. 2022. “Lbl2Vec: An Embedding-Based Approach for Unsupervised Document Retrieval on Predefined Topics.” ArXiv Preprint. https://doi.org/10.48550/arXiv.2210.06023.
- Sharangpani, R., K. E. Boulton, E. Wells, and C. Kim. 2011. “Attitudes and Behaviors of International Air Travelers Toward Pandemic Influenza.” Journal of Travel Medicine 18 (3): 203–208. https://doi.org/10.1111/j.1708-8305.2011.00500.x.
- Shaw, G. M., and H. Eric. 2012. “HIV transmission.” Cold Spring Harbor Perspectives in Medicine 2 (11): a006965.
- Shellvarajah, M., C. Hatz, and P. Schlagenhauf. 2017. “Malaria Prevention Recommendations for Risk Groups Visiting Sub-Saharan Africa: A Survey of European Expert Opinion and International Recommendations.” Travel Medicine and Infectious Disease 19:49–55. https://doi.org/10.1016/j.tmaid.2017.09.002.
- Shepherd, H. E. R., F. S. Atherden, H. M. T. Chan, A. Loveridge, and A. J. Tatem. 2021. “Domestic and International Mobility Trends in the United Kingdom During the COVID-19 Pandemic: An Analysis of Facebook Data.” International Journal of Health Geographics 20 (1): 46. https://doi.org/10.1186/s12942-021-00299-5.
- Sievert, K., P. O’Neill, Y. Koh, J. H. Lee, A. Dev, and S. Le. 2018. “Barriers to Accessing Testing and Treatment for Chronic Hepatitis B in Afghan, Rohingyan, and South Sudanese Populations in Australia.” Journal of Immigrant & Minority Health 20 (1): 140–146. https://doi.org/10.1007/s10903-017-0546-z.
- Sigler, T., S. Mahmuda, A. Kimpton, J. Loginova, P. Wohland, E. Charles-Edwards, and J. Corcoran. 2021. “The Socio-Spatial Determinants of COVID-19 Diffusion: The Impact of Globalisation, Settlement Characteristics and Population.” Globalization and Health 17 (1). https://doi.org/10.1186/s12992-021-00707-2.
- Sinha, I., A. A. Sayeed, D. Uddin, A. Wesolowski, S. I. Zaman, M. A. Faiz, A. Ghose, et al. 2020. “Mapping the Travel Patterns of People with Malaria in Bangladesh.” BMC Medicine 18 (1): 45. https://doi.org/10.1186/s12916-020-1512-5.
- Smith, J. L., P. Ghimire, K. R. Rijal, A. Maglior, S. Hollis, R. Andrade-Pacheco, G. Das Thakur, et al. 2019. “Designing Malaria Surveillance Strategies for Mobile and Migrant Populations in Nepal: A Mixed-Methods Study.” Malaria Journal 18 (1): 158. https://doi.org/10.1186/s12936-019-2791-1.
- Smith, R. D. 2006. “Trade and Public Health: Facing the Challenges of Globalisation.” Journal of Epidemiology & Community Health 60 (8): 650–651.
- Sokal, A., E. D’Ortenzio, N. Houhou-Fidouh, S. Brichler, J. Dorchies, O. Cabras, I. Leparc-Goffart, Y. Yazdanpanah, and S. Matheron. 2016. “Zika Virus Infection: Report of the First Imported Cases in a Paris Travel Centre.” Journal of Travel Medicine 24 (1). https://doi.org/10.1093/jtm/taw066.
- Souza, R. C. S. N. P., R. M. Assunção, D. B. Neill, and W. Meira. 2019. “Detecting Spatial Clusters of Disease Infection Risk Using Sparsely Sampled Social Media Mobility Patterns.” In Proceedings of the 27th ACM SIGSPATIAL International Conference on Advances in Geographic Information Systems. https://doi.org/10.1186/1471-2458-11-357.
- Squiers, L., J. Herrington, B. Kelly, C. Bann, S. Becker-Dreps, L. Stamm, M. Johnson, and L. McCormack. 2018. “Zika Virus Prevention: U.S. Travelers’ Knowledge, Risk Perceptions, and Behavioral Intentions-A National Survey.” The American Journal of Tropical Medicine and Hygiene 98 (6): 1837–1847. https://doi.org/10.4269/ajtmh.17-0898.
- Swain, S. N., Saggurti, N., Battala, M., Verma, R.K. and Jain, A.K. 2011. “Experience of Violence and Adverse Reproductive Health Outcomes, HIV Risks Among Mobile Female Sex Workers in India.” BMC Public Health 11 (1): 1–10.
- Talapko, J., I. Skrlec, T. Alebic, M. Jukic, and A. Vcev. 2019. “Malaria: The Past and the Present.” Microorganisms 7 (6). https://doi.org/10.3390/microorganisms7060179.
- Taylor, M. R., K. E. Agho, G. J. Stevens, and B. Raphael. 2008. “Factors Influencing Psychological Distress During a Disease Epidemic: Data from Australia’s First Outbreak of Equine Influenza.” BMC Public Health 8:347. https://doi.org/10.1186/1471-2458-8-347.
- Teich, A., A. B. Lowenfels, L. Solomon, and G. P. Wormser. 2018. “Gender Disparities in Zika Virus Knowledge in a Potentially At-Risk Population from Suburban New York City.” Diagnostic Microbiology and Infectious Disease 92 (4): 315–318. https://doi.org/10.1016/j.diagmicrobio.2018.07.003.
- Texier, G., M. Vanessa, B. Meili, B. Jean-Paul, and R. Christophe. 2013. “Environmental determinant of malaria cases among travellers.” Malaria Journal 12 (1): 1–11.
- Tiittala, P., M. Ristola, K. Liitsola, J. Ollgren, P. Koponen, H. M. Surcel, E. Hiltunen-Back, I. Davidkin, and P. Kivela. 2018. “Missed Hepatitis B/C or Syphilis Diagnosis Among Kurdish, Russian, and Somali Origin Migrants in Finland: Linking a Population-Based Survey to the National Infectious Disease Register.” BMC Infectious Diseases 18 (1): 137. https://doi.org/10.1186/s12879-018-3041-9.
- Tsuzuki, A., V. T. Duoc, T. Sunahara, M. Suzuki, L. Hoang, H. Nguyen, Y. Yukiko, et al. 2014. “Possible Association Between Recent Migration and Hospitalisation for Dengue in an Urban Population: A Prospective Case-Control Study in Northern Vietnam.” Tropical Biomedicine 31 (4): 698–708.
- Vahedi, B., M. Karimzadeh, and H. Zoraghein. 2021. “Spatiotemporal Prediction of COVID-19 Cases Using Inter- and Intra-County Proxies of Human Interactions.” Nature Communications 12 (1): 6440. https://doi.org/10.1038/s41467-021-26742-6.
- Valdez, L. D., H. H. Aragao Rego, H. E. Stanley, and L. A. Braunstein. 2015. “Predicting the Extinction of Ebola Spreading in Liberia Due to Mitigation Strategies.” Scientific Reports 5:12172. https://doi.org/10.1038/srep12172.
- van de Pas, R., D. Kolie, A. Delamou, and W. Van Damme. 2019. “Health Workforce Development and Retention in Guinea: A Policy Analysis Post-Ebola.” Human Resources for Health 17 (1): 63. https://doi.org/10.1186/s12960-019-0400-6.
- Vanden Berghe, W., C. Nöstlinger, H. Hospers, and M. Laga. 2013. “International Mobility, Sexual Behaviour and HIV-Related Characteristics of Men Who Have Sex with Men Residing in Belgium.” BMC Public Health 13 (1): 1–9.
- Vannoni, M., M. McKee, J. C. Semenza, C. Bonell, and D. Stuckler. 2020. “Using Volunteered Geographic Information to Assess Mobility in the Early Phases of the COVID-19 Pandemic: A Cross-City Time Series Analysis of 41 Cities in 22 Countries from March 2nd to 26th 2020.” Globalization and Health 16 (1): 85. https://doi.org/10.1186/s12992-020-00598-9.
- Vignier, N., R. Dray Spira, J. Pannetier, A. Ravalihasy, A. Gosselin, F. Lert, N. Lydie, et al. 2018. “Refusal to Provide Healthcare to Sub-Saharan Migrants in France: A Comparison According to Their HIV and HBV Status.” European Journal of Public Health 28 (5): 904–910. https://doi.org/10.1093/eurpub/cky118.
- Vogel, N., C. Theisen, J. P. Leidig, J. Scripps, D. H. Graham, and G. Wolffe. 2015. “Mining Mobile Datasets to Enable the Fine-Grained Stochastic Simulation of Ebola Diffusion.” Procedia Computer Science 51: 765–774. https://doi.org/10.1016/j.procs.2015.05.197.
- Volkman, H. R., E. J. Walz, D. Wanduragala, E. Schiffman, A. Frosch, J. D. Alpern, P. F. Walker, et al. 2020. “Barriers to Malaria Prevention Among Immigrant Travelers in the United States Who Visit Friends and Relatives in Sub-Saharan Africa: A Cross-Sectional, Multi-Setting Survey of Knowledge, Attitudes, and Practices.” Public Library of Science One 15 (3): e0229565. https://doi.org/10.1371/journal.pone.0229565.
- von Wyl, V., R. D. Kouyos, S. Yerly, J. Boni, C. Shah, P. Burgisser, T. Klimkait, et al. 2011. “The Role of Migration and Domestic Transmission in the Spread of HIV-1 Non-B Subtypes in Switzerland.” The Journal of Infectious Diseases 204 (7): 1095–1103. https://doi.org/10.1093/infdis/jir491.
- Wai, K. T., K. Myat Phone, O. Tin, Z. PeThet, N. Myat Htut, T. Moe, and K. Thar Tun. 2014. “Spatial Distribution, Work Patterns, and Perception Towards Malaria Interventions Among Temporary Mobile/Migrant Workers in Artemisinin Resistance Containment Zone.” BMC Public Health 14 (1): 1–10.
- Wang, K.-W., J.-Q. Wu, H.-X. Zhao, Y.-Y. Li, R. Zhao, Y. Zhou, and H. L. Ji. 2013. “Unmarried Male Migrants and Sexual Risk Behavior: A Cross-Sectional Study in Shanghai, China.” BMC Public Health 13 (1): 1–10.
- Wang, S., I. Ka-Wai Lai, and J. Weng-Chou Wong. 2022. “An Exploratory Study of Pandemic-Restricted Travel—A New Form of Travel Pattern on the During-And Post-COVID-19 Era.” International Journal of Environmental Research and Public Health 19 (7): 4149. https://doi.org/10.3390/ijerph19074149.
- Wang, S., M. Zhang, H. Tao, F. Xiaokang, Z. Gao, B. Halloran, and Y. Liu. 2021. “A Bibliometric Analysis and Network Visualisation of Human Mobility Studies from 1990 to 2020: Emerging Trends and Future Research Directions.” Sustainability 13 (10). https://doi.org/10.3390/su13105372.
- Wei, S., X. Chen, G. Li, W. Zhou, W. Shi, and X. Wang. 2014. “Sexually Transmitted Infections Among migrants’ Wives Remaining in Rural Homes - a Pilot Study of the Remaining Women in Rural Wuhan, China.” Health Psychology and Behavioral Medicine 2 (1): 82–87. https://doi.org/10.1080/21642850.2013.872991.
- Wesolowski, A., C. O. Buckee, K. Engo-Monsen, and C. J. E. Metcalf. 2016. “Connecting Mobility to Infectious Diseases: The Promise and Limits of Mobile Phone Data.” The Journal of Infectious Diseases 214 (suppl_4): S414–S420. https://doi.org/10.1093/infdis/jiw273.
- Wesolowski, A., N. Eagle, A. J. Tatem, L. S. David, M. N. Abdisalan, W. S. Robert, and O. B. Caroline. 2012. “Quantifying the Impact of Human Mobility on Malaria.” Science 338 (6104): 267–270.
- Wesolowski, A., T. Qureshi, M. F. Boni, P. R. Sundsoy, M. A. Johansson, S. B. Rasheed, K. Engo-Monsen, and C. O. Buckee. 2015. “Impact of Human Mobility on the Emergence of Dengue Epidemics in Pakistan.” Proceedings of the National Academy of Sciences of the United States of America 112 (38): 11887–11892. https://doi.org/10.1073/pnas.1504964112.
- Widmar, N. J., S. R. Dominick, A. Ruple, and W. E. Tyner. 2017. “The Influence of Health Concern on Travel Plans with Focus on the Zika Virus in 2016.” Preventive Medicine Reports 6:162–170. https://doi.org/10.1016/j.pmedr.2017.02.023.
- Wilson, A. L., O. Courtenay, L. A. Kelly-Hope, T. W. Scott, W. Takken, S. J. Torr, and S. W. Lindsay. 2020. “The Importance of Vector Control for the Control and Elimination of Vector-Borne Diseases.” Public Library of Science Neglected Tropical Diseases 14 (1): e0007831. https://doi.org/10.1371/journal.pntd.0007831.
- Wu, Y. C., C. S. Chen, and Y. J. Chan. 2020. “The Outbreak of COVID-19: An Overview.” Journal of the Chinese Medical Association: JCMA 83 (3): 217–220. https://doi.org/10.1097/JCMA.0000000000000270.
- Xiao, Q., H. Liu, and B. Wu. 2020. “How Bachelorhood and Migration Increase the HIV Transmission Risk Through Commercial Sex in China?” AIDS and Behavior 24 (3): 791–801. https://doi.org/10.1007/s10461-019-02640-3.
- Yanni, E. A., N. Marano, P. Han, P. J. Edelson, S. Blumensaadt, M. Becker, S. Dwyer, et al. 2010. “Knowledge, Attitudes, and Practices of US Travelers to Asia Regarding Seasonal Influenza and H5N1 Avian Influenza Prevention Measures.” Journal of Travel Medicine 17 (6): 374–381. https://doi.org/10.1111/j.1708-8305.2010.00458.x.
- Yechezkel, M., A. Weiss, I. Rejwan, E. Shahmoon, S. Ben-Gal, and D. Yamin. 2021. “Human Mobility and Poverty as Key Drivers of COVID-19 Transmission and Control.” BMC Public Health 21 (1): 596. https://doi.org/10.1186/s12889-021-10561-x.
- Zeng, C., J. Zhang, Z. Li, X. Sun, X. Yang, B. Olatosi, S. Weissman, and X. Li. 2022. “Population Mobility and Aging Accelerate the Transmission of Coronavirus Disease 2019 in the Deep South: A County-Level Longitudinal Analysis.” Clinical Infectious Diseases 74 (Suppl_3): e1–e3. https://doi.org/10.1093/cid/ciac050.
- Zhang, C., C. Chen, W. Shen, F. Tang, H. Lei, Y. Xie, Z. Cao, et al. 2020. “Impact of Population Movement on the Spread of 2019-nCov in China.” Emerging Microbes & Infections 9 (1): 988–990. https://doi.org/10.1080/22221751.2020.1760143.
- Zhang, M., S. Wang, H. Tao, F. Xiaokang, X. Wang, H. Yaxin, B. Halloran, et al. 2022. “Human Mobility and COVID-19 Transmission: A Systematic Review and Future Directions.” Annals of GIS 28 (4): 501–514. https://doi.org/10.1080/19475683.2022.2041725.
- Zhang, Q., K. Sun, M. Chinazzi, A. Pastore y Piontti, N. E. Dean, D. P. Rojas, S. Merler, et al. 2017. “Spread of Zika Virus in the Americas.” Proceedings of the National Academy of Sciences of the United States of America 114 (22): E4334–E4343. https://doi.org/10.1073/pnas.1620161114.
- Zhang, X., A. P. Martinez-Donate, N. E. Simon, M. F. Hovell, M. G. Rangel, C. Magis-Rodriguez, and C. L. Sipan. 2017. “Risk Behaviours for HIV Infection Among Travelling Mexican Migrants: The Mexico-US Border as a Contextual Risk Factor.” Global Public Health 12 (1): 65–83. https://doi.org/10.1080/17441692.2016.1142591.
- Zheng, D., Q. Luo, and B. W. Ritchie. 2021. “Afraid to Travel After COVID-19? Self-Protection, Coping and Resilience Against Pandemic ‘Travel Fear.” Tourism Management 83. https://doi.org/10.1016/j.tourman.2020.104261.
- Zhu, G., T. Liu, J. Xiao, B. Zhang, T. Song, Y. Zhang, L. Lin, et al. 2019. “Effects of Human Mobility, Temperature and Mosquito Control on the Spatiotemporal Transmission of Dengue.” Science of the Total Environment 651 (Pt 1): 969–978. https://doi.org/10.1016/j.scitotenv.2018.09.182.
- Zhu, N., D. Zhang, W. Wang, X. Li, B. Yang, J. Song, X. Zhao, et al. 2020. “A Novel Coronavirus from Patients with Pneumonia in China, 2019.” The New England Journal of Medicine 382 (8): 727–733. https://doi.org/10.1056/NEJMoa2001017.
- Zuckerman, J. N., and B. Hoet. 2008. “Hepatitis B Immunisation in Travellers: Poor Risk Perception and Inadequate Protection.” Travel Medicine and Infectious Disease 6 (5): 315–320. https://doi.org/10.1016/j.tmaid.2008.05.001.