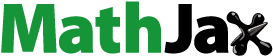
ABSTRACT
The uncertainties in microwave land surface emissivity (MLSE) measurements have long limited the use of spaceborne microwave radiometer data. As an emerging observation method, Global Navigation Satellite System Reflectometry (GNSS-R) has demonstrated great potential in several land and ocean applications. In this study, a method for obtaining daily MLSE dataset in the pan-tropical region from Cyclone GNSS (CYGNSS) observations is presented and evaluated. The CYGNSS observations are first aggregated into the Equal-Area-Scalable-Earth (EASE) 2.0 36 km grid by a combined weight function of distance, time, and signal-to-noise ratio variance. Then, the method employs a pixel-by-pixel regression algorithm to conduct the daily MLSE retrieval using reference emissivity derived from the Soil Moisture Active Passive (SMAP) brightness temperature. The CYGNSS MLSE shows good agreement with SMAP MLSE, delivering an overall root-mean-square error (RMSE) of 0.022 and 0.017 for horizontal and vertical polarization, respectively, during the training set spanning the whole year of 2018. Furthermore, on the test set from January 2019 to May 2019, the RMSE values amounted to 0.030 and 0.023 for horizontal and vertical polarization, respectively. Temperature records from the International Soil Moisture Network are employed to calculate the emissivity and for in-situ validation, which yield an RMSE of 0.034 and 0.026 for the two polarizations, respectively. The proposed algorithm provides an encouraging approach to obtain accurate daily MLSE dataset for microwave remote sensing. Compared to the SMAP MLSE, the CYGNSS MLSE has a remarkable improvement of 86% in temporal resolution, greatly complementing the existing microwave emissivity datasets.
1. Introduction
Land surface emissivity (LSE) is a critical quantity in the knowledge of the surface thermal radiation and is significant for studying land physical processes (Min, Lin, and Li Citation2009; Wu et al. Citation2022; Zhang et al. Citation2023). Thermal infrared and microwave are the most concerned bands in the study of LSE. In comparison with thermal infrared, microwaves are capable of penetrating clouds and are less affected by the atmosphere for their longer wavelength. However, the application of microwave radiometry for land surface parameter retrievals is quite challenging due to the complexity compared to the ocean surface. The land parameters affecting microwave land surface emissivity (MLSE) mainly include soil moisture, roughness, soil type, vegetation, and surface water.
MLSE cannot be directly measured but can be retrieved from emission measurements such as spaceborne radiometers (Ringerud, Kummerow, and Peters-Lidard Citation2015). So far, many studies have retrieved MLSE using the top of atmosphere (TOA) microwave brightness temperatures from satellite sensors such as Special Sensor Microwave Imagers (SSM/I) (Prigent, Rossow, and Matthews Citation1997), Advanced Microwave Sounding Unit (AMSU) (Karbou et al. Citation2005), and Advanced Microwave Scanning Radiometer-Earth Observing System (AMSR-E) (Moncet et al. Citation2011; Norouzi et al. Citation2011). Statistical modeling is a commonly used empirical approach which looks at the statistical relationship between emissivity and predictor variables from historical data (Tian et al. Citation2015; Turk, Haddad, and You Citation2014). One of the simplest statistical modeling methods involves establishing a regression relationship between brightness temperature and emissivity. In addition, physical modeling is also a basic approach to estimate emissivity at a small scale, which employs numerical models to calculate emissivity based on their physical relationship with other land surface variables such as soil moisture, soil texture, and vegetation properties (Ringerud et al. Citation2013; Weng, Yan, and Grody Citation2001).
The uncertainty in MLSE greatly limits the use of spaceborne microwave radiometer data. Therefore, it is necessary to improve the existing emissivity acquisition methods (Wu et al. Citation2022). In the past few years, reflected signals from Global Navigation Satellite System (GNSS) have been found to be a signal of opportunity to observe the earth surface, and this technology is called the Global Navigation Satellite System-Reflectometry (GNSS-R) (Jing et al. Citation2024). The GNSS-R receiver is equipped with a configuration of bistatic radar, offering a low-cost observation approach with no need to transmit signals. At present, about 130 available GNSS satellites along with their abundant signal resources have the potential to provide observations with high spatial and temporal resolution (Jin, Wang, and Dardanelli Citation2022). Furthermore, the GNSS-R receiver can also be carried on ground and airborne platforms, providing a way to meet needs in different scenarios. The main observation of the GNSS-R receiver is the delay-Doppler map (DDM), which is created by cross correlating the received signal with a locally generated replica for different path delays and Doppler shifts. GNSS-R data have been used in several land and ocean applications, such as soil moisture retrieval (Chew and Small Citation2020; Dong and Jin Citation2021; Yan et al. Citation2020), forest biomass estimation (Zribi et al. Citation2019), sea ice detection (Alonso-Arroyo, Zavorotny, and Camps Citation2017) and sea wind speed retrieval (Chen et al. Citation2022; Li et al. Citation2023; Ruf et al. Citation2016; Saïd et al. Citation2021).
Compared to existing MLSE measurements, GNSS-R derived MLSE is advantageous as it does not rely on knowledge of surface temperature. The surface reflectivity is the main derivation from DDM on land. The electromagnetic scattering modeling for GNSS-R on rough surfaces is typically based on the Kirchhoff approximation, and the surface roughness is commonly decomposed into three scales: topographic, intermediate, and microwave scales. A recent study has presented a comparison between three electromagnetic scattering models (Campbell et al. Citation2022). In theory, for a perfectly flat interface between two homogeneous media, the sum of reflectivity and emissivity obtained with the same system is 1. However, for the rough land surface, the impinging GNSS signal is scattered not only in the specular direction but also in other directions. The GNSS-R receiver primarily tracks signals in the near-specular direction, while passive microwave radiometry observes the upper hemisphere. The two would only yield the same results if the surface was completely flat and devoid of diffused scattering. Thus, it is inappropriate to assume that microwave emissivity is simply one minus the GNSS-R reflectivity. Given the unique advantages of GNSS-R technology, the connection between GNSS-R reflectivity and microwave emissivity is valuable to discuss.
This work proposes a pixel-by-pixel method to obtain daily MLSE in the pan-tropical region from CYGNSS observations. This work aims to provide a new approach to complement the existing microwave emissivity datasets. This paper is structured as follows: Section 2 introduces the data used in this study. Section 3 describes the proposed daily MLSE retrieval model. Section 4 presents the results, both in the pan-tropical region and over the in-situ sites, and section 5 summarizes the conclusions.
2. Data
2.1. CYGNSS data
CYGNSS was launched on 15 December 2016 by NASA, and comprises eight low Earth orbit satellites. The signals observed are the Global Positioning System (GPS) L1 (1.58 Ghz) coarse-acquisition codes and eight satellites produce 32 measurements per second (increased to 64 measurements per second after July 2019). CYGNSS can monitor variations in the pan-tropical region (within ±38° latitudes). The determination of spatial resolution is still unsolved at present, as it depends on the roughness of the surface in the specular and near-specular directions. For coherent reflected signals over a smooth surface, a current consensus is that, the size of the footprint is defined by the first Fresnel zone. Thus, during the period of one CYGNSS measurement, the theoretical smallest area of ground is ~7 × 0.5 km (turned to ~3.5 × 0.5 km after July 2019) (Chew and Small Citation2018).
In this work, CYGNSS Level1 (L1) v2.1 data from January 2018 to December 2018 are used for model training, while data from January 2019 to May 2019 are used for test (CYGNSS Citation2017). The following criteria are used to filleted the CYGNSS data: 1) the maximum of analog power greater than −147, 2) the antenna gain greater than 0 dB 3) the incidence angle (i.e. the angle between the transmitter to specular point ray and the surface normal) less than 40°, 4) the maximum of analog power at a delay bin of 7–10, 5) the quality flags (possible low quality and low confidence in the GPS equivalent isotopically radiated power) provided by CYGNSS, and 6) the water mark described in section 2.4. The above criteria are concluded from the previous CYGNSS-based studies (Dong and Jin Citation2021; Eroglu et al. Citation2019; Yang et al. Citation2022), which can increase the reliability of CYGNSS observations.
2.2. SMAP data
The Soil Moisture Active Passive (SMAP) mission was developed by NASA and incorporated radar and radiometers to perform the earth observation missions (Entekhabi, Njoku, et al. Citation2010). Unfortunately, the radar failed in 2015, leaving the only operation of radiometer. The SMAP instruments operate at L band (1.41 GHz) and provide a conically scanning antenna beam with an incidence angle of 40°. In this work, gridded brightness temperature data at horizontal and vertical polarizations (H and V) from the SMAP L1C v005 product (Chan, Njoku, and Colliander Citation2020) and surface temperature data from the SMAP L3 v007 product (O’Neill et al. Citation2020) are used, which are stored in the form of Equal-Area-Scalable-Earth (EASE) 2.0 36 km grid. The brightness temperature data have been corrected for atmospheric effects, Faraday rotation, and low-level Radio Frequency Interference (RFI) effects. Products of descending half-orbit pass are employed here, at which time the soil, vegetation, and air are in approximate thermal equilibrium. This descending half-orbit data can help simplify the physical model and improve the accuracy of derived soil moisture estimations (Entekhabi, Njoku, et al. Citation2010). The surface temperature is derived from the Goddard Earth Observing System Model, Version 5 (GEOS-5), forward processing (FP) system and has been modified by the Choudhury two-layer approach (Entekhabi et al. Citation2010).
2.3. ISMN data
The International Soil Moisture Network (ISMN) was set up in 2009 with the objective of supporting global satellite products (Dorigo et al. Citation2011). ISMN stores a variety of in-situ hydrometeorological measurements such as soil moisture, temperature of soil and air, and precipitation. In this work, soil temperature records at 10 cm depth from 132 in-situ sites within the Continental United States (CONUS) are employed to calculate emissivity (). The CYGNSS data within 0.15° (approximately 14 km) of each site are collected and aggregated using the weight function described in section 3.3 to calculate the effective reflectivity. The corresponding brightness temperature data are obtained by linear interpolation from the data of the nearest four grid nodes.
2.4. GSW data
The Global Surface Water (GSW) dataset, jointly developed by the European Joint Research Centre (JRC) and the United States Geological Survey (USGS), is a comprehensive and high-resolution collection of information that maps the occurrence, changes, and dynamics of Earth’s surface water bodies over the past three decades (Pekel et al. Citation2016). The “seasonality” data are employed in this work, which is available at https://global-surface-water.appspot.com. The spatial resolution of this product is about 30 m × 30 m, and each pixel indicates the duration of open water in a year (0–12). Since the water body has a significant impact on the GNSS-R and brightness temperature data, we employ the water body removal method (Yang et al. Citation2023) and do not perform retrievals in 36 km pixels with a percentage of 30 m water pixels greater than 10%.
3. Method
3.1. Land emissivity derivation from brightness temperature
Derivation of emissivity from SMAP atmospherically corrected surface brightness temperature is founded on the radiative transfer equation commonly known as the model. The brightness temperature at the top of vegetation layer
for low frequencies can be modeled from emission components of soil and overlying vegetation canopy () (Jackson and Schmugge Citation1991):
Figure 2. Schematic of emission and GNSS-R reflectivity from vegetation and soil. Atmosphere contribution is not included here.
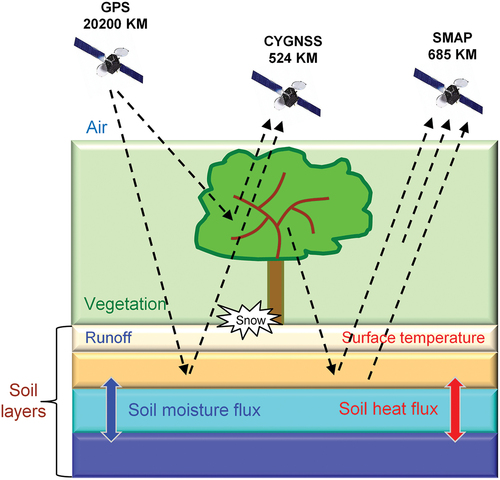
where, and
are the soil effective temperature and vegetation temperature,
and
are the rough soil emissivity and reflectivity,
is the vegetation transmissivity,
is the vegetation opacity,
is the vegetation single-scattering albedo,
is the incidence angle, and superscript
denotes the polarization (horizontal or vertical polarization).
and
are linked to the plane soil surface reflectivity
by
where is a dimensionless quantity that is linked to wavenumber and standard deviation of roughness.
The term “effective temperature” emphasizes that, when the physical temperatures of the media are not uniform, the effective temperatures in model are weighted means of the actual temperatures. At the time of SMAP descending pass, the soil, vegetation, and air are in approximate thermal equilibrium. If
and
are assumed to be equal and
is set equal to 0 for simplicity, the microwave emissivity at the top of the vegetation canopy is given by
where the emissivity of land surface is proportional to its brightness temperature
divided by its physical temperature
.
3.2. Reflectivity estimation from CYGNSS observations
Under the assumption that the land scattered signals are mainly coherent, the CYGNSS effective reflectivity can be derived through (Clarizia et al. Citation2019; Gleason et al. Citation2018):
where is the peak value of analog power,
and
are the ranges from transmitter and receiver to the specular point respectively,
is the GPS transmitted power,
is the gain of transmitter antenna,
is the gain of receiver antenna, and
is the signal wavelength. For GNSS-R reflectivity, the left-handed circular polarization is the main mode at low incidence angle.
3.3. Daily MLSE retrieval model
The passive microwave reflectivity is generated by the integration of bistatic scattering coefficients over the upper hemisphere, which is different from
that is generated in the specular and near-specular directions. This fundamental difference implies that the GNSS reflectometer is more affected by the surface roughness than passive microwave radiometry. Thus, it is inappropriate to assume that
can be simply replaced by
. In addition, GNSS-R generally employs reflected signals from GNSS satellites, which are circularly polarized and different from that of brightness temperatures. There is no denying that passive microwave emissivity is certainly correlated with GNSS-R reflectivity due to the common sensitivity to bio-geophysical parameters such as soil moisture and vegetation. However, owing to the absence of reliable small-scale surface roughness data and system errors from geometry, equipment, polarization, and frequency between the two observations, detailed physical derivation is still difficult at present. Therefore, this work focuses on the statistical relationship between them and retrieves emissivity from CYGNSS reflectivity.
We have already described that has a finer spatial resolution than
’s 36 km resolution. So, in order to be spatially comparable,
must be aggregated to the same EASE 2.0 grid before modeling. The coarser-resolution surface reflectivity
which matched the spatial resolution of SMAP can be aggregated from the heterogeneous finer-resolution
at date
and weight function
according to:
where refers to the location index of the EASE grid cell, and
refers to the location index of
in a certain grid cell. The weight
determines how much each reflection point contributes to the estimated reflectivity of the central cell and is determined by three measures as follows:
1) The location distance factor is defined as:
where is the location distance between the cell center and CYGNSS reflection point,
and
are the coordinates of the EASE grid cell,
and
are the coordinates of the CYGNSS observation. This measures the spatial variability between the observations. The spatial similarity is normally better for a closer point, and a higher weight should be assigned.
2) The time difference factor is defined as:
where is the acquisition time of CYGNSS data in a day,
is the time of SMAP descending pass. This calculation is performed independently for each day, including both on matched and non-matched dates. The metric indicates temporal changes such as soil moisture, temperature, and atmosphere occurring between CYGNSS and SMAP acquisition times.
3) The signal-to-noise ratio (SNR) is an observation derived from DDM, which is related to soil moisture and surface characteristics such as roughness and vegetation (Camps et al. Citation2016). In a certain 36 km EASE grid cell, each CYGNSS observation typically represents a footprint of about 7 × 0.5 km, and thus the variance of SNR can be used as the metric of small-scale variations in a grid cell. The variance of SNR is greater, the variability of this grid cell is more prominent, and such discrete observations are unreliable to derivate the aggregated reflectivity. The variance metric factor is:
This is an approximate measure to determine the homogeneity of a 36 km grid cell. A small value of implies a more representative reflectivity among all collected data in the grid cell and should be assigned a higher weight.
Then, the combined distance difference factor, time difference factor, and SNR variance metric factor can be computed with:
and a normalized reverse distance is used to derivate the final weight function :
This aggregation can mitigate the difference in spatial and temporal resolution between SMAP and CYGNSS data. However, it must be noted some potential error sources. The operating CYGNSS L1 calibration algorithm has not developed an algorithm to estimate specular point position specifically for land surfaces, which could cause residual estimation errors (Gleason et al. Citation2018) to the location distance factor. Although this work uses the SNR factor to represent the spatial heterogeneity (i.e. vegetation and roughness), it is expected to exist errors that are caused by the uncertainty in SNR observations. It would be preferable across the future to develop an indicator that incorporates high-resolution vegetation and roughness data. In addition, despite a criterion of incident angle larger than 40° used to filter the CYGNSS data, there is still a slight influence from the angle. This can be observed in both the simulation and observations (), where a slight increasing trend in CYGNSS reflectivity is noticeable as the incidence angle increases.
Figure 3. (a) Simulation of Fresnel reflection coefficient varying with incidence angle. The lines from bottom to top represent different soil moisture levels, from 0 to 0.5 cm3cm−3, with an interval of 0.05cm3cm−3. (b) Same as (a), but the Fresnel reflection coefficient has been normalized to show that soil moisture does not significantly change the relationship between Fresnel coefficient and incidence angle. (c) The CYGNSS reflectivity varying with incidence angle over the Sahara Desert [15-21N,0-8E] from 1 January to 10 January 2018.
![Figure 3. (a) Simulation of Fresnel reflection coefficient varying with incidence angle. The lines from bottom to top represent different soil moisture levels, from 0 to 0.5 cm3cm−3, with an interval of 0.05cm3cm−3. (b) Same as (a), but the Fresnel reflection coefficient has been normalized to show that soil moisture does not significantly change the relationship between Fresnel coefficient and incidence angle. (c) The CYGNSS reflectivity varying with incidence angle over the Sahara Desert [15-21N,0-8E] from 1 January to 10 January 2018.](/cms/asset/daed1bfa-fa98-430d-bd2d-8a71c4132c0d/tgsi_a_2374368_f0003_c.jpg)
Under the assumption in section 3.1, this work regards as the reference of surface emissivity, and here mainly discusses the method to generate daily MLSE dataset based on the CYGNSS data. displays the differences in
and
between January and July and the annual mean values. The correlation between the changes of
and
is −0.63, and −0.51 for the comparison in yearly averaged values. This indicates a more obvious temporal correlation between
and
than the spatial correlation. Therefore, inspired by Chew and Small (Citation2018)’s contribution to soil moisture retrieval, a pixel-by-pixel regression algorithm is employed here to model the relationship between
and
, and calculate the CYGNSS emissivity
at horizontal and vertical polarization. This pixel-by-pixel algorithm is effective in reducing the impact of intrinsic variability in different areas, such as roughness and soil type, and can significantly improve the accuracy of retrieval (Chen et al. Citation2022).
Figure 4. The differences in and
between January and July and the annual mean values. (a)
. (b)
. (c)
. (d)
.
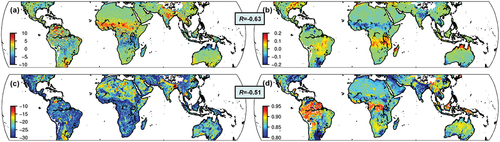
After completing the above calculation, the linear regression is performed independently in each EASE grid cell to derive the model coefficients from the matchups through:
where is the day of matchups,
and
are the linear coefficients,
is the emissivity retrievals from CYGNSS reflectivity. The polarization
is determined by the polarization of the input SMAP brightness temperature. Note that
and
vary in each grid cell. Then, the
at predicated date
can be calculated using the derived coefficients.
To mitigate the risk of overfitting, grid cells with data matchups fewer than 20 are marked as low confidence and excluded from the model training. Furthermore, the use of one-year data for training as well as the limited number of input parameters helped to focus the model on the most relevant information and prevented it from capturing complex, unnecessary patterns that could lead to overfitting.
shows the generation process of daily emissivity from CYGNSS reflectivity. The average number of days for collecting SMAP emissivity in all grid cells is 145, and the CYGNSS MLSE has a collection period of 270 days on average. Notably, the temporal resolution of CYGNSS MLSE has significantly improved by 86%, greatly complementing the existing microwave emissivity datasets.
3.4. Outlier removal
The biases in CYGNSS observations and aggregation could bring uncertainties to the retrieval. Thus, an outlier removal method is used here. The “3σ-Hampel identifier” is a more robust filter developed from the well-known “3σ-edit rule”, which can effectively remove the observations with more than three standard deviations from the median (Pearson et al. Citation2016). The model coefficients are calculated repeatedly until no outliers are generated, and about 7% of observations are removed.
4. Result
4.1. Pan-tropical results
shows the average and
for each grid cell in January 2018.
can well reflect the spatial variations in
both in the two polarizations. Overall, a high spatial consistency between them is obtained (R = 0.89 for horizontal polarization emissivity and 0.92 for vertical polarization emissivity, respectively). However,
seems to underestimate emissivity in some areas such as the Pampas Steppe and Central Africa.
The temporal correlation and root-mean-square error (RMSE) values of against
for each grid cell on the training set are shown in . It is clear that the consistency varies across different regions. In certain areas characterized by barren landscapes or dense forests such as the Sahara Desert and the Amazon rainforest, where the variations in emissivity are limited, it is expected to observe low values for both R and RMSE. Conversely, in moderate vegetation areas where emissivity undergoes significant seasonal fluctuations,
exhibits a satisfactory correlation with
, yielding an RMSE value of around 0.04 for horizontal polarization and around 0.03 for vertical polarization, respectively. Meanwhile, missing results are found in the Tibetan Plateau region, which can be explained by the effect of topographic relief on CYGNSS observation qualities. In addition, due to the systematic difference in the two polarization brightness temperature observations, lower RMSE values are obtained in vertical polarization results.
Figure 7. Statistical indices for against
on the training set. (a) R and (b) RMSE for horizontal polarization emissivity. (c) R and (d) RMSE for vertical polarization emissivity.
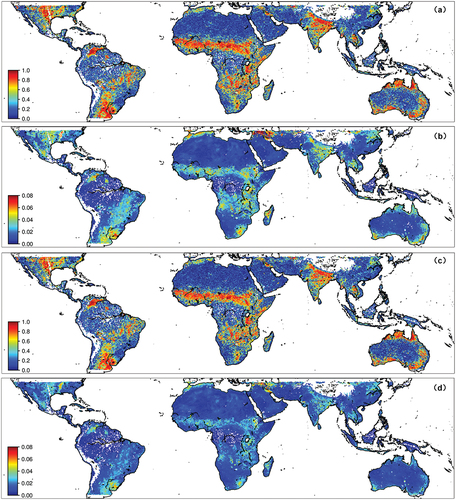
The values for horizontal polarization emissivity range from −0.021 to 0.001 (5th to 95th percentile, as applicable for all values in this paragraph) and −0.014 to 0.001 for vertical polarization, respectively (). On the other hand, the
values for horizontal polarization emissivity range from 0.398 to 0.921 and 0.601 to 0.962 for vertical polarization, respectively. It is observed that low
values and high
values are predominant in barren landscapes or dense forests, which aligns with the spatial patterns depicted in . Near-zero
values typically indicate a low spatial heterogeneity in emissivity. In forested regions, this can be attributed to the interference of microwave signals by dense vegetation, while in arid areas, the lack of soil moisture variability leads to limited fluctuations in emissivity.
Figure 8. Maps and histograms of (a) values and (b)
values for horizontal polarization emissivity, and (c)
values and (b)
values for vertical polarization emissivity, respectively. The histograms only include data with R > 0.4.
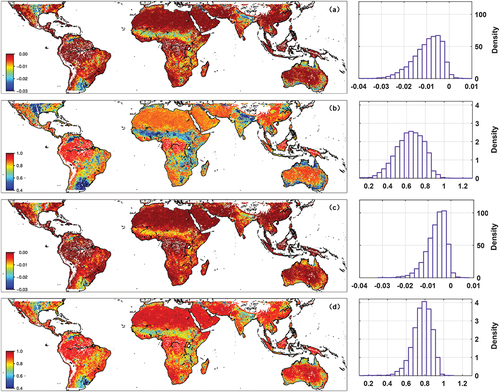
displays the density plot of versus
for all samples on the training set. The dots are well distributed around the 1:1 line and better consistency can be found in dense data. More scattered dots are observed at low emissivity, which is attributed to the high uncertainties in CYGNSS reflectivity under high soil moisture. Specifically, the R and RMSE are 0.89 and 0.022 for horizontal polarization, and 0.90 and 0.017 for vertical polarization, respectively.
shows the probability density function (PDF) of absolute differences between and
on the training set. More than 75% of
have an error of less than 0.02 and the mean error is 0.015, while they are 80% and 0.011 for
. The proportion of retrievals with high error is small, which indicates the high accuracy and reliability of emissivity retrievals from CYGNSS.
displays the model performance on the test set in terms of temporal RMSE distributions and density plots for both horizontal and vertical polarization, respectively. Good consistency between and
can be seen from this figure. The spatial patterns in RMSE distributions are similar to those observed in the training set results, but significant errors are found in central Madagascar and the northern Arabian Peninsula, which are characterized by plateau terrain. Overall, the R and RMSE are 0.79 and 0.030 for horizontal polarization, and 0.83 and 0.023 for vertical polarization, respectively.
Figure 11. Spatial RMSE distributions and density plots for against
on the test set. (a) Horizontal polarization. (b) Vertical polarization.
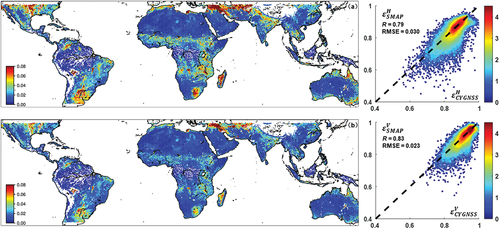
To evaluate the performance of in different land types, errors and mean model coefficients are calculated according to the International Geosphere Biosphere Program (IGBP) classes (as shown in ). Classes with a small type percentage are filtered out. The results indicate that accuracy is dependent on the land cover, with the poorest performance observed in croplands due to rapid vegetation growth. Overall, the RMSE for horizontal polarization is consistently below 0.03 for all land types, and below 0.02 for vertical polarization, respectively. As depicted in , the spatial patterns align with these findings, where areas characterized by low
values and high
values, such as barren areas and dense forests (e.g. Evergreen Broadleaf Forest, Mixed Forests, and Barren or Sparsely Vegetated), exhibit lower errors. These areas tend to display limited variations in emissivity, suggesting that
relies more on
in these areas rather than the sensitivity of CYGNSS reflectivity to emissivity. However, this also highlights the model’s susceptibility to overfitting in such areas, particularly when emissivity undergoes rapid changes, although such cases are rare.
Table 1. Error statistics and mean model coefficients for different IGBP land types.
Furthermore, to further analyze the dependence of retrievals on ,
values are set to zero for the calculations of
and compared with
. As shown in , the mean RMSE values for all land types are 0.020 and 0.015 for horizontal and vertical polarizations, respectively. However, when
, these values increase to 0.149 and 0.100, respectively. These results demonstrate the significant reliance of
on
, with a substantial increase in RMSE even in areas where
has low level. It is noteworthy that the retrieval performance in areas with moderate vegetation is more dependent on
compared to other areas, which is consistent with the observed levels of
.
4.2. In-situ results
Here, we focus on the results obtained specifically for some ISMN sites. Different from the pan-tropical reference emissivity, the reference here is calculated from SMAP brightness temperature and in-situ temperature records. The CYGNSS data within 0.15° (approximately 14 km) of each site are collected and aggregated using the weight function described in section 3.3 to calculate the effective reflectivity, and the corresponding brightness temperature data are interpolated from the data of the four nearest grid nodes. shows the comparisons between and
for all sites. A good correlation and a low RMSE can be observed for the two polarizations. Similar to pan-tropical results, both
and
have a better performance at high emissivity, and tend to underestimate at low emissivity. lists the RMSE errors for each site. The RMSE ranges from 0.004 to 0.081 for horizontal polarization and 0.005 to 0.076 for vertical polarization, with mean RMSE values of 0.031 and 0.024, respectively.
Table 2. Error statistics for all sites.
To further assess the accuracy of CYGNSS-retrieved emissivity compared with in-situ measurements, the time series and emissivity comparisons of six sites are displayed in . The sites are representatively selected from the Atmospheric Radiation Measurement (ARM), Soil Climate Analysis Network (SCAN), and U.S. Climate Reference Network (USCRN), where MLSE values exhibit ample variations. Limited in space, only results of horizontal polarization are shown here. The gray lines represent the both at matched date and the predicted date, while the blue dots represent the reference
. The time-series plots manifest the satisfactory consistency between
and
, especially on the days with drastic variations. The R values for the six sites range from 0.65 to 0.88, and the RMSE ranges from 0.022 to 0.048, respectively. Additionally, it is evident that CYGNSS can effectively provide daily emissivity estimations. The average number of days for collecting SMAP emissivity at these sites is 143, whereas the number for aggregated CYGNSS reflectivity is 311. Studies have proved that soil moisture is a key factor affecting emissivity. However, it is clear from the figure that the peaks of
and soil moisture do not always correspond one-to-one. This indicates emissivity is a complex parameter affected by many factors such as soil moisture, vegetation structure, and biomass. Thus, the CYGNSS derived reflectivity, which is also produced under the influence of the same environmental factors, can be a suitable alternative to MLSE for microwave remote sensing.
Figure 13. Time series (left) and error statistics (right) of versus
at six sites: Waukomis [36.33N, 97.89W], AAMU-jtg [34.78N, 86.59W], Levelland [33.59N, 102.37W], Starkville [33.67N, 88.74W], Goodwell-2-SE [36.59N, 101.62W], and Newton-8-W [31.34N, 84.45W]. The blue dots refer to
, the gray lines refer to
, and the green bars refer to soil moisture.
![Figure 13. Time series (left) and error statistics (right) of εCYGNSSH versus εSMAPH at six sites: Waukomis [36.33N, 97.89W], AAMU-jtg [34.78N, 86.59W], Levelland [33.59N, 102.37W], Starkville [33.67N, 88.74W], Goodwell-2-SE [36.59N, 101.62W], and Newton-8-W [31.34N, 84.45W]. The blue dots refer to εSMAPH, the gray lines refer to εCYGNSSH, and the green bars refer to soil moisture.](/cms/asset/843e1777-cbc7-46df-a7b2-b9fe2b8adbbd/tgsi_a_2374368_f0013_c.jpg)
5. Conclusions
This study presents and evaluates a method for estimating daily MLSE in the pan-tropical region using GNSS-R data. The proposed method uses a combined weight function of distance, time, and SNR variance to aggregate CYGNSS observations into the EASE 2.0 36 km grid, and employs a pixel-by-pixel regression algorithm to retrieve daily MLSE. The CYGNSS MLSE exhibits good agreement with SMAP brightness temperature derived MLSE, with an overall RMSE of 0.022 and 0.017 for horizontal and vertical polarization on the training set, and an RMSE of 0.030 and 0.023 on the test set, respectively. ISMN temperature records are utilized for in-situ validation, and both show good consistency with an RMSE of 0.034 and 0.026 for horizontal and vertical polarization, respectively. The accuracy of CYGNSS MLSE depends on the land cover, with errors obtained in dense vegetation and barren areas being significantly less than in other areas. Additionally, the temporal resolution of CYGNSS MLSE shows a remarkable improvement of 86% compared to SMAP MLSE, which can greatly complement the microwave emissivity dataset at the daily scale.
The satisfactory CYGNSS MLSE demonstrates an encouraging approach to obtain a daily MLSE dataset for microwave remote sensing. When compared to SMAP sensors, CYGNSS has an obvious advantage in terms of spatial and temporal resolution. In this work, CYGNSS data are aggregated in the EASE grid to allow for spatial comparison with SMAP data. Once the physical model for transforming the GNSS reflectometer to microwave radiometry is developed, it is expected that CYGNSS emissivity can be independently calculated with higher spatial and temporal resolution, without the need for SMAP data as a reference. Furthermore, it is worth noting that most existing emissivity studies only focus on clear-sky conditions, while this study retrieves MLSE under all weather conditions.
Disclosure statement
No potential conflict of interest was reported by the author(s).
Data availability statement
The CYGNSS data are openly available at https://podaac.jpl.nasa.gov., the SMAP data are available at https://smap.jpl.nasa.gov/data/., the ISMN data are available at https://ismn.earth/en/., and the GSW data are available at https://global-surface-water.appspot.com/.
Additional information
Funding
Notes on contributors
Yifan Zhu
Yifan Zhu is currently a PhD candidate at Wuhan University. He obtained his M.Sc. degree from Nanjing Normal University in 2021. His current research focuses on GNSS-R.
Fei Guo
Fei Guo is currently a professor at Wuhan University. He obtained his M.Sc. and PhD degrees with distinction in geodesy from Wuhan University in 2009 and 2013, respectively. His current research mainly focuses on GNSS-R and precise GNSS positioning.
Xiaohong Zhang
Xiaohong Zhang is currently a professor at Wuhan University. He obtained his M.Sc. and Ph.D. degrees with distinction in geodesy and engineering surveying at Wuhan University in 1999 and 2002, respectively. His main research interests include PPP, GNSS/INS navigation, and their applications in geoscience and engineering.
Wentao Yang
Wentao Yang is currently a PhD candidate at Wuhan University. He received the MS degree from Chang’an University in 2022. His research interests are in Global Navigation Satellite System (GNSS) remote sensing.
References
- Alonso-Arroyo, A., V. U. Zavorotny, and A. Camps. 2017. “Sea Ice Detection Using UK TDS-1 GNSS-R Data.” IEEE Transactions on Geoscience and Remote Sensing 55 (9): 4989–5001. https://doi.org/10.1109/TGRS.2017.2699122.
- Campbell, J. D., R. Akbar, A. Bringer, D. Comite, L. Dente, S. T. Gleason, L. Guerriero, E. Hodges, J. T. Johnson, and S. Kim. 2022. “Intercomparison of Electromagnetic Scattering Models for Delay-Doppler Maps Along a CYGNSS Land Track with Topography.” IEEE Transactions on Geoscience and Remote Sensing 60:1–13. https://doi.org/10.1109/TGRS.2022.3210160.
- Camps, A., H. Park, M. Pablos, G. Foti, C. P. Gommenginger, P. Liu, and J. Judge. 2016. “Sensitivity of GNSS-R Spaceborne Observations to Soil Moisture and Vegetation.” IEEE Journal of Selected Topics in Applied Earth Observations and Remote Sensing 9 (10): 4730–4742. https://doi.org/10.1109/JSTARS.2016.2588467.
- Chan, S., E. G. Njoku, and A. Colliander. 2020. SMAP L1C Radiometer Half-Orbit 36 Km EASE-Grid Brightness Temperatures, Version 5 [Data Set]. Boulder, Colorado USA: NASA National Snow and Ice Data Center Distributed Active Archive Center. Accessed December 1, 2024. https://doi.org/10.5067/JJ5FL7FRLKJI.
- Chen, F., X. Zhang, F. Guo, J. Zheng, Y. Nan, and M. Freeshah. 2022. “TDS-1 GNSS Reflectometry Wind Geophysical Model Function Response to GPS Block Types.” Geo-Spatial Information Science 25 (2): 312–324. https://doi.org/10.1080/10095020.2021.1997076.
- Chen, S., Q. Yan, S. Jin, W. Huang, T. Chen, Y. Jia, S. Liu, and Q. Cao. 2022. “Soil Moisture Retrieval from the CyGNSS Data Based on a Bilinear Regression.” Remote Sensing 14 (9): 1961. https://doi.org/10.3390/rs14091961.
- Chew, C. C., and E. E. Small. 2018. “Soil Moisture Sensing Using Spaceborne GNSS Reflections: Comparison of CYGNSS Reflectivity to SMAP Soil Moisture.” Geophysical Research Letters 45 (9): 4049–4057. https://doi.org/10.1029/2018GL077905.
- Chew, C., and E. Small. 2020. “Description of the UCAR/CU Soil Moisture Product.” Remote Sensing 12 (10): 1558. https://doi.org/10.3390/rs12101558.
- Clarizia, M. P., N. Pierdicca, F. Costantini, and N. Floury. 2019. “Analysis of CYGNSS Data for Soil Moisture Retrieval.” IEEE Journal of Selected Topics in Applied Earth Observations and Remote Sensing 12 (7): 2227–2235. https://doi.org/10.1109/JSTARS.2019.2895510.
- Cygnss. 2017. Cygnss Level 1 Science Data Record Version 2.1. Edited: NASA Physical Oceanography Distributed Active Archive Center. https://doi.org/10.5067/CYGNS-L1X21.
- Dong, Z., and S. Jin. 2021. “Evaluation of the Land GNSS-Reflected DDM Coherence on Soil Moisture Estimation from CYGNSS Data.” Remote Sensing 13 (4): 570. https://doi.org/10.3390/rs13040570.
- Dorigo, W. A., W. Wagner, R. Hohensinn, S. Hahn, C. Paulik, A. Xaver, A. Gruber, et al. 2011. “The International Soil Moisture Network: A Data Hosting Facility for Global in situ Soil Moisture Measurements.” Hydrology and Earth System Sciences 15 (5): 1675–1698. https://doi.org/10.5194/hess-15-1675-2011.
- Entekhabi, D., E. G. Njoku, P. E. O’Neill, K. H. Kellogg, W. T. Crow, W. N. Edelstein, J. K. Entin, et al. 2010. “The Soil Moisture Active Passive (SMAP) Mission.” Proceedings of the IEEE 98 (5): 704–716. https://doi.org/10.1109/JPROC.2010.2043918.
- Entekhabi, D., S. Yueh, P. E. O’Neill, K. H. Kellogg, A. Allen, R. Bindlish, M. Brown, S. Chan, A. Colliander, W. T. Crow, et al. 2010. “SMAP Handbook: Soil Moisture Active Passive: Mapping Soil Moisture and Freeze/Thaw from Space; Jet Propulsion Laboratory, California Institute of Technology: Pasadena, CA, USA.” Accessed January 8, 2020. https://smap.jpl.nasa.gov/system/internal_resources/details/original/178_SMAP_Handbook_FINAL_1_JULY_2014_Web.pdf.
- Eroglu, O., M. Kurum, D. Boyd, and A. C. Gurbuz. 2019. “High Spatio-Temporal Resolution CYGNSS Soil Moisture Estimates Using Artificial Neural Networks.” Remote Sensing 11 (19): 2272. https://doi.org/10.3390/rs11192272.
- Gleason, S., C. S. Ruf, A. J. O’Brien, and D. S. Mckague. 2018. “The CYGNSS Level 1 Calibration Algorithm and Error Analysis Based on On-Orbit Measurements.” IEEE Journal of Selected Topics in Applied Earth Observations and Remote Sensing 12 (1): 37–49. https://doi.org/10.1109/JSTARS.2018.2832981.
- Jackson, T. J., and T. J. Schmugge. 1991. “Vegetation Effects on the Microwave Emission of Soils.” Remote Sensing of Environment 36 (3): 203–212. https://doi.org/10.1016/0034-4257(91)90057-D.
- Jin, S., Q. Wang, and G. Dardanelli. 2022. “A Review on Multi-GNSS for Earth Observation and Emerging Applications.” Remote Sensing 14 (16): 3930. https://doi.org/10.3390/rs14163930.
- Jing, C., W. Li, W. Wan, F. Lu, X. Niu, X. Chen, A. Rius, et al. 2024. “A Review of the BuFeng-1 GNSS-R Mission: Calibration and Validation Results of Sea Surface and Land Surface.” Geo-Spatial Information Science: 1–15. https://doi.org/10.1080/10095020.2024.2330547.
- Karbou, F., C. Prigent, L. Eymard, and J. R. Pardo. 2005. “Microwave Land Emissivity Calculations Using AMSU Measurements.” IEEE Transactions on Geoscience and Remote Sensing 43 (5): 948–959. https://doi.org/10.1109/TGRS.2004.837503.
- Li, Z., F. Guo, F. Chen, Z. Zhang, and X. Zhang. 2023. “Wind Speed Retrieval Using GNSS-R Technique with Geographic Partitioning.” Satellite Navigation 4 (1): 4. https://doi.org/10.1186/s43020-022-00093-z.
- Min, Q., B. Lin, and R. Li. 2009. “Remote Sensing Vegetation Hydrological States Using Passive Microwave Measurements.” IEEE Journal of Selected Topics in Applied Earth Observations and Remote Sensing 3 (1): 124–131. https://doi.org/10.1109/JSTARS.2009.2032557.
- Moncet, J., P. Liang, J. F. Galantowicz, A. E. Lipton, G. Uymin, C. Prigent, and C. Grassotti. 2011. “Land Surface Microwave Emissivities Derived from AMSR-E and MODIS Measurements with Advanced Quality Control.” Journal of Geophysical Research: Atmospheres 116 (D16): 116. https://doi.org/10.1029/2010JD015429.
- Norouzi, H., M. Temimi, W. B. Rossow, C. Pearl, M. Azarderakhsh, and R. Khanbilvardi. 2011. “The Sensitivity of Land Emissivity Estimates from AMSR-E at C and X Bands to Surface Properties.” Hydrology and Earth System Sciences 15 (11): 3577–3589. https://doi.org/10.5194/hess-15-3577-2011.
- O’Neill, P. E., S. Chan, E. G. Njoku, T. Jackson, R. Bindlish, and J. Chaubell. 2020. SMAP L3 Radiometer Global Daily 36 Km EASE-Grid Soil Moisture, Version 7. Boulder, Colorado USA: NASA National Snow and Ice Data Center Distributed Active Archive Center. Accessed November 1, 2024. https://doi.org/10.5067/HH4SZ2PXSP6A.
- Pearson, R. K., Y. Neuvo, J. Astola, and M. Gabbouj. 2016. “Generalized Hampel Filters.” Eurasip Journal on Advances in Signal Processing 2016 (1): 87. https://doi.org/10.1186/s13634-016-0383-6.
- Pekel, J., A. Cottam, N. Gorelick, and A. S. Belward. 2016. “High-Resolution Mapping of Global Surface Water and its Long-Term Changes.” Nature 540 (7633): 418–422. https://doi.org/10.1038/nature20584.
- Prigent, C., W. B. Rossow, and E. Matthews. 1997. “Microwave Land Surface Emissivities Estimated from SSM/I Observations.” Journal of Geophysical Research: Atmospheres 102 (D18): 21867–21890. https://doi.org/10.1029/97JD01360.
- Ringerud, S., C. Kummerow, and C. Peters-Lidard. 2015. “A Semi-Empirical Model for Computing Land Surface Emissivity in the Microwave Region.” IEEE Transactions on Geoscience & Remote Sensing 53 (4): 1935–1946. https://doi.org/10.1109/TGRS.2014.2351232.
- Ringerud, S., C. Kummerow, C. Peters-Lidard, Y. Tian, and K. Harrison. 2013. “A Comparison of Microwave Window Channel Retrieved and Forward-Modeled Emissivities Over the U.S. Southern Great Plains.” IEEE Transactions on Geoscience & Remote Sensing 52 (5): 2395–2412. https://doi.org/10.1109/TGRS.2013.2260759.
- Ruf, C. S., R. Atlas, P. S. Chang, M. P. Clarizia, J. L. Garrison, S. Gleason, S. J. Katzberg, et al. 2016. “New Ocean Winds Satellite Mission to Probe Hurricanes and Tropical Convection.” Bulletin of the American Meteorological Society 97 (3): 385–395. https://doi.org/10.1175/BAMS-D-14-00218.1.
- Saïd, F., Z. Jelenak, J. Park, and P. S. Chang. 2021. “The NOAA Track-Wise Wind Retrieval Algorithm and Product Assessment for CyGNSS.” IEEE Transactions on Geoscience and Remote Sensing 60:1–24. https://doi.org/10.1109/TGRS.2021.3087426.
- Tian, Y., C. D. Peters Lidard, K. W. Harrison, Y. You, S. Ringerud, S. Kumar, and F. J. Turk. 2015. “An Examination of Methods for Estimating Land Surface Microwave Emissivity.” Journal of Geophysical Research Atmospheres 120 (21): 111–114, 128. https://doi.org/10.1002/2015JD023582.
- Turk, F. J., Z. S. Haddad, and Y. You. 2014. “Principal Components of Multifrequency Microwave Land Surface Emissivities. Part I: Estimation Under Clear and Precipitating Conditions.” Journal of Hydrometeorology 15 (1): 3–19. https://doi.org/10.1175/JHM-D-13-08.1.
- Weng, F., B. Yan, and N. C. Grody. 2001. “A Microwave Land Emissivity Model.” Journal of Geophysical Research: Atmospheres 106 (D17): 20115–20123. https://doi.org/10.1029/2001JD900019.
- Wu, Y., J. Bao, Z. Liu, Y. Bao, and G. P. Petropoulos. 2022. “Investigation of the Sensitivity of Microwave Land Surface Emissivity to Soil Texture in MLEM.” Remote Sensing 14 (13): 3045. https://doi.org/10.3390/rs14133045.
- Yan, Q., W. Huang, S. Jin, and Y. Jia. 2020. “Pan-Tropical Soil Moisture Mapping Based on a Three-Layer Model from CYGNSS GNSS-R Data.” Remote Sensing of Environment 247:111944. https://doi.org/10.1016/j.rse.2020.111944.
- Yang, T., W. Wan, J. Wang, B. Liu, and Z. Sun. 2022. “A Physics-Based Algorithm to Couple CYGNSS Surface Reflectivity and SMAP Brightness Temperature Estimates for Accurate Soil Moisture Retrieval.” IEEE Transactions on Geoscience and Remote Sensing 60:1–15. https://doi.org/10.1109/TGRS.2022.3156959.
- Yang, W., F. Guo, X. Zhang, and Y. Zhu. 2023. “An Improved Method for Water Body Removal in Spaceborne GNSS-R Soil Moisture Retrieval.” IEEE Transactions on Geoscience and Remote Sensing 61:1–8. https://doi.org/10.1109/TGRS.2023.3264629.
- Zhang, J., T. Zhao, S. Tan, N. Rodriguez-Fernandez, H. Xue, N. Yang, Y. Kerr, and J. Shi. 2023. “Characterizing the Channel Dependence of Vegetation Effects on Microwave Emissions from Soils.” Geo-Spatial Information Science 1–17. https://doi.org/10.1080/10095020.2023.2275616.
- Zribi, M., D. Guyon, E. Motte, S. Dayau, J. P. Wigneron, N. Baghdadi, and N. Pierdicca. 2019. “Performance of GNSS-R GLORI Data for Biomass Estimation Over the Landes Forest.” International Journal of Applied Earth Observation and Geoinformation 74:150–158. https://doi.org/10.1016/j.jag.2018.09.010.