Abstract
The aim of this study is to compare the changes that occurred in the main urban land-cover classes of Ulaanbaatar city, Mongolia, during a centralized economy with those that occurred during a market economy and to describe the socio-economic reasons for the changes. For this purpose, multi-temporal remote sensing and geographical information system (GIS) data sets, as well as census data, are used. To extract the reliable urban land-cover information from the selected remotely sensed data sets, a refined parametric classification algorithm that uses spatial thresholds defined from local and contextual knowledge is constructed. Before applying the classification decision rule, some image fusion techniques are applied to the selected remotely sensed data sets to define the most efficient fusion method for training sample selection and for defining local and contextual knowledge. Overall, the study indicates that during the centralized economy significant changes occurred in a ger area of the city, whereas during the market economy the changes occurred in all areas.
1. Introduction
In recent years, cities all over the world have experienced rapid growth because of the significant increase in world population and the flow of people from rural to urban areas. Specifically, larger towns and cities in developing nations have experienced rapid population increases and many are facing the emergence of unplanned and uncontrolled settlements at their fringes (Amarsaikhan et al. Citation2001, Amarsaikhan Citation2005). When urban areas expand, natural areas such as forests and grassland, as well as some agricultural lands, are transformed into housing, industry and infrastructure. Moreover, rapid urbanization processes followed by an industrialization process can contribute to other environmental changes, such as increases in the urban heat island effect and increased water and air pollution (Tachizuka et al. Citation2002).
To prevent rapid urban expansion, especially from the unplanned urbanization process, urban planners and decision-makers need to regularly evaluate development procedures using updated urban planning maps. However, many city planners in developing countries lack access to updated maps and often rely on old data that are not relevant (Amarsaikhan et al. Citation2001, Amarsaikhan Citation2005). One possible solution is the use of remotely sensed images with different spatial and spectral resolutions. Over the past few years, remote sensing platforms, techniques and technologies have evolved. System capabilities have greatly improved. Meanwhile, the cost of many of these data sets has drastically decreased. Now the highest spatial resolution image can be acquired with centimetres-accuracy, whereas the ordinary high-resolution images can be acquired with a few metres accuracy (Amarsaikhan and Sato Citation2003). It is now possible to extract different thematic information at various scales, to integrate the extracted information with other historical data sets stored in a GIS and to conduct sophisticated analyses.
Mongolia, as is the case with many countries of the developing world, has a problem with rapid urban expansion and the growth of population in the main cities. In general, much of Mongolia's urban growth has taken place since the middle of 1970s, because, at that time, the government encouraged migration to urban areas, specifically to Ulaanbaatar, in the belief that this would increase the industrialization and productivity in the country. To accommodate the growing population in the capital city, the government mainly constructed high-rise apartment blocks. However, the number of apartments fell well short of satisfying the demands of the growing population. Therefore, rural people migrated to Ulaanbaatar, and set up gers (portable dwellings) for their accommodation. The gers districts were usually established in urban fringes. This created a unique culture in Ulaanbaatar, making it the only capital city in the world that has a mixed culture of modern civilization and nomadic culture. The history of the Mongol ger, goes back to about 2500–3000 B.C. and it is still used as dwelling everywhere in Mongolia.
The gers situated in urban areas are surrounded by fences that occupy usually 600–800 m2. The families living in ger districts usually have houses made of brick or wood beside their gers. A Mongol ger and a typical example of a ger district represented in a Quickbird image are shown in .
Figure 1. A Mongol ger (a) and a typical example of a ger district in Ulaanbaatar represented in a Quickbird image of 2002 (b) (1-ger and 2-house).
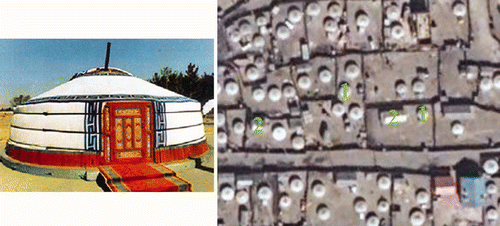
In 1990, Mongolia entered market economy and it totally changed the lives in the society. For the isolated rural people, it had become very difficult to reach the central market. Meanwhile, many things started to centralize in the capital city and Ulaanbaatar became a dream location for many rural people. Therefore, many rural families officially and unofficially moved to Ulaanbaatar. As a result, the population of Ulaanbaatar increased significantly and the city area expanded rapidly. To analyse such changes, urban planners need detailed and regularly updated maps. However, if there are no such maps, then an updated map generated through an information extraction procedure from remotely sensed data with an acceptable resolution can (at least) give them an impression about the changes in the city.
In general, it should be interesting to study the urban growth in Ulaanbaatar city comparing the growth that occurred during the main industrialization period (1969–1990) when Mongolia had a centralized (i.e. socialist) economy to changes that occurred during the market economy. For this study, changes that occurred in Ulaanbaatar between 1969 and 1990 were compared with the changes that occurred between 1990 and 2001 using historical GIS data as well as multi-temporal remotely sensed images, and the socio-economic reasons for the changes were described. Such comparisons may be helpful for the planners to consider directions of the urbanization process and how it impacts the population and society in both spatial and socio-economic terms. To extract the reliable urban land-cover information from the selected remotely sensed data sets, a refined parametric classification algorithm that uses spatial thresholds defined from the local and contextual knowledge was used. Before applying the classification decision rule, some image fusion techniques were applied to the selected remotely sensed data sets to define the most efficient fusion method that creates the best colour image for training sample selection and for defining local and contextual knowledge.
2 Test site and data sources
Ulaanbaatar is situated in the central part of Mongolia, on the Tuul River, at an average height of 1350 m above sea level. It is home to nearly 1 million inhabitants (National Statistical Office of Mongolia 2007). The city is surrounded by the mountains which are spurs of the Khentii Mountain Ranges. Founded in 1639 as a small town named Urga, today it has prospered as the main political, economic, business, scientific and cultural centre of the country. The part of the capital city selected here for study is about 28 km × 20 km. It covers the majority of the area belonging to the capital city, although there are some areas extending outside of the selected image frame. For the selected area, land-cover classes such as building area, ger area, forest, grassland, soil and water can be identified.
Landsat TM data acquired on 10 September 1990 with a spatial resolution of 30 m, SPOT PAN data acquired on May 1990 with a spatial resolution of 10 m and Landsat ETM + data acquired on 31 August 2001 were used. The ETM + data consisted of a panchromatic band resampled to a pixel resolution of 14 m and seven multi-spectral bands resampled to a pixel resolution of 28 m. In addition, topographic maps of 1969 and 1984 (1:50,000), and a general urban planning map were available. shows the recent look of the test area in the ETM image and some examples of its land cover.
3 Radiometric correction and georeferencing of the panchromatic and multi-spectral images
All the available images were thoroughly analysed in terms of radiometric quality and geometric distortion. For the SPOT PAN data some line dropouts occurred, whereas the panchromatic band of the ETM + data had some radiometric noise. To correct the error in the SPOT PAN data, pixel values in lines above and below the bad lines were averaged. For the panchromatic band of the ETM + data, a 3 × 3 average filter was applied to the entire image. In case of the multi-spectral images, band 1 (i.e., blue band) of both TM and ETM + had noise from the atmosphere and they were, therefore, excluded from the analysis. Likewise, the TM and ETM + thermal bands were excluded from the further analysis.
To merge data sets with different spatial resolutions, high geometric accuracy and good geometric correlation between the data sets are needed. Initially, the panchromatic image of the ETM + data was georeferenced to a UTM map projection using a topographic map of 1984 (1:50,000). The ground control points (GCP) were selected on well-defined cross-sections of roads, streets and other clearly delineated sites. In total, 16 regularly distributed points were selected. For the transformation, a second-order transformation and nearest neighbour resampling approach were applied (RMSE = 0.92). To georeference the other images, 16 regularly distributed GCPs were selected on each image comparing the locations of the selected points with other information such as the already georeferenced panchromatic image of the ETM + and the selected topographic maps. Then, the images were successively georeferenced to the UTM map projection using the topographic map of 1984. For the actual transformations, a second-order transformation and nearest neighbour resampling approach were applied. The RMS errors of the image transformations were 0.98 for the SPOT PAN, 0.65 for the TM and 0.79 for the ETM+. In each case, the image was resampled to a pixel resolution of 14 m.
4 Image fusion
The test area is a complex urban environment. In the area, the three main land-cover classes (building area, ger area and soil) have similar spectral characteristics. Therefore, to enhance the spectral and spatial variations of these statistically overlapping classes, as well as to merge the images with different spatial resolutions, different image fusion techniques were compared. Within the framework of the study, it was decided that the fused image should be used for determining the sites for the training sample selection and also for defining the local and contextual knowledge about the classes of objects.
After applying radiometric correction and georeferencing procedures, data with different spatial resolutions can easily be integrated. The image fusion is the integration of different digital images to create a new image and obtain more information than can be separately derived from any of them (Pohl and Van Genderen Citation1998, Ricchetti Citation2001). In the case of the present study, for the urban areas, the panchromatic images provide more spatial information because of their higher spatial resolution, whereas the multi-spectral images provide the information about the spectral variations of the urban classes. In this study, the fusion was performed at a pixel level and the following methods were applied: (a) Brovey transform, (b) intensity–hue–saturation (IHS) transformation, (c) principal component analysis (PCA) and (d) texture enhancement. Detailed reviews of these techniques are given in Pohl and Van Genderen (Citation1998), Richards and Jia (Citation1999), Mather (Citation1999), Gonzalez and Woods (Citation2002), Amarsaikhan and Douglas (Citation2004) and Amarsaikhan and Sato (Citation2004).
To obtain a reliable colour image that can illustrate the spectral and spatial variations of the selected classes of objects, different band combinations were used. The image created by the Brovey transform illustrated good colour separation among the selected classes, whereas images created by the IHS and texture enhancement methods were not useful for distinguishing between some ger areas and soil class. The PCA image demonstrated a better result than any other methods, because it illustrated good colour as well as spatial separations among the available classes. Therefore, for both data sets, the PCA images were selected for determining the sites for the training sample selection as well as for defining the local and contextual knowledge about the classes of objects.
The PCA was performed using the available panchromatic and multi-spectral bands and the results are shown in . As can be seen from , PC1 of the 1990 data sets is dominated by the variance of the middle-infrared bands, whereas PC2 is dominated by the variance of the near-infrared band, though the red band has a high negative loading. TM5 has a contribution to the PC3, however, TM4 has a very high negative loading in it. In the case of 2001 data sets, PC1 is also dominated by the variance of the middle-infrared bands, but the red band has a contribution, too. The PC2 in these data sets is dominated by the variance of the middle-infrared band, though the red band has a high negative loading. PC3 shows a high negative loading from the near infrared band, however, panchromatic and middle infrared bands have almost equal amount of negative and positive loadings in it. Furthermore, it can be seen from that the first three PCs in both data sets contain almost 99% of the total variance. The inspection of other PCs indicated that they contained noise from the total dataset. Therefore, they were excluded from the final analysis. shows the comparison of the multi-temporal PC images.
Figure 3. Comparison of the multi-temporal PC images. (a) PC image of 1990 data sets (red, PC1; green, PC2, blue, PC3), (b) PC image of 2001 data sets (red, PC1; green, PC2; blue, PC3).
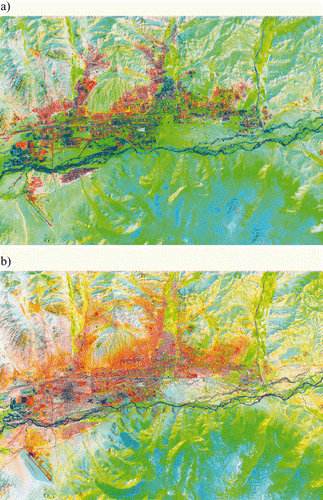
Table 1. Principal component coefficients.
5 Classification of the images
The high-resolution multi-spectral remotely sensed images such as Landsat and SPOT can provide reliable and updated information for monitoring of land-cover changes related to urbanization at a regional scale, whereas the very-high resolution images such as IKONOS and Quickbird can provide the most reliable real time information at a local scale. Over the years, multi-spectral remotely sensed data sets have been widely used for urban land-cover mapping at a regional scale and for the generation of land-cover information, diverse classification methods have been applied. The early methods mainly involved supervised and unsupervised methods and hence, many techniques have been developed. At present, the most commonly used multi-spectral remotely sensed data classification techniques are parametric and non-parametric methods and neural networks (Cortijo and Blanca Citation1997, Borak and Strahler Citation1999, Kavzoglu and Mather Citation2002, Linderman et al. Citation2004, Amarsaikhan et al. Citation2007). In this study, to extract the reliable urban land-cover information from the selected remotely sensed data sets, a refined parametric classification algorithm that uses spatial thresholds defined from the local and contextual knowledge was used.
5.1 The selected features and evaluation of the training signatures
Generally, in the classification process, it is desirable to include only the features in which the signatures of the selected classes are highly separable in multi-dimensional feature space. In this study, as the features, the three PCs defined from RS data sets of 1990 and 2001 were selected because they contained almost 99% of the total variance of the input scenes.
To define the sites for the training signature selection, initially, from the multi-temporal PC images, two to five areas of interest (AOI) have been selected for each available class (i.e., building area, ger area, forest, grass land, soil and water) using the local and contextual knowledge. The local knowledge has been defined from the historical GIS data sets (i.e., topographic and urban planning maps), whereas the contextual knowledge was defined based on the spectral variations of the land surface features as well as the texture information delineated on the PC images. The separability of the selected training signatures was firstly checked in feature space and then evaluated using Jeffries–Matusita distance (ERDAS Citation1999) and it revealed that high statistical overlaps exist between the classes building area and ger area. It is obvious, because ger families usually have houses beside their gers. Therefore, these two classes have very similar spectral characteristics. Then, the samples which demonstrated the best possible separability were chosen to form the final signatures. The final signatures included about 126–498 pixels (i.e., building area, 397 pixels; ger area, 316 pixels; forest, 298 pixels; grass land, 197 pixels; soil, 498 pixels; water, 126 pixels).
5.2 The refined parametric classification method
For the actual classification, the Mahalanobis distance classifier (MDC) has been used. The MDC is a parametric method, in which the criterion to determine the class membership of a pixel is the minimum Mahalanobis distance between the pixel and the class centre (Richards and Jia Citation1999).
Initially, to check the performance of the standard method, the selected PCA features were classified using the MDC. However, on the classified images there were different mixed classes between the classes: building area and ger area. This should be evident, because the previous signature analysis indicated that the signature distributions of these classes had significant overlaps in the multi-dimensional feature space. To separate the statistically mixed classes, the class specific features as well as spatial thresholds (i.e., spatial boundaries) can be applied. The class specific features can be determined through the feature extraction process; however, the application of this approach would become difficult if there is a fewer number of bands. The spatial thresholds can be defined from the knowledge about the test area or historical GIS data sets. The idea of the spatial threshold is that it uses a polygon boundary to separate the overlapping classes within a selected image frame and only the pixels falling within the threshold boundary are used for the classification (i.e. the overlapping classes are spatially separated). In that case, the likelihood of the pixels to be correctly classified will significantly increase, because the pixels belonging to the class that overlaps with the class to be classified using the threshold boundary are (spatially) temporarily excluded from the decision making process. In such a way, the image can be classified several times using different threshold boundaries and the results can be merged (Amarsaikhan and Sato Citation2003).
In this study, to separate the statistically overlapping classes, different spatial thresholds determined based on the local and contextual knowledge have been used. The local knowledge was based on the knowledge about the site as well as the historical GIS data sets, whereas the contextual knowledge was based on the spectral and textural variations of the selected classes in different parts of the PCA images. To determine the initial spatial thresholds, first the appropriate polygon boundaries related to the selected classes were defined from different parts of the selected image frame based on the historical GIS data sets. As these polygon boundaries represented old information, it was necessary to update them. For this purpose, new polygon boundaries were defined from the PC images based on the contextual knowledge (i.e., defining class boundaries in relation to its neighbourhood) and added to the initial spatial thresholds, thus creating a set of polygon boundaries. The results of the classifications using the defined spatial thresholds are shown in .
Figure 4. Comparison of the classification results for the selected classes. (a) Classified image using 1990 data sets, (b) classified image using 2001 data sets.
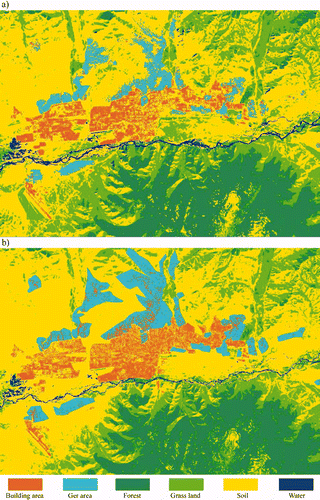
For the accuracy assessment of the classification results, the overall performance was used. This approach creates a confusion matrix in which the selected reference pixels are compared with the classes in the classified image and as a result, an accuracy report is generated indicating the percentages of the correspondence (ERDAS Citation1999). In this study, as ground truth information different AOIs containing the purest pixels were been selected. The confusion matrices produced for the refined parametric classification method are shown in . As seen from the confusion matrices, overall accuracy for the 1990 data sets was 91.98%, whereas for the 2001 data sets it was 92.89%, indicating an overall accuracy of more than 90% for each of the selected classes. A general diagram of the refined parametric classification is shown is .
Table 2. Confusion matrix produced for the classified PC images.
6 Urban land-cover change analysis
In this study, we wanted to compare the general changes occurred in the main land-cover classes (building area, ger area, forest, grassland, soil and water) of Ulaanbaatar area during the centralized economy (1969–1990) with the changes occurred during a market economy (1990–2001) using historical GIS data as well as multi-temporal remotely sensed images, and to explain the socio-economic reasons for the changes.
Initially, to create the primary historical GIS data, the classes: building area, ger area, forest and water were digitized in a Gauss-Kruger map projection from a topographic map of 1969 (1:50,000) (). On this topographic map, the areas related to the grassland were not delineated. Therefore, it was not possible to distinguish between grassland areas and soil classes. The apartments, residential houses, industrial buildings and all other building areas were included in the building area class, because on the remotely sensed images it was not possible to distinguish among these classes, because of their very similar spectral characteristics. However, using ground truth data and knowledge about some local areas one can observe that the main industrial areas are mainly distributed in the southern part of the city and some are located in the western part.
Figure 6. Topographic map of Ulaanbaatar area of 1969, scale 1:50,000. The size of the displayed area is about 28 km × 20 km.
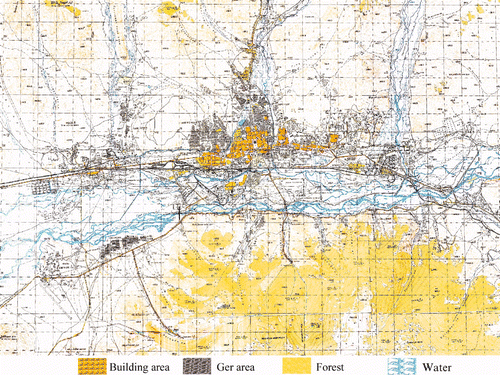
To define the total area of each class expressed in hectares (ha), the vector map was rasterized with a pixel resolution of 14 m and then a number of pixels falling into each of the classes was calculated. Likewise, from the classified PCA images, the total number of pixels falling into each of the classes has been calculated. The total areas related to each class defined from the digitized map as well as classified multi-temporal images are shown in . Although, we have census data of Ulaanbaatar city, it is not possible to directly relate it to the current analysis, because our study area does not cover all the areas from where the final census data are collected. However, as the test area covers the majority of the area belonging to the capital city, it is possible to use the census data for a comparison of the general population increase with the actual urban expansion process.
Table 3. The total areas for each class in different years, evaluated from multi-temporal GIS and RS data sets.
As seen from , in 1969 in Ulaanbaatar city, the building area and ger area covered 2498.92 ha and 978.86 ha, respectively, whereas in 1990 these two urban classes covered 3996.71 ha and 2719.65 ha, respectively. Moreover, it is seen that the forest and water resources had been reduced. The available census data indicated that in 1969 the population of the capital city was 267,400, whereas in 1990 it had become 574,900. As seen, within the 21-year period of the centralized economy, the building areas were increased by only 59.94%, whereas the ger areas were increased more than two-fold. Meanwhile, the population increased by more than two times. These changes are related to the following:
-
Because of the industrialization process, many people came from different parts of the country seeking better lives.
-
During the centralized economy, the government constructed mostly high-rise apartment blocks with many stories to accommodate the growing population. These residential blocks, although they could house the families of many ger districts, occupied a much smaller space than the ger parcels. For example, one apartment block with five stories which could accommodate 60 families while occupying about 1000 m2, whereas one family living in a ger district occupied usually 600–800 m2.
-
During the centralized economy, many people came from rural sites with their gers to the city. Although, the government constructed high-rise apartment blocks, they could not satisfy the growing demands of the increasing population. Therefore, those people who could not get apartments usually used their gers as dwelling houses. Therefore, the ger districts underwent this significant expansion.
Furthermore, as seen from , within the 11-year period since the country entered the market economy, the building area and ger area had been increased by 20.41% and 58.26%, respectively. Meanwhile, forest, grassland and water resources had been reduced, expanding the areas of bare soil. The reasons for these changes are:
-
Because of the market economy many people moved to the areas with good infrastructures, thus increasing the population. For example, census data indicated that in 2001, the population of the capital city had become 812,500.
-
Since the country entered the market economy, the government has not constructed new residential apartments. The apartment prices of the existing construction companies are very high, so, most people cannot afford them. Therefore, most people moving to Ulaanbaatar built up their gers surrounded by fences, thus significantly expanding the ger districts.
-
During the market economy, most of the constructed buildings are western cottage style houses or buildings with few stories, not like the former apartment blocks. Therefore, they occupied larger areas than the high-rise apartments and also contributed to the expansion of the city areas.
-
Currently in Mongolia there is a boom to own land. The new land law issued in 2002 (New Land Law of Mongolia Citation2002) gives distinct rights to land owners and they can use their land for receiving bank loans, therefore, the interests of people to own land parcels have greatly increased.
7 Conclusions
The overall idea of the research was to compare the changes that occurred in the main urban land cover classes of Ulaanbaatar city, Mongolia during a centralized economy with the changes occurred during a market economy and describe the socio-economic reasons for the changes. For this purpose, multi-temporal images and GIS data sets as well as census data were used.
To extract the reliable urban land-cover information from the available remotely sensed data sets, a refined parametric classification algorithm based on the Mahalanobis distance classifier that uses spatial thresholds defined from the local and contextual knowledge was constructed. Before applying the classification decision rule, different image fusion techniques such as Brovey transform, IHS, texture enhancement and PCA were explored to define the most efficient fusion method that could create the best colour image used for training sample selection as well as for defining the local and contextual knowledge and the PCA images demonstrated better results than any other methods. As seen from the classification results, the spatial thresholds defined from the local and contextual knowledge could significantly improve the performance of the classification and for the accurate classification proper spatial thresholds should be applied.
As seen from the urban land-cover change analysis, during the centralized economy significant changes occurred in the ger area of the city, whereas during the market economy the changes occurred in both areas. Moreover, as seen from the analysis, during all this time, the natural resources such as forest and water had been reduced.
Because of the spatial resolution of the image data sets, the results of this study could be used for a decision-making process at a regional scale. For detailed analysis, large scale maps and very high resolution RS images covering all areas should be used.
Acknowledgements
A part of this research was conducted under the sponsorship for Humboldt Fellows. The authors are also thankful to the reviewers for their useful comments and suggestions.
References
- Amarsaikhan , D. . Applications of RS and GIS for urban planning and management in Mongolia . Proceedings of Mongolia-Korea Conference in Urban Planning . Ulaanbaatar. pp. 25 – 39 .
- Amarsaikhan , D. and Douglas , T. 2004 . Data fusion and multisource data classification . International Journal of Remote Sensing , 17 : 3529 – 3539 .
- Amarsaikhan , D. , Ganzorig , M. , Ache , P. and Blotevogel , H. 2007 . The integrated use of optical and InSAR data for urban land cover mapping . International Journal of Remote Sensing , 28 ( 6 ) : 1161 – 1171 .
- Amarsaikhan , D. , Ganzorig , M. and Saandar , M. 2001 . Urban change study using RS and GIS . Asian Journal of Geoinformatics , 2 ( 2 ) : 73 – 79 .
- Amarsaikhan , D. and Sato , M. . The role of high resolution satellite images for urban area mapping in Mongolia . ‘Reviewed Papers’ part of Proceedings of the Computers for Urban Planning and Urban Management (CUPUM)' 03 International Conference . Sendai, Japan. pp. 1 – 12 .
- Amarsaikhan , D. and Sato , M. 2004 . Validation of the Pi-SAR data for land cover mapping . Journal of the Remote Sensing Society of Japan , 24 ( 2 ) : 133 – 139 .
- Borak , J. S. and Strahler , A. H. 1999 . Feature selection and land cover classification of a MODIS-like data set for a semiarid environment . International Journal of Remote Sensing , 20 : 919 – 938 .
- Cortijo , F. J. and Blanca , N. P. 1997 . A comparative study of some non-parametric spectral classifiers. Applications to problems with high-overlapping training sets . International Journal of Remote Sensing , 18 : 1259 – 1275 .
- ERDAS . 1999 . Field guide , 5th ed. , Atlanta, Georgia : ERDAS .
- Gonzalez , R. C. and Woods , R. E. 2002 . Digital image processing , 2nd ed. , Upper Saddle River, New Jersey : Prentice-Hall .
- Kavzoglu , T. and Mather , P. M. 2002 . The role of feature selection in artificial neural network applications . International Journal of Remote Sensing , 23 : 2919 – 2937 .
- Linderman , M. , Liu , J. , Qi , J. , An , L. , Ouyang , Z. , Yang , J. and Tan , Y. 2004 . Using artificial neural networks to map the spatial distribution of understorey bamboo from remote sensing data . International Journal of Remote Sensing , 25 : 1685 – 1700 .
- Mather , P. M. 1999 . Computer processing of remotely-sensed images: an introduction , 2nd ed. , New York : Wiley .
- National Statistical Office of Mongolia . 2007 . Mongolian statistical year book , Ulaanbaatar, , Mongolia : National Statistical Office of Mongolia .
- 2002 . New land law of Mongolia , Ulaanbaatar, , Mongolia : New Land Law of Mongolia .
- Pohl , C. and Van Genderen , J. L. 1998 . Multisensor image fusion in remote sensing: concepts, methods and applications . International Journal of Remote Sensing , 19 : 823 – 854 .
- Ricchetti , E. 2001 . Visible–infrared and radar imagery fusion for geological application: a new approach using DEM and sun-illuminated model . International Journal of Remote Sensing , 22 : 2219 – 2230 .
- Richards , J. A. and Jia , X. 1999 . Remote sensing digital image analysis – an introduction , 3rd ed. , Berlin : Springer-Verlag .
- Tachizuka , Sh. , Hung , T. , Ochi , Sh. and Yasuoka , Y. 2002 . “ Monitoring of long-term urban expansion by the use of remote sensing images from different sensors ” . Available online at: www.gisdevelopment.net/aars/acrs/2002/urb