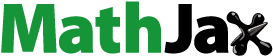
ABSTRACT
Disinformation is a form of offensive counterintelligence via deception and neutralization in order to strategically manipulate an audience or create further fractures in existing divisions. Disinformation strategies include leaking, lying, seeding, and smearing. These strategies vary according to whether the information conveyed is true or false, and whether the source uses or hides its identity. This study characterizes the strategic relationship between lying and leaking, and the extent true and false sources of disinformation are believed. Additional characterizations include noisy and neutralizing disinformation, the importance of medium versus message, echo chambers, and the half-life of secrets.
Introduction
Disinformation, also known as active measures, is a form of offensive counterintelligence via deception and neutralization in order to strategically manipulate an audience or create further fractures in existing divisions. According to the U.S.’s Cybersecurity & Infrastructure Security Agency (CISA), ‘Disinformation is [information that is] deliberately created to mislead, harm, or manipulate a person, social group, organization, or country.’Footnote1
Upon reflection, disinformation strategies cannot be restricted to false or misleading information because they would hardly be believed if always known to lack veracity. Indeed, the U.S. CIA regards the most effective means of disinformation to be seeding or conditioning whereby factual content is carried by phony outlets – when the identity of the Source is fake but the content is accurate (Rid Citation2020). Consequently, a disinformation strategy can involve the truth, with the Source of the information or the identity of the publisher hidden instead. An example is using a fake website to disseminate truthful information harmful to an adversary. The misleading aspect of disinformation can be the Source’s identity rather than the content. Hence, disinformation involves strategic manipulation by means of content, outlet, or both.
A historical example of the medium being the message is the CIA’s Cold War Operation QKOpera, where front organization Council for Cultural Freedom subsidized magazines and sponsored international exhibits and conferences involving U.S. artists, academics, musicians, and writers (Saunders Citation1999). While the individuals involved were often unwitting participants, the CIA viewed the works and events as exemplifying the superior conditions for incubating cultural advances under the U.S.’s version of democratic capitalism. Such operations typify organizations who see their own ideology as both stronger than the adversary’s and more vulnerable (Rid Citation2020).
In Whaley’s (Citation1982) classic treatise, deception involves both hiding the real and showing the false. Hence, some but not all disinformation strategies misinform. As Arce (Citation2023) points out, disinformation also involves showing the real and hiding the false. Consequently, a situation of asymmetric information exists whereby the Source of the information knows whether it is true or false but the Target does not. A second dimension of disinformation is whether the Source shares (reveals) its identity when disseminating information or instead hides its identity. In the present analysis, all information, whether true or false, is shared, but the source may or may not share its identity. Hence, the term ‘reveal’ denotes a source sharing its identity, with ‘hide’ denoting its complement. This leads to the disinformation strategies listed in and investigated here within.
Table 1. Disinformation strategies.
Among these strategies, lying is an example of false information stemming from a revealed Source. For example, in response to the #SyrianGasAttack Twitter hashtag documenting Syrian fighter jets dropping nerve gas on the town Khan Al Shekhoun in 2017, Russian and Syrian state actors attempted to neutralize international outrage through the false #SyrianHoax hashtag (Cunningham Citation2020). As another example, the conventional wisdom is someone lied about the status of WMDs in Iraq prior to the 2003 invasion by the U.S.-led coalition of the willing.Footnote2
Leaks are instead true information from a revealed Source. Whistleblowing is an example. ‘Doxing’ – online leaking – is another. In contrast to the other four categories, leaks can often be detrimental to Source and beneficial to the Target. In considering the leaking strategy, it need not be the case that the original owner of leaked information is the entity doing the leaking. Instead, the leaking strategy can correspond to the information owner being sufficiently lax in its practices that leaks are inevitable, as was the case with how Chelsea Manning and Edward Snowden obtained and leaked insufficiently protected classified information (Howard Citation2023). What leaks and lies have in common is the ease of attribution of the original information owner. What differentiates them is the relative ease of confirming the veracity of a leak versus a lie.
By contrast, an intelligence agency practices seeding when it employs a double agent to pass truthful secrets to an adversary.Footnote3 Another example of seeding is when Russia’s main intelligence directorate (GRU) hacked Clinton campaign and DNC documents, initially posting them on front website DCLeaks. Such seeding or conditioning establishes the Source’s bona fides because the information is true. Seeding can draw the Target’s attention away from its current focus.Footnote4 It is a form of influence operation known by Russian intelligence as kompromat: obtaining compromising information and tactically using it to leverage public opinion (Greenberg Citation2019).
Finally, (cyber) smearing occurs when the Source’s identity is hidden or phony and the information is false, such as with Internet troll farms and social network bots. Fake (verifiably untrue) news is an example. Deep fakes are another. As the truth is not necessarily a crucial component to influence and change behavior (Hammond-Errey Citation2019), smears often proliferate in echo chambers catering to fractions of politically polarized groups receptive to the message.
Disinformation traditionally takes place in environments placing a premium on secrecy. The Target of disinformation faces a decision whether to believe information based on its source. In the information age, online disinformation represents a fundamental change affording digital platforms unprecedented influence in terms of the scale and speed of information dispersal as well as the range of content types and platforms in which it is manifest (Culloty and Suiter Citation2021). One result is the effective practice of using troll farms to covertly neutralize social media activists by diverting them off topic via ‘whataboutism’ (Sobolev Citation2022). Another is memetic conflict (conflict based on memes) (Clark and Mitchell Citation2019) where narratives, ideas, public opinion, and personal beliefs are more important than objective facts. Fake and state-run platforms also have advantages over gatekeeping media due to AI and big data capabilities for creating echo chambers.
This study presents a game between an informed Source and uniformed Target whereby lying, leaking, seeding, and smearing all occur, with the effect of the digital revolution also present. An initial contribution is the recognition of two counterpart pairs of disinformation: leaking is the counterpart of lying, and smearing is the counterpart of seeding. Modeling each phenomenon as a strategy acknowledges their intentionality on the part of the Source and the Source’s understanding of their counterpart character, as does the Target.
The resulting equilibrium characterizes the relationship between lying and leaking, seeding and smearing, and the extent true and false information are believed under conditions of incomplete and imperfect information. That is, the Target of disinformation understands the information itself may be true or false and also the information’s source may be hidden. This additionally facilitates characterization of the tradeoff between disseminating false information via credible media (lying) versus echo chambers (smears). Finally, expanding opportunities for hiding the Source in the digital revolution are shown to lie behind Swire’s (Citation2015) concept of declining half-life of secrets, meaning the amount of time that states can keep information secret is declining. This phenomenon is shown to be a product of the link between seeding and smearing. With more interaction, more opportunities to reveal and conceal information arise (Redlinger and Johnston Citation1980), especially as the digital revolution increases interaction via spoofing websites, bot accounts on Twitter, and trolls on Facebook.
Disinformation in Games of Strategy
This section reviews game-theoretic analyses explicitly addressing disinformation and also serves to delineate the present analysis from preceding ones. Ostensibly, all game-theoretic analyses of lying, cheap talk, signaling, signal jamming, disclosure, persuasion, and so on, are about disinformation. To avoid overgeneralizing, the focus is on game-theoretic analyses that either explicitly identify disinformation as the specific subject matter under study or are cast within Whaley’s (Citation1982) typology of deception. Also, Phillips and Pohl (Citation2021) document that a large time gap exists between analyses of U.S. and Soviet disinformation (dezinformatsiya) during the closing stages of the Cold War and more recent qualitative studies comparing and contrasting that era with new tactics of disinformation in the digital age (e.g. Clark and Mitchell Citation2019; Culloty and Suiter Citation2021; Rid Citation2020).
Related theoretical work includes Greenberg (Citation1982), who outlines four potential consequences stemming from the use of deception in situations of strategic decision-making. First, deception may result in secrecy, where the Target cannot assign a posterior belief of probability one to the true state of nature (here, whether the information is true or false). Second, it introduces noise; i.e. the Target cannot conclude which strategy the Source follows. In a game-theoretic context, this translates into the Target having imperfect information regarding each Source strategy involving truthful information and its counterpart involving false information. Third, a false signal induces the Target to make a type I or II error with certainty. Fourth, deception produces misperception in that the Target assigns incorrect probabilities to the states of nature.
While giving examples, Greenberg (Citation1982) provides no games where these properties occur in equilibrium. In contradistinction, the equilibrium for the present game exhibits secrecy and extends the concept of noise to Bayesian types in games of incomplete information. In addition, type I and II errors are possible, and occur with positive probability, but neither with probability one. Pragmatically, because it violates prudence, ‘one would expect pure misleading deceptions to obtain rarely because they require a target to be so sure of a false alternative that they stake all on preparing for it’ (Daniel and Herbig Citation1982, 158).
Phillips and Pohl (Citation2021) illustrate how disinformation can produce misperception by a sequence of Bayesian analysts in a target agency. Each analyst receives an imperfect signal about whether a piece of intelligence is genuine or not and observes the conclusions of those analysts coming before them about the nature of the intelligence. Given the conclusions of the first two analysts, three results are possible: (i) a cascade resulting in correct beliefs about the veracity of information; (ii) an incorrect cascade producing misperception, thereby resulting in a type I or II error; and (iii) oscillations where no cascade occurs. Phillips and Pohl’s (Citation2021) point is all three are consequences of applying Bayes’ rule correctly, with the culprit being low signal quality rather than purposeful deception. In the present study, Bayesian beliefs can lead to type I or II actions, which also have payoff consequences. This facilitates an equilibrium analysis involving beliefs, actions, and payoffs based on the four potentialities in as compared to low signal quality. Nevertheless, the analysis provides another motivation for generating accurate priors (intelligence) because in equilibrium the Target cannot increase accuracy by updating its priors in a costless way via Bayes’ rule since the Source’s actions neutralize the updating process.
More broadly, the game differs from the structure of two canonical signaling games in economics, where one type never sends a false signal. Specifically, in the Beer-Quiche game, in equilibrium strong types never eat quiche; and in education signaling, high productivity types never act as low productivity types. Pooling or semi-pooling occurs on one type only. By contrast, the equilibrium here involves all permutations of {true, false} information types with {revealing, hiding} strategies by the Source. In addition, the Target’s goal is to correctly assess the information’s veracity, but it faces different negative consequences for type I versus type II errors. In contrast, for Beer-Quiche the Receiver earns the same negative payoff for fighting a weak Sender type as it does for not fighting a strong type, and in education signaling the Receiver instead benefits if it hires high productivity types at the lower wage, implying there is no penalty for such an error in the Receiver’s payoff structure. Consequently, in equilibrium, a high productivity type never accepts a low wage offer. Here instead, type I and II errors have negative but differing consequences. There is a difference between being erroneously accepting and erroneously excepting.
The analysis is more in line with models of signaling in analyses of conflict such as when militant or fundamentalist terrorist types act as their policy- or concessions-driven counterparts and vice-versa (Arce and Sandler Citation2007). In Jelnov (Citation2018), weak and strong nonstate actors can pool on not attacking the state or attacking it, with the latter potentially inducing state countermeasures that outsiders judge as disproportionally excessive. Multiple pooling equilibria also occur in Gleditsch et al. (Citation2018), where different government types pool on either signing or not signing a humanitarian convention, such as proscribing land mines, when in conflict with a nonstate actor that either signs or does not sign a similar convention.
The difference here is, no separating equilibria occur. In separating equilibria, a situation of ex-ante incomplete information becomes one of ex-post complete (and accurate) information, which violates the intent of disinformation.
Finally, disinformation is inexpensive relative to other forms of counterintelligence. ‘It is neither labor-intensive nor capital-intensive. It is among the least expensive types of modern intelligence work yet yields a high return for a relatively small amount of investment’ (Handel Citation1982, 143). Consequently, rather than introducing a signaling cost parameter, each of the four strategies of disinformation is evaluated in terms of its opportunity cost; i.e. relative to the strategy not chosen. Extensive form games such as the one specified below lend themselves to this form of counterfactual analysis. The situation is not one of cheap talk either because the preferences of the Source are not biased in one direction vis-à-vis the Target’s preferences.
Specifying the Disinformation Game
depicts the disinformation game consistent with the preceding discussion. There are three players in the game: Nature, the Source, and the Target; with only the Source and Target having payoffs. Hence, at the initial node in , Nature determines whether the information is true (T) with probability
or false (F) with probability
This captures the Target’s incomplete information about the validity of the information the Source attempts to convey. The Source knows its type, corresponding to the validity of its information: true (T-type) or false (F-type), represented by the two nodes immediately succeeding node
Information Structure
The information structure takes this form owing to the following considerations. To begin, the narrowest interpretation of a game with incomplete information (Bayesian game) is that at least one player is uncertain about how their actions affect another’s payoffs. This interpretation is satisfied in that the Target does not know if information is true (T) or false (F); hence, it does not know what the Source’s payoff will be if the Target believes the Source. This is similarly the case if the Target does not believe the Source. As adopted, however, the incomplete information structure is meant to represent more than this. In particular, it also captures an environment where the Target has known unknowns – things the Target knows it does not know.
Specifically, the Target knows the potential states of the world but does not know whether they are true or false. The Target forms priors and
about these potentialities based on its intelligence. The Source knows the potentialities as well and Nature determines whether the Source knows a potential state in the Target’s worldview is actually true or false. In this way, mere ignorance or incorrect guesses do not qualify as disinformation (Freelon and Wells Citation2020). Instead, disinformation requires a strong degree of confidence in knowing the state of nature, which can be a belief held by the Target is factually false (Kuklinski et al. Citation1998). Hence, it is an incomplete information environment in which only the Source knows whether the information is true or false. This is a broader interpretation of a Bayesian game, one in which at least one player is uncertain about the other player’s knowledge. To wit, ‘counterintelligence is to intelligence as epistemology is to philosophy’ (Powers Citation1979, 328).
The knowledge-based interpretation is also consistent with incomplete information generating a point in time when some players already have private information about the game that other players do not know. Nature chooses from a number of possible games to be played, with the probabilities given by the priors. Here, the games correspond to whether the information is true or false. The Source knows the true state/game. Since Nature, rather than the Source, determines the game, the Source does not need to consider a counterfactual corresponding to a state of information it did not create. In contrast, if the Source creates false information, without a move by Nature the extensive form requires the Source to form an ex-ante plan for what it would hypothetically do if it instead creates true information. The Bayesian approach avoids this pretense. The Source can – but need not – have a say as to whether the state is true or false. This allows for Target uncertainty about the Source’s information and how the Source arrives at knowing the state.
Alternatively, this information structure also allows for a population of types, with Nature deciding who will actually play. In this approach, there are Source types with true information and Source types with false information. The difference is as follows. For the case where Nature determines the Source’s knowledge about whether a potential state of the world is true or false, the Source’s strategies are contingent upon its type. For the case where Nature instead decides whether the state of the world is a Source type with true information versus a Source type with false information, the Source decides on their strategies given their type.
Strategies
Once again, Nature determines whether the information is true (T) or false (F) The Source knows its type, corresponding to the validity of its information: true (T-type) or false (F-type), represented by the two nodes immediately succeeding node
At these nodes the Source either attempts to reveal its information through legitimate means, such as reputable gatekeeping media, by leaking (L) or lying (
), or hides itself as the Source and seeds (S) or smears (s) via spoofing websites, botnets posing as users on social media, Internet troll farms, and so on.
The Target has four decision nodes, corresponding to a leaking Source, a lying Source, a seeding Source, and a smearing Source. Nodes in the same information set are joined by a dotted line, indicating the strategies of disinformation create imperfect information (noise) about their counterparts. Hence, leaks and lies are in the same information set, denoted as in , and seeding and smearing are in the same information set, denoted as
The information sets represent the Target understanding the difference between receiving information from gatekeeping media,
versus alternative platforms without a gatekeeper’s legal obligations or reputation for fact-checking,
. That is, the Source’s strategies are not simply messages (signals or cheap talk); the medium of communication varies as well.
It follows that, given the Source controls the medium when either seeding or smearing, strategies and s reach information set
with certainty. By contrast, leaking and lying pass through gatekeeping media and may not pass the fact-checking process. Given true information is more likely to pass fact-checking than false information, the likelihood of reaching information set
is greater for leaks than lies. Furthermore, at the margin what matters is the relative probability that leaking versus lying reaches
, rather than their absolute magnitudes. Hence, leaks are modeled as reaching information set
with probability one and lies pass gatekeeping to
with probability
, where
This is represented by a gatekeeping node,
which is a move by Nature where a lie (
) reaches information set
with probability
The lie is unsuccessful with probability
thereby capturing the relative difference between leaking and lying. At information set
the Target makes a decision to believe the information (B) or not (N). It similarly believes (b) or not (n) at information set
.
Purposefully provocative examples of this information structure and strategies are given in . In all cases, the state of the world was a subjective possibility for the Target at the time of the disinformation campaign, the Source knew the veracity of the state of nature, and the Source’s identity was either revealed or hidden at the time of the event. For example, members of the U.S. military visited China for the World Military Games in Wuhan during October 2019 (Verma Citation2020). Similarly, Russia interfered with the 2016 U.S. presidential election and Donald Trump won the election. Indeed, during the election Russia hid its identity on front website DCLeaks and posted true information it obtained by hacking the Democratic National Committee (DNC). By contrast, principal sources for the Steele Dossier, who did not have insider knowledge of Russian election interference or the Trump campaign, have subsequently testified under oath that the information was rumor and speculation, thereby characterizing its veracity. Both the Panama papers, which are 11.5 million documents from Panamanian offshore law firm Mossack Fonseca published by the International Consortium of Investigative Journalists (ICIJ), and the Steele Dossier successfully passed through independent gatekeeping media. Furthermore, the Panama Papers illustrate it need not be the source itself (Mossack Fonseca) doing the leaking but instead operates in an environment whereby information is obtained and leaked by a third party. By contrast, China’s false narrative on the U.S. origins of COVID passed through state-controlled press and television. A preponderance of Targets initially believed the first three examples in , with very few outside of China believing the final example. The next subsection delineates the associated incentives (payoffs) for doing so.
Table 2. Examples.
Payoffs
Payoffs are expressed with the Source’s payoff listed on top at each terminal node and the Target’s payoff listed beneath. The Target’s payoffs are denoted by Greek letters. The Target’s goal is to be correct. It achieves this payoff goal, if (i) it believes true information, (ii) it does not believe false information, or (iii) the gatekeeper identifies a lie. Errors in belief are costly for the Target. A type I error, not believing the Source’s true information, yields a payoff of
Implicitly, the Target does not act on the information when acting is to the Source’s disadvantage and the Target’s advantage. A type II error, believing the Source’s false information, yields a payoff of
Implicitly, the Target acts on the information when not acting is to the Source’s disadvantage and the Target’s advantage. If one approaches the value of intelligence as creating complete and perfect information in the disinformation game, then
can be viewed as the Target’s ex-post regret for not believing true information and
its ex-post regret for believing false information.
The Source’s goal is operational control over the means by which their information – true or false – is believed. ‘The main interpretative assumption of the term disinformation is its intentionality’ (Krzak Citation2022, 266). For true information, seeding (S) involves Source control by hiding the provenance of information. By contrast, leaking (L) implies less operational control. In an environment where secrecy is at a premium, a believing Target (B) at the leak node has negative consequences for the Source. As such, modeling leaking as a strategy allows for a theory of rational leaking where leaking has a potential downside in the payoff dimension, but an upside in inducing imperfect information about its counterpart, lying. When information is false, lying () is an attempt to manipulate gatekeeping media into legitimizing false information, which is preferred to hiding the Source of false information via smearing (
).
The Source’s payoffs are denoted by Latin letters with a hat over the letter for F-types. Payoffs for each type of Source are unique up to a positive affine transformation. The T-type’s payoffs are defined relative to the baseline outcome where a leak is not believed, with a normalized payoff of zero for the Source. Payoffs relative to this point reflect the Source’s intentionality and whether it achieves its goals via manipulation. For example, when a leak is believed, the T-type receives its lowest payoff, -L < 0, because it did not control the release of information and does not desire the leak. ‘A leak is analogous to radioactive decay – there is a potentially toxic effect when the secret leaves its previous location’ (Swire Citation2015, 3). At the same time, without leaks there would be no uncertainty at information set and therefore no reason to believe any lies. By comparison, if a T-type both controls the flow of information through strategy S, leading to the seeding node, and the information is believed, it achieves its goal, receiving payoff G > 0. When inducing the seeding node and the information is not believed, the Source is unsuccessful, receiving payoff -U < 0, where -U > -L.
The F-type’s baseline payoff of zero occurs when a smear is believed. Here, false information is believed, but transmitted via a less-preferred means: s instead of . The F-type’s greatest success is when a lie,
, is believed, with payoff
When an F-type’s lie or smear is not believed to be unsuccessful, with a payoff of -
. An F-type’s lie is also unsuccessful when it does not pass through gatekeeping media at node
, which occurs with probability
.
The payoffs have two additional characteristics of note. First, the game differs from cheap talk analyses where, as compared to the Receiver’s preferences, the Sender’s preferences are biased (shifted) in one direction only. Second, payoffs are not monotonic in L or S for the T-type. They are weakly monotonic (in ) for the F-type. These properties feed into the Target’s beliefs and best responses to the Source’s messages, as characterized in the following section.
Equilibrium
This game has no separating equilibrium. In a separating equilibrium, each Source type, T and F, rationally select strategies leading to different information sets. Hence, the situation becomes one of ex-post complete information because the Target knows which Source type acted to induce each information set. As the Target’s goal is to be right, it believes at the information set induced by the T-type and does not believe at the information set induced by the F-type. But if the Target is believing with probability one at the information set induced by the T-type, the F-type prefers instead to induce that information set as well. This then contradicts the property that the T- and F-types prefer to induce different information sets in a separating equilibrium. A separating equilibrium is orthogonal to disinformation’s purpose of creating strategic uncertainty.
If both Source types instead pool on pure strategies inducing the same information set: counterparts L and induce
or counterparts S and s induce
, then the Target ‘mixes’ over its response at that information set because it does not know which type induced it. As sequential rationality in extensive-form games is evaluated in terms of local strategies at each information set, the term ‘mix’ is used throughout to refer to the Target’s likelihood of selecting local strategy B versus N at
and b versus n at
, rather than a distribution over (B, b), (B, n), (N, b) and (N, n). Given mixing in local strategies is the optimal response to pooling on either L and
or S and s, the Target mixes at both information sets. If the Target mixes, then each Source type does so as well. Just as bluffing occurs in poker, a T-type Source does not always play strategy S nor does an F-type Source always play strategy
. Unlike bluffing in poker, where the value of a hand (type) is rank-ordered, there is no reason to assume T trumps F or vice-versa. Disinformation is different than bluffing but similarly requires strategic indeterminacy to maintain secrecy.
Let denote a strictly mixed local strategy corresponding to the likelihood pure local strategy ‘
’ occurs under the corresponding circumstances, where
for a T-type Source,
for an F-type Source,
for the Target at information set
, and
for the Target at information set
. Furthermore, the Target formulates the posterior belief it is at node j,
such that
at information set
and
at information set
.
The distinction between the Target’s posterior belief that information is true at a given information set, and
, and the strategy of believing at that information set,
and
is that posteriors are largely derived mechanically via Bayes’ rule whereas strategies are selected using the posteriors to generate expected payoffs and best replies based on the incentives of both Source and Target. Upon calculating posteriors, within the intelligence community information is characterized as conceivable/remote if it is a low-probability event; it is possible/unlikely if the chance of it happening is less than 50%; its chances are 50:50; it is likely if its probability is in the 60–70% range, and at 80% or above is almost likely (Safire Citation1995, Kaplan Citation2012). Given such beliefs, the Target must ultimately decide whether or not to act on the information; i.e. a decision is made or a strategy is selected.
Furthermore, an alternative perspective to and
being the likelihood the Target believes at the respective information set is given by Nash’s (Citation1950) mass action interpretation of mixed strategies. Specifically,
and
represent a cross-sectional distribution of Targets – plural – that believe at the information set. In applied game theory, this interpretation of a mixture as a population distribution is initially taken up by Cornell and Roll (Citation1981), and, more recently, by Arce (Citation2018). Here, the mass action interpretation facilitates the identification of echo chambers for a subpopulation of Targets. Consequently, those with similar beliefs (posteriors) about the state of nature can come to alternative conclusions (strategies). In this way, in equilibrium both type I and type II errors occur, but neither with probability one. From the mass action perspective, disinformation alters Abraham Lincoln’s adage: you can fool all people some of the time and some people all of the time. But you can never fool all people all of the time, to: you can fool some people some of the time, but never all people all of the time.
An assessment is a perfect Bayesian equilibrium (PBE) if (i) the strategies are best replies at their respective information sets given the equilibrium beliefs, and (ii) the beliefs supporting strategies at an information set are consistent in they correspond to Bayes’ rule as derived from the preceding equilibrium strategies and moves by Nature.Footnote5 Derivation of the PBE occurs in the Appendix. Its characterization is given and discussed below.
Property 1 (Disinformation neutralizes Bayesian updating):
Ex-ante disinformation is noisy – creates imperfect information – in that counterpart strategies leaking and lying lead to information set and seeding and smearing lead to information set
. Property 1 extends noisiness to the Bayesian context: the Target cannot tell what strategy is followed by a given Source’s type. The Source conducts its disinformation campaign so the Target assigns the same probability that information is truthful at information set
as it does at
:
Similarly, the Target’s posterior beliefs assign the same probability to false information at
as it does at
:
In this way, disinformation neutralizes the updating process. Ex-post it is as if two additional information sets are created; one containing the leaking and seeding nodes, and another containing the lying and smearing nodes.
Neutralization of Bayesian updating places a premium on the Target’s intelligence for forming priors rather than evaluating information upon disclosure. The Target must expend resources to increase certainty instead of costlessly doing so via Bayesian updating. It also places a premium on the Source’s counterintelligence for understanding what information is epistemically plausible for the Target. The onus falls on the Source to know what disinformation – regardless of whether it is true or false – fits within the Target’s accepted set of plausible worldviews. As in Arquilla and Ronfeldt’s (Citation1993) version of netwar-as-disinformation, disinformation requires the Source to know everything about the Target and keep the Target from knowing much about the Source.
A novel consequence is even though beliefs about the Source’s type are the same at each information set (neutralization), strategies subject to gatekeeping (L and ) are believed to a different degree than strategies not subject to gatekeeping (S and s); i.e.
:
Property 2 (The medium is the message): Believing the Source is media-specific, and
. Furthermore, the Source is believed less when lying or leaking
than when seeding or smearing
,
, when
.
Given , the medium is, indeed, the message. The likelihood the Target believes gatekeeping media differs from believing a potentially phony outlet. Moreover, it is possible that the likelihood gatekeeping media is believed is less than the likelihood a potentially phony outlet is believed,
. From a historical perspective, this property identifies conditions in agreement with the CIA’s finding that disinformation works best when phony outlets carry factual content; i.e. seeding.
In addition, Property 2 introduces the first of two ‘too-good-to-be-true’ characterizations of the equilibrium. A large lie that is believed implies a large value for Source payoff This potentially decreases the value of
such that condition
holds for even the most vigilant gatekeeper with a low value of
. In such circumstances the Target cannot rely on gatekeeping media to do its work for it. Accordingly,
because at gatekeeping information set
the lie is too-good-to-be-true.
The conditions leading to inequality create an environment placing a premium on the medium in terms of hiding versus revealing the Source’s identity. A prominent example is Russia’s use of DCLeaks as a front website for releasing Clinton campaign and DNC documents because the potential for the subpopulation of Americans targeted to believe this information in the form of a seed,
, is greater than that for believing the information in the form of a leak,
such as Russia leaking the information through a reputable gatekeeper like the New York Times. Also, under these conditions, the Source is believed less when lying than when smearing. This provides the incentive to capitalize on advances in computing power, artificial intelligence, and machine learning to increase the use of echo chambers and deep fakes.
As echo chambers require more than one Target, Nash’s (Citation1950) mass action interpretation of a cross-sectional subpopulation of believing Targets, applies here. Within this context, the emergence of fake news echo chambers to further polarize political groups is explained by the greater proportion of believing Targets at the information set containing the smear node. False information is not nearly as challenged when it stems from false Sources.Footnote6 The tendency for Targets to believe a less-reputable Source hiding its identity at the
information set is the echo chamber effect. Moreover, it is the occurrence of seeding that creates both imperfect information at
and provides the incentive for believing at
Hence, as the digital revolution facilitates cyber smearing, it is accompanied by a loss of secrets due to its counterpart strategy, seeding. In other words, the declining half-life of secrets and the echo chamber effect are linked because the former is a product of seeding and the latter a product of smearing. Each strategy needs the other in order for both to lead to believing Targets, and the digital revolution facilitates both strategies.
The equilibrium is also consistent with a theory of rational leaks because the payoffs for believing at the leaking node occur with positive probability. Indeed, leaks occur without the need to specify divided loyalties within the Source organization. Here, a believed leak is an unambiguous cost to the Source. The policy implication is practitioners of disinformation must attune themselves to the occurrence of leaks. A tight coupling exists between lies and leaks, as characterized by the following two properties.
Property 3 (Lying, is tied to leaking,
):
Without leaks there can be no lies: . Furthermore, the more the Source leaks the more it can lie and the less the Source leaks the less it can lie. The degree to which lies and leaks are believed is characterized by another ‘too-good-to-be-true’ property:
Property 4 (Credibility of leaks): The Target’s willingness to believe the Source decreases in the Source’s cost of a leak, L:
and
.
This property is consistent with evidence suggesting the bigger the leak, , the less likely the Target believes it (because it is too-good-to-be-true), and, with Property 2, deceptive leaks (seeding) are more likely to be believed than actual leaks (Daniel and Herbig Citation1982). Both leaks and lies can be too-good-to-be-true. At the same time, not only are leaks the counterpart of lies, the cost of a leak carries over to the believability of seeding and smearing,
. Hence, one cannot meaningfully analyze a disinformation phenomenon such as fake news (smearing) in isolation, or in restricted conjunction with its counterpart (seeding), owing to the spillover from leaking.
Conclusion
This study considers the relationship between four strategies of disinformation: leaking, lying, seeding, and smearing. Here, lying and leaking refer to the source disseminating disinformation through traditional gatekeeping media having legal obligations or reputations for fact-checking and vetting its sources. Consequently, lying and leaking have an equilibrium relationship. In contradistinction, seeding and smearing involve phenomena such as using Internet trolls or botnets on social media, deep fakes, or spoofing websites to facilitate keeping the source of disinformation hidden. In equilibrium, conditions exist such that seeding and smearing strategies are believed more than leaking and lying strategies. As the digital revolution facilitates seeding and smearing, this explains both increases in social-media-as-disinformation and the declining half-life of secrets through seeding. An example is the GRU’s use of false-flag ‘ISIS’ website CyberCaliphate to release hard-to-find and stolen documents from the U.S. Department of Defense.
Overall, disinformation is characterized as neutralizing, meaning the Target’s posterior probability that information is true is the same irrespective of whether the Source reveals or hides its identity. This also holds for false information. The policy implication for the Target is its intelligence must focus on generating accurate priors rather than relying on the less costly process of updating priors upon the release of disinformation. It also cannot rely on gatekeeping media to do its work for it. Consequently, any policy to address one form of disinformation, such as fake news, must be considered within the context of a disinformation campaign involving all four types of disinformation as possibilities. The policy implication for the Source of disinformation is its counterintelligence must focus on epistemically understanding the Target’s plausible worldview and using this worldview to generate disinformation.
Finally, both leaks and lies can be too-good-to-be-true in that they are believed less than seeds or smears even though leaks and lies pass through gatekeeping media whereas the Source has greater control over the (digital) outlets for seeding and smearing. The combination of greater likelihood of believing smears and seeds with the way these strategies are facilitated by the digital revolution is shown to lead to two modern consequences of disinformation: echo chambers and the declining half-life of secrets.
Acknowledgments
I thank participants in the 2023 ICES conference, particularly Ugurhan Berkok, Sam Ransbotham, and two anonymous referees for comments.
Disclosure statement
No potential conflict of interest was reported by the author(s).
Notes
2. Arce (Citation2023) identifies several possibilities: Saddam Hussein purposefully maintained ambiguity to keep his enemies off guard; an Iraqi human intelligence source lied to foreign intelligence handlers; Saddam destroyed the evidence that he destroyed WMDs because he denied having them in the first place; U.S. intelligence lied to U.S. decision makers; the U.S. lied to the UN.
3. Grant (Citation2021) examines the decision to become a double agent and Sources’ decisions to trust double agents. This interesting phenomenon involving two-sided incomplete information lies outside the scope of the present paper.
4. Krzak (Citation2022) calls such seeding apagogic disinformation in that it uses the truth as a form of proof by contradiction.
5. As all information sets are reached in equilibrium, beliefs at probability zero information sets do not arise.
6. Balcaen et al. (Citation2023) find that the likelihood of belief increases if the false information is framed as containing ‘scientific evidence’ as opposed to conspiracy theories.
References
- Arce, D. 2018. “Malware and Market Share.” Journal of Cybersecurity 4 (1): 1–6. https://doi.org/10.1093/cybsec/tyy010.
- Arce, D. 2023. “Cybersecurity for Defense Economists.” Defence and Peace Economics 34 (5): 705–725. https://doi.org/10.1080/10242694.2022.2138122.
- Arce, D., and T. Sandler. 2007. “Terrorist Signaling and the Value of Intelligence.” British Journal of Political Science 37 (4): 573–586. https://doi.org/10.1017/S0007123407000324.
- Arquilla, J., and D. Ronfeldt. 1993. “Cyberwar is Coming!” Comparative Strategy 12 (2): 141–165. https://doi.org/10.1080/01495939308402915.
- Balcaen, P., C. Buts, C. du Bois, and L. Tkacheva. 2023. “The Effect of Disinformation About COVID-19 on Consumer Confidence: Insights from a Survey Experiment.” Journal of Behavioral and Experimental Economics 102:101698. https://doi.org/10.1016/j.socec.2022.101968.
- Clark, R. M., and W. L. Mitchell. 2019. Deception, Counterdeception and Counterintelligence. Thousand Oaks, CA: CQ Press.
- Cornell, B., and R. Roll. 1981. “Strategies for Pairwise Competitions in Markets and Organizations.” Bell Journal of Economics 12 (1): 201–213. https://doi.org/10.2307/3003517.
- Culloty, E., and J. Suiter. 2021. Disinformation and Manipulation in Digital Media. New York: Routledge.
- Cunningham, C. 2020. Cyber Warfare – Truth, Tactics, and Strategies. Birmingham, UK: Packt.
- Daniel, D. C., and K. L. Herbig. 1982. “Propositions on Military Deception.” In Military Deception and Strategic Surprise, edited by J. Gooch, 155–177. London: Routledge.
- Freelon, D., and C. Wells. 2020. “Disinformation as Political Communication.” Political Communication 37 (2): 145–156. https://doi.org/10.1080/10584609.2020.1723755.
- Gleditsch, K. S., S. Hug, L. I. Schubiger, and J. Wucherpfennig. 2018. “International Conventions and Nonstate Actors: Selection, Signaling, and Reputation Effects.” Journal of Conflict Resolution 62 (2): 346–380. https://doi.org/10.1177/0022002716650924.
- Grant, W. C. 2021. “Trusting a Double Agent.” Defense and Peace Economics 32 (8): 941–955. https://doi.org/10.1080/10242694.2020.1800896.
- Greenberg, A. 2019. Sandworm. A New Era of Cyberwar and the Hunt for the Kremlin’s Most Dangerous Hackers. New York: Doubleday.
- Greenberg, I. 1982. “The Role of Deception in Decision Theory.” Journal of Conflict Resolution 26 (1): 139–156. https://doi.org/10.1177/0022002782026001005.
- Hammond-Errey, M. 2019. “Understanding and Assessing Information Influence and Foreign Interference.” Journal of Information Warfare 18 (1): 1–22.
- Handel, M. I. 1982. “Intelligence and Deception.” In Military Deception and Strategic Surprise, edited by J. Gooch, 122–154. London: Routledge.
- Howard, R. 2023. Cybersecurity First Principles. Hoboken, NJ: Wiley.
- Jelnov, A. 2018. “Proportional Use of Force in Asymmetric Military Operation.” Defense and Peace Economics 29 (6): 648–657.
- Kaplan, E. H. 2012. “OR Forum – Intelligence Operations Research: The 2010 Philip McCord Morse Lecture.” Operations Research 60 (6): 1297–1309. https://doi.org/10.1287/opre.1120.1059.
- Krzak, A. 2022. “Operational Disinformation of Soviet Counterintelligence During the Cold War.” International Journal of Intelligence & CounterIntelligence 35 (2): 265–278. https://doi.org/10.1080/08850607.2021.2014280.
- Kuklinski, J. H., P. J. Quirk, D. W. Schwieder, and R. F. Rich. 1998. “’just the Facts, Ma’am’: Political Facts and Public Opinion.” ANNALS of the American Academy of Political and Social Science 560 (1): 143–154. https://doi.org/10.1177/0002716298560001011.
- Nash, J. 1950. “Non-Cooperative Games. PhD Dissertation, Princeton University Department of Mathematics.” In The Essential John Nash, edited by H. W. Kuhn and S. Nasar, 53–84. Princeton, NJ: Princeton University Press.
- Phillips, P. J., and G. Pohl. 2021. “Disinformation Cascades, Espionage & Counter-Intelligence.” The International Journal of Intelligence, Security, and Public Affairs 23 (1): 34–47. https://doi.org/10.1080/23800992.2020.1834311.
- Powers, T. 1979. The Man Who Kept the Secrets. NY: Knopf.
- Redlinger, L. J., and S. Johnston. 1980. “Secrecy, Informational Uncertainty, and Social Control.” Journal of Contemporary Ethnography 8 (4): 387–397. https://doi.org/10.1177/089124168000800401.
- Rid, T. 2020. Active Measures. The Secret History of Disinformation and Political Warfare. New York: Farrar, Straus and Grioux.
- Safire, W. 1995. “On Language: Spookspeak in Deutchland.” New York Times Magazine, 19 November.
- Saunders, F. S. 1999. Who Paid the Piper? The CIA and the Cultural Cold War. London: Granta Publications.
- Sobolev, A. 2022. “How Pro-Government ‘Trolls’ Influence Online Conversations in Russia.” Accessed July 20, 2023. www.wpsanet.org/papers/docs/2019W-Feb-Anton-Sobolev-Trolls-VA.pdf.
- Swire, P. July 2015. “The Declining Half-Life of Secrets.” New America Cybersecurity. 1:2–11.
- Verma, R. 2020. “China’s Diplomacy and Changing the COVID-19 Narrative.” International Journal: Canada’s Journal of Global Policy Analysis 75 (2): 248–258. https://doi.org/10.1177/0020702020930054.
- Whaley, B. 1982. “Toward a General Theory of Deception.” Journal of Strategic Studies 5 (1): 178–192. https://doi.org/10.1080/01402398208437106.
Appendix
Denoting E[⋅] as the (conditional) expected payoff for a player or player type, sequential rationality requires E[B] = E[N] for the Target at . That is,
Simplifying,
Bayes’ rule and consistency require
Similarly, E[b] = E[n] for the Target at . That is,
Simplifying,
Bayes’ rule and consistency require
(A1) and (A3) establish Property 1.▄
Combining the consistency conditions in (A2) and (A4):
which holds when . This is Property 3.▄
For type T, sequential rationality requires E[L] = E[S]; i.e.
In order for the following condition must be met
which itself implies
For type F, E[] = E[
]; i.e.,
implying
This is Property 2. Moreover, inequality holds when
; i.e.,
.▄
Together, (A5) and (A7) imply Solving for
It is straightforward to check that (A6) holds. Finally, combining (A7) and (A8) implies
From (A8) and (A9), both and
are decreasing in
. This is Property 4.▄