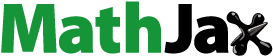
ABSTRACT
Energy plays critical direct and indirect roles in the livelihoods of human beings. Despite its relevance, the literature highlights that a majority of rural households are energy insecure and very much rely on unclean energy sources that compromise their health. Against this background, this paper estimates the determinants of energy security at the household level based on evidence from Melani-inland and Hamburg-coastal communities in the rural Eastern Cape Province of South Africa. The study used the multidimensional energy poverty index (MEPI) to calculate the energy security status of households in the two communities while the Tobit regression model was used to investigate the main factors affecting household energy security. The results indicated that in both communities, households were energy secure. However, access to additional energy measures was low, which could affect their energy security if the primary source (ESKOM electricity) is interrupted. Tobit regression results revealed that factors like marital status, household size, electricity affordability and source of income are the most critical factors influencing the energy security status of households in the study areas. Therefore, the paper recommends policy options that improve access of rural households to additional measures on energy and affordability of electricity.
KEYWORDS:
Introduction
Energy is one of the essential natural resources needed for human health and wellbeing. However, despite the Integrated National Electrification Programme (INEP) conducted by the South African government to address energy insecurity, only 80% of the rural population is connected to electricity.Footnote1 In South Africa, the non-electrified households come from low-income backgrounds and therefore are unable to pay for electricity connection.Footnote2 In addition, South African low-income households connected to the electricity grid still cannot afford to pay for electricity to improve their livelihoods.Footnote3 To date, about four million rural households still tend to use fuelwood for heating and cooking in South Africa.Footnote4 Even though countries are moving forward, the idea of decreasing human dependence on energy sources associated with environmental and human sickness, such as wood, paraffin, cow dung,Footnote5 South African rural communities still depend on these alternative sources of energy, for different household activities such as cooking, lighting, and food preservation.Footnote6,Footnote7
Despite the measures taken by the government to address poverty in both urban and rural areas, South Africa still ranks among the countries with the highest poverty levels.Footnote8,Footnote9 Francis and WebsterFootnote10 stated that in South Africa, poverty levels have increased from 53.2% in 2011 to 55.5% in 2015, indicating that about 30.4 million people are living in poverty. The Eastern Cape province of South Africa ranks as one of the provinces with high poverty levels. According to StatsSA,Footnote11 about 67.3% of households in the Eastern Cape are multidimensionally poor. The Daily DispatchFootnote12 attests that about 36% of the population in the Province solely depends on a social grants. As stated in the General Household Survey,Footnote13 energy security can reduce poverty, improve livelihoods, and living standards of households with low levels of income. Studies suggest that access to good, uninterrupted electricity is central to eradicating poverty and unemployment.Footnote14,Footnote15 Furthermore, scholars have argued that increasing access to modern energy will, therefore, reduce human morbidity, while also promoting environmental sustainability.Footnote16,Footnote17
Literature in the South African context has identified a high prevalence of energy insecurity, more specifically in rural and peri-urban areas and informal settlements.Footnote18,Footnote19 Recently,South Africa has also experienced fuel prices and electricity tariffs increases,Footnote20 which have negative implications for households energy access. As a result, households in rural communities of the Eastern Province tend to use cheaper alternatives for their energy needs, such as firewood, coal, and paraffin. These alternatives are considered to be unsafe, especially for low-income households.Footnote21 Thus, this paper argues that understanding the factors influencing household energy security in rural areas is essential for designing suitable evidence-based policies to support the targeted measures in addressing energy poverty in South Africa.
Objectives
The following specific objectives guided the study:
To profile the energy security status of households in the study areas.
To investigate factors influencing energy security in the study areas.
Research methodology
The study area
The Keiskamma catchment in the Eastern Cape Province of South Africa was selected as the research area because it is similar to many other coastal and inland catchments in the Eastern Cape Province. The catchment covers Melani inland and Hamburg coastal communities, as illustrated in .
Hamburg coastal community is a small rural area that falls under the Ngqushwa local municipality. The community is located near the Keiskamma Estuary, where the Keiskamma River runs to the Indian Ocean. Melani terrestrial community is a communal area situated approximately 12 km north of Alice town in the Eastern Cape Province, South Africa. A communal area is an area that is owned by the community and traditional leadership. According to Moyo et al.Footnote22 in a communal area in the Eastern Cape, land tenure varies from communal, quitrent, permission to occupy, and trust. The communal area is located in the Raymond Mhlaba Local Municipality (RMLM), previously known as Nkonkobe Local Municipality (NLM). The study employed a purposive random sampling technique to select the study site and simple random sampling to select the respondents. Guided by previous studies, the Keiskamma catchment was purposively selected to accommodate reported household energy poverty levels in the area. Previous studies have found the catchment to be vulnerable to the unavailability of water, energy, and food resources.Footnote23,Footnote24,Footnote25,Footnote26
Sampling method and sample size
The study randomly selected 283 households for direct questioning, making use of questionnaires. The study selected 141 households from Melani and 142 from Hamburg to make up a total of 283 households. The sample size was derived from equation one, following Israel.Footnote27 The equation assists in getting a 95% confidence level and a proportion of 0.5%.Footnote28
(1)
(1) Where n is the sample size, N is the population size, and e is the level of precision. Thus, this formula can be applied with the known number of households, and a margin of error of 5% is:
According to the former Nkonkobe Municipality,Footnote29 Melani community has a population of 500 households, and Hamburg has a total population of 454 households,Footnote30 which makes a combination of 945 households. However, there might be a possibility of population growth or shrinkage between the indicated years and to date. Thus, to obtain a 95% confidence level with a 5% error level, 283 households would be the lowest accepted number.
Theoretical framework
The study employed a sustainable livelihoods framework to help understand and analyse the livelihoods of the poor.Footnote31 This framework is useful when considering factors and activities influencing poor households that make a living for themselves.Footnote32 As noted in Scoones,Footnote33 livelihoods are considered sustainable when individuals can cope with and recover from shocks and stresses and must be able to maintain their assets while also considering the natural resources. Donohue and BiggsFootnote34 argued that the sustainable livelihood approach assumes that poverty is not just based on the shortfall of income but also looks at the multidimensional concept which needs aspects such as education affordability and health, among others. Further, this multidimensional framework has its focus on household level and assets that the household controls, which are influenced by external factors and shocks.Footnote35 The sustainable livelihood approach provides measures to link socio-economic and environmental concerns.Footnote36 Thus, this framework can be used to understand significant factors that influence the household's ability to change their livelihoods.Footnote37
Rendering Chambers and Conway,Footnote38 households are comprised of financial, social, physical, human, and natural capital. According to Marten,Footnote39 financial capital is defined as the availability of stocks, including savings, pension, and wage income. Social capital is defined as the relationships and networks to enable people to co-operate. Physical capital is mainly the essential infrastructure humans depend on for their livelihoods such as water supply and sanitation. Human capital is defined as the skills and ability of people to work to pursue different livelihood strategies, while natural capital is defined as the natural resources that sustain life (energy, water, and food). Energy security is one of the natural resources that assist in the livelihoods of rural households. Against this background, the sustainable livelihoods approach was used to link the socio-economic factors influencing the energy security of households, which is one of their livelihood drivers. By so doing, this paper describes the energy security status and looks at the drivers of energy security for rural households in the study area.
Method of data analysis
Measuring energy security
A multidimensional energy poverty index (MEPI) was calculated to formulate the energy security status of households in each on the study sites. The MEPI consists of three broad categories of energy use: lighting, cooking, and additional measures.Footnote40 These three categories are given a score of 33.33% each. Thus, depending on the fact that a particular quality indicates energy secure or energy insecure, each category was assigned a value of 0 or 1, respectively. For instance, for lighting, a household not having access to electricity, was coded as 1, while a household with electricity was coded as 0. Under cooking, a household using an electric stove was coded as 0, and a household not having access to an electric stove was coded as 1. Under additional measures, a household using firewood, cow dung, crop residue and coal for cooking, lighting, heating purposes was given a value of 0, and households not using them were given a value of 1.
Following various studies (e.g. Sadath and AcharyFootnote41; Nussbaumer et al.Footnote42; Edoumiekumo et al.Footnote43), the MEPI was represented by the weight of the individual component multiplied by the assigned value. Then the index was obtained by summing up the values across all components. However, MEPI may be vulnerable to weights assigned to different dimensions. Thus, in order to test the sensitivity of the proposed index to the weights used, a sensitivity analysis using different weights, and the ranking of the dimensions, was conducted. Sensitivity was obtained from the rank sum method of assigning weights. The three dimensions of energy poverty, namely cooking, lighting, and additional measures were ordered based on the relative importance of the individual measures. Below is the formula for the weights which were used:
(2)
(2) Where
is the rank of ith objective, and K is the total number of objectives. The energy poverty dimension ranked first will have a weight of 50%, second 33.33%, and third 16.66%. The study used two ordering schemes, with the first being cooking, lighting, and additional measures; and the second was lighting, cooking, and additional measures. If there is more than one indicator in a given dimension, it is equally divided among them. For example, based on the first ordering, cooking has the highest weight of 50%, and it is equally divided by 25% between the two sub-dimensions. Lighting has the weight 33.33%, and additional measures weigh 16.66%, divided among the five sub-dimensions at a rate of 3.33% each. The same method is followed in the second ordering as well. The linear index is interpreted as the closer the household's poverty index is to 0, the lower the energy poverty levels for the household. The closer the household's poverty index is to 100, the higher the levels of energy poverty levels of the household.
Tobit logit regression model
The Tobit regression model is a censoring regression model designed to measure a linear relationship between variables that have either a left or right censoring on the dependent variable.Footnote44 Maziya et al.Footnote45 used a Tobit regression model to measure the relationship between factors influencing household food security amongst smallholder farmers. Mekuria and MekonnenFootnote46 used Tobit regression to estimate the determinants of crop-livestock diversification in the mixed farming system. While earlier, Chebil et al..Footnote47 also used Tobit regression to look at the association between the economic efficiency measure and its determinants for irrigated wheat farms in Tunisia. Given that the nature of the dependent variable is positively censored (MEPI), this study used the Tobit regression model to analyse the determinants of energy security.
The Tobit regression model is estimated as illustrated in equation 3Footnote48;
(3)
(3) Where
MEPI i:
the constant term in the model; β = a vector of the variable coefficients; and
= error term. The Tobit regression model was considered appropriate as it takes account of the continuous but truncated nature of the dependent variable (min=0; max=100). The Akaike's Information Criterion (AIC) and the Bayesian Information Criterion (BIC) were used to compare the model's suitability to simple linear regression.
Variables
Dependent variable
MEPI is a continuous variable measured as a percentage, which consists of three broad categories of energy use: lighting, cooking, and additional measures.
Independent variables and literature
Household income is one of the significant factors contributing to energy security at the household level.Footnote49 In general, households with high incomes are more energy secureFootnote50 than low earning households. However, in some rural areas of South Africa, energy in the form of wood is freely available, and households can access it at all times.Footnote51,Footnote52 Against this background, the influence of income on energy security depends on the area and type of energy source.
Marital status also contributes to the energy security of households in one way or another. For instance, households with a married couple or who are committed in their relationship are more likely to have access to energy than those who are single. The reason is that in households with a married couple, the couple combines their income when buying energy and also share household expenses.Footnote53 In the event of a married couple with low income and more expenditure, energy poverty might be worse.Footnote54 Besides the income effect, for rural areas where firewood is the main source, maritial stutus may have a positive influence through additional labour force to gather free avialble firewood. Therefore, based on the above uncertainties, the influence of marital status on energy security is not apparent.
Household size contributes to the factors influencing household energy security.Footnote55 Thus, a household with a large number of people is more likely to be energy poor than those with few people staying in one house because the more people that reside in one houshehold, the larger the odds are that the fixed houheold budget must be distributed, therefore increasing energy insecurity. From another angle, large household sizes may positively influence energy security through availability of labour force to gather firewood in rural areas.Footnote56,Footnote57 Therefore, based on the above uncertainties, the influence of household size on energy security is not obvious.
Employment status is one of the main factors which influences energy security. Unlike unemployed households, employed households are likely to have access to good clean energy through the income effect.Footnote58,Footnote59 To the contrary, for a typical rural setting where firewood is the main source of energy, unemployed household heads may have more time to gather firewood than their employed counterparts. Based on the above information, either a positive or negative effect is expected.
Race is considered as one of the significant factors influencing energy security at the household level. More Africans still lack energy security than Whites, Asians, and Indians. This is linked to their earning disparities, where Whites earn about eight times the average per capita income and expenditure compared to Africans.Footnote60,Footnote61 Thus, the study hypothesises that race has a negative association with energy security in the study area due to inequalities in the distribution of resources among different races.
Electricity affordability is one of the significant factors contributing to the factors influencing energy security in rural areas. Njiru and LetemaFootnote62 attested that in rural areas, households tend to spend their limited income on unhealthy sources of energy, mainly because they cannot afford to pay for clean energy sources. Hence, it was hypothesised that electricity affordability and energy security in the study area are inversely related.
The age of the household head is also one of the significant factors contributing to the factors influencing energy security in rural areas. The elderly household heads are more at risk of being energy insecure than the younger household heads.Footnote63 Because there more household head ages, they tend to adopt more unclean sources of energy, therefore leading to energy insecurity.Footnote64 Based on the above information, the study hypothesised that the age of the household head is likely to have a negative influence on energy security.
Credit access is one of the significant factors contributing to the factors influencing energy security in rural areas. Households with access to credit are more likely to be energy secure than those without access.Footnote65 This is because these households have the flexibility to benefit from renewable energy sources, purchase improved household appliances, or access better connections.Footnote66 Based on the above information, the study hypothesised that access to credit has a positive influence on energy security.
Household income source is another of the significant factors contributing to the factors influencing energy security in rural areas. According to Yadama,Footnote67 multiple income streams such as agriculture and forestry assist households to gain access to clean energy. In rural areas, agriculture as a source of income plays a role in both incomes and also assisting households with energy as a resource of forestry.Footnote68 Based on the above information, the study hypothesised that having multiple sources of income has a positive influence on energy security.
Results and discussion
This section presents descriptive and econometrics results. To address the first objective, which is to profile energy security in the study areas, this section calculated the MEPI for respondents from the study areas. The also papers presents econometrics results based on the Tobit regression findings.
Socio-economic and demographic characteristics of the sample
presents descriptive statistics of the 283 households surveyed in the study. Concerning the respondents’ socio-economic characteristics, in both communities, the average age of the household head was 59 years ranging from 25 to 96 years old; in both communities females dominated in gender as indicated by 74.6% females in Hamburg and 66.7% in Melani. The majority of households in Hamburg were married (61.3%), while only (45.4%) of the sampled households in Melani were married. The average household size in both communities was four members. Most of the sampled households in Hamburg indicated that their household head had attained secondary education (43%), while most households in Melani indicated that their household head had attained primary education (44.7%).
Table 1. Descriptive statistics of sampled households.
The majority of the sampled households in both communities revealed that they mostly depend on the social grant as their source of income: Hamburg (76.8%) and Melani (84.4%). In Melani, there was no dependence on agriculture as a source of income, whereas respondents in Hamburg indicated that they use agriculture as a source of income (1.4%). In both communities, the respondents indicated high levels of unemployment: Hamburg (88%) and Melani (90.8%).
Energy security status of households
The study adopted the MEPI used in Sadath and Acharya (2017) to calculate the energy security status of households. Data on lighting sources, cooking, and additional measures (e.g. cow dung, wood, and paraffin) were obtained from the households in the study area. The MEPI was calculated to obtain the energy security status of each household in the study area. Following Nagothu (2016), access to electricity, access to an electric stove, additional measures (paraffin, gas, crop residue, and cow dung) and the MEPI at community level were calculated.
presents the results of energy poverty in the two communities, namely Hamburg and Melani. The average MEPI for Hamburg respondents was 17%, which implies low energy poverty. Results also indicate that 95.1% of the respondents from Hamburg had access to electricity, 72.5% had access to an electric stove for cooking, and 31.2% had access to additional energy measures. The intensity of the energy deprivation index was also very low (0.25).
Table 2. Multidimensional Energy Poverty Index estimates for Melani and Hamburg.
The average MEPI for Melani respondents was 16%, which implies very low energy poverty. Results also indicate that 98.6% of the respondents from Melani had access to electricity, 89.4% had access to an electric stove for cooking, and 40.1% had access to additional measures of energy. The intensity of the energy deprivation index was also very low (0.21). These findings suggests tha energy is lacking in quantity and quality for the respondents in the the two study areas; however, access to additional measures for energy was relatively low.
Econometrics results
Determinants of energy security status in rural areas
presents the results of the factors influencing energy security status in the study area, which were estimated using a Tobit regression model. The MEPI was used as the dependent variable on the Tobit regression model. The Tobit regression model was used because of its desirable characteristics of yielding consistent maximum likelihood estimates and accommodating the nature of the truncated dependent variable (Maziya et al., 2017). The variance inflation factor (VIF) was used to test the severity of multi-collinearity between the independent variables. The resulting values were less than 10, suggesting that multi-collinearity was not a problem. The results show that the chi-square (p-value= 0.0001) was high and statistically significant for a log livelihood ratio with a Pseudo R2 of 0.255. The low R-Squared value might be explained by the fact that the variables used in the model could not fully account for energy poverty as energy poverty can be explained by several other factors that were not considered in this study, such as gender, location, education.
Table 3. Determinants of energy security status: Tobit regression model results.
The results indicate that factors such as the marital status of the household head, household size, affordability, and source of income (agriculture) influence the energy security status of households in the study area. Household size and affordability were significant at 1%, while the marital status of the household head and source of income (agriculture) were significant at 5%. From the nine independent variables used in the model, five variables, namely age, employment status, amount of income, race, and credit access were insignificant.
From the four significant independent variables, two had positive signs, namely marital status and household size. The positive sign indicates that an increase in the predator variable will increase the multidimensional energy poverty index; therefore, lowering energy security. Two predator variables, namely source of income (agriculture) and affordability, had negative signs, implying that an increase in these independent variables will be associated with a decrease in the MEPI and increase energy security.
Marital status had a positive and significant (p-value: 0.005) relationship with MEPI. The results indicate that a positive unit change in the household's marital status (from being unmarried to married) is associated with a 4.040 units increase in the household's multidimensional energy poverty index, holding all other independent variables constant. These findings suggest that married households have more chances of being energy insecure than unmarried households. This might be explained by the fact that married households have more expenses to cover with their income than those committed to their relationships and family. Similar observations were noted by Tchereni et al. who argued that married households are more likely to be energy-poor than those who are not married because married households tend to have more expenditures to cover with their income, thus limiting the energy security status.
The size of the household had a positive and significant (p-value: 0.013) association with MEPI. A unit increase in household size is associated with a 0.748 units increase in the household MEPI, holding all other independent variables constant. These findings suggest that the bigger the household size, the more the household is likely to be energy insecure. This could be supported by the fact that the more people living in one household, the more they consume more energy in household activities, such as cooking, bathing, boiling water for tea, amongst others. Ismail and Khembo concurred that households with a significant number of household members staying in one house are more likely to be energy poor than households with a low number of people residing in the same household. According to Ismail and Khembo (2015), when the number of the household increases, a fixed household budget must be distributed among more people causing energy poverty. BridgeFootnote69 attested that an increase in household size is associated with a high consumption of resources.
Electricity affordability had a negative and significant (p-value: 0.000) relationship with MEPI. Results reveal that a unit increase in electricity affordability is associated with a −3.043 units decrease in the household's MEPI, holding all other independent variables constant. The results suggest that households who can afford to pay electricity bills are more likely to be energy secure. Affordability gives households purchasing power to consider a wide variety of energy sources, increasing their energy security compared to households with lower affordability. Similar observations were noted by Njiru and Letema, indicating that poor households in rural areas without access to affordable energy tend to spend their share of limited income on expensive and unhealthy forms of energy, thereby promoting energy poverty. Thus, affordability contributes to the improvement of the household's energy security in monetary terms. Further, Ismail and Khembo claimed that in most rural areas, low-income households cannot afford electricity provided by the national grid although they have access to it.
The source of income (agriculture) had a negative and significant (p-values: 0.024) relationship with MEPI. The results indicate that a positive unit change from other income sources to agriculture is associated with a −19.2234 units decrease in the household MEPI, holding all other independent variables constant. Therefore, the results suggest that households who move from other income sources to agriculture are more likely to be energy secure. The observed association might be explained by the fact that agriculture (for example keeping cattle) is a better rural income source than other income sources and will, therefore, assist in improving the energy purchasing power of rural households, thereby making them more energy secure than other households who depend on other income sources. Comparable conclusions by KilianFootnote70 suggest that households with low salaries are constrained by the high energy prices, which lower their purchasing power due to high energy bills, thereby leaving them energy impoverished. Also, Truen et al.Footnote71 stated that having more income streams may also contribute to higher-income levels, thereby improving the purchasing power for households, which will enable them to pay electricity bills.
Conclusion
The primary purpose of this paper was to assess the determinants of energy security in rural areas of the Eastern Cape Province using two specific areas, namely the Hamburg coastal community and the Melani inland community. The paper concludes that the majority of respondents in the study areas were energy secure. However, access to additional measures of energy was very low, which could affect their energy security if the primary source (ESKOM electricity) is interrupted in terms of electricity affordability or load shedding. The study further noted that household energy poverty is driven by marital status, household size, electricity affordability, and source of income. Therefore, the paper concludes that rural household energy security is influenced by socio-economic factors worth targeting to promote rural energy security among households.
Recommendations
Based on the study findings, the paper makes the following recommendations for purposes of promoting rural household energy security:
Implement energy-saving mechanisms in big household sizes to improve the energy security status (like boiling bathing water using one big pot) or using alternative measures for household energy use.
Ensuring that electricity is affordable to rural households.
Encouraging households to have multiple sources of income, such as agriculture, which might help in supplementing their income, which will then assist in paying their electricity bills.
Ensuring rural households who are not connected to electricity have access to supplementary measures of energy.
Acknowledgments
This study was part of a big project conducted by the African Climate Development Initiative (ACDI), University of Cape Town (UCT), in collaboration with the University of Fort Hare (UFH), and the University of KwaZulu-Natal (UKZN). The project was funded by the Water Research Commission (WRC) (K5/2718) entitled, ‘exploring the evidence of water-energy-food nexus linkages to sustainable local livelihoods and wellbeing in South Africa.’
Disclosure statement
The authors reported no potential conflicts of interest.
Notes on contributors
Mr. Thulani Ningi was born in Keiskammahoek (Qoboqobo) in the Eastern Cape Province, South Africa. He attended high school at Phakamisani Senior Secondary School, in Keiskammahoek. In 2016, he obtained his first degree in Agriculture: Agricultural Economics at the University of Fort Hare. In 2017 he obtained his Honours degree in Agriculture: Agricultural Economics. Thulani is currently studying toward a Master's degree with interest in water-energy-food nexus at the household level and working on a project which is a collaboration between the University of Fort Hare (RSVC), University of Cape Town (ACDI) and the University of KwaZulu-Natal. Thulani's research interests are on the water-energy-food nexus, food security, water security, energy security, welfare, and social justice.
Prof. Amon Taruvinga was born in Zimbabwe. He received his BSc (Hons) degree in Agricultural Economics from the University of Zimbabwe and his Ph.D., also in Agricultural Economics, from the University of Fort Hare, South Africa (2012). He is currently an Associate Professor in the Department of Agricultural Economics and Extension at the University of Fort Hare, South Africa, and a CIRCLE Visiting Fellow (CVF). He is also the Platform Chair for Southern Africa Climate Change & Biodiversity Research Platform (SACC&BRP). Prof Taruvinga specialises in biodiversity, environmental economics, human-wildlife interactions, food security, and climate change, and smallholder agriculture. He is interested in promoting sustainable human-wildlife interaction.
Dr. Leocadia Zhou is the Director for the Risk and Vulnerability Science Centre (RVSC) hosted in the Faculty of Science & Agriculture at the University of Fort Hare. Her strength lies in the multi-disciplinary approach and rapport with government officials at Provincial and National level and she has been heavily involved in bridging the gap between science and policy interface
Additional information
Funding
Notes
1 African News Agency, ‘Eskom Expresses Pride on Improved Access to Electricity.’
2 Odeku and Meyer, ‘Socioeconomic Implications of Energy Poverty in South African Poor Rural Households.’
3 Africa et al., ‘Assessment of Household Energy Poverty Levels in Louiville.’
4 IEA, ‘South Africa Energy Outlook – Analysis - IEA.’
5 Bretschger and Schaefer, ‘Dirty History versus Clean Expectations’
6 Department of Energy, ‘A Survey of Energy-Related Behaviour and Perceptions in South Africa.’
7 Blimpo, Postepska, and Xu, ‘Why is Household Electricity Uptake Low in Sub-Saharan Africa?’
8 Musemwa, ‘Factors Affecting Household Access to Enough Food in the Eastern Cape Province of South Africa.’
9 Chirau, ‘Female Heade d Household ’ s Rural Livelihood T Rajectories in Post- Apartheid Former Bantustans of South Africa.’
10 Francis and Webster, ‘Poverty and Inequality in South Africa.’
11 Statistics South Africa, ‘General Household Survey.’
12 Daily Dispatch, ‘Eastern Cape Remains South Africans Poorest Province.’
13 Statistics South Africa, ‘General Household Survey.’
14 Gladwin-Wood, ‘Free Basic Electricity’
15 IEA, ‘World Energy Outlook 2012 – Analysis - IEA.’
16 Barron and Torero, ‘Household Electrification and Indoor Air Pollution.’
17 Oyedepo, ‘Energy and Sustainable Development in Nigeria.’
18 Makonese, Masekameni, and Annegarn, ‘Energy Use Scenarios in an Informal Urban Settlement in Johannesburg, South Africa.’
19 Lloyd, ‘Challenges in Household Energisation and the Poor.’
20 Merten Marianne, ‘Electricity Price Goes up — Way beyond Inflation — … ’
21 Shackleton et al., ‘The Importance of Dry Woodlands and Forests in Rural Livelihoods and Poverty Alleviation in South Africa.’
22 Moyo et al., ‘Communal Area Grazing Strategies.’
23 Mhangara, Kakembo, and Lim, ‘Soil Erosion Risk Assessment of the Keiskamma Catchment, South Africa Using GIS and Remote Sensing.’
24 Africa, ‘Final Project Report for 2011 START Grants for Global Change Research in Africa The Impact of Climate Change on Food Security among Coastal Communities of Keiskamma, in the Eastern Cape, South Africa.’
25 Ndhleve, Musemwa, and Zhou, ‘How Severe Hunger Is amongst Rural Households of the Eastern Cape Province of South Africa.’
26 DWAF, ‘Development of an Internal Strategic Perspective for the Amatole-kei Area of the Mzimvubu to Keiskamma Water Management Area (wma no. 12) Executive Summary, Situation Assessment and Strategy Tables.’
27 Israel, ‘Sampling the Evidence of Extension Program Impact 1 The Evaluation Purpose Defining the Population.’
28 Ibid.
29 Nkonkobe Municipality, ‘Nkonkobe Municipality-Integrated Development Plan Review Document 2010/2011.’
30 Statistics South Africa, ‘Local Municipality | Statistics South Africa.’
31 Ashley and Carney, ‘Sustainable Livelihoods ’
32 Donohue and Biggs, ‘Monitoring Socio-Environmental Change for Sustainable Development.’
33 Scoones, ‘Livelihoods Perspectives and Rural Development.’
34 Donohue and Biggs, ‘Monitoring Socio-Environmental Change for Sustainable Development.’
35 Scoones, ‘Sustainable Rural Livelihoods a Framework for Analysis.’
36 Brocklesby and Fisher, ‘Community Development in Sustainable Livelihoods Approaches.’
37 FAO, ‘Analytical Framework.’
38 Chambers and Conway, ‘Sustainable Rural Livelihoods.’
39 Martens, ‘Livelihoods and Climate Change in Hamburg.’
40 Sadath and Acharya, ‘Assessing the Extent and Intensity of Energy Poverty Using Multidimensional Energy Poverty Index.’
41 Ibid.
42 Nussbaumer, Bazilian, and Modi, ‘Measuring Energy Poverty.’
43 Edoumiekumo, Tombofa, and Karimo, ‘Multidimensional Energy Poverty in the South-South Geopolitical Zone of Nigeria.’
44 Maziya, Mudhara, and Chitja, ‘What Factors Determine Household Food Security among Smallholder Farmers?.’
45 Ibid.
46 Mekuria and Mekonnen, ‘Determinants of Crop-Livestock Diversification in the Mixed Farming Systems.’
47 Chebil, Frija, and Thabet, ‘Economic Efficiency Measures and Its Determinants for Irrigated Wheat Farms in Tunisia.’
48 Africa et al., ‘Agricultural Economics Research, Policy and Practice in Southern Africa What Factors Determine Household Food Security among Smallholder Farmers ?.’
49 Ismail and Khembo, ‘Determinants of Energy Poverty in South Africa.’
50 Woldeamanuel, ‘Determinants of Household Energy Consumption in Urban Areas of Ethiopia.’
51 Diederichs, ‘SALGA Energy Guideline Series DRAFT Guideline on Technologies for Reducing Energy Poverty in Low-Income Households.’
52 Uhunamure, Nethengwe, and Musyoki, ‘Driving Forces for Fuelwood Use in Households in the Thulamela Municipality, South Africa.’
53 Ibid.
54 Tchereni, Grobler, and Dunga, ‘Economic Analysis of Energy Poverty in South Lunzu, Malawi.’
55 Woldeamanuel, ‘Determinants of Household Energy Consumption in Urban Areas of Ethiopia.’
56 Démurger and Fournier, ‘Poverty and Firewood Consumption.’
57 Semenya and Machete, ‘Factors That Influence Firewood Use among Electrified Bapedi Households of Senwabarwana Villages, South Africa.’
58 Gerbery and Richard, ‘Exploring Multi-Dimensional Nature of Poverty in Slovakia.’
59 Woldeamanuel, ‘Determinants of Household Energy Consumption in Urban Areas of Ethiopia.’
60 Mpeta, Fourie, and Inwood, ‘Black Living Standards in South Africa before Democracy.’
61 Gradín, ‘Race, Poverty and Deprivation in South Africa.’
62 Njiru and Letema, ‘Energy Poverty and Its Implication on Standard of Living in Kirinyaga, Kenya.’
63 Jessel, Sawyer, and Hernández, ‘Energy, Poverty, and Health in Climate Change.’
64 Abebaw, ‘Household Determinants of Fuelwood Choice in Urban Ethiopia.’
65 Cecelski, ‘Energy and Poverty Reduction.’
66 Ibid.
67 Yadama, ‘Fires, Fuel, and the Fate of 3 Billion.’
68 Ibid.
69 Bridge, ‘Individual and Household-Level Effects of Energy Poverty on Human Development.’
70 Kilian, ‘The Economic Effects of Energy Price Shocks.’
71 Truen, ‘Updating the South Africa-SADC Remittance Channel Estimates.’
References
- Abebaw, Degnet. ‘Household Determinants of Fuelwood Choice in Urban Ethiopia: A Case Study of Jimma Town Author (s): Degnet Abebaw Source: The Journal of Developing Areas, Vol . 41, No . 1 (Fall, 2007), Pp . 117–126 Published by: College of Business, Tennessee’. The Journal of Developing Areas 41, no. 1 (2007): 117–126.
- Africa, South. ‘Final Project Report for 2011 START Grants for Global Change Research in Africa The Impact of Climate Change on Food Security among Coastal Communities of Keiskamma, in the Eastern Cape, South Africa,’ 2012.
- Africa, South, Shalala Mgwambani, Kevin K Kasangana, Daniel Masekameni, Tafadzwa Makonese, and Thokozani P Mbonane. ‘Assessment of Household Energy Poverty Levels in Louiville,’ 2006.
- African News Agency. ‘Eskom Expresses Pride on Improved Access to Electricity,’ May 10, 2018. https://www.iol.co.za/news/south-africa/eskom-expresses-pride-on-improved-access-to-electricity-17259230.
- Ashley, Caroline, and Diana Carney. ‘Sustainable Livelihoods: Sustainable Livelihoods,’ n.d.
- Barron, Manuel, and Maximo Torero. ‘Household Electrification and Indoor Air Pollution’. Journal of Environmental Economics and Management 86 (2017): 81–92. doi:10.1016/j.jeem.2017.07.007.
- Blimpo, Moussa P., Agnieszka Postepska, and Yanbin Xu. ‘Why is Household Electricity Uptake Low in Sub-Saharan Africa?’. World Development 133 (2020): 105002. doi:10.1016/j.worlddev.2020.105002.
- Bretschger, Lucas, and Andreas Schaefer. ‘Dirty History Versus Clean Expectations: Can Energy Policies Provide Momentum for Growth?’. European Economic Review 99 (2017): 170–190. doi:10.1016/j.euroecorev.2017.01.001.
- Bridge, Brandon. ‘Individual and Household-Level Effects of Energy Poverty on Human Development,’ 2017, 63.
- Brocklesby, Mary Ann, and Eleanor Fisher. ‘Community Development in Sustainable Livelihoods Approaches - An Introduction’. Community Development Journal 38, no. 3 (2003): 185–198. doi:10.1093/cdj/38.3.185.
- Cecelski, E. W. ‘Energy and Poverty Reduction: The Role of Women as a Target Group.’ Debate on Sustainable Energy in Danish Development Assistance, Copenhagen: Landstingssalen, Christiansborg, September 5 (2000): 2000.
- Chambers, R., and G. R. Conway. ‘Sustainable Rural Livelihoods: Practical Concepts for the 21st Century’. IDS Discussion Paper 296 (1992).
- Chebil, Ali, Aymen Frija, and Chokri Thabet. ‘Economic Efficiency Measures and its Determinants for Irrigated Wheat Farms in Tunisia: A DEA Approach’. New Medit 14, no. 2 (2015): 32–38.
- Chirau, Takunda J. ‘Female Heade d Household’s Rural Livelihood T Rajectories in Post- Apartheid Former Bantustans of South Africa: Emerging Evidence from the Eastern Cape’. Bangladesh Sociological Society 15, no. 1 (1994): 148–163.
- Daily Dispatch. ‘Eastern Cape Remains South Africans Poorest Province,’ August 23, 2017. https://www.dispatchlive.co.za/news/2017-08-23-e-cape-remains-sas-poorest-province/.
- Démurger, Sylvie, and Martin Fournier. ‘Poverty and Firewood Consumption: A Case Study of Rural Households in Northern China’. China Economic Review 22, no. 4 (2011): 512–523. doi:10.1016/j.chieco.2010.09.009.
- Department of Energy. ‘A Survey of Energy-Related Behaviour and Perceptions in South Africa: The Residential Sector.’ 2012. http://www.energy.gov.za/files/media/Pub/DoE-2013-Survey-of-EnergyRelated-Behaviour-and-Perception-in-SA.pdf
- Diederichs, Nicci, Magaret Mckenzie, and Abigail Knox. ‘SALGA Energy Guideline Series DRAFT Guideline on Technologies for Reducing Energy Poverty in Low-Income Households. Guideline: Technologies for Reducing Energy Poverty in Low-Income Households,’ 2013. www.urbanearth.co.za.
- Donohue, Caroline, and Eloise Biggs. ‘Monitoring Socio-Environmental Change for Sustainable Development: Developing a Multidimensional Livelihoods Index (MLI)’. Applied Geography 62 (2015): 391–403. doi:10.1016/j.apgeog.2015.05.006.
- DWAF. ‘Development of an Internal Strategic Perspective for the Amatole-kei Area of the Mzimvubu to Keiskamma Water Management Area (wma no. 12) Executive Summary, Situation Assessment and Strategy Tables.’ Pretoria, 2004. http://www.dwaf.gov.za/documents/.
- Edoumiekumo, Samuel Gowon, Stephen S. Tombofa, and Tamarauntari Moses Karimo. ‘Multidimensional Energy Poverty in the South-South Geopolitical Zone of Nigeria’. Journal of Economics and Sustainable Development 4, no. 20 (2013): 96–104.
- FAO. ‘Analytical Framework: Sustainable Livelihoods,’ 2002. http://www.fao.org/3/j2602e/j2602e02.htm.
- Francis, David, and Edward Webster. ‘Poverty and Inequality in South Africa: Critical Reflections’. Development Southern Africa 36, no. 6 (November 2, 2019): 788–802. doi:10.1080/0376835X.2019.1666703.
- Gerbery, Daniel and Filčák Richard. ‘Exploring Multi-Dimensional Nature of Poverty in Slovakia: Access to Energy and Concept of Energy Poverty’. Ekonomický Časopis 62, no. 06 (2014): 579–597.
- Gladwin-Wood, C., and M. Mathebula. ‘Free Basic Electricity: Do You Have a Right to It?,’ 2016. https://www.schindlers.co.za/2016/free-basic-electricity-do-you-have-a-right-to-it/?pdf=6670.
- Gradín, Carlos. ‘Race, Poverty and Deprivation in South Africa’. Journal of African Economies 22, no. 2 (2013): 187–238. doi:10.1093/jae/ejs019.
- IEA. ‘South Africa Energy Outlook – Analysis - IEA,’ 2019. https://www.iea.org/articles/south-africa-energy-outlook.
- IEA. ‘World Energy Outlook 2012 – Analysis - IEA,’ 2012. https://www.iea.org/reports/world-energy-outlook-2012.
- Ismail, Zaakirah, and Patrick Khembo. ‘Determinants of Energy Poverty in South Africa’. Journal of Energy in Southern Africa 26, no. 3 (2015): 66–78.
- Israel, Glenn D. ‘Sampling the Evidence of Extension Program Impact 1 The Evaluation Purpose Defining the Population,’ 2013. http://edis.ifas.ufl.edu/pd006.
- Jessel, Sonal, Samantha Sawyer, and Diana Hernández. ‘Energy, Poverty, and Health in Climate Change: A Comprehensive Review of an Emerging Literature’. Frontiers in Public Health 7 (2019): 357. doi:10.3389/fpubh.2019.00357.
- Kilian, Lutz. ‘The Economic Effects of Energy Price Shocks’. Journal of Economic Literature 46, no. 4 (2008): 871–909. doi:10.1257/jel.46.4.871.
- Lloyd, Philip. ‘Challenges in Household Energisation and the Poor’. Journal of Energy in Southern Africa 25, no. 2 (2014): 2–8. doi:10.17159/2413-3051/2014/v25i2a2662.
- Makonese, T., D. M. Masekameni, and H. J. Annegarn. ‘Energy Use Scenarios in an Informal Urban Settlement in Johannesburg, South Africa.’ In Proceedings of the 24th Conference on the Domestic Use of Energy, DUE 2016. Institute of Electrical and Electronics Engineers Inc., 2016. https://doi.org/10.1109/DUE.2016.7466703.
- Martens, Brendon. ‘Livelihoods and Climate Change in Hamburg: Issues for Food Security a Thesis Submitted in Fulfilment of the Requirements for the Degree of Masters in Economics of by Supervisor: Professor Gavin Fraser,’ 2015.
- Maziya, Mbongeni, Maxwell Mudhara, and Joyce Chitja. ‘What Factors Determine Household Food Security among Smallholder Farmers? Insights from Msinga, KwaZulu-Natal, South Africa’. Agrekon 56, no. 1 (2017): 40–52. doi:10.1080/03031853.2017.1283240.
- Mekuria, Wuletaw, and Kindu Mekonnen. ‘Determinants of Crop-Livestock Diversification in the Mixed Farming Systems: Evidence from Central Highlands of Ethiopia’. Agriculture and Food Security 7, no. 1 (2018): 1–15. doi:10.1186/s40066-018-0212-2.
- Merten Marianne. ‘Electricity Price Goes up — Way beyond Inflation — … ,’ March 8, 2019. https://www.dailymaverick.co.za/article/2019-03-08-electricity-price-goes-up-way-beyond-inflation-as-ramaphosa-answers-questions-on-eskom-in-the-house/#gsc.tab=0.
- Mhangara, Paidamwoyo, Vincent Kakembo, and Kyoung Jae Lim. ‘Soil Erosion Risk Assessment of the Keiskamma Catchment, South Africa Using GIS and Remote Sensing’. Environmental Earth Sciences 65, no. 7 (2012): 2087–2102. doi:10.1007/s12665-011-1190-x.
- Moyo, B., S. Dube, M. Lesoli, and P. J. Masika. ‘Communal Area Grazing Strategies: Institutions and Traditional Practices’. African Journal of Range and Forage Science 25, no. 2 (2008): 47–54. doi:10.2989/AJRFS.2008.25.2.2.481.
- Mpeta, Bokang, Johan Fourie, and Kris Inwood. ‘Black Living Standards in South Africa before Democracy: New Evidence from Height’. South African Journal of Science 114, no. 1–2 (2018): 1–8. doi:10.17159/sajs.2018/20170052.
- Musemwa, L. ‘Factors Affecting Household Access to Enough Food in the Eastern Cape Province of South Africa’. Journal of Development and Agricultural Economics 5, no. 3 (2013): 84–91. doi:10.5897/jdae12.039.
- Ndhleve, S., L. Musemwa, and L. Zhou. ‘How Severe Hunger Is amongst Rural Households of the Eastern Cape Province of South Africa.’ Journal of Economics and Sustainable Development 4, no. 3 (2013): 220–228.
- Njiru, Christine W., and Sammy C. Letema. ‘Energy Poverty and Its Implication on Standard of Living in Kirinyaga, Kenya’. Journal of Energy 2018 (2018): 1–12. doi:10.1155/2018/3196567.
- Nkonkobe Municipality. ‘Nkonkobe Municipality-Integrated Development Plan Review Document 2010/2011,’ 2012. http://www.nkonkobe.co.za/nkonkobeidp20112012.pdf.
- Nussbaumer, Patrick, Morgan Bazilian, and Vijay Modi. ‘Measuring Energy Poverty: Focusing on What Matters’. Renewable and Sustainable Energy Reviews 16, no. 1 (2012): 231–243. doi:10.1016/j.rser.2011.07.150.
- Odeku, Kola Olusola, and Edson Meyer. ‘Socioeconomic Implications of Energy Poverty in South African Poor Rural Households’. Academy of Entrepreneurship Journal 25, no. 3 (2019): 1–12.
- Oyedepo, Sunday Olayinka. ‘Energy and Sustainable Development in Nigeria: The Way Forward Sustainable Energy Renewable Energy Energy Efficiency Energy Conservation Review Background’. Energy, Sustainability and Society (2012): 1–17. http://energsustainsoc.springeropen.com/articles/10.1186/21920567215.
- Sadath, Anver C, and Rajesh H Acharya. ‘Assessing the Extent and Intensity of Energy Poverty Using Multidimensional Energy Poverty Index: Empirical Evidence from Households in India’. Energy Policy 102, no. December 2016 (2017): 540–550. doi:10.1016/j.enpol.2016.12.056.
- Scoones, Ian. ‘Livelihoods Perspectives and Rural Development’. The Journal of Peasant Studies 36, no. 1 (2009): 171–196. doi:10.1080/03066150902820503.
- Scoones, Ian. ‘Sustainable Rural Livelihoods a Framework for Analysis’. Analysis 72 (1998): 1–22. doi:10.1057/palgrave.development.1110037.
- Semenya, Khomotso, and Fannie Machete. ‘Factors that Influence Firewood Use among Electrified Bapedi Households of Senwabarwana Villages, South Africa’. African Journal of Science, Technology, Innovation and Development 11, no. 6 (2019): 719–729. doi:10.1080/20421338.2019.1572336.
- Shackleton, Charlie M., Sheona E. Shackleton, Erik Buiten, and Neil Bird. ‘The Importance of Dry Woodlands and Forests in Rural Livelihoods and Poverty Alleviation in South Africa’. Forest Policy and Economics 9, no. 5 (2007): 558–577. doi:10.1016/j.forpol.2006.03.004.
- Statistics South Africa. ‘General Household Survey,’ 2018. http://www.statssa.gov.za/publications/P0318/P03182018.pdf.
- Statistics South Africa. ‘Local Municipality | Statistics South Africa,’ 2013. http://beta2.statssa.gov.za/?page_id=993&id=ngqushwa-municipality.
- Tchereni, Betchani H M, Wynand Grobler, and Steven H Dunga. ‘Economic Analysis of Energy Poverty in South Lunzu, Malawi’. Economic Analysis 4, no. 4 (2013): 154–164.
- Truen, S., K. Kgaphola, and M. Mokoena. ‘Updating the South Africa-SADC Remittance Channel Estimates,’ 2016. https://finmark.org.za/updating-the-sa-sadc-remittance-channel-estimates-2016/.
- Uhunamure, Solomon Eghosa, Nthaduleni Samuel Nethengwe, and Agnes Musyoki. ‘Driving Forces for Fuelwood Use in Households in the Thulamela Municipality, South Africa’. Journal of Energy in Southern Africa 28, no. 1 (2017): 25–34.
- Woldeamanuel, Alemayehu Agizew. ‘Determinants of Household Energy Consumption in Urban Areas of Ethiopia.’ IUSSP, 2017. https://iussp.confex.com/iussp/ipc2017/meetingapp.cgi/Paper/1820.
- Yadama, N. Gautam. ‘Fires, Fuel, and the Fate of 3 Billion: The State of the Energy Impoverished: 9780199336678: Medicine & Health Science Books @ Amazon.Com,’ 2016. https://www.amazon.com/Fires-Fuel-Fate-Billion-Impoverished/dp/0199336679.