Abstract
Compared with age-matched employees, university students report higher levels of chronic stress and this may affect their decision-making. The impact of chronic stress and physiological reactivity upon cognitive function is receiving more attention, but few studies have empirically assessed the associations of these variables concurrently. Our aim was to investigate if chronic student stress, as assessed by effort-reward imbalance (ERI) and overcommitment, and physiological reactivity, were related to decision-making. As measures of physiological reactivity, we collected salivary alpha-amylase (sAA) and continuously recorded heart rate variability (HRV) data from male students (n = 79) at pretest and immediately after some computerized decision-making tasks (simple and choice- reaction times). Our findings suggest that students who are higher in overcommitment and who are more physiologically reactive (sAA and HRV indices) at the pretest stage may be more “at-risk” of poor decision-making than others. If others can replicate our findings in more diverse samples, this will contribute to an evidence base for interventions targeted at reducing overcommitment, ERI, and dysregulated autonomic reactivity to improve decision-making.
1. Introduction
High stress amongst university students is a serious public health issue, with university students reporting higher chronic stress than the general population (Stewart-Brown et al., Citation2000) and poorer health than their age-matched working peers (Vaez, Kristenson, & Laflamme, Citation2004; Wege, Li, Muth, Angerer, & Siegrist, Citation2017). Emerging evidence also suggests that the dysregulated autonomic activity associated with chronic stress may contribute to the diminished speed and accuracy of decision-making and reaction-time (RT; Landolt et al., Citation2017). Identifying the underlying links between stress and physiological indicators of the stress response, and between stress and the speed and accuracy of decision-making, may provide an evidence base for targeted intervention.
1.1. Effort-reward imbalance among university students
The “effort-reward imbalance” (ERI; Siegrist, Citation1996) model posits that increased stress occurs when there is an imbalance between the perceived efforts expended and perceived rewards received (Siegrist, Citation1996). The stress due to the imbalance, subsequently places individuals at risks of health problems (Siegrist, Citation2010) and maybe the product of psychological, neuro-biological and behavioral pathways (Tsutsumi & Kawakami, Citation2004). The model includes an intrinsic component termed “overcommitment” (OC) that assesses an individual’s motivational pattern of excessive work-related engagement.
Both ERI and OC have been related to physiological indicators suggestive of elevated chronic stress and ensuing ill-health. In particular, recent meta-analyses of predominantly naturalistic designs, have shown higher levels of ERI and OC are related to non-adaptive cardiovascular (Eddy, Wertheim, Kingsley, & Wright, Citation2017), immune (Eddy, Heckenberg, Wertheim, Kent, & Wright, Citation2016), and hypothalamic-pituitary-adrenal axis responses (Eddy, Wertheim, Hale, & Wright, Citation2018).
The ERI model has shown an ability to accurately predict adverse health outcomes, and physiological reactivity (Siegrist & Li, Citation2016), but less is known about how an ERI impacts work or university performance. This study will focus on the pathways between ERI, physiological states, and decision-making.
1.2. Chronic stress, physiological reactivity, and decision-making
A recent meta-analysis (k = 26, N = 1829) suggests that the association between reduced decision-making in response to an acute stressor is dependent upon the type of stressor, the decision-situation, but not individual difference in gender, age, physiological reactivity, or time between stressor exposure and the decision-making test (Starcke & Brand, Citation2016). However, this evidence emanates solely from research assessing decision-making under uncertainty post-acute stress, and as such, may not apply to decision-making in other circumstances or in response to chronic stress. Although chronic stress may have a greater impact on decision-making than acute stress (Baradell & Klein, Citation1993), chronic stress has seldom been investigated in this context. Decision-making is broadly defined in this study as the speed and accuracy of responses to stimuli (i.e. simple and choice RTs), and the importance of this definition lies in the recognition that these attributes are integral components of higher-order decision-making (Noorani & Carpenter, Citation2016).
Chronic stress and subsequent dysregulated physiological stress responses are regarded as important factors in poor decision-making (Putman, Antypa, Crysovergi, & van der Does, Citation2010; Starcke & Brand, Citation2012). Research examining the effects of chronic stress on decision-making is limited, however, with only three known studies concurrently assessing physiological indices and self-reported chronic stress (i.e. Landolt et al., Citation2017; Radenbach et al., Citation2015; van Honk, Schutter, Hermans, & Putman, Citation2003).
Radenbach et al. (Citation2015) demonstrated that inter-individual variability in cortisol and heart rate reactivity predicted decrements in decision-making (n = 39 males) and further, that physiological reactivity attenuated the link between self-reported stress and decision-making. Similarly, higher ERI in jockeys (n = 32) was associated with decrements in decision-making and this relationship was stronger for those with lower heart rate variability (HRV) (Landolt et al., Citation2017). Finally, lower basal cortisol levels were related to impairments in decision-making skills (van Honk et al., Citation2003). Taken together, the findings suggest that interindividual differences in basal levels of stress, and stress reactivity, may impact decision-making. We will seek to extend these findings by investigating if higher anticipatory stress to a laboratory-based test of cognitive function, or the rate of recovery towards basal levels is most associated with decision-making
1.3. The present investigation
This study will assess several measures of the autonomic nervous system (HRV and salivary alpha-amylase (sAA)) as we aim to identify the relationships between ERI and physiology, with outcomes of decision-making in university students. Consistent with the ERI model, it was expected that students reporting higher academic stress would have non-adaptive physiological responses and poorer decision-making. Further, we anticipated that higher pre and post-test physiological responses would be related to performance on the decision-making tasks.
2. Method
2.1. Participants
Full-time male university students (Mage = 22.57, SDage = 3.80 age range = 18–35) were recruited (N = 79) using a script published on online social-media and via face-to-face invitation at the university campus. Eligibility criteria included being at least 18 years old, proficient in English, and male, due to potential gender differences in sAA (Nater, Abbruzzese, Krebs, & Ehlert, Citation2006) and cardiovascular responses to laboratory stressors (Feldman, Cohen, Hamrick, & Lepore, Citation2004). Participants who reported taking medication, were presently experiencing ill-health or physically frail, or who had chronic health, thyroidal, heart or mental health issues were asked to self-exclude. These exclusion criteria have been reported to confound the physiological measures utilized in this study (Nater et al., Citation2005; Task Force of the European Society of Cardiology and the North American Society of Pacing and Electrophysiology, Citation1996). Participants were required not to have eaten, consumed caffeinated or alcoholic beverages, smoked or engaged in physical exercise within one hour of participating, as these activities have been found to affect sAA concentration (Nater, Rohleder, Schlotz, Ehlert, & Kirschbaum, Citation2007). Participants were required to be proficient in English in order to read and fully understand the questionnaires. Participants were advised that they would be compensated with a double movie pass for their participation. Institutional ethics approval was granted for this study (HEC17-015).
2.2. Materials
2.2.1. University stress
The effort-reward imbalance university scale (ERI-U; Wege et al., Citation2017) was used to measure perceived university stress. It included a total of 14-items across 3 subscales measured on a 4-point Likert scale with an answer format: “strongly disagree” (1), “disagree” (2), “agree” (3), and “strongly agree” (4). Three items measure efforts, such as “I have constant time pressure due to a heavy study load”, six items measure rewards, such as “I receive the respect I deserve from my supervisors (teachers)”, and five items measure OC, such as “people close to me say I sacrifice too much for my study”. An ERI ratio was computed from the efforts and rewards scales using a correction factor to account for the uneven number of items. Ratio scores greater than 1 indicate more stress and greater risks of ill health (Siegrist, Citation2010). The efforts (α = 0.70), OC (α = 0.76) and rewards (α = 0.64) scales showed adequate internal consistency (i.e. >0.60, Hair, Black, Babin, & Anderson, Citation2010).
2.2.2. Heart rate variability
Generally, a “normal” response to a perceived stressor involves reduced vagal tone and increased adrenaline from sympathetic nerves, which increases heart rate while the parasympathetic system initiates arousal regulation and decreases HRV (Thayer, Yamamoto, & Brosschot, Citation2010). Two measures of HRV in the time domain were determined, the standard deviation of the normal-to-normal RR intervals (SDNN) and the square root of the sum of the mean of squared differences between adjacent normal-to-normal RR intervals (RMSSD). The SDNN index includes all cyclic components responsible for variability and therefore reflects overall HRV (Task Force of the European Society of Cardiology & the North American Society of Pacing & Electrophysiology, Citation1996). The RMSSD index estimates high frequency variations in HRV and provides an estimate of parasympathetic activity.
Consecutive RR intervals were recorded using a wireless heart rate monitor (RS800CX; Polar, Finland). The Polar heart rate monitors have been shown to produce electrocardiography comparable measures of RR-interval with derived time-domain HRV (Nater, La Marca, et al., Citation2006). After removing non-sinus RR-intervals, consecutive RR-intervals were analyzed using customized commercial software (LabVIEW 2016; National Instruments, UK) to determine short-term HRV in the time domain for 5-min. epochs in user-selected time blocks.
As the reaction-time (RT) phase was ∼10 minutes in duration, the mean SDNN and RMSSD score were calculated by averaging results for the two 5 min. epochs. Mean SDNN and RMSSD were also determined during pretest, and RT phases (see procedure below).
2.2.3. sAA
sAA is a digestive enzyme that serves as an indirect marker of sympathoadrenal medullary (SAM) responses, with higher concentrations reported at both pre and post-acute stress (Nater et al., Citation2005). sAA is often used alongside the hormone cortisol in acute testing as another marker of the stress response and for its ability to return to basal states quickly post-exposure and for its shorter time lag between stress exposure and salivary secretion (Gordis, Granger, Susman, & Trickett, Citation2006).
Saliva samples were assayed using the Salimetrics® 98 Well Kinetic Enzyme Assay Kit (No. 11902; State College, PA, USA). These procedures have been previously described (O’Donnell, Landolt, Hazi, Dragano, & Wright, Citation2015). Optical density measurements were conducted at 450 nm using a Synergy™ HT Multi-Detection Micro-Plate Reader and concentrations of the compound were analyzed using the KC4 v3.4 microplate data analysis software (Bio-Tek Instruments, Inc., Winooski, VT). The assay has a lower detection limit of 0.1 nmol/L with intra- and inter-assay coefficients of variations <8%.
2.2.4. Decision-making
Decision-making skills were assessed using the simple RT and choice RT tasks from the CogState computerized cognitive test battery (CogState Research software; Version 6; CogState Limited, 2011) on a 14 inch laptop computer. Simple RT is a measure of psychomotor function while choice RT assesses visual attention (Maruff, Falleti, Collie, Darby, & McStephen, Citation2005). Tasks from the CogState battery have shown to test specific areas of cognition measuring RT to the millisecond, are sensitive to very mild cognitive changes and include minimal practice effects (Maruff et al., Citation2005). During the “detection” task, simple RT is assessed. Participants are presented with a playing card face-down in the center of the screen and are required to press the response key as quickly as possible when the card turns face-up. The “identification” task assesses choice RT and is similar to the simple RT test as participants are instructed to respond to a flipped card as quickly as possible, this time indicating with the appropriate response keys whether the card is red or black. For both the simple reaction and choice RT tasks, higher scores indicate slower RTs. The CogState test battery also undergoes several internal integrity checks, such that the performance accuracy on the choice RT task should be 90% or greater. In alignment with most literature using the CogState software, we used the speed of reaction, and not the accuracy of reaction to determine simple and choice RT. For further information and a video displaying the CogState tasks please visit: https://www.cogstate.com/clinical-trials/computerized-cognitive-assessment/featured-batteries/.
2.3. Procedure
Upon arrival, participants were provided with the participant information statement and were asked if any exclusion criteria applied to them. They were then invited into the laboratory to provide written consent before being given access to a private room and instructions on fitting the heart rate monitor. Recording of RR-interval began immediately and continued throughout the experiment.
2.3.1. PT phase (M = 6 min., SD = 0.74, range = 6–12 min.)
First, a pretest (Pre) saliva sample was obtained. Participants were given a Salivette® and instructed to dip the cotton swab into the mouth without touching it and rotate in a circular motion for 60 s without chewing, to collect saliva from all three salivary glands in the mouth. Next, participants, while seated at a desk, with the laptop computer to their left, completed the questionnaire pack.
2.3.2. RT phase (M = 12 min., SD = 1.38, range = 9–17 min.)
Participants were then directed to complete the CogState RT tasks. Instructions and practice trials are routinely presented before each CogState task to ensure participants understand the rules and requirements. Participants completed the simple RT task followed by the choice RT task. At the completion of the test battery, participants provided a second saliva sample as described above. Following the completion of this experiment, participants were exposed to an additional stress test (Maastricht Acute Stress Test) but results are not reported here because the test was performed independently from the particular experimental setting presented in our analyses. In acute stress tests such as the Trier Social Stress Test and the MAST, the strongest autonomic reactions (lower SDNN, and RMSSD, and higher sAA) often occur in the anticipatory (pretest; PT) phase of the experiment (Smeets et al., Citation2012). Our team can also report higher HR and HRV in the PT phase of our pilot studies using CogState, hence why the difference between PT and RT phases were used to assess anticipatory physiological reactivity.
2.3.3. Maastricht acute stress phase (M = 13 min., SD = 0.84, range = 10–15)
The MAST information is reported here to demonstrate the sequence of phases in the study. The data is not discussed in this paper. The MAST involves participants immersing their hand in very cold water on several occasions. Further, in-between the immersions, participants are required to perform mental arithmetic as quickly as possible. Full details of the MAST are provided elsewhere (Smeets et al., Citation2012).
2.4. Data analysis
All analyses were performed using IBM SPSS statistics computer software package (Version 22.0). A series of paired t-tests were used to test if the physiological measures (HRV and sAA) differed between the pretest and the RT phases of the experiment. Bivariate Pearson correlation coefficients were calculated to investigate relationships between key variables. Two hierarchical regressions were used to assess the relationship between university stress, indices of stress physiology with choice and simple RT. Additional analyses were conducted to assess if physiological reactivity mediated or moderated the relationship between OC and choice RT. All statistical analyses were conducted using a criterion value of p<.05, unless otherwise specified, and the moderation analysis was conducted using the SPSS PROCESS macro (Hayes, Citation2013) with bootstrapping (N = 5000 resamples).
3. Results
3.1. Data management
The ERI, SDNN (pre and RT), and sAA (pre and RT) distributions were positively skewed (z > 3.29) and corrected with a square root transformation (Tabachnick & Fidell, Citation2014). Although descriptive statistics for the ERI, SDNN, and sAA report untransformed M and SD’s, all subsequent parametric analyses utilized the transformed variables. All data fell within 2.59 (p = .001) standard deviations of the variable mean with no values adjusted or excluded as outliers. The other assumptions for regression; linearity, multicollinearity, and homoscedasticity, were checked with no violations to report.
3.2. Normative and experimental phase comparisons
The mean ERI of the student sample (M = 0.81, SD = 0.29) was lower (p<.001, Cohen’s d = 0.91) than data from a medical education sample (n = 406, M = 1.09, SD = 0.32 Wege et al., Citation2017).
We compared the physiological assessments across the pretest and RT phases (). As expected, sAA and SDNN, decreased and increased respectively, during the RT phase. The RMSSD data did not change between phases.
Table 1. Mean scores for sAA and heart rate variability indices across conditions (N = 79).
3.3. Academic stress and physiological reactivity
Pearson correlation coefficients were calculated to assess the associations of the independent variables entered into the subsequent regression analyses (). Older students were more stressed and overcommitted than younger students and students with high ERI were more overcommitted.
Table 2. Pearson correlation coefficients for the study variables.
3.4. University stress, physiological reactivity, and reaction-time
A hierarchical regression assessed the relationship of the psychological stress variables (ERI, OC), and the physiological stress variables at pre and RT phases (sAA, SDNN, RMSSD) with simple and choice RT (), after controlling for age. It was important to statistically control for age when considering the associations between the key stress, physiological and decision-making variables, as age was correlated with ERI, OC, and sAA at pretest ().
Table 3. Hierarchical regression Analyses assessing the relationship between psychological and physiological variables (pretest and RT phases) with simple and choice reaction time, after controlling for age.
For the simple RT model, SDNN and RMSSD (pre) were associated with simple RT. Similarly, in the choice RT model, both SDNN and RMSSD at pretest were associated with choice RT, but additionally, OC was associated with choice RT. Specifically, more overcommitted students and those with greater SDNN and lesser RMSSD activity at pretest had longer choice RTs which are indicative of poorer decision-making.
To assess if “third variable relationships” better explained the data, we assessed if any of the physiological variables in the pretest phase moderated or mediated the relationship between OC and choice RT. Difference scores for the HRV and sAA variables were calculated by subtracting PT scores from RT scores. Neither changes in sAA (β < 0.001, p=.804), SDNN (β < 0.001, p=.912), or RMSSD (β<.001, p=.737) moderated the relationship between OC and choice RT. Changes in sAA, SDNN and RMSSD between the anticipatory and RT phase did not mediate the relationship between OC and choice RT with all ranges of the 95% confidence interval for the direct effect containing zero. Finally, as the bivariate relationship between ERI and choice reaction was significant but was rendered non-significant with the inclusion of OC in the model (); we assessed if OC mediated the relationship between ERI and choice RT. OC did mediate this relationship, indirect effect b = 0.099, CI [0.029, 0.198], as the confidence interval did not include zero and suggests that high OC helps to explain the relationship between high academic stress and poor choice RT.
The final analyses (with OC removed) assessed if any of the physiological variables moderated the relationship between ERI and choice RT. SDNN (β = 0.001, p=.581) and RMSSD (β = 0.005, p=.132) pretest scores did not moderate the relationship between ERI and choice RT, but the sAA pretest score did (β = 0.003, p=.029). We used the Johnson-Neyman technique (Johnson & Fay, Citation1950) to assess for interactive moderation as unlike simple slopes analysis, this allows the exact computation of conditions and boundary values of the regions of significance (Bauer & Curran, Citation2005). The Johnson-Neyman significance regions illustrate that the relationship between ERI and choice RT differed for those with low (bottom 58%), compared with high (top 42%), pretest sAA (). We can report the point of dichotomy of the sAA moderator variable (identified using the Johnson-Neyman technique) shows the low sAA group (M = 86.24, SD = 40.41) had lower concentrations than the high sAA group (M = 258.69, SD = 95.55), t (77)=11.55, p<.001.
Figure 1. Illustration of the association between effort-reward imbalance (ERI) and choice reaction-time for those with low (n = 46) and high (n = 33) levels of salivary alpha-amylase (sAA) at pretest. Note: The ERI and sAA variables were mean-centered prior to moderation analysis, but we display the raw ERI, not the z-score in our illustration
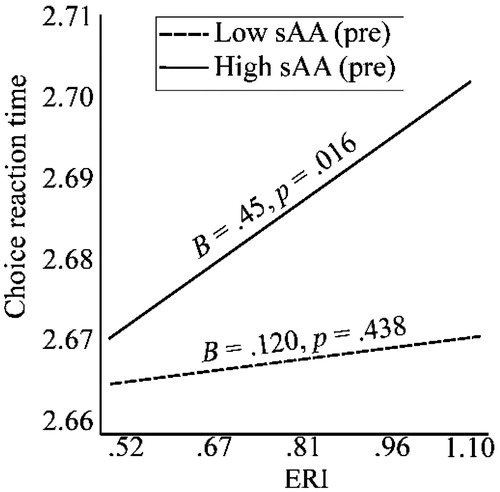
Our interpretation of the data () is that high OC and higher SDNN, and lower RMSSD at pretest are associated with poorer choice-RT. Additionally, when OC is removed from the equation, there is a strong relationship between high ERI and poor choice-RT (), but only for those with high sAA at pretest (top 42%, n = 33).
4. Discussion
We investigated the relationship between university stress and student decision-making (simple and choice RT). Higher OC, and autonomic arousal at pretest, were associated with poorer choice-RT. Higher levels of the disposition/cognitive style, OC, were more associated with poorer choice RT than the ERI ratio which assesses structural elements of the academic environment. Specifically, OC mediated the relationship between ERI and low choice RT. Finally, in the absence of OC, sAA at pretest moderated the relationship between ERI and choice RT, suggesting that increased SAM arousal may be a more important predictor of poor choice RT than ERI.
4.1. University stress and reaction-time
The sample in the present investigation had substantially lower levels of academic stress as assessed by ERI, than a large study of medical students from Germany (Wege et al., Citation2017). Potentially, the difference may be attributed to cultural factors or the different study requirements in-between countries, but we would argue that an ERI ratio of .80 is considerably higher than many studies of employee groups and as such, would suggest that the samples were experiencing chronic stress. This is further demonstrated by the fact that 20% of students reported an ERI >1, which may predict poor health (Siegrist, Citation2010).
The psychosocial variables ERI and OC were not related to simple RT. However, HRV at pretest was associated with poorer simple RTs. Higher levels of OC and higher SDNN and lower RMSSD at pretest were related to poorer choice RTs. Similar to our earlier work (Landolt et al., Citation2017), the more difficult task (choice-RT) appears to be more affected by autonomic arousal. Although speculative, the relationship between autonomic arousal and cognitive performance may be moderated by cognitive demand.
Pabst, Brand, and Wolf. (Citation2013) reported the impacts of both time from stressor exposure, and sAA and cortisol secretion upon decision-making. Similarly, our findings show how related measures of the anticipatory physiological stress response can have different associations with simple and choice RT. In the 26 studies included in the Starcke and Brand (Citation2016) meta-analysis, the majority of physiological measurements were either cortisol or sAA, and only one assessed HRV. The use of continuously recorded HRV assessments, where the measurement of the physiological response is less impacted by a time-lag than sAA or cortisol, may explain the significant associations between HRV and choice RT in the present investigation. That said, the measurement of circulating cortisol has obvious advantages, as higher cortisol levels appear to have a direct impact upon decision-making (Pabst et al., Citation2013).
The impact of acute stress upon HRV has recently been the subject of a meta-analysis with acute psychological stress related to decreased RMSSD and decreased SDNN (Castaldo et al., Citation2015). However, the SDNN findings were highly heterogeneous (I2 = 92%, p < .01) with some data suggesting increased SDNN in response to acute stress. HRV measures at pretest were associated with decision-making, but as the Castaldo et al. (Citation2015) meta-analysis reports exclusively on HRV changes between study phases, and not pretest data specifically, further empirical evidence is required to determine the meaningfulness of our finding.
Overcommitted individuals experience an ERI more intensely due to overestimating their own capabilities and underestimating work demands (Siegrist & Li, Citation2016). Poor coping skills are a characteristic of OC and the theoretical path of the stress and coping model (Lazarus & Folkman, Citation1984), would suggest that OC is more likely than ERI to lead to dysregulated physiological reactivity and poor RT. The stress and coping model suggests it is not the stressor per se, but the inability to cope with stress, and the negative emotions this elicits, that triggers dysregulated physiological reactivity.
Others have reported on the associations between markers of psychological and physiological stress and poor-decision-making and RTs (e.g. Landolt et al., Citation2017; van Honk et al., Citation2003), but we could not locate research that had linked factors such as OC to poor decision-making or RT. Our finding that sAA reactivity moderated the association between ERI and choice-RT aligns with two recent studies (Landolt et al., Citation2017; Radenbach et al., Citation2015) where cortisol and HRV respectively, moderated the link between chronic stress and poor RT. However, similar to the findings in the acute stress and decision-making literature (Starcke & Brand, Citation2016), differences in stress exposure, gender, age, design, and physiological markers between chronic stress and decision-making studies, compromise the conclusions that can be drawn from this small literature.
In summary, the findings suggest that high ERI or OC may not be the single key driver in the relationship with poor RT and that increased autonomic arousal to an upcoming stressor may be an important predictor of poor choice RT. When considered with other findings of decreased cognitive functioning (for example, reduced episodic memory) in association with increased circulating glucocorticoids (Wolf, Citation2009), findings from this study provide strong evidence to further test associations of chronic stress physiology with measures of RT.
4.3. Implications
Our findings provide further support for the broader applicability of the ERI model in university settings as higher OC and ERI were associated with poorer choice RTs. Potentially, higher-order decision-making (i.e. choice RT vs. simple RT) may be more impacted by chronic stress and autonomic arousal than more simple tasks. This finding matches earlier research (Landolt et al., Citation2017), but more empirical work with a broader array of decision-making tasks is required to substantiate this claim. With poor decision-making now added to the potential negative outcomes associated with the experience of a stressful university environment, it is important to establish preventative or health-promoting strategies for students (Wege et al., Citation2017).
4.4. Strengths and limitations
We used a male-only sample due to the reported sex differences in physiological reactivity and this impacted our ability to generalize our findings to female students. While we consciously opted for internal over external validity, we can report that our sample size (n = 79) more than doubles that of the only other research that has concurrently assessed chronic stress, psychophysiology and decision-making (i.e. Landolt et al., Citation2017, N = 32; Radenbach et al., Citation2015, N = 39; van Honk et al., Citation2003 N = 30). However, our findings regarding the ERI scores as a measure of chronic stress should be treated with some caution given the modest internal consistency, and the fact that we did not assess factors beyond university life that may have impacted perceptions of chronic stress. Additionally, we did not control for potential confounders of sAA and HRV measurements such as body mass index, smoking, or mental health, but would suggest that the impact of these variables on the outcomes is limited to a certain degree given the within-group design employed. Our research incorporated a cross-sectional design and therefore inferences cannot be made regarding causality, but unlike traditional cross-sectional studies, we assessed within-participant changes at two time-points for assessments of HRV and sAA. Finally, to counteract potential issues with inflated Type I error due to common method variance, this study incorporated psychological, behavioral (RT), and physiological variables of stress.
4.5. Conclusions
This is the first study with university students to use the validated ERI model to assess the relationships between chronic stress and RT utilizing psychological, behavioral, and physiological measures. A student’s career, degree, and grades may be predicated on decision-making within exams and other academic tasks, and our findings suggest that students who are higher in OC and are more physiologically reactive (higher SDNN, lower RMSSD) to stressors may be more “at-risk” of poor decision-making than others. If others can replicate our findings in more diverse samples, this will contribute to an evidence base for targeted intervention.
Disclosure statement
No potential conflict of interest was reported by the authors.
Additional information
Notes on contributors
Raymond Kuhnell
Mr Raymond Kuhnell has completed a degree in Psychological Science (Honours) at La Trobe University and has worked on several projects assessing the relationships between stress and physiological reactivity.
Zoe Whitwell
Ms Zoe Whitwell has completed a degree in Psychological (Honours) at La Trobe University and has worked on several projects assessing the relationships between stress and physiological reactivity.
Steven Arnold
Mr Steven Arnold has completed a degree in Psychological Science (Honours) at La Trobe University and has worked on several projects assessing the relationships between stress and physiological reactivity.
Michael I. C. Kingsley
Michael Kingsley is Associate Professor of Exercise Physiology and Director of Research for La Trobe Rural Health School, Australia. He is an Accredited Exercise Scientist with particular expertise in the measurement and evaluation of physiological responses to stress.
Matthew W. Hale
Matthew Hale is Senior Lecturer and neuroscientist at La Trobe University. His research is focused on understanding the neuronal circuitry that underlies emotional states such as anxiety, stress and sociability.
Morten Wahrendorf
Morten Wahrendorf is head of the working group “work and health” at the Institute for Medical Sociology University Düsseldorf, Germany. His research areas are work stress, health inequalities, life course epidemiology, comparative welfare research and ageing.
Nico Dragano
Nico Dragano is a Professor for Medical Sociology at the University Hospital Düsseldorf, Germany. Main fields of interest are psychosocial risk factors at work, digitization of work, cardiovascular disease epidemiology and occupational prevention. He is affiliated with international networks, e.g. the IPD-Work consortium on stress research in European cohort studies.
Bradley J. Wright
Bradley Wright is a Senior Lecturer at La Trobe University and conducts research in the area of performance psychology. He has researched the links between occupational stress (individual and organisational factors) and ensuing ill-health by focussing on pre-clinical indices of stress and ill-health (immune, cardiovascular and hormonal systems).
References
- Baradell, J.G., & Klein, K. (1993). Relationship of life stress and body consciousness to hypervigilant decision making. Journal of Personality and Social Psychology, 64, 267–273. doi:10.1037//0022-3514.64.2.267
- Bauer, D.J., & Curran, P.J. (2005). Probing interactions in fixed and multilevel regression: Inferential and graphical techniques. Multivariate Behavioral Research, 40, 373–400. doi:10.1207/s15327906mbr4003_5
- Castaldo, R., Melillo, P., Bracale, U., Caserta, M., Triassi, M., & Pecchia, L. (2015). Acute mental stress assessment via short term HRV analysis in healthy adults: a systematic review with meta-analysis. Biomedical Signal Processing and Control, 18, 370–377. doi:10.1016/j.bspc.2015.02.012
- Eddy, P., Heckenberg, R., Wertheim, E.H., Kent, S., & Wright, B.J. (2016). A systematic review and meta-analysis of the effort-reward imbalance model of workplace stress with indicators of immune function. Journal of Psychosomatic Research, 91, 1–8. doi:10.1016/j.jpsychores.2016.10.003
- Eddy, P., Wertheim, E.H., Hale, M.W., & Wright, B.J. (2018). A systematic review and meta-analysis of the effort-reward imbalance model of workplace stress and hypothalamic-pituitary-adrenal axis measures of stress. Psychosomatic Medicine, 80, 103–113. doi:10.1097/PSY.0000000000000505
- Eddy, P., Wertheim, E.H., Kingsley, M., & Wright, B.J. (2017). Associations between the effort-reward imbalance model of workplace stress and indices of cardiovascular health: A systematic review and meta-analysis. Neuroscience & Biobehavioral Reviews, 83, 252–266. doi:10.1016/j.neubiorev.2017.10.025
- Feldman, P., Cohen, S., Hamrick, N., & Lepore, S.J. (2004). Psychological stress, appraisal, emotion and cardiovascular response in a public speaking task. Psychology & Health, 19, 353–368. doi:10.1080/0887044042000193497
- Gordis, E.B., Granger, D.A., Susman, E.J., & Trickett, P.K. (2006). Asymmetry between salivary cortisol and α-amylase reactivity to stress: Relation to aggressive behavior in adolescents. Psychoneuroendocrinology, 31, 976–987. doi:10.1016/j.psyneuen.2006.05.010
- Hair, J.F., Black, W.C., Babin, B.J., & Anderson, R.E. (2010). Multivariate data analysis: A global perspective (7th ed.). New Jersey: Pearson.
- Hayes, A.F. (2013). Introduction to mediation, moderation, and conditional process analysis: A regression-based approach. New York, NY: Guilford Press.
- Johnson, P.O., & Fay, L.C. (1950). The Johnson-Neyman technique, its theory and application. Psychometrika, 15, 349–367. doi:10.1007/BF02288864
- Landolt, K., Maruff, P., Horan, B., Kingsley, M., Kinsella, G., O’Halloran, P.D., … Wright, B.J. (2017). Chronic work stress and decreased vagal tone impairs decision making and reaction time in jockeys. Psychoneuroendocrinology, 84, 151–158. doi:10.1016/j.psyneuen.2017.07.238
- Lazarus, R.S., & Folkman, S. (1984). Stress, appraisal, and coping. New York: Springer Publishing Co.
- Maruff, P., Falleti, M.G., Collie, A., Darby, D., & McStephen, M. (2005). Fatigue-related impairment in the speed, accuracy and variability of psychomotor performance: comparison with blood alcohol levels. Journal of Sleep Research, 14, 21–27. doi:10.1111/j.1365-2869.2004.00438.x
- Nater, U.M., Abbruzzese, E., Krebs, M., & Ehlert, U. (2006). Sex differences in emotional and psychophysiological responses to musical stimuli. International Journal of Psychophysiology, 62, 300–308. doi:10.1016/j.ijpsycho.2006.05.011
- Nater, U.M., La Marca, R., Florin, L., Moses, A., Langhans, W., Koller, M.M., & Ehlert, U. (2006). Stress- induced changes in human salivary alpha- amylase activity— associations with adrenergic activity. Psychoneuroendocrinology, 31, 49–58. doi:10.1016/j.psyneuen.2005.05.010
- Nater, U.M., Rohleder, N., Gaab, J., Berger, S., Jud, A., Kirschbaum, C., & Ehlert, U. (2005). Human salivary amylase reactivity in a psychosocial stress paradigm. International Journal of Psychophysiology, 55, 333–342. doi:10.1016/j.ijpsycho.2004.09.009
- Nater, U.M., Rohleder, N., Schlotz, W., Ehlert, U., & Kirschbaum, C. (2007). Determinants of the diurnal course of salivary alpha-amylase. Psychoneuroendocrinology, 32, 392–401. doi:10.1016/j.psyneuen.2007.02.007
- Noorani, I., & Carpenter, R.H.S. (2016). The LATER model of reaction time and decision. Neuroscience & Biobehavioral Reviews, 64, 229–251. doi:10.1016/j.neubiorev.2016.02.018
- O’Donnell, E., Landolt, K., Hazi, A., Dragano, N., & Wright, B.J. (2015). An experimental study of the job demand–control model with measures of heart rate variability and salivary alpha-amylase: Evidence of increased stress responses to increased break autonomy. Psychoneuroendocrinology, 51, 24–34. 2014.09.017 doi:10.1016/j.psyneuen
- Pabst, S., Brand, M., & Wolf, O.T. (2013). Stress and decision making: A few minutes make all the difference. Behavioural Brain Research, 250, 39–45. doi:10.1016/j.bbr.2013.04.046
- Putman, P., Antypa, N., Crysovergi, P., & van der Does, W.A. (2010). Exogenous cortisol acutely influences motivated decision making in healthy young men. Psychopharmacology, 208, 257. doi:10.1007/s00213-009-1725-y
- Radenbach, C., Reiter, A.M.F., Engert, V., Sjoerds, Z., Villringer, A., Heinze, H.-J., … Schlagenhauf, F. (2015). The interaction of acute and chronic stress impairs model-based behavioral control. Psychoneuroendocrinology, 53, 268–280. doi:10.1016/j.psyneuen.2014.12.017
- Siegrist, J. (1996). Adverse health effects of high-effort/low-reward conditions. Journal of Occupational Health Psychology, 1, 27–41. doi:10.1037/1076-8998.1.1.27
- Siegrist, J. (2010). Effort-reward imbalance at work and cardiovascular diseases. International Journal of Occupational Medicine and Environmental Health, 23, 279–285. doi:10.2478/v10001-010-0013-8
- Siegrist, J., & Li, J. (2016). Associations of extrinsic and intrinsic components of work stress with health: a systematic review of evidence on the effort-reward imbalance model. International Journal of Environmental Research and Public Health, 13, 432. doi:10.3390/ijerph13040432
- Smeets, T., Cornelisse, S., Quaedflieg, C.W., Meyer, T., Jelicic, M., & Merckelbach, H. (2012). Introducing the Maastricht Acute Stress Test (MAST): A quick and non-invasive approach to elicit robust autonomic and glucocorticoid stress responses. Psychoneuroendocrinology, 37, 1998–2008. doi:10.1016/j.psyneuen.2012.04.012
- Starcke, K., & Brand, M. (2012). Decision making under stress: a selective review. Neuroscience & Biobehavioral Reviews, 36, 1228–1248. doi:10.1016/j.neubiorev.2012.02.003
- Starcke, K., & Brand, M. (2016). Effects of stress on decisions under uncertainty: A meta-analysis. Psychological Bulletin, 142, 909. doi:10.1037/bul0000060
- Stewart-Brown, S., Evans, J., Patterson, J., Petersen, S., Doll, H., Balding, J., & Regis, D. (2000). The health of students in institutes of higher education: an important and neglected public health problem? Journal of Public Health, 22, 492–499. doi:10.1093/pubmed/22.4.492
- Tabachnick, B.G., & Fidell, L.S. (2014). Using Multivariate Statistics (6th ed.). Essex, United Kingdom: Pearson.
- Task Force of the European Society of Cardiology and the North American Society of Pacing and Electrophysiology. (1996). Heart rate variability: standards of measurement, physiological interpretation and clinical use. Task Force of the European Society of Cardiology and the North American Society of Pacing and Electrophysiology. Circulation, 93, 1043–1065. doi:10.1161/01.CIR.93.5.1043
- Thayer, J.F., Yamamoto, S.S., & Brosschot, J.F. (2010). The relationship of autonomic imbalance, heart rate variability and cardiovascular disease risk factors. International Journal of Cardiology, 141, 122–131. doi:10.1016/j.ijcard.2009.09.543
- Tsutsumi, A., & Kawakami, N. (2004). A review of empirical studies on the model of effort–reward imbalance at work: reducing occupational stress by implementing a new theory. Social Science & Medicine, 59, 2335–2359. doi:10.1016/j.socscimed.2004.03.030
- Vaez, M., Kristenson, M., & Laflamme, L. (2004). Perceived quality of life and self-rated health among first-year university students: A comparison with their working peers. Social Indicators Research, 68, 221–234. doi:10.1023/B:SOCI.0000025594.76886.56
- van Honk, J., Schutter, J.L.G.D., Hermans, J.E., & Putman, J.P. (2003). Low cortisol levels and the balance between punishment sensitivity and reward dependency. NeuroReport, 14, 1993–1996. doi:10.1097/00001756-200310270-00023
- Wege, N., Li, J., Muth, T., Angerer, P., & Siegrist, J. (2017). Student ERI: Psychometric properties of a new brief measure of effort-reward imbalance among university students. Journal of Psychosomatic Research, 94, 64–67. doi:10.1016/j.jpsychores.2017.01.008
- Wolf, O.T. (2009). Stress and memory in humans: Twelve years of progress? Brain Research, 1293, 142–154. doi:10.1016/j.brainres.2009.04.013