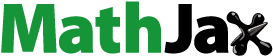
Abstract
Chronic stress is a well-established risk factor for cardiometabolic disease. Caregiving for individuals with cancer is perceived as a chronic stressor yet research on the risk for cardiometabolic disease in this population, opposed to the elderly and those with Alzheimer’s disease, is limited. Additionally, few studies have explored the early physiological changes that occur in family caregivers suggesting an elevated risk for illness. This cross-sectional study was designed to examine levels of cardiometabolic risk biomarkers and their correlates in caregivers of patients with colorectal cancer. Caregivers completed questionnaires that measure exposures to stress and vulnerability factors, psychological distress, and health habits as potential correlates. Traditional lipid and nontraditional lipoprotein particle biomarkers (e.g. concentration and size for all lipoprotein classes) were assayed from blood serum. Caregivers (N = 83, mean age = 49.8, 73% female) displayed levels of cardiometabolic biomarkers that suggest an elevated risk for cardiometabolic disease. Caregivers who were Hispanic, married, highly educated, employed, reported more hours spent caregiving daily, experienced higher caregiver burden associated with the lack of family support and impact on schedule, and psychological distress, demonstrated an elevated risk for cardiometabolic disease; primarily determined by nontraditional lipid biomarkers – large TRL-P, LDL-P, small HDL-P, large HDL-P, TRL-Z, LDL-Z and HDL-Z. These findings suggest that traditional lipid biomarkers may not be robust enough to detect early physiological changes associated with cardiometabolic disease risk in family caregivers. Moreover, findings reiterate the importance of assessing caregiver burden and providing evidence-based interventions to manage caregiving stress with the potential to improve caregivers’ cardiometabolic health.
Introduction
More than 2.9 million individuals served as caregivers for a family member or friend with cancer in the United States in 2020 (National Alliance for Caregiving [NAC], Citation2020). Caring for an ill family member is a psychologically demanding experience (Jansen et al., Citation2018), which is associated with poorer physical health (Kim et al., Citation2015; Shaffer et al., Citation2017) and negative changes in health promoting behaviors, such as physical activity and healthy diet (Ross et al., Citation2020). Accordingly, it is important to identify sub-groups of individuals who are at higher risk for developing health problems (NAC, Citation2020).
Biomarkers, which are defined as “biological molecules found in blood, other body fluids, or tissues that are signs of normal or abnormal processes, conditions, or diseases” (National Cancer Institute, Citation2015), can be useful indicators of caregivers’ overall health and well-being (Park et al., Citation2018). Biomarkers are objective measures, distinct from self-reported measures, which offer additional depth to the assessment of an individual’s health status. A comprehensive assessment of biomarkers may help identify precise pathways to poor long-term health outcomes (Corwin & Ferranti, Citation2016). The cardiovascular health of family caregivers is an imperative research priority, as cumulating evidence suggests cancer caregiving may be a risk factor for developing cardiometabolic diseases (Buyck et al., Citation2013; Ji et al., Citation2012; Kim et al., Citation2015; Lee et al., Citation2003; Ross et al., Citation2017), the leading cause of death in the U.S. (Centers for Disease Control & Prevention [CDC], Citation2017).
Existing studies with caregivers have identified several coagulation factors, such as D-dimer, von Willebrand factor, plasminogen activator, and P-selectin, as predictors of cardiovascular outcomes (Aschbacher et al., Citation2009; von Känel et al., Citation2010a; Citation2010b). More recent evidence, although not from caregivers, has suggested that more novel biomarkers of lipid metabolism, may be stronger predictors of cardiovascular outcomes (Clarke & Marzinke, Citation2020; Jeyarajah et al., Citation2006; Otvos et al., Citation2011). These novel biomarkers of lipid metabolism are identified using nuclear magnetic resonance (NMR) spectroscopy, a nontraditional approach, and measure particle concentrations for all lipoprotein classes [triglyceride-rich lipoprotein (TRL), low-density lipoprotein (LDL), and high-density lipoprotein (HDL)] and subclasses (large, medium, and small), as well as particle sizes for TRL, LDL, and HDL. This lipoprotein-focused approach has been proposed as an alternate to the enzymatically measured lipid concentrations (traditional lipids): total cholesterol, high-density lipoprotein cholesterol (HDL-C), low-density lipoprotein cholesterol (LDL-C), and triglycerides, because the traditional lipids can differ among individuals due to both variations in particle size as well as metabolic processes (Otvos et al., Citation2011). The nontraditional lipid biomarkers have greater sensitivity and specificity in predicting cardiovascular disease outcomes compared with traditional lipids in non-caregiver populations with and without cardiovascular disease (Clarke & Marzinke, Citation2020; Jeyarajah et al., Citation2006; Kontush, Citation2015; Matyus et al., Citation2014; Otvos et al., Citation2011; Urbina et al., Citation2017).
While the evidence supporting the superiority of the nontraditional lipid metabolism biomarkers has been accumulating (Matyus et al., Citation2014; Urbina et al., Citation2017), only one study to date demonstrated that the nontraditional lipid panel was more robust in identifying at-risk individuals than traditional lipid biomarkers among caregiver populations (Ross et al., Citation2017). Identifying biomarkers that play an important role in caregivers’ cardiovascular health will help advance the science by elucidating the potential mechanisms linking caregiving to downstream effects on cardiometabolic health (Corwin & Ferranti, Citation2016; Vitaliano et al., Citation2003).
Vitaliano et al., (Citation2003) present a theoretical model of stress and health/illness that calls attention to potential various factors (exposure to stress and vulnerability, psychological distress, risky health habits, physiological responses) that might contribute to illness in caregivers. Adapting this framework based on the illness of interest, cardiometabolic disease, is critical to increase the scientific rigor of the analysis. Based on the framework (Vitaliano et al., Citation2003) and previous research (National Institutes of Health, Citation2019), exposure to stress and vulnerability factors considered in this study as possibly contributing to cardiometabolic disease risk included sociodemographic (age, sex, race/ethnicity, marital status, education, employment status, relationship to the patient), caregiver morbidities, and caregiving stress (patients’ cancer stage, hours spent for caregiving daily, perceived caregiving burden). Psychological distress included mood disturbance. Possible health habits included physical activity, alcohol consumption, and fruit and vegetable consumption. These factors that may influence physiological responses and subsequent cardiometabolic health problems are therefore reflected in this study’s conceptual model (). This study aimed to document levels of cardiometabolic risk biomarkers (the traditional and nontraditional lipid biomarkers) and investigate factors (exposure to stress and vulnerability, psychological distress, and health habits) that are associated with cardiometabolic risk biomarkers in family caregivers of patients with colorectal cancer.
Figure 1. Conceptual model of stress and health/illness. *Adapted for the Analysis of Cardiometabolic Health in Cancer Caregivers.
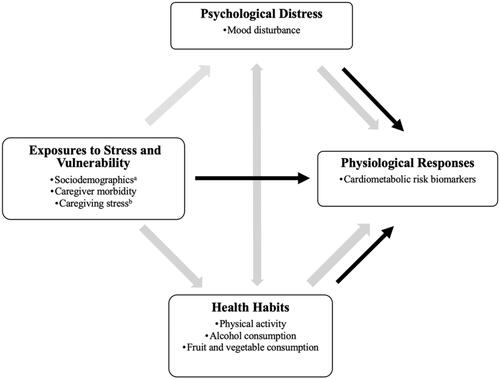
Methods
Study design and participants
Participants were family caregivers of individuals who were diagnosed with colon or rectal cancer from oncology clinics in Miami, FL., approximately four months prior to participating in the study. Eligibility criteria were: (a) 18 years or older, (b) able to read and speak English or Spanish, and (c) self-identified non-Hispanic White, Hispanic, or African American. Of 85 caregivers enrolled in the study between 2012 and 2015, a total of 83 caregivers were included in the analyses for this study; two had missing or incomplete outcome data. This study is based on cross-sectional analysis of the initial assessment of a longitudinal study that was to evaluate the quality of life among caregivers of patients with cancer (121909-RSG-12-042-01-CPPB). All participants provided written informed consent before participation, which was approved by the University of Miami Institutional Review Board.
Measures
Caregivers were asked to complete a sociodemographic, caregiver morbidity, caregiver stress, psychological distress, and health habit questionnaire at home or in the clinic. Before completing the questionnaire, non-fasting blood samples were drawn by the study phlebotomist.
Exposures to stress and vulnerability
Three categories represent exposures to stress and vulnerability factors. First, sociodemographic factors included self-reported age, gender, race/ethnicity, marital status, education, employment status, household income and relationship to the patient. Second, caregiver morbidity was assessed using the Medical Morbidities Index for Caregivers of Chronic Illnesses (MMICCI: Kim et al., Citation2013). The MMICCI quantifies the number of health conditions that required physician’s care. The caregiver responded “yes or no” if they ever received a physician’s care or had taken prescription medication on a list of 40 medical conditions. A higher count of “yes” responses indicates more morbid conditions. Third, caregiving stress was quantified by three sub-factors, including (1) the patient’s cancer stage, (2) hours spent for caregiving daily, and (3) perceived caregiving burden. The cancer stage was extracted from the medical record or patients’ self-report when the medical record information was not available. The number of hours spent for caregiving daily was determined by asking “How many hours a day have you been helping this person through his/her experience with cancer?” The hours spent by caregivers caring for patients were categorized into three groups: (1) <8 hours/day; (2) 8–16 hours/day; and (3) >16 hours/day (Yabroff & Kim, Citation2009). Perceived caregiving burden was assessed using the Caregiving Reaction Assessment (CRA: Given et al., Citation1992). Caregivers completed four subscales of the CRA: (a) impact on schedule (5 items); (b) lack of family support (5 items); (c) impact on finance (3 items); and d) caregiver esteem (7 items), using a 5-point Likert-type response option (1 = strongly disagree to 5 = strongly agree). Each subscale score was computed by taking the mean of the scale items, after reversing coded, if necessary, with higher scores suggesting more burden, except caregiver esteem. The subscales had acceptable internal consistency in the current sample (Cronbach alpha for impact on schedule = 0.77; lack of family support = 0.72; impact on finance = 0.71; and caregiver esteem = 0.79).
Psychological distress
Mood disturbance was assessed using a 30-item Profile of Mood States Short Form (POMS-SF: McNair et al., Citation1992). The POMS-SF consists of six subscales: depression, tension, anger, confusion, fatigue, and vigor. A total score of mood disturbance was calculated by subtracting the sum score of vigor from the sum of the other five subscales. Higher mood disturbance scores indicate greater psychological distress. The reliability was good with the current sample, α = 0.90.
Health habits
Indicators of health habits included (1) physical activity, (2) alcohol consumption, and (3) fruit and vegetable consumption. Physical activity was assessed using the International Physical Activity Questionnaire (IPAQ: Booth, Citation2000) for the amount of vigorous activity, moderate activity, walking, and sitting over the last seven days. Physical activity levels were categorized into three groups: (1) high (e.g. vigorous intensity activity on at least 3 days achieving a minimum total physical activity of at least 1500 MET minutes a week); (2) moderate (e.g. 3 or more days of vigorous intensity activity and/or walking of at least 30 minutes per day); and (3) low (not meeting any of the criteria for either of the previous categories). Alcohol consumption was assessed using a single item, “On days you do drink, how many drinks of alcohol (beer, wine, liquor) do you have on average?” Alcohol consumption levels were categorized into three groups according to American Heart Association (Citation2018): (1) no drinking; (2) moderate drinking (one to two drinks per days for men and one drink per day for women); and (3) heavy drinking (more than two drinks per days for men and more than one drink per day for women). Fruit and vegetable consumption was assessed by asking “How many days per week do you eat at least five servings of fruits and vegetables (i.e. 1 medium sized piece of fruit, 1 cup of raw, leafy vegetables, ½ cup of cooked beans or peas, 6 oz. of 100% fruit or vegetable juice, ¼ cup of dried fruit)?” Responses were dichotomized: meet American Cancer Society (ACS) recommendations (≥ 5 days per a week) vs. do not meet (<5 days per a week).
Cardiometabolic risk biomarkers
Traditional and nontraditional lipid biomarkers, as measures of cardiometabolic diseases risk, were quantified from blood serum samples using a further-optimized deconvolution algorithm, called the fourth-generation lipoprotein profile algorithm (LP4), NMR spectroscopy (Clarke & Marzinke, Citation2020). The lipid biomarkers were calculated from the amplitudes of their spectroscopically unique lipid methyl group NMR signals. Relative mass percentage was also quantified from methyl group signals, which gave estimations of concentrations and sizes of lipoprotein subclass by size. First, the traditional lipid panel included total cholesterol, HDL-C, LDL-C, and triglycerides. Second, the nontraditional lipid biomarker panel included the lipoprotein particle concentration for all lipoprotein classes (TRL, LDL, HDL), subclasses (large, medium, small), and particle size (diameter) for TRL, LDL, and HDL (Clarke & Marzinke, Citation2020; Jeyarajah et al., Citation2006). Based on the existing literature (Jeyarajah et al., Citation2006), this study included large TRL particle concentration (large TRL-P) and particle size (TRL-Z), LDL cholesterol particle concentration (LDL-P), small LDL-P and particle size (LDL-Z), and HDL particle concentration (HDL-P), large HDL-P, small HDL-P, and size (HDL-Z). Higher cholesterol and triglycerides levels suggest increased risk for cardiometabolic disease. Furthermore, higher levels of LDL-C, large TRL-P, TRL-Z, LDL-P, small LDL-P, and small HDL-P along with lower levels of HDL-C and HDL-P, large HDL-P, LDL-Z, and HDL-Z are also associated with increased risk for cardiometabolic disease (Clarke & Marzinke, Citation2020; Jeyarajah et al., Citation2006).
Statistical analysis
Means, standard deviation, frequencies, and percentage were reported for all variables. Data distribution and normality were checked using the Shapiro-Wilk tests and normal QQ plots for all continuous variables. Bivariate parametric (Pearson’s correlation, t-test, and one-way analysis of variance) or non-parametric tests (Spearman’s correlation, Wilcoxon signed-rank test, and Kruskal-Wallis test) were used to examine the bivariate associations of cardiometabolic biomarkers with exposures to stress and vulnerability (age, sex, race/ethnicity, marital status, education, employment status, the relationship to the patient, caregiver morbidity, the patient’s cancer stage, hours spent caregiving daily, and four aspects of perceived caregiving burden), psychological distress (mood disturbances), and health habits (physical activity, alcohol consumption, and fruit and vegetable consumption). Hierarchical regression models were used to test the primary research inquiry identifying predictors of traditional and nontraditional lipid biomarkers. Known factors such as age, sex, and caregiver morbidity (Gao et al., Citation2019; Tran et al., Citation2018) were entered in the first block. Other factors including exposures to stress and vulnerability, psychological distress, and health habits, were entered in the second block if they were correlated with each cardiometabolic lipid biomarker at p < 0.20 in the bivariate analysis. Normality and homoscedasticity were checked by residual and normal plot. Transformations were performed if necessary. Multicollinearity was checked by tolerance and variance inflation factor (VIF). Statistical analyses were performed with IBM SPSS statistics (IBM Corp., Armonk, NY, USA), with an alpha level of 0.05.
Results
Sample characteristics
As shown in , the majority of participants were middle-aged (49.814.4), female (72.8%), Hispanic (65.5%), married (71.6%), employed (61.5%), and had some college education (65.4%). Caregivers reported, on average, three medical comorbid conditions, most of them spent less than eight hours providing care to the patients (53.4%) who were diagnosed with stage II or III colorectal cancer (52.8%). The levels of traditional lipid and nontraditional lipoprotein particle biomarkers are presented in .
Table 1. Characteristics of the sample and study variables (N = 83).
Table 2. Comparison among caregivers of colorectal cancer and reference range values on cardiometabolic disease risk factors.
Bivariate analyses of key factors related to lipoprotein biomarkers
Bivariate analyses for traditional lipids and nontraditional lipoprotein particle biomarkers are shown in Supplementary Table S1. The findings revealed that the exposures to stress and vulnerability factors were mainly correlated with nontraditional lipid biomarkers. Specifically, older age was correlated with higher levels of triglyceride (p = 0.002), large TRL-P (p = 0.005), small LDL-P (p = 0.020), and lower levels of LDL-Z (p = 0.019). Males had significantly higher levels of triglycerides (p = 0.014), and lower levels of LDL-Z (p = 0.011) and HDL-Z (p = 0.012). Non-Hispanic/non-White caregivers had a higher level of large HDL-P than non-Hispanic/White (p = 0.029) and Hispanic (p = 0.032) caregivers. Being married was correlated with higher levels of triglyceride (p = 0.001), large TRL-P (p = 0.015), and lower levels of LDL-Z (p = 0.018) and HDL-Z (p = 0.038). Being employed was correlated with higher levels of LDL-C (p = 0.016), and LDL-Z (p = 0.032). A greater number of comorbidities was correlated with higher levels of triglyceride (p < 0.001), large TRL-P (p < 0.001), LDL-P (p = 0.047), small LDL-P (p = 0.001) and TRL-Z (p = 0.048), and lower levels of LDL-Z (p = 0.003) and HDL-Z (p = 0.044).
Relative to caregiver stress, factors representing caregiver burden were significantly related to nontraditional biomarkers. A greater impact on finances as part of perceived caregiving burden was associated with lower levels of large HDL-P (p = 0.019) and HDL-Z (p = 0.024) while a lower level of caregiver esteem was associated with higher levels of small LDL-P (p = 0.029) and TRL-Z (p = 0.016), and lower levels of LDL-Z (p = 0.018).
Multiple regression models: Traditional lipid and nontraditional lipoprotein particle biomarkers
Multiple linear regression models on traditional and nontraditional lipid biomarkers were tested (). Detailed results on multiple linear regression models with all covariates can be found in the Supplementary Table S2. Multiple collinearity of independent variables showed that tolerance and VIF ranged from 0.393 − 0.890, and 1.040 − 2.267 respectively in all models. Among traditional lipid biomarkers, employed caregivers had higher levels of total cholesterol and LDL-C=0.325, t = 1.434, p = 0.007;
=0.343, t = 2.960, p = 0.004, respectively). Caregivers who had completed associate degree/some college had lower levels of LDL-C than those who had a graduate or professional degree (
= −0.340, t = −2.311, p = 0.024). Non-Hispanic/non-White caregivers had lower levels of triglyceride than Hispanic caregivers (
= −0.258, t = −2.311, p = 0.025). Caregivers who were married (
=0.290, t = 2.700, p = 0.009) and perceived lack of family support (
=0.285, t = 2.923, p = 0.005) had higher levels of triglycerides. The only significant predictors associated with HDL-C levels were sex (male) and number of morbidities (higher) (F(8, 58) = 2.672, p = 0.014). The proposed set of predictors accounted for 12.9–50.4% of the variance in these traditional lipid biomarkers.
Table 3. Multiple regressions analysis on traditional lipids and nontraditional lipoprotein particle biomarkers.
Among nontraditional lipid biomarkers, caregivers who were married (=0.340, t = 3.327, p = 0.002) and perceived lack of family support (
=0.277, t = 2.463, p = 0.017) had a higher level of large TRL-P. Providing care 8–16 hours daily were associated with lower levels of TRL-P compared to those providing care >16 hours daily (
=−0.225, t = −2.074, p = 0.043). Caregivers with associate degree/some college had lower levels of LDL-P compared to those with a graduate or professional degree (
= −0.343, t = −2.327, p = 0.023), and employed caregivers had higher levels of LDL-P (
=0.254, t = 2.187, p = 0.032). Caregivers who reported less perceived impact on schedule (
= −0.262, t = −2.159, p = 0.034) and greater mood disturbance (
=0.309, t = 2.500, p = 0.015) had higher levels of small HDL-P. Caregivers who perceived lack of family support (
=−0.288, t = −2.336, p = 0.023) had lower levels of large HDL-P. Caregivers providing care <8 hours and 8–16 hours daily had higher levels of TRL-Z and LDL-Z (
=0.361, t = 2.548, p = 0.014;
=0.266, t = 2.210, p = 0.031, respectively) than those providing care >16 hours daily. Finally, caregivers who were married (
=−0.245, t = −2.095, p=.041) and perceived lack of family support (
=−0.257, t = −2.172, p = 0.034) had lower level of HDL-Z. The proposed set of predictors accounted for 10.7% to 38.6% of the variance in these nontraditional lipid biomarkers. None of the proposed predictors were significantly associated with small LDL-P (F(9, 57)=2.433, p = 0.020). The final model for HDL-P was not significant (F(12, 51)=1.309, p = 0.243).
Discussion
The findings of this study support existing literature suggesting that the chronic stress and burden of caregiving may increase the risk of cardiometabolic disease, more specifically a risk toward metabolic dysfunction, among family caregivers of individuals with cancer (Ji et al., Citation2012; Kim et al., Citation2015; Lee et al., Citation2003; Ross et al., Citation2017). The caregivers in this study reported a relatively high level of caregiver burden, particularly regarding a lack of family support, that was comparable to caregivers of patients undergoing allogeneic hematopoietic stem cell transplantation (Ross et al., Citation2017), a therapy notorious for caregiver isolation from family and community. This specific burden was associated with levels of triglycerides, a traditional lipid biomarker, and large TRL-P, large HDL-P and HDL-Z, nontraditional lipoprotein biomarkers, that suggest an increased risk for metabolic dysfunction; a level of risk that may be underappreciated with only a traditional lipid panel. The same pattern was present with married caregivers in levels of triglycerides and large TRL-P. This new evidence builds on existing research of cardiometabolic disease biomarkers in family caregivers (Aschbacher et al., Citation2009; Buyck et al., Citation2013; Ji et al., Citation2012; Ross et al., Citation2017; von Känel et al., Citation2010a, Citation2010b) by exploring the role of nontraditional lipoprotein particle biomarkers as a set of emerging variables to assess the physiological impact of caregiving.
Prior research exploring the link between caregiver stress and cardiometabolic risk among family caregivers of patients with cancer is limited (Park et al., Citation2018); however, research in caregivers of patients with cardiovascular disease and dementia make similar conclusions - caregiver burden appears to increase risk in cardiometabolic disease (Aschbacher et al., Citation2009; Mochari-Greenberger & Mosca, Citation2012; von Känel et al., Citation2010a, Citation2010b). In addition, the results of this study are consistent with prior research exploring the relationship between many weekly hours of informal caring and the risk of cardiovascular disease. (Mortensen et al., Citation2018). More specifically, when burden is operationalized by the number of hours of caring, an increase in the number of hours is associated with an increased risk of cardiometabolic disease. The contributing factors for these physiological changes, despite the reason for serving as a caregiver, is less clear. One recurrent hypothesis is that the caregiver’s ability to attend to their own health needs is negatively affected by the demands on their time (Sisk, Citation2000). Caregivers who reported higher caregiver burden were less likely to engage in health-promoting and self-care behaviors, such as exercising regularly, maintaining a healthy diet, getting enough sleep, taking breaks and seeking preventive health care for themselves (Mochari-Greenberger & Mosca, Citation2012; Peng et al., Citation2019; Ross et al., Citation2020; Sisk, Citation2000; Vitaliano et al., Citation2003); all of which might increase the risk of cardiometabolic disease. Engaging caregivers during patient visits will help clinicians more fully understand their individual needs and to subsequently align a recommendation or intervention to reduce their burden (Jadalla et al., Citation2020). An array of evidenced based interventions is available to guide providers and reduce the caregiver burden providing “space” for self-care and attention to their own health.
The increased risk of cardiometabolic disease also may be conditional on whether the caregiver role is in conjunction with other major responsibilities, such as being married, parenting, and working outside the home (Kim et al., Citation2006; Mortensen et al., Citation2018; Schulz & Eden, Citation2016; Young & Grundy, Citation2008). For example, married people may be more likely to have responsibilities for providing care to other family members (double-duty caregiver), including a martial partner, dependent children, or a parent-in-law than the unmarried caregiver. In the case of employed caregivers, those who are working ≥55 hours weekly are at higher risk of cardiovascular disease compared to those working 35–40 hours weekly (Mortensen et al., Citation2018). Thus, caregiving stress may be amplified by competing responsibilities (e.g. spouse, parent, or employee). The findings from this study suggest that caregivers’ multiple life roles and the needs of these caregivers to detect conflicts in role-balance are important considerations when assessing caregivers’ stress and health.
A strength of this exploratory study was the inclusion of innovative nontraditional lipoprotein particle profile biomarkers. The study used the most recently developed software algorithm, LP4, which allows for a more detailed and precise investigation of cardiometabolic disease (Clarke & Marzinke, Citation2020). In addition, we included a broad range of sociodemographic, psychological and behavioral factors that could account for the differences in cardiometabolic risk in cancer caregivers. However, several limitations of this study should be acknowledged. First, the statistical analysis is cross-sectional and therefore it is not possible to ascertain whether the caregivers’ cardiometabolic health changed as a result of their caregiving experience. Longitudinal research would build on these findings and identify changes in cardiometabolic biomarkers across different caregiving trajectories. Second, the sample size for this study was relatively small for the number of tests that were performed, which might lead to an increase in the risk of spurious results in the multiple testing. Further, the current study was limited by the lack of a comparison group. These factors need to be considered in interpreting the findings. Replication of this study should include a larger sample size and employ a control or comparison group to confirm the present study’s findings. A larger sample size would permit one to empirically test relationships based on the theoretical model of stress and health/illness, such as mediation effects of psychological distress or health habits between exposures to stress/vulnerability and cardiometabolic risk biomarkers. Finally, since sex differences are pervasive in cardiometabolic diseases, more attention to the influence of sex in future studies should be explored (Gao et al., Citation2019).
Conclusion
Findings suggest family caregivers of adult cancer patients may be at increased risk for cardiometabolic illness due in part to the caregiving experience. An intriguing component of this study is the inclusion of a nontraditional set of lipoprotein particle biomarkers. Specifically, lipoprotein particle concentration and size which are more sensitive indicators of cardiometabolic risk compared to traditional measures in caregiver populations. This finding is consistent with previous studies suggesting nontraditional lipid concentration and size have a high sensitivity and specificity for detecting cardiometabolic disease risk compared to traditional lipid profile (Clarke & Marzinke, Citation2020; Matyus et al., Citation2014; Urbina et al., Citation2017).
While studies continue to explore the upstream mechanisms that contribute to increases in caregiver burden and stress, providers must continue to address the stress and burden of caregiving in practice. Caregivers may benefit from targeted interventions and support programs designed to cope with or decrease caregiving burdens to improve caregivers’ cardiometabolic health, especially those with competing responsibilities (Jadalla et al., Citation2020). Integrating nontraditional lipoprotein particle profile might provide a powerful tool for identifying cardiometabolic risk and assessing the efficacy of interventions to reduce cardiometabolic risk in caregiver population.
Supplemental Material
Download MS Word (96.9 KB)Acknowledgement
The authors thank Gwenyth Wallen, RN, PhD, Stephen Klagholz, MS, and all the families who participated in this investigation.
Disclosure statement
No potential conflict of interest was reported by the author(s).
Additional information
Funding
Notes on contributors
Lena J. Lee
Lena J. Lee, PhD, RN is a nurse scientist at National Institutes of Health Clinical Center, Bethesda, MD, USA.
Youngmee Kim
Youngmee Kim, PhD is a professor at University of Miami, Department of Psychology, Coral Gables, FL, USA.
Robert Shamburek
Robert Shamburek, MD is a clinician/scientist at National Heart, Lung, and Blood Institute, Bethesda, MD, USA.
Alyson Ross
Alyson Ross, PhD, RN was a nurse scientist at National Institutes of Health Clinical Center, Bethesda, MD, USA.
Li Yang
Li Yang, PhD is a statistician at National Institutes of Health Clinical Center, Bethesda, MD, USA.
Margaret F. Bevans
Margaret F. Bevans is a director, Office of Research Nursing at National Institutes of Health Clinical Center, Bethesda, MD, USA.
References
- American Heart Association. (2018). Alcohol and heart health. http://www.heart.org/en/healthy-living/healthy-eating/eat-smart/nutrition-basics/alcohol-and-heart-health
- Aschbacher, K., Roepke, S. K., von Känel, R., Mills, P. J., Mausbach, B. T., Patterson, T. L., Dimsdale, J. E., Ziegler, M. G., Ancoli-Israel, S., & Grant, I. (2009). Persistent versus transient depressive symptoms in relation to platelet hyperactivation: A longitudinal analysis of dementia caregivers. Journal of Affective Disorders, 116(1–2), 258–87. https://doi.org/10.1016/j.jad.2008.11.008
- Booth, M. L. (2000). Assessment of physical activity: An international perspective. Research Quarterly for Exercise and Sport, 71(sup2), 114–120. https://doi.org/10.1080/02701367.2000.11082794
- Buyck, J.-F., Ankri, J., Dugravot, A., Bonnaud, S., Nabi, H., Kivimäki, M., & Singh-Manoux, A. (2013). Informal caregiving and the risk for coronary heart disease: The Whitehall II study. The Journals of Gerontology Series A: Biological Sciences and Medical Sciences, 68(10), 1316–1323. https://doi.org/10.1093/gerona/glt025
- Centers for Disease Control and Prevention [CDC]. (2017). Deaths and mortality. https://www.cdc.gov/nchs/fastats/deaths.htm
- Clarke, W., & Marzinke, M. A. (2020). Nuclear magnetic resonance technology and clinical applications. In D. D. H. Franke & M. A. Connelly (Eds.), Contemporary practice in clinical chemistry (pp. 187–200). Academic Press.
- Corwin, E. J., & Ferranti, E. P. (2016). Integration of biomarkers to advance precision nursing interventions for family research across the life span. Nursing Outlook, 64(4), 292–298. https://doi.org/10.1016/j.outlook.2016.04.007
- Gao, Z., Chen, Z., Sun, A., & Deng, X. (2019). Gender differences in cardiovascular disease. Medicine in Novel Technology and Devices, 4, 100025. https://doi.org/10.1016/j.medntd.2019.100025
- Given, C. W., Given, B., Stommel, M., Collins, C., King, S., & Franklin, S. (1992). The caregiver reaction assessment (CRA) for caregivers to persons with chronic physical and mental impairments. Research in Nursing & Health, 15(4), 271–283. https://doi.org/10.1002/nur.4770150406
- Jadalla, A., Ginex, P., Coleman, M., Vrabel, M., & Bevans, M. (2020). Family caregiver strain and burden: A systematic review of evidence-based interventions when caring for patients with cancer. Clinical Journal of Oncology Nursing, 24(1), 31–50. https://doi.org/10.1188/20.CJON.31-50
- Jansen, L., Dauphin, S., Van den Akker, M., Burghgraeve, T. D., Schoenmakers, B., & Buntinx, F. (2018). Prevalence and predictors of psychosocial problems in informal caregivers of older cancer survivors – a systematic review: Still major gaps in current research. European Journal of Cancer Care, 27(6), e12899. https://doi.org/10.1111/ecc.12899
- Jeyarajah, E. J., Cromwell, W. C., & Otvos, J. D. (2006). Lipoprotein particle analysis by nuclear magnetic resonance spectroscopy. Clinics in Laboratory Medicine, 26(4), 847–870. https://doi.org/10.1016/j.cll.2006.07.006
- Ji, J., Zöller, B., Sundquist, K., & Sundquist, J. (2012). Increased risks of coronary heart disease and stroke among spousal caregivers of cancer patients. Circulation, 125(14), 1742–1747. https://doi.org/10.1161/CIRCULATIONAHA.111.057018
- Kim, Y., Baker, F., Spillers, R. L., & Wellisch, D. K. (2006). Psychological adjustment of cancer caregivers with multiple roles. Psycho-oncology, 15(9), 795–804. https://doi.org/10.1002/pon.1013
- Kim, Y., Carver, C. S., Cannady, R. S., & Shaffer, K. M. (2013). Self-reported medical morbidity among informal caregivers of chronic illness: The case of cancer. Quality of Life Research, 22(6), 1265–1272. https://doi.org/10.1007/s11136-012-0255-y
- Kim, Y., Carver, C. S., Shaffer, K. M., Gansler, T., & Cannady, R. S. (2015). Cancer caregiving predicts physical impairments: Roles of earlier caregiving stress and being a spousal caregiver. Cancer, 121(2), 302–310. https://doi.org/10.1002/cncr.29040
- Kinzer, A. B., Shamburek, R. D., Lightbourne, M., Muniyappa, R., & Brown, R. J. (2019). Advanced lipoprotein analysis shows atherogenic lipid profile that improves after metreleptin in patients with lipodystrophy. Journal of the Endocrine Society, 3(8), 1503–1517. https://doi.org/10.1210/js.2019-00103
- Kontush, A. (2015). HDL particle number and size as predictors of cardiovascular disease. Frontiers in Pharmacology, 6, 218.
- Lee, S., Colditz, G. A., Berkman, L. F., & Kawachi, I. (2003). Caregiving and risk of coronary heart disease in U.S. women: a prospective study. American Journal of Preventive Medicine, 24(2), 113–119. https://doi.org/10.1016/S0749-3797(02)00582-2
- Matyus, S. P., Braun, P. J., Wolak-Dinsmore, J., Jeyarajah, E. J., Shalaurova, I., Xu, Y., Warner, S. M., Clement, T. S., Connelly, M. A., & Fischer, T. J. (2014). NMR measurement of LDL particle using the Vantera® clinical analyzer. Clinical Biochemistry, 47(16–17), 203–210. https://doi.org/10.1016/j.clinbiochem.2014.07.015
- McNair, D. M., Lorr, M., & Droppleman, L. F. (1992). Profile of mood states manual. Educational and Industrial Testing Service.
- Mochari-Greenberger, H., & Mosca, L. (2012). Caregiver burden and nonachievement of health lifestyle behaviors among family caregivers of cardiovascular disease patients. American Journal of Health Promotion, 27(2), 84–89. https://doi.org/10.4278/ajhp.110606-QUAN-241
- Mortensen, J., Dich, N., Lange, T., Ramlau-Hansen, C. H., Head, J., Kivimäki, M., Leineweber, C., & Hulvej Rod, N. (2018). Weekly hours of informal caregiving and paid work, and the risk of cardiovascular disease. European Journal of Public Health, 28(4), 743–747. https://doi.org/10.1093/eurpub/ckx227
- National Alliance for Caregiving [NAC]. (2020). Caregiving in the U.S. https://www.caregiving.org/wp-content/uploads/2021/01/full-report-caregiving-in-the-united-states-01-21.pdf
- National Cancer Institute. (2015). NCI dictionary of cancer terms. http://www.cancer.gov/publications/dictionaries/cancer-terms?cdrid=45618
- National Institutes of Health. (2019). Overview of established risk factors for cardiovascular disease. https://www.uptodate.com/contents/overview-of-established-risk-factors-for-cardiovascular-disease
- Otvos, J. D., Mora, S., Shalaurova, I., Greenland, P., Mackey, R. H., & Goff, D. C. Jr. (2011). Clinical implications of discordance between low-density lipoprotein cholesterol and particle number. Journal of Clinical Lipidology, 5(2), 105–113. https://doi.org/10.1016/j.jacl.2011.02.001
- Park, J., Ross, A., Klagholz, S. D., & Bevans, M. F. (2018). The role of biomarkers in research on caregivers of cancer patients: A scoping review. Biological Research for Nursing, 20(3), 300–311. https://doi.org/10.1177/1099800417740970
- Peng, H.-L., Lorenz, R. A., & Chang, Y.-P. (2019). Factors associated with sleep in family caregivers of individuals with dementia. Perspectives in Psychiatric Care, 55(1), 95–102. https://doi.org/10.1111/ppc.12307
- Ross, A., Shamburek, R., Wehrlen, L., Klagholz, S. D., Yang, L., Stoops, E., Flynn, S. L., Remaley, A. T., Pacak, K., Shelburne, N., & Bevans, M. F. (2017). Cardiometabolic risk factors and health behaviors in family caregivers. PLOS One, 12(5), e0176408. https://doi.org/10.1371/journal.pone.0176408
- Ross, A., Lee, L. J., Wehrlen, L., Cox, R., Yang, L., Perez, A., Bevans, M., Ding, A., & Wallen, G. (2020). Factors that influence health-promoting behaviors in cancer caregivers. Oncology Nursing Forum, 47(6), 692–702. https://doi.org/10.1188/20.ONF.692-702
- Sisk, R. J. (2000). Caregiver burden and health promotion. International Journal of Nursing Studies, 37(1), 37–43. https://doi.org/10.1016/S0020-7489(99)00053-X
- Shaffer, K. M., Kim, Y., Carver, C. S., & Cannady, R. S. (2017). Effects of caregiving status and changes in depressive symptoms on development of physical morbidity among long-term cancer caregivers. Health Psychology, 36(8), 770–778. https://doi.org/10.1037/hea0000528
- Schulz, R., & Eden, J. (2016). Family caregiving roles and impacts. In Committee on Family Caregiving for Older Adults, Board on Health Care Services, Health and Medicine Division, & National Academies of Sciences, Engineering, and Medicine (Eds.), Families Caring for an Aging America (pp. 73-122). National Academies Press.
- Tran, J., Norton, R., Conrad, N., Rahimian, F., Canoy, D., Nazarzadeh, M., & Rahimi, K. (2018). Patterns and temporal trends of comorbidity among adult patients with incident cardiovascular disease in the UK between 2000 and 2014: A population-based cohort study. PLOS Medicine, 15(3), e1002513. https://doi.org/10.1371/journal.pmed.1002513
- Urbina, E. M., McCoy, C. E., Gao, Z., Khoury, P. R., Shah, A. S., Dolan, L. M., & Kimball, T. R. (2017). Lipoprotein particle number and size predict vascular structure and function better than traditional lipids in adolescent and young adults. Journal of Clinical Lipidology, 11(4), 1023–1031. https://doi.org/10.1016/j.jacl.2017.05.011
- Vitaliano, P. P., Zhang, J., & Scanlan, J. M. (2003). Is caregiving hazardous to one's physical health? A meta-analysis. Psychological Bulletin, 129(6), 946–972. https://doi.org/10.1037/0033-2909.129.6.946
- von Känel, R., Ancoli-Israel, S., Dimsdale, J. E., Mills, P. J., Mausbach, B. T., Ziegler, M. G., Patterson, T. L., & Grant, I. (2010a). Sleep and biomarkers of atherosclerosis in elderly Alzheimer caregivers and controls. Gerontology, 56(1), 41–50. https://doi.org/10.1159/000264654
- von Känel, R., Mausbach, B. T., Dimsdale, J. E., Mills, P. J., Patterson, T. L., Ancoli-Israel, S., Ziegler, M. G., Roepke, S. K., Allison, M., & Grant, I. (2010b). Problem behavior of dementia patients predicts low-grade hypercoagulability in spousal caregivers. The Journals of Gerontology, 65(9), 1004–1011.
- Yabroff, K. R., & Kim, Y. (2009). Time costs associated with informal caregiving for cancer survivors. Cancer, 115(S18), 4362–4373. https://doi.org/10.1002/cncr.24588
- Young, H., & Grundy, E. (2008). Longitudinal perspectives on caregiving, employment history and marital status in midlife in England Wales. Health & Social Care in the Community, 16(4), 388–399. https://doi.org/10.1111/j.1365-2524.2007.00750.x