Abstract
The prefrontal cortex (PFC) is one of several brain structures that are sensitive to chronic stress exposure. There have been several studies which have examined the effects on chronic stress, using various protocols such as chronic unpredictable stress and chronic social defeat stress, on the PFC transcriptome. In this report, a bioinformatic meta-analysis of publicly available RNA sequencing datasets (101 samples) from seven chronic stress studies was carried out to identify core PFC transcriptional signatures that underpin behavioral phenotypes including resilience and susceptibility. The results showed 160 differentially expressed genes in chronic stress mice compared to controls with significant enrichment in mechanisms associated with translation and localization of membrane-bound proteins with a putative effect on synaptic plasticity in glutamatergic neurons. Moreover, the meta-analysis revealed no differentially expressed genes in resilient mice but 144 in susceptible mice compared to controls, of which 44 were not identified in the individual studies. Enrichment analysis revealed that susceptibility genes were most affected in oligodendrocytes and linked to mechanisms which mediate biochemical, bidirectional communication between this cell-type and myelinated axons. These results provide new avenues for further research into the neurobiology and treatment of chronic stress-induced disorders.
Introduction
The prefrontal cortex (PFC) is one of several limbic structures which directs the autonomic and neuroendocrine response to stress exposure in addition to its array of cognitive and behavioral functions (Arnsten, Citation2009; Ulrich-Lai & Herman, Citation2009). Chronic stress exposure has several reported effects on the PFC structure, morphology, and connectivity with other structures (for review, see McKlveen et al., Citation2019; Negrón-Oyarzo et al., Citation2016); this includes a reduction in the dendritic complexity of pyramidal neurons (Goldwater et al., Citation2009), alterations in synaptic architecture (Shepard & Coutellier, Citation2018), an imbalance in (glutamatergic) excitatory vs (interneuron) inhibitory neurotransmission in favor of the latter (Shepard & Coutellier, Citation2018), and a decoupling of connections between the PFC and its output structures such as the amygdala (Jovanovic et al., Citation2011). These findings have led to the theory that chronic stress exposure takes the PFC “offline,” removing the “top-down” control and influence over functions, and other brain structures that it would otherwise have which are critical for return to homeostasis (Datta & Arnsten, Citation2019).
Complementing these findings, changes in the PFC transcriptome detected using RNA-sequencing strategies have revealed new insights and mechanisms which potentially underpin many of the above stress-induced phenotypic changes (Bagot et al., Citation2016; Bondar et al., Citation2018; Cheng et al., Citation2018; Labonté et al., Citation2017; Laine et al., Citation2018; Wang et al., Citation2019; Weger et al., Citation2020). One of the benefits of these RNA-studies is that authors have deposited the sequencing datasets into the NCBI Gene Expression Omnibus (GEO) database (Barrett et al., Citation2013). This is free to access and researchers can download and re-analyze the datasets to carry out meta-analyses, accounting for the variability within studies and study-specific effects (Rau et al., Citation2014; Rung & Brazma, Citation2013).
Accordingly, in this study the datasets from the above studies were downloaded and analyzed using the same bioinformatic pipeline to identify PFC transcriptional signatures with high signal-to-noise ratio which underpin the behavioral, physiological, and endocrinological responses to chronic stress, including resilience and susceptibility. Moreover, functional enrichment analyses were carried out to highlight potential mechanisms and cell-types implicated in the etiology of stress-induced psychopathologies.
Methods
The NCBI Geo Database was queried with the search term “chronic AND mouse AND stress” with the additional filter, “Expression profiling by high throughput sequencing” (Barrett et al., Citation2013). Projects were selected for analysis which met the following criteria adapted from a similar recent comparative analysis of studies using both acute and chronic stress protocols (Flati et al., Citation2020): (i) had an accompanying publication with metadata which could be referenced, (ii) at least six samples were sequenced with three biological replicates per group, (iii) exclude studies/samples in which mice received injections or were transgenics, (iv) exclude studies which focus on early life stress, (v) whole transcriptome sequencing. This resulted in data from 101 samples from seven studies () which were imported into a Galaxy environment.
Table 1. List and details of studies used in the meta-analysis.
Reads were aligned to the reference Mus musculus genome (GRCm38/mm10) using HISAT2, annotated (Gencode v.M25) and counted to gene exons using featureCounts. Default settings were used for all analyses. Biostatistics and visualization were run in R (version 3.6.3) with the Rstudio GUI (version 1.2.5033). Filtering was done to include only protein-coding genes with at least 10 counts in half the samples of each study.
Differential gene expression of individual datasets was analyzed using the DESeq2 package with default parameters (Love et al., Citation2014). For the meta-analysis of datasets, two approaches were used. The first was by combining sample data from all studies. There were several lab-specific experimental variables for each study () which ranged from housing type, age of the mouse to the sequencing strategy. As such a new variable known as “lab,” was used and DESeq2 analysis was adjusted to control for the “lab effect” (design=∼lab + group). The second approach was using the metaRNASeq package to perform inverse normal p value combination tests as previously described (Fernández-Beltrán et al., Citation2021; Rau et al., Citation2014; Ziff et al., Citation2022).
Overlap analyses between datasets were carried out and visualized using the UpSetR package (Conway et al., Citation2017).
Enrichment analysis was carried out using EnrichR on significant differentially expressed genes (Chen et al., Citation2013) using the following libraries: GO_Biological Process_2021, GO_Molecular Function_2021, GO_Cellular Component_2021, KEGG_2019_Mouse, DSigDB, and Allen_Brain_Atlas_10x_scRNA_2021. Cluster identification and cell-type information were obtained from the Allen Institute transcriptomic atlas (https://taxonomy.shinyapps.io/ctx_hip_browser/). GSEA analysis was performed as previous described (Mootha et al., Citation2003; Subramanian et al., Citation2005) using the ontology gene set database (ms_gsea_c5 = msigdb(species=“Mus musculus”, category=“C5”).
Identification of predicted upstream regulators of differentially expressed genes was done using QIAGEN IPA (QIAGEN Inc., https://digitalinsights.qiagen.com/IPA).
The top five significant results of each analysis have been presented in bar plots. Unless otherwise stated, significance for all analyses was set at FDR <0.05.
Results
Because different sequencing platforms were used for the analysis in each of the studies, there was a need first correlate measures of average gene expression (normalized counts) between datasets. The higher the correlation, the greater the chance of finding similarities between datasets. Across all seven studies, there were 8767 genes that were commonly identified and there were significant Pearson correlations between all studies suggesting expression profiles are largely comparable ().
Figure 1. Analysis of RNA-seq datasets from studies which have used chronic stress protocols in male mice to examine the impact on the PFC transcriptome. (A) Pearson correlation plot of average gene expression across all the seven studies that were the included in this study. (B) Heatmaps indicating degree of fold-change and the number of differentially expressed genes in each study and in the combined analysis (bottom row) for stress vs control mice. (C) UpSet plot of overlaps between the various datasets that were analyzed. The vertical lines which connect black circles indicate intersecting sets of genes between studies. The y-axis of the histogram indicates the number of intersecting genes and the bar plot on the right-hand side indicates the number of genes in each set. (D–G) EnrichR analysis of differentially expressed genes in the combined analysis for stress vs control mice. (H) Predicted upstream regulators of the differentially expressed genes in the combined analysis as determined using IPA.
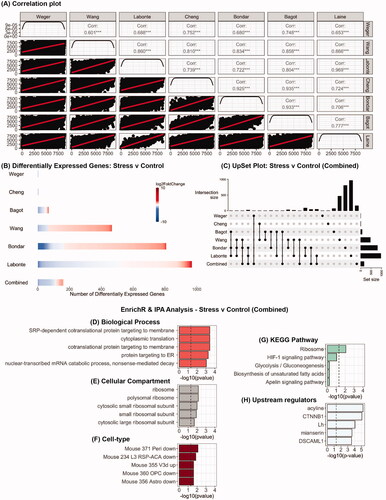
Stress vs control
The number of genes that were differentially expressed in stressed mice compared to controls in each of the seven studies varied from none to 969 (, Supplementary Tables 1(A–F)) and this is reflective of the impact of methodological differences on differential gene expression in the PFC. In combining all the datasets there were 160 genes that were differentially expressed (Supplementary Table 1G). Additionally, a total of 259 differentially expressed genes were found to overlap across six of the seven studies and the combined analysis (). MetaRNAseq analysis yielded no significant findings. To avoid bias for any specific study, EnrichR analysis was carried out on the combined analysis (160 genes) and yielded significant findings across multiple libraries. Notably, there was significant enrichment in functional mechanisms in stressed mice associated with transport of proteins to target membranes () and ribosomal function (). There was also significant enrichment for genes that were highly expressed in specific glutamatergic neurons (Mouse 355 V3d up). IPA analysis identified several putative upstream regulators of differentially expressed genes in stressed mice including luteinizing hormone (Lh), and CTNNB1 also known as β-catenin (). GSEA analysis revealed no significant findings.
Resilient/susceptible vs control
There were 216 differentially expressed genes in resilient mice but only from one study (Bagot et al., Citation2016). In contrast, there were a 388 differentially expressed genes in susceptible mice across both studies (, Supplementary Tables 2(A–C)). Analysis of combined datasets revealed no differentially expressed genes in resilient mice compared to controls but 92 differentially expressed genes in susceptible mice (, Supplementary Table 2D). MetaRNAseq analysis of all the datasets yielded 144 differentially expressed genes in susceptible mice of which 44 were not identified in the individual studies alone (Supplementary Tables 2(E,F)). Additionally, a total of 310 differentially expressed genes were found to overlap across the two studies, the combined and metaRNAseq analyses in the susceptible mice (). In particular, there were 76 differentially expressed genes that were common to the combined and metaRNAseq analyses (Supplementary Table 2G). As above, to avoid bias for any specific study, EnrichR analysis was carried out on this set of 76 genes revealed significant effects on channel activity (), gap junction (), and oligodendrocytes were the most affected cell-type (). IPA analysis of this gene set revealed the transcription factor Sox2 as one of the upstream regulators. GSEA analysis revealed no significant enrichment.
Figure 2. Analysis of RNA-seq datasets from studies which have used chronic social defeat stress to examine the impact on the PFC transcriptome in male mice classified as resilient or susceptible. (A) Heatmaps indicating degree of fold-change and the number of differentially expressed genes in each study and in the combined analysis (bottom row) for susceptible vs control and resilient vs control mice. (B) UpSet plot of overlaps between the various datasets that were analyzed. (C–E) EnrichR analysis for differentially expressed genes that were common in the combined analysis and metaRNAseq analysis for susceptible vs control mice. (F) Predicted upstream regulators of the differentially expressed genes that were common in the combined analysis and metaRNAseq analysis for susceptible vs control mice.
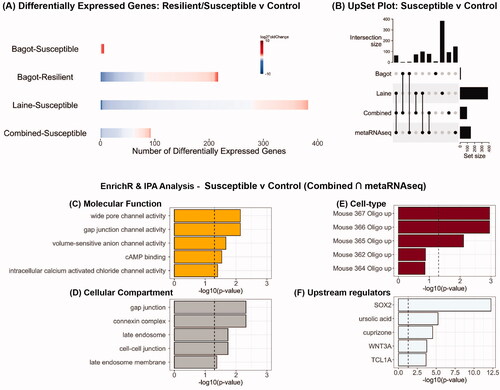
Discussion
The PFC is one of the several brain structures sensitive to stress exposure which can, if chronic or uncontrollable, lead to it being taken “off-line,” thus compromising its important top-down role in controlling cognition, mood, and behavior (Datta & Arnsten, Citation2019; Woo et al., Citation2021). Here, I have presented the results of a bioinformatic meta-analysis of several studies that have examined the impact of chronic stress on the PFC to identify common transcriptional signatures which underpin stress-induced phenotypes.
Using the same bioinformatic pipeline on each dataset revealed wide ranging effects on chronic stress on the PFC transcriptome. However, analysis of the combined datasets yielded 160 differentially expressed genes which suggests their general involvement in the response to chronic stress, regardless of paradigm specifics. Enrichment analysis of these genes revealed significant involvement in several processes including signal recognition particle (SRP)-co-translational targeting of proteins to membranes as well as ribosomal function. The SRP-pathway is initiated by the binding of SRP to a specific amino acid sequence on a nascent protein emerging from a translating ribosome. Translation is briefly halted while this complex is delivered to the plasma membrane via a cognate SRP receptor where it unloads the cargo. Translation resumes, SRP and SR dissociate and move on to other targets (Egea et al., Citation2005; Nagai et al., Citation2003; Saraogi & Shan, Citation2011). Combined with the findings that specific glutamatergic neurons are more affected than other cell types provide supporting evidence that chronic stress in the PFC disrupts excitatory neurotransmission and synaptic plasticity, going “off-line,” in part due to the dysregulated expression of membrane-bound proteins (Arnsten, Citation2009; Moghaddam, Citation2002).
Selected top predicted upstream regulators for this set of differentially expressed genes in chronic stress mice included Lh and β-catenin. Lh is released in a pulsatile manner by gonadotrophs in the anterior pituitary downstream of an activated hypothalamic–pituitary–gonadal axis. This is a neuroendocrine network which functions to control reproductive function but is also under the control of the hypothalamic–pituitary–adrenal axis. Indeed, evidence from cross-species experiments have reported an inverse relationship between stress-induced increase in corticosterone levels and Lh levels (Son et al., Citation2022). Notably, work in rats has shown that stress-induced effects on Lh levels are sensitive to glucocorticoid receptor antagonists (Briski et al., Citation1995), antibodies to corticotropin releasing factor (Nemoto et al., Citation2010), serotonin synthesis inhibitors, and serotonin receptor antagonists (Armario et al., Citation1993). Lh binds to Lh-receptors and activates a range of intracellular signaling cascades which may culminate in transcriptional responses, though this evidence mainly comes from work using non-neural tissue (Carvalho et al., Citation2003; Davis, Citation1994; Sasson et al., Citation2004). β-catenin is a component of the Wnt-signaling pathway which when activated facilitates translocation of β-catenin into the nucleus where it mediates transcription of target genes. Postmortem studies have reported dysregulation in PFC β-catenin expression and this has also been observed in animal models of aspects of depression (Teo et al., Citation2018). It is worth noting here that in the nucleus accumbens, β-catenin is implicated in stress resilience (Dias et al., Citation2014). β-catenin in the PFC has also been implicated in the mechanism of action of antidepressants (Chen et al., Citation2012; Mohamed et al., Citation2020).
Overall, susceptibility to chronic stress was associated with greater degree of transcriptional change than resilience. This transcriptional change had significant effects at the bidirectional, biochemical communication interface between oligodendrocytes and the axons which they myelinate as indicated by the enrichment analysis. Oligodendrocytes express glutamatergic and GABAergic receptors which influence their ability to facilitate axonal conduction and they, in turn, are also susceptible to depolarization by passing action potentials in the axons (Edgar & Sibille, Citation2012; Mazuir et al., Citation2021). Recent studies have reported the differential impact of chronic stress on aspects of PFC myelination in susceptible and resilient mice, with the former group being more affected (Antontseva et al., Citation2020; Bonnefil et al., Citation2019; Laine et al., Citation2018). The findings reported herein suggest that the susceptibility phenotype may be, in part, due a breakdown in oligodendrocyte-axonal information transfer in the PFC and consequently lead to its disengagement and decoupling from the neural networks over which it exerts control (Edgar & Sibille, Citation2012).
Sox2 was identified as a predicted upstream regulator of gene expression changes in susceptible mice. It is a conserved transcription factor with a significant role in neurodevelopment but also expressed in adulthood in a region- and cell-type specific manner; in astrocytes, deletion of the Sox2 gene induces resistance to the effects of traumatic brain injury in mice (Chen et al., Citation2019) while deletion in GABAergic neurons in the suprachiasmatic nucleus, the brain’s “internal clock,” results in increased anxiety-like behaviors and sex-specific effects on motivation (Boehler et al., Citation2021). Its functional role in the PFC and the stress-response remains undetermined. An important caveat with this discussion is that susceptibility was defined as reduced social interaction with a novel conspecific. But this could also be a manifestation of an adaptive, high-vigilance response developed as a protective strategy over the course of the social defeat protocol (Meshalkina & Kalueff, Citation2016). Examining the PFC transcriptional response in stressed mice with differing reward learning and processing abilities is one approach to be considered in future work to rule out this alternative explanation and more accurately discriminate between phenotypes (Gururajan et al., Citation2019).
The deposition of transcriptomics into public database provides opportunities for researchers to make new discoveries and generate testable hypotheses at low-cost through integrative meta-analyses using bioinformatic tools. There are pros and cons to using this approach (Feichtinger et al., Citation2012; Ramasamy et al., Citation2008). Pros include (i) the ability to increase statistical power and obtain more precise estimates of gene expression by increasing the sample size, (ii) the ability to reveal a set of generalizable, reproducible, core-over-represented genes – a so-called meta-signature – in analogous studies, (iii) and reducing the probability of false negative results. Cons are that (i) studies with larger sample sizes may have a larger influence on the meta-analysis outcome than a smaller ones, (ii) the “overlooking” of any bias in the publications from which data are extracted, and (iii) the inherent assumption that different experimental paradigms make similar demands on the organism. Other limitations of this bioinformatic study are the (i) absence of sufficient RNA-sequencing data from chronic stress studies in females which met the exclusion criteria (Deonaraine et al., Citation2020; Girgenti et al., Citation2019; Issler et al., Citation2020) and that (ii) all input studies using bulk RNA-sequencing techniques which does not reflect the known heterogeneity in PFC cell-type specific gene expression responses in stress-related disorders (Nagy et al., Citation2020). The inclusion of both male and female animals in the same study and cell-type analyses in the future should address this knowledge deficit to provide high-resolution insights into sex-specific PFC transcriptional signatures. With this proviso, the results lead to the hypothesis that chronic stress disrupts mechanisms that regulate synthesis and localization of membrane-bound proteins involved in neurotransmission and synaptic plasticity in the PFC. Moreover, the findings also indicate the specific sensitivity of oligodendrocytes in the PFC to chronic stress that may lead to stress-susceptible phenotypes in mice. Future validation will be required to rigorously test these hypotheses before translation into better treatments for stress-induced psychopathologies.
Supplemental Material
Download MS Excel (152.9 KB)Supplemental Material
Download MS Excel (372.8 KB)Acknowledgements
AG wishes to thank Dr Kandarp Joshi and the Sydney Informatics Hub, a Core Research Facility of the University of Sydney, for their assistance with data analysis and Prof. Avi Ma'ayan with interpretation of the EnrichR brain cell-type analysis.
Disclosure statement
No potential conflict of interest was reported by the author.
Data availability statement
All data generated or analyzed during this study are freely available on the NCBI GEO website. Accession details have been included in . Scripts for analysis of data are available on request.
Additional information
Funding
Notes on contributors
Anand Gururajan
Dr Anand Gururajan is a Research Fellow at the Brain & Mind Centre at the University of Sydney. His research is focused on understanding the impact of stress on the brain by using animal models and high-resolution, high-throughput sequencing techniques, with a view to identifying new drug targets for the treatment of stress disorders such as major depression and PTSD.
References
- Antontseva, E., Bondar, N., Reshetnikov, V., & Merkulova, T. (2020). The effects of chronic stress on brain myelination in humans and in various rodent models. Neuroscience, 441, 226–238. https://doi.org/10.1016/j.neuroscience.2020.06.013
- Armario, A., Marti, O., Gavalda, A., & Lopez-Calderon, A. (1993). Evidence for the involvement of serotonin in acute stress-induced release of luteinizing hormone in the male rat. Brain Research Bulletin, 31(1–2), 29–31. https://doi.org/10.1016/0361-9230(93)90006-W
- Arnsten, A. F. T. (2009). Stress signalling pathways that impair prefrontal cortex structure and function. Nature Reviews Neuroscience, 10(6), 410–422. https://doi.org/10.1038/nrn2648
- Bagot, R. C., Cates, H. M., Purushothaman, I., Lorsch, Z. S., Walker, D. M., Wang, J., Huang, X., Schlüter, O. M., Maze, I., Peña, C. J., Heller, E. A., Issler, O., Wang, M., Song, W. M., Stein, J. L., Liu, X., Doyle, M. A., Scobie, K. N., Sun, H. S., … Nestler, E. J. (2016). Circuit-wide transcriptional profiling reveals brain region-specific gene networks regulating depression susceptibility. Neuron, 90(5), 969–983. https://doi.org/10.1016/j.neuron.2016.04.015
- Barrett, T., Wilhite, S. E., Ledoux, P., Evangelista, C., Kim, I. F., Tomashevsky, M., Marshall, K. A., Phillippy, K. H., Sherman, P. M., Holko, M., Yefanov, A., Lee, H., Zhang, N., Robertson, C. L., Serova, N., Davis, S., & Soboleva, A. (2013). NCBI GEO: Archive for functional genomics data sets—update. Nucleic Acids Research, 41(Database issue), D991–D995. https://doi.org/10.1093/nar/gks1193
- Boehler, N. A., Fung, S. W., Hegazi, S., Cheng, A. H., & Cheng, H.-Y M. (2021). Sox2 ablation in the suprachiasmatic nucleus perturbs anxiety- and depressive-like behaviors. Neurology International, 13(4), 541–554. https://doi.org/10.3390/neurolint13040054
- Bondar, N., Bryzgalov, L., Ershov, N., Gusev, F., Reshetnikov, V., Avgustinovich, D., Tenditnik, M., Rogaev, E., & Merkulova, T. (2018). Molecular adaptations to social defeat stress and induced depression in mice. Molecular Neurobiology, 55(4), 3394–3407. https://doi.org/10.1007/s12035-017-0586-3
- Bonnefil, V., Dietz, K., Amatruda, M., Wentling, M., Aubry, A. V., Dupree, J. L., Temple, G., Park, H. J., Burghardt, N. S., Casaccia, P., & Liu, J. (2019). Region-specific myelin differences define behavioral consequences of chronic social defeat stress in mice. eLife, 8. https://doi.org/10.7554/eLife.40855
- Briski, K. P., Vogel, K. L., & McIntyre, A. R. (1995). The antiglucocorticoid, RU486, attenuates stress-induced decreases in plasma-luteinizing hormone concentrations in male rats. Neuroendocrinology, 61(6), 638–645. https://doi.org/10.1159/000126890
- Carvalho, C. R., Carvalheira, J. B., Lima, M. H., Zimmerman, S. F., Caperuto, L. C., Amanso, A., Gasparetti, A. L., Meneghetti, V., Zimmerman, L. F., Velloso, L. A., & Saad, M. J. (2003). Novel signal transduction pathway for luteinizing hormone and its interaction with insulin: Activation of Janus kinase/signal transducer and activator of transcription and phosphoinositol 3-kinase/Akt pathways. Endocrinology, 144(2), 638–647. https://doi.org/10.1210/en.2002-220706
- Chen, C., Zhong, X., Smith, D. K., Tai, W., Yang, J., Zou, Y., Wang, L.-L., Sun, J., Qin, S., & Zhang, C.-L. (2019). Astrocyte-specific deletion of Sox2 promotes functional recovery after traumatic brain injury. Cerebral Cortex, 29(1), 54–69. https://doi.org/10.1093/cercor/bhx303
- Chen, E. Y., Tan, C. M., Kou, Y., Duan, Q., Wang, Z., Meirelles, G. V., Clark, N. R., & Ma’ayan, A. (2013). Enrichr: Interactive and collaborative HTML5 gene list enrichment analysis tool. BMC Bioinformatics, 14(1), 128. https://doi.org/10.1186/1471-2105-14-128
- Chen, Y. C., Tan, Q. R., Dang, W., Wang, H. N., Zhang, R. B., Li, Z. Y., Lin, H., & Liu, R. (2012). The effect of citalopram on chronic stress-induced depressive-like behavior in rats through GSK3beta/beta-catenin activation in the medial prefrontal cortex. Brain Research Bulletin, 88(4), 338–344. https://doi.org/10.1016/j.brainresbull.2012.03.004
- Cheng, Y., Sun, M., Chen, L., Li, Y., Lin, L., Yao, B., Li, Z., Wang, Z., Chen, J., Miao, Z., Xin, N., Huang, L., Allen, E. G., Wu, H., Xu, X., & Jin, P. (2018). Ten-eleven translocation proteins modulate the response to environmental stress in mice. Cell Reports, 25(11), 3194–3203.e4. https://doi.org/10.1016/j.celrep.2018.11.061
- Conway, J. R., Lex, A., & Gehlenborg, N. (2017). UpSetR: An R package for the visualization of intersecting sets and their properties. Bioinformatics, 33(18), 2938–2940. https://doi.org/10.1093/bioinformatics/btx364
- Datta, D., & Arnsten, A. F. T. (2019). Loss of prefrontal cortical higher cognition with uncontrollable stress: Molecular mechanisms, changes with age, and relevance to treatment. Brain Sciences, 9(5), 113. https://doi.org/10.3390/brainsci9050113
- Davis, J. S. (1994). Mechanisms of hormone action: Luteinizing hormone receptors and second-messenger pathways. Current Opinion in Obstetrics and Gynecology, 6(3), 254–261. https://doi.org/10.1097/00001703-199406000-00010
- Deonaraine, K. K., Wang, Q., Cheng, H., Chan, K. L., Lin, H. Y., Liu, K., Parise, L. F., Cathomas, F., Leclair, K. B., Flanigan, M. E., Li, L., Aleyasin, H., Guevara, C., Hao, K., Zhang, B., Russo, S. J., & Wang, J. (2020). Sex-specific peripheral and central responses to stress-induced depression and treatment in a mouse model. Journal of Neuroscience Research, 98(12), 2541–2553. https://doi.org/10.1002/jnr.24724
- Dias, C., Feng, J., Sun, H., Shao, N. Y., Mazei-Robison, M. S., Damez-Werno, D., Scobie, K., Bagot, R., LaBonté, B., Ribeiro, E., Liu, X., Kennedy, P., Vialou, V., Ferguson, D., Peña, C., Calipari, E. S., Koo, J. W., Mouzon, E., Ghose, S., … Nestler, E. J. (2014). β-catenin mediates stress resilience through Dicer1/microRNA regulation. Nature, 516(7529), 51–55. https://doi.org/10.1038/nature13976
- Edgar, N., & Sibille, E. (2012). A putative functional role for oligodendrocytes in mood regulation. Translational Psychiatry, 2(5), e109. https://doi.org/10.1038/tp.2012.34
- Egea, P. F., Stroud, R. M., & Walter, P. (2005). Targeting proteins to membranes: Structure of the signal recognition particle. Current Opinion in Structural Biology, 15(2), 213–220. https://doi.org/10.1016/j.sbi.2005.03.007
- Feichtinger, J., Thallinger, G. G., McFarlane, R. J., & Larcombe, L. D. (2012). Microarray meta-analysis: From data to expression to biological relationships. In Z. Trajanoski (Ed.), Computational medicine: Tools and challenges (pp. 59–77). Springer.
- Fernández-Beltrán, L. C., Godoy-Corchuelo, J. M., Losa-Fontangordo, M., Williams, D., Matias-Guiu, J., & Corrochano, S. (2021). A transcriptomic meta-analysis shows lipid metabolism dysregulation as an early pathological mechanism in the spinal cord of SOD1 mice. International Journal of Molecular Sciences, 22(17), 9553. https://doi.org/10.3390/ijms22179553
- Flati, T., Gioiosa, S., Chillemi, G., Mele, A., Oliverio, A., Mannironi, C., Rinaldi, A., & Castrignanò, T. (2020). A gene expression atlas for different kinds of stress in the mouse brain. Scientific Data, 7(1), 437. https://doi.org/10.1038/s41597-020-00772-z
- Girgenti, M. J., Wohleb, E. S., Mehta, S., Ghosal, S., Fogaca, M. V., & Duman, R. S. (2019). Prefrontal cortex interneurons display dynamic sex-specific stress-induced transcriptomes. Translational Psychiatry, 9(1), 292. https://doi.org/10.1038/s41398-019-0642-z
- Goldwater, D. S., Pavlides, C., Hunter, R. G., Bloss, E. B., Hof, P. R., McEwen, B. S., & Morrison, J. H. (2009). Structural and functional alterations to rat medial prefrontal cortex following chronic restraint stress and recovery. Neuroscience, 164(2), 798–808. https://doi.org/10.1016/j.neuroscience.2009.08.053
- Gururajan, A., Reif, A., Cryan, J. F., & Slattery, D. A. (2019). The future of rodent models in depression research. Nature Reviews Neuroscience, 20(11), 686–701. https://doi.org/10.1038/s41583-019-0221-6
- Issler, O., van der Zee, Y. Y., Ramakrishnan, A., Wang, J., Tan, C., Loh, Y. E., Purushothaman, I., Walker, D. M., Lorsch, Z. S., Hamilton, P. J., Peña, C. J., Flaherty, E., Hartley, B. J., Torres-Berrío, A., Parise, E. M., Kronman, H., Duffy, J. E., Estill, M. S., Calipari, E. S., … Nestler, E. J. (2020). Sex-specific role for the long non-coding RNA LINC00473 in depression. Neuron, 106(6), 912–926.e5. https://doi.org/10.1016/j.neuron.2020.03.023
- Jovanovic, H., Perski, A., Berglund, H., & Savic, I. (2011). Chronic stress is linked to 5-HT1A receptor changes and functional disintegration of the limbic networks. NeuroImage, 55(3), 1178–1188. https://doi.org/10.1016/j.neuroimage.2010.12.060
- Labonté, B., Engmann, O., Purushothaman, I., Menard, C., Wang, J., Tan, C., Scarpa, J. R., Moy, G., Loh, Y.-H E., Cahill, M., Lorsch, Z. S., Hamilton, P. J., Calipari, E. S., Hodes, G. E., Issler, O., Kronman, H., Pfau, M., Obradovic, A. L. J., Dong, Y., … Nestler, E. J. (2017). Sex-specific transcriptional signatures in human depression. Nature Medicine, 23(9), 1102–1111. https://doi.org/10.1038/nm.4386
- Laine, M. A., Trontti, K., Misiewicz, Z., Sokolowska, E., Kulesskaya, N., Heikkinen, A., Saarnio, S., Balcells, I., Ameslon, P., Greco, D., Mattila, P., Ellonen, P., Paulin, L., Auvinen, P., Jokitalo, E., & Hovatta, I. (2018). Genetic control of myelin plasticity after chronic psychosocial stress. eNeuro, 5(4), ENEURO.0166-18.2018. https://doi.org/10.1523/ENEURO.0166-18.2018
- Love, M. I., Huber, W., & Anders, S. (2014). Moderated estimation of fold change and dispersion for RNA-seq data with DESeq2. Genome Biology, 15(12), 550. https://doi.org/10.1186/s13059-014-0550-8
- Mazuir, E., Fricker, D., & Sol-Foulon, N. (2021). Neuron-oligodendrocyte communication in myelination of cortical GABAergic cells. Life, 11(3), 216. https://doi.org/10.3390/life11030216
- McKlveen, J. M., Moloney, R. D., Scheimann, J. R., Myers, B., & Herman, J. P. (2019). Braking the prefrontal cortex: The role of glucocorticoids and interneurons in stress adaptation and pathology. Biological Psychiatry, 86(9), 669–681. https://doi.org/10.1016/j.biopsych.2019.04.032
- Meshalkina, D. A., & Kalueff, A. V. (2016). Commentary: Ethological evaluation of the effects of social defeat stress in mice: Beyond the social interaction ratio. Frontiers in Behavioral Neuroscience, 10(155).
- Moghaddam, B. (2002). Stress activation of glutamate neurotransmission in the prefrontal cortex: Implications for dopamine-associated psychiatric disorders. Biological Psychiatry, 51(10), 775–787. https://doi.org/10.1016/S0006-3223(01)01362-2
- Mohamed, A. M., Habib, M. Z., Ebeid, M. A., Abdelraouf, S. M., Faramawy, Y. E., Aboul-Fotouh, S., & Magdy, Y. (2020). Amisulpride alleviates chronic mild stress-induced cognitive deficits: Role of prefrontal cortex microglia and Wnt/beta-catenin pathway. European Journal of Pharmacology, 885, 173411. https://doi.org/10.1016/j.ejphar.2020.173411
- Mootha, V. K., Lindgren, C. M., Eriksson, K.-F., Subramanian, A., Sihag, S., Lehar, J., Puigserver, P., Carlsson, E., Ridderstråle, M., Laurila, E., Houstis, N., Daly, M. J., Patterson, N., Mesirov, J. P., Golub, T. R., Tamayo, P., Spiegelman, B., Lander, E. S., Hirschhorn, J. N., Altshuler, D., & Groop, L. C. (2003). PGC-1α-responsive genes involved in oxidative phosphorylation are coordinately downregulated in human diabetes. Nature Genetics, 34(3), 267–273. https://doi.org/10.1038/ng1180
- Nagai, K., Oubridge, C., Kuglstatter, A., Menichelli, E., Isel, C., & Jovine, L. (2003). Structure, function and evolution of the signal recognition particle. EMBO Journal, 22(14), 3479–3485. https://doi.org/10.1093/emboj/cdg337
- Nagy, C., Maitra, M., Tanti, A., Suderman, M., Théroux, J. F., Davoli, M. A., Perlman, K., Yerko, V., Wang, Y. C., Tripathy, S. J., Pavlidis, P., Mechawar, N., Ragoussis, J., & Turecki, G. (2020). Single-nucleus transcriptomics of the prefrontal cortex in major depressive disorder implicates oligodendrocyte precursor cells and excitatory neurons. Nature Neuroscience, 23(6), 771–781. https://doi.org/10.1038/s41593-020-0621-y
- Negrón-Oyarzo, I., Aboitiz, F., & Fuentealba, P. (2016). Impaired functional connectivity in the prefrontal cortex: A mechanism for chronic stress-induced neuropsychiatric disorders. Neural Plasticity, 2016, 7539065.
- Nemoto, T., Iwasaki-Sekino, A., Yamauchi, N., & Shibasaki, T. (2010). Role of urocortin 2 secreted by the pituitary in the stress-induced suppression of luteinizing hormone secretion in rats. American Journal of Physiology, Endocrinology and Metabolism, 299(4), E567–E575. https://doi.org/10.1152/ajpendo.00163.2010
- Ramasamy, A., Mondry, A., Holmes, C. C., & Altman, D. G. (2008). Key issues in conducting a meta-analysis of gene expression microarray datasets. PLoS Medicine, 5(9), e184. https://doi.org/10.1371/journal.pmed.0050184
- Rau, A., Marot, G., & Jaffrézic, F. (2014). Differential meta-analysis of RNA-seq data from multiple studies. BMC Bioinformatics, 15(1), 91. https://doi.org/10.1186/1471-2105-15-91
- Rung, J., & Brazma, A. (2013). Reuse of public genome-wide gene expression data. Nature Reviews Genetics, 14(2), 89–99. https://doi.org/10.1038/nrg3394
- Saraogi, I., & Shan, S-o (2011). Molecular mechanism of co-translational protein targeting by the signal recognition particle. Traffic, 12(5), 535–542. https://doi.org/10.1111/j.1600-0854.2011.01171.x
- Sasson, R., Rimon, E., Dantes, A., Cohen, T., Shinder, V., Land‐Bracha, A., & Amsterdam, A. (2004). Gonadotrophin‐induced gene regulation in human granulosa cells obtained from IVF patients. Modulation of steroidogenic genes, cytoskeletal genes and genes coding for apoptotic signalling and protein kinases. Molecular Human Reproduction, 10(5), 299–311. https://doi.org/10.1093/molehr/gah041
- Shepard, R., & Coutellier, L. (2018). Changes in the prefrontal glutamatergic and parvalbumin systems of mice exposed to unpredictable chronic stress. Molecular Neurobiology, 55(3), 2591–2602. https://doi.org/10.1007/s12035-017-0528-0
- Son, Y. L., Ubuka, T., & Tsutsui, K. (2022). Regulation of stress response on the hypothalamic-pituitary-gonadal axis via gonadotropin-inhibitory hormone. Frontiers in Neuroendocrinology, 64, 100953. https://doi.org/10.1016/j.yfrne.2021.100953
- Subramanian, A., Tamayo, P., Mootha, V. K., Mukherjee, S., Ebert, B. L., Gillette, M. A., Paulovich, A., Pomeroy, S. L., Golub, T. R., Lander, E. S., & Mesirov, J. P. (2005). Gene set enrichment analysis: A knowledge-based approach for interpreting genome-wide expression profiles. Proceedings of the National Academy of Sciences of the United States of America, 102(43), 15545–15550. https://doi.org/10.1073/pnas.0506580102
- Teo, C. H., Soga, T., & Parhar, I. S. (2018). Brain beta-catenin signalling during stress and depression. Neuro-Signals, 26(1), 31–42. https://doi.org/10.1159/000487764
- Ulrich-Lai, Y. M., & Herman, J. P. (2009). Neural regulation of endocrine and autonomic stress responses. Nature Reviews Neuroscience, 10(6), 397–409. https://doi.org/10.1038/nrn2647
- Wang, X., Ma, S., Mao, M., Li, C., Shen, X., Xu, S., & Yang, J. (2019). RNA-sequencing and bioinformatics analysis of long noncoding RNAs and mRNAs in the prefrontal cortex of mice following repeated social defeat stress. BioMed Research International, 2019, 7505260.
- Weger, M., Alpern, D., Cherix, A., Ghosal, S., Grosse, J., Russeil, J., Gruetter, R., de Kloet, E. R., Deplancke, B., & Sandi, C. (2020). Mitochondrial gene signature in the prefrontal cortex for differential susceptibility to chronic stress. Scientific Reports, 10(1), 18308. https://doi.org/10.1038/s41598-020-75326-9
- Woo, E., Sansing, L. H., Arnsten, A. F. T., & Datta, D. (2021). Chronic stress weakens connectivity in the prefrontal cortex: Architectural and molecular changes. Chronic Stress, 5, 24705470211029254. https://doi.org/10.1177/24705470211029254
- Ziff, O. J., Clarke, B. E., Taha, D. M., Crerar, H., Luscombe, N. M., & Patani, R. (2022). Meta-analysis of human and mouse ALS astrocytes reveals multi-omic signatures of inflammatory reactive states. Genome Research, 32(1), 71–84. https://doi.org/10.1101/gr.275939.121