Abstract
Maternal prenatal distress (PD), frequently defined as in utero prenatal stress exposure (PSE) to the developing fetus, influences the developing brain and numerous associations between PSE and brain structure have been described both in neonates and in older children. Previous studies addressing PSE-linked alterations in neonates’ brain activity have focused on connectivity analyses from predefined seed regions, but the effects of PSE at the level of distributed functional networks remains unclear. In this study, we investigated the impact of prenatal distress on the spatial and temporal properties of functional networks detected in functional MRI data from 20 naturally sleeping, term-born (age 25.85 ± 7.72 days, 11 males), healthy neonates. First, we performed group level independent component analysis (GICA) to evaluate an association between PD and the identified functional networks. Second, we searched for an association with PD at the level of the stability of functional networks over time using leading eigenvector dynamics analysis (LEiDA). No statistically significant associations were detected at the spatial level for the GICA-derived networks. However, at the dynamic level, LEiDA revealed that maternal PD negatively associated with the stability of a frontoparietal network. These results imply that maternal PD may influence the stability of frontoparietal connections in neonatal brain network dynamics and adds to the cumulating evidence that frontal areas are especially sensitive to PSE. We advocate for early preventive intervention strategies regarding pregnant mothers. Nevertheless, future research venues are required to assess optimal intervention timing and methods for maximum benefit.
Introduction
Maternal prenatal distress (PD) is common as ca. 30% of pregnant women report psychosocial stress via work-related stress as well as depressive/anxiety symptoms (Loomans et al., Citation2013). This may be relevant for transmission of intergenerational health risks (O’Connor et al., Citation2014). Correspondingly, maternal PD can be conceptualized as in utero prenatal stress exposure (PSE) to the fetus. PSE has potentially numerous adverse effects on later development such as increased risk for psychiatric disorders (O’Donnell et al., Citation2014) and less advanced cognitive development (O’Connor et al., Citation2014), including numerous phenotypes that are closely linked to brain function. Indeed, numerous studies have indicated that there are close links between PSE and child’s structural brain development (Lautarescu et al., Citation2020). Timing and nature of PSE remains also a factor, as several studies have found variable effects of distinct types of PSE on offspring outcomes, such as psychiatric disease burden and resilience factors (Guo et al., Citation2019; Khashan et al., Citation2008; Serpeloni et al., Citation2019).
Studies addressing PSE-linked functional associations have been scarce and mainly used seed-based connectivity analyses of the bilateral amygdala (Posner et al., Citation2016; Qiu et al., Citation2015; Scheinost et al., Citation2016; Soe et al., Citation2018). In these studies, the functional connectivity from amygdala to subcortical and prefrontal regions had negative associations with different measures of PSE, mainly maternal depression and anxiety symptoms, quantified by a binary system of maternal clinical diagnosis of depression/anxiety (Scheinost et al., Citation2016), Center for Epidemiological Studies Depression Scale (CES-D) (Posner et al., Citation2016) and Edinburgh Postnatal Depression Scale (EPDS) (Qiu et al., Citation2015; Soe et al., Citation2018). Our recent work extended the analyses to whole brain voxel-wise activity profiles and this work implicated a positive association between neonatal fractional amplitude of low-frequency fluctuations (fALFF) in ventromedial prefrontal cortex (vmPFC) and a positive association of frontoparietal areas with vmPFC seed-based connectivity (Rajasilta et al., Citation2022). No studies have however, mapped the effects of PSE to neonate distributed functional networks, both in terms of their spatial configuration or their dynamics over time.
In the current study, we used a composite distress score of maternal depressive and anxiety symptoms as a measure for PSE. We derived our composite distress score as a sum of individual Symptom Checklist 90 (SCL-90) anxiety subscale and Edinburgh Postnatal Depression Scale (EPDS) questionnaire scores for each mother, filled our during the 2nd trimester. We analyzed functional MRI data from 20 naturally sleeping, term-born (postmenstrual age 43.7, SD 0.7 weeks) healthy neonates acquired over 6 min with a combination of independent component analysis (ICA) and a novel method to detect the activation of functional networks over time, called Leading Eigenvector Dynamics Analysis (LEiDA) (Cabral et al., Citation2017; Stark et al., Citation2020). We hypothesized, as observed in our prior study (Rajasilta et al., Citation2022), that PSE would be associated with the dynamics of these networks. This study was explorative and thus we did not place a more specific hypothesis.
Methods
This study was conducted in accordance with the Declaration of Helsinki, and it was approved by the joint Ethics Committee of the Hospital District of Southwest Finland and University of Turku (15.03.2011) §95, ETMK: 31/180/2011. Informed written consents were obtained from parents before MRI scans were conducted.
Participants
Twenty-eight full-term born healthy infants () were randomly recruited from the FinnBrain Birth Cohort Study (Karlsson et al., Citation2018) to be included to (f)MRI scans (functional data were scanned during year 2015). Exclusion criteria included perinatal complications of neurological involvement, less than 5 points in the 5 min Apgar, previously diagnosed central nervous system anomaly, postmenstrual age at delivery less than 32 weeks and birth weight less than 1500 g.
Table 1. Demographics of subjects included in GICA and LEiDA analyses.
Demographics and maternal prenatal psychological distress measures
Obstetric data was obtained from the Finnish Medical Birth Register of the National Institute for Health and Welfare (www.thl.fi). All questionnaires assessing maternal psychological health were filled in by the mothers during the 24th gestational week. Maternal depressive symptoms during pregnancy were assessed by implementing EPDS, while assessment of maternal anxiety symptoms was performed with SCL-90. SCL-90 and EPDS scores were summed to generate a measure of maternal psychological distress (PSE composite distress score).
MR image acquisition
For a detailed description of the visit, preparations, hearing protection, MR sequences, etc., please see our prior report (Rajasilta et al., Citation2020). All scans were carried out during natural sleep at the gestation corrected age of 25.85 ± 7.72 days. Infants were fed with (breast) milk prior to the scanning session and protected from excess noise by dual hearing protection (silicone putty earplugs and earmuffs). Parents were allowed to stay in the scanner room throughout the scan, during which the session was continuously monitored by research personnel. Each infant underwent an MRI scanning session of the brain, including a 6-min resting-state fMRI sequence, conducted with Siemens Magnetom Verio 3 T MRI scanner (Siemens Medical Solutions, Erlangen, Germany) equipped with a 12-element Head Matrix coil. MRI enables safe and noninvasive studies of brain structure and function even in infants. As part of a maximum of 60-min scan, we acquired (1) T1-and T2-weighted anatomical scans at 1mm3 spatial resolution and (2) a 6-min duration EPI (Echo-planar imaging) sequence with 42 slices with voxel size of 3 × 3 × 3 mm, TR 2500 ms, TE 30 ms, FOV of 216 × 216 mm and flip-angle (FA) of 80 degrees.
Image preprocessing
The generated images of 20 subjects were preprocessed with MELODIC toolbox of FSL: motion correction, slice timing correction, brain extraction, spatial smoothing FWHM of 5 mm, grand-mean intensity normalization of the entire 4D dataset and high-pass temporal filtering (σ = 100 s). The images were co-registered to UNC infant T2 template space with linear full search and 12 degrees of freedom (DOF) using FLIRT. Subject-level ICA was used for separating noise and signal components for manual denoising. Subject-level ICA yielded 24–45 components per subject, out of which on average 51.2% (35.0–60.7%) were classified as noise components and regressed out of the data with fslregfilt. Motion parameters of the whole included sample are available in .
Table 2. Motion parameters of whole sample (N = 20).
Spatial alterations in brain networks
We first performed group ICA (GICA), which identifies networks that are common to all participants. Based on our prior work, the number of GICA components was set to 40 (Rajasilta et al., Citation2020). For this data set, out of GICA runs with 30, 40, 50, 60 and automatic dimensionality estimation (yielding 111 components), setting the number of components to 40 generated a well-balanced compromise of plausible signal and noise components (the signal components are provided in Supplementary figure 4). We then used dual regression that uses the group level ICA maps to perform multivariate temporal regression of the individual component time courses to yield subject specific spatial maps, whose properties can then be investigated in voxel-wise general linear model (GLM) with chosen covariates.
Temporal alterations in brain networks
LEiDA was chosen as the processing method for network dynamics investigation, as it detects the activation of functional networks with high temporal precision, unlike correlation-based measures of functional connectivity, which need to be calculated over sliding time windows affecting the derived metrics. Indeed, the sliding-window approach has been shown to amplify spurious variations and there is no consensus on the optimal sliding-window parameters, especially in infant data (Leonardi & Van De Ville, Citation2015; Savva et al., Citation2019). Moreover, LEiDA only considers a single vector of size 1xN to describe the phase relationships between N brain regions at each time point – rather than the full NxN matrix, which has shown to reveal functional networks that closely overlap with canonical “resting-state networks” or “intrinsic connectivity networks” in adult (Cahart et al., Citation2022; Lord et al., Citation2019; Vohryzek et al., Citation2020). To our knowledge, LEiDA has not yet been applied on infant fMRI data, but it was recently applied on early adolescent data revealing meaningful networks, indicating that these patterns may be detected across human developmental stages (Fasano et al., Citation2023).
To apply LEiDA we first obtained the average fMRI signals in 90 cortical and subcortical areas defined according to the UNC neonate AAL atlas (Automated Anatomical Labelling) using fslmeants from FSL. The time courses were then bandpass filtered (0.02–0.10 Hz), the analytic phase obtained using the Hilbert transform, and the leading eigenvectors of the phase coherence matrices were calculated at each time point (Cabral et al., Citation2017). The eigenvectors were then clustered via K-means clustering using cosine similarity as the distance measure and 200 replicates. For the purpose of this explorative study, the number of clusters (K) was varied between 2 and 8, based on previous studies indicating that the optimal number of intrinsic networks is typically between 3 and 8 (Cabral et al., Citation2017; Lord et al., Citation2019; Fasano et al., Citation2023). Each clustering yielded K patterns of phase-relationships in brain activity – or Functional Connectivity (FC) states – whose probability of occurrence can be used in statistical inference (Cabral et al., Citation2017). We placed an emphasis on describing the brain networks that emerge from each clustering solution and exploring their associations to PSE (rather than formally assessing the best clustering solution).
For the purpose of this study 20 out of 27 scanned neonates had usable data, i.e., 6 subjects were excluded due to clear over motion and one participant due to corrupted signals.
Statistical analyses
First, we used FSL randomize to perform voxel-wise statistics testing associations between GICA maps and PSE with 5000 permutations. We included as covariates/controlled for neonate sex, gestational age at scanning, and age from birth. Second, we used JASP (version 0.16.1.0) to test associations of LEiDA-derived network probabilities (conventional tabular data) of occurrence and PSE with ANCOVA models, including Levene’s test and normality checks. We included the following covariates: neonate sex, postmenstrual age at scanning, and age from birth in all models. We then performed “sensitivity analyses” that controlled for neonate birth weight and maternal pre-pregnancy Body Mass Index (BMI). We corrected the statistical tests for multiple comparison with Bonferroni correction over the number of clusters, i.e., for each independent hypothesis tested per LEiDA clustering solution.
Results
Spatial alterations in brain networks
GICA networks were expectedly well aligned with our prior report (Rajasilta et al., Citation2020). We did not find associations between GICA defined networks (displayed in Supplementary Materials, Figure 4) and PSE using dual regression.
Temporal alterations in brain networks
The repertoire of network patterns – or FC states – captured with LEiDA was found to reveal canonical resting-state networks described in the literature (Supplemental figure 1) replicating prior reports with static and dynamic methods (Eyre et al., Citation2021; Rajasilta et al., Citation2020). While the patterns obtained when clustering with K = 2 to K = 5 did not show statistically significant associations with PSE, when clustering the patterns into six clusters, we observed a robust effect of maternal composite distress score on the probability of occurrence of the frontoparietal network (, Supplemental figure 2) after controlling for neonate sex, postmenstrual age at scanning and age from birth, F(1, 18) = 15.774, p = 0. 001, ω2 = 0.293, which survived Bonferroni correction over the number of independent hypotheses tested (corrected p = 0.006). This association was specific to the implicated frontoparietal network (Supplemental figure 3).
Figure 1. Maternal prenatal distress associates negative with the probability of occurrence of a frontoparietal network: (A) visualization of the brain network #3 identified from clustering solution with 6 clusters; (B) scatter plot of the association between probability of occurrence and PSE (spearman’s rho = −0.659, p = 0.004, Bonferroni-corrected p = 0.024).
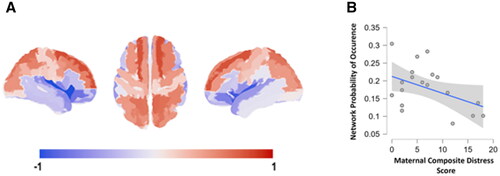
Sensitivity analyses
Levene’s test and normality checks were carried out and the assumptions met. The effect sizes were smaller but statistically significant in our sensitivity analyses where maternal-pre-pregnancy BMI and neonate birth weight were additionally controlled for, F(1, 18) = 7.757, p = 0.015, ω2 = 0.170). This network also appeared in clustering solutions with seven (FC state 4) and eight (FC state 4) networks and had similar associations with PSE composite distress score in fully controlled ANCOVA models that included all covariates of the sensitivity analyses (K = 7, F(1, 18) = 7.997, p = 0.014, ω2 = 0.183; K = 8, F(1, 18) = 5.637, p = 0.034, ω2 = 0.127). This gives assurance that the effects are not subject to clustering solutions. We also performed ANCOVA and partial correlation analysis for our main result with mean FD as an additional covariate of no interest. No significant change to our main result was observed for either models (For ANCOVA, F(1, 18) = 14.020; p = 0.002, ω2 = 0.283; For partial correlation analysis, Spearman’s rho = −0.653, p = 0.006, Bonferroni corrected p = 0.036).
Finally, we performed ANCOVA and partial correlation analysis to uncover whether neonate age variables independently associated with our GICA results and frontoparietal network probability of occurrence. There were no statistically significant relationships between any age variable and GICA results. The brain network of interest from LEiDA showed a negative associated to age from birth (Spearman’s rho = − 0.471, p = 0.036) and was also statistically significant in our ANCOVA model F(1, 18) = 10.636, p = 0.005)
Discussion
The current study probed the links between PSE and neonatal whole brain networks for both spatial and dynamic features. We found a statistically robust negative association with the probability of occurrence (stability) of a frontoparietal network and PSE that was defined as a composite distress score of depressive and anxiety symptoms. No associations were found between static network FC and PSE. To bring our findings to context, we briefly review the four prior fMRI studies that have addressed the links between PSE and neonate brain function, as well as one fetal fMRI study. Here we focus on the functional MRI studies that focused on the associations between neonatal brain functional and maternal PSE, but we note that there is a rich literature on maternal health, including maternal PSE, and neonate brain structure (Lautarescu et al., 2020; Pulli et al., Citation2019).
Thomason et al. (Citation2021) investigated prenatal stress exposure-associated functional connectivity changes in ca. 32.9 week-old fetuses (N = 118; 48 female). PSE was assessed using a single summation score derived from five different self-reports questionnaires: Center for Epidemiological Studies Depression Scale (CES-D), the State Trait Anxiety Inventory (STAI-T), the Penn State Worry Questionnaire (PSWQ), the Perceived Stress Scale (PSST) and the Satisfaction with Life Scale (SWLS). They also employed maternal salivary cortisol measurements, when available. Fetal brain networks were derived using functional parcellation of the functional MRI data (197 ROIs). Brain-stress relationships were then assessed using mixture modeling. The researchers found alterations in fetal FC encompassing intra-hemispheric superior frontal and motor regions, as well as interhemispheric posterior insula, temporoparietal brain regions and striatum. Supporting the current study, they also found significant differences in FC between anterior and posterior frontal and parietal regions, as well as within connectivity of superior frontal gyrus associating with intrauterine stress exposure. Finally, they revealed an inverse relationship between fetal gestational age and overall magnitude of maternal stress.
Scheinost et al. (Citation2016) used two data sets/cohorts focusing on very/extremely preterm neonates with or without PSE, defined as a binary classification for maternal prenatal diagnosis of depression, compared with term controls. Seed-based connectivity analyses revealed that very preterm subjects exhibit reduced amygdala connectivity to subcortical structures when compared to term controls. Extremely preterm neonates with PSE have further reduced amygdala FC to subcortical structures when compared to extremely preterm neonates without PSE indicating that PSE further reduces amygdala FC to subcortical structures.
Posner et al. (Citation2016) studied 64 infants with (n = 20) and without (n = 44) in utero exposure to prenatal maternal depression. PSE-exposed infants had more negative functional connectivity between amygdala and dorsal prefrontal cortex (AMG-dPFC) and altered AMG-dPFC FC further associated with greater prenatally assessed stress responses (fetal heart rate measurements). Of note, this study also assessed structural connectivity and found decreased structural connectivity between right amygdala and right ventral prefrontal cortex in neonates with PSE exposure.
Qiu et al. (Citation2015) studied older infants, 24 infants at 6 months age (12 males and 12 females). PSE was assessed via self reports with Edinburgh prenatal depression scale (EPDS) at 26 weeks of gestation and 3 months after delivery. Pre- and postnatal EPDS scores that were found to be similar. They found a positive association between EPDS scores and infant left amygdala FC between left temporal cortex, insula and bilateral anterior cingulate cortex (ACC), medial prefrontal cortex (mPFC) and ventromedial prefrontal cortex (vmPFC).
Another study from the same cohort studied similar associations in older children. Soe et al. (Citation2018) scanned 128 children at 4.4–4.8 years of age. PSE was measured with maternal self reports via EPDS-questionnaire at 26 wk of gestation, 3 months, 1, 2, 3 and 4.5 years after delivery. The authors used prenatal EPDS scores, a mean EPDS score value of postnatal EPDS scores and their difference as variables of interest. They found no associations in boys. In girls they found that prenatal PSE (depressive symptoms) associated with FC of amygdala and cortico-striatal circuitry (orbitofrontal cortex, insula, anterior cingulate cortex, temporal pole, striatum). Further, in girls only, greater pre- than post-natal depressive symptoms associated with lower FC between left amygdala and bilateral anterior cingulate cortex, left caudate; right amygdala and left orbitofrontal cortex, insula and temporal pole.
Taken together, the main method for studying links between PSE in newborns has been seed-based connectivity of the amygdala, and implicated regions encompass striatal and frontal brain areas with PSE associating to decreased connectivity. All prior studies, including our own, is cross sectional, and definition of maternal prenatal “stress” is variable across studies. Our study used a general population-based, non-clinical sample and used a continuum of symptom scores similar to some prior reports (Qiu et al., Citation2015; Soe et al., Citation2018). Although no clear clinical cutoff scores have been established for EPDS and SCL, generally a score of 10 has been implicated as a clinically meaningful threshold for symptoms of depression or anxiety in pregnancy (Karlsson et al., Citation2018). It is important to note that maternal prenatal stress is only partly captured by depressive and anxiety measurements. More comprehensive approaches may reveal patterns of stress exposure that better explain offspring outcomes at peripartum and postnatally (Walsh et al., Citation2019).
During normal development, amygdala seed-based FC becomes negatively associated with age at many overlapping posterior brain regions found in the frontoparietal areas (Gabard-Durnam et al., Citation2018). The developmental trajectories of the connectivity indicate that frontoparietal networks are slow to achieve adult-like topology (Gao et al., Citation2015), which may render them vulnerable to stressors (Graham et al., Citation2021). Overall, there is cumulating evidence pointing toward stress exposure associated with accelerated brain maturation (Gao et al., Citation2015; Graham et al., Citation2021), and this may well be reflected in amygdalar FC to frontal and parietal regions.
The overall implication of destabilized frontoparietal network dynamics is intriguing as similar network patterns are frequently linked to later child outcomes – albeit with different fMRI methods. Frontoparietal regions have been linked to PSE in fetal studies (Graham et al., Citation2021) and also to behavioral phenotypes in 1-month-old infants (Kelsey et al., Citation2021). Later in development, frontoparietal networks are often referred to as attention networks and are implicated in neurodevelopmental disorders (Wang et al., Citation2019) and mental health (Schultz et al., Citation2018). The frontoparietal network identified in LEiDA associated negatively with both maternal prenatal distress scores and age from birth (but not with PMA), which could be a sign of accelerated early postnatal brain development. This would be in line with stress acceleration hypothesis (Callaghan & Tottenham, Citation2016). However, we note that there is scarce information on normative knowledge on neonatal brain dynamics and that we did not obtain measures of early child rearing, which could also be affected by perinatal distress.
Limitations
The current study had a small sample size, and future larger-scale studies are needed to confirm our findings that are best regarded as preliminary. Additionally, normal features and developmental changes in neonatal dynamic functional connectivity are not well understood, which limits the interpretability of the findings. However, there are recent reports with similar methodology that give insights into typical functional network dynamics in neonates (França et al., Citation2022). Our cross-sectional measurements do not allow causal inference and distress scales are based on self-reports, and are unable to capture mediating biological/physiological mechanisms.
Conclusions
We found that stability of a frontoparietal network is negatively associated with maternal mid-pregnancy PSE (defined as a composite distress score of depressive and anxiety symptoms). This study adds to the cumulating evidence that frontal brain areas and associated networks are potentially especially sensitive to PSE. There is a dire need for studies replicating and extending prior studies in larger sample sizes to define the clinical relevance of these findings. Similarly, future research should also be directed toward intervention efficacy in pregnant mothers to uncover best strategies for potential harm mitigation.
Supplemental Material
Download Zip (3.2 MB)Acknowledgements
We thank all FinnBrain families that took part in the MRI studies, our radiographer Krisse Kuvaja for performing the imaging, and the FinnBrain Birth Cohort study staff.
Disclosure statement
No potential conflict of interest was reported by the author(s).
Data availability statement
The Finnish law and ethical permissions do not allow the sharing of the data used in this study.
Additional information
Funding
Notes on contributors
Jetro J. Tuulari
Jetro J. Tuulari, M.D., Ph.D., has completed a post-doctoral fellowship at the Department of Psychiatry at the University of Oxford, UK and is currently working as the principal investigator of the FinnBrain Neuroimaging Lab and director of Turku Brain and Mind Center, based at the University of Turku, Finland.
Olli Rajasilta
Olli Rajasilta is a medical doctor specializing in neurology and a PhD student in FinnBrain study, based at the University of Turku, Finland.
Joana Cabral
Joana Cabral is a Biomedical Engineer with a PhD in Theoretical and Computational Neuroscience. After a postdoc at the Department of Psychiatry at the University of Oxford, UK, Joana is currently Assistant Researcher at the Life and Health Sciences Research Institute as part of the STRESS.COM team.
Morten L. Kringelbach
Morten Kringelbach is a professor and a principal investigator at Department of Psychiatry (University of Oxford, UK), Center for Music in the Brain, and Flavour Institute (Aarhus University, Denmark). He is also the director of the Centre for Eudaimonia and Human Flourishing based at the University of Oxford, UK.
Linnea Karlsson
Linnea Karlsson is a professor, co-leader of the Centre for Population Health Research in the University of Turku, and Principal Investigator of the FinnBrain Birth cohort study based at the University of Turku, Finland.
Hasse Karlsson
Hasse Karlsson is a professor and Principal Investigator of the FinnBrain Birth cohort study based at the University of Turku, Finland. He is also the Chair of the National Steering Board at Neurocenter Finland.
References
- Cabral, J., Vidaurre, D., Marques, P., Magalhães, R., Silva Moreira, P., Miguel Soares, J., Deco, G., Sousa, N., & Kringelbach, M. L. (2017). Cognitive performance in healthy older adults relates to spontaneous switching between states of functional connectivity during rest. Scientific Reports, 7(1), 1. https://doi.org/10.1038/s41598-017-05425-7
- Cahart, M.-S., Dell’Acqua, F., Giampietro, V., Cabral, J., Timmers, M., Streffer, J., Einstein, S., Zelaya, F., Williams, S. C. R., & O’Daly, O. (2022). Test-retest reliability of time-varying patterns of brain activity across single band and multiband resting-state functional magnetic resonance imaging in healthy older adults. Frontiers in Human Neuroscience, 16, 980280. https://doi.org/10.3389/fnhum.2022.980280
- Callaghan, B. L., & Tottenham, N. (2016). The Stress Acceleration Hypothesis: Effects of early-life adversity on emotion circuits and behavior. Current Opinion in Behavioral Sciences, 7, 76–8. https://doi.org/10.1016/j.cobeha.2015.11.018
- Eyre, M., Fitzgibbon, S. P., Ciarrusta, J., Cordero-Grande, L., Price, A. N., Poppe, T., Schuh, A., Hughes, E., O’Keeffe, C., Brandon, J., Cromb, D., Vecchiato, K., Andersson, J., Duff, E. P., Counsell, S. J., Smith, S. M., Rueckert, D., Hajnal, J. V., Arichi, T., … Edwards, A. D. (2021). The Developing Human Connectome Project: Typical and disrupted perinatal functional connectivity. Brain: a Journal of Neurology, 144(7), 2199–2213. https://doi.org/10.1093/brain/awab118
- Fasano, M. C., Cabral, J., Stevner, A., Vuust, P., Cantou, P., Brattico, E., & Kringelbach, M. L. (2023). The early adolescent brain on music: Analysis of functional dynamics reveals engagement of orbitofrontal cortex reward system. Human Brain Mapping, 44(2), 429–446. https://doi.org/10.1002/hbm.26060
- França, L. G. S., Ciarrusta, J., Gale-Grant, O., Fenn-Moltu, S., Fitzgibbon, S., & Chew, A. (2022). Neonatal brain dynamic functional connectivity: Impact of preterm birth and association with early childhood neurodevelopment. bioRxiv, 22, 2022.11.16.516610. https://www.biorxiv.org/content/ https://doi.org/10.1101/2022.11.16.516610v2%0Ahttps://www.biorxiv.org/content/10.1101/2022.11.16.516610v2.abstract
- Gabard-Durnam, L. J., O’Muircheartaigh, J., Dirks, H., Dean, D. C., Tottenham, N., & Deoni, S. (2018). Human amygdala functional network development: A cross-sectional study from 3 months to 5 years of age. Developmental Cognitive Neuroscience, 34(February), 63–74. https://doi.org/10.1016/j.dcn.2018.06.004
- Gao, W., Alcauter, S., Elton, A., Hernandez-Castillo, C. R., Smith, J. K., Ramirez, J., & Lin, W. (2015). Functional network development during the first year: Relative sequence and socioeconomic correlations. Cerebral Cortex, 25(9), 2919–2928. https://doi.org/10.1093/cercor/bhu088
- Graham, A. M., Marr, M., Buss, C., Sullivan, E. L., & Fair, D. A. (2021). Understanding vulnerability and adaptation in early brain development using network neuroscience. Trends in Neurosciences, 44(4), 276–288. https://pubmed.ncbi.nlm.nih.gov/33663814 https://doi.org/10.1016/j.tins.2021.01.008
- Guo, C., He, P., Song, X., & Zheng, X. (2019). Long-term effects of prenatal exposure to earthquake on adult schizophrenia. The British Journal of Psychiatry: The Journal of Mental Science, 215(6), 730–735. https://doi.org/10.1192/bjp.2019.114
- Karlsson, L., Tolvanen, M., Scheinin, N. M., Uusitupa, H.-M., Korja, R., Ekholm, E., Tuulari, J. J., Pajulo, M., Huotilainen, M., Paunio, T., & Karlsson, H. (2018). Cohort profile: The FinnBrain Birth Cohort Study (FinnBrain). International Journal of Epidemiology, 47(1), 15–16j. https://doi.org/10.1093/ije/dyx173
- Kelsey, C. M., Farris, K., & Grossmann, T. (2021). Variability in infants’ functional brain network connectivity is associated with differences in affect and behavior. Frontiers in Psychiatry, 12(June), 685754. https://doi.org/10.3389/fpsyt.2021.685754
- Khashan, A. S., Abel, K. M., McNamee, R., Pedersen, M. G., Webb, R. T., Baker, P. N., Kenny, L. C., & Mortensen, P. B. (2008). Higher risk of offspring schizophrenia following antenatal maternal exposure to severe adverse life events. Archives of General Psychiatry, 65(2), 146–152. https://doi.org/10.1001/archgenpsychiatry.2007.20
- Lautarescu, A., Craig, M. C., & Glover, V. (2020). Chapter Two – Prenatal stress: Effects on fetal and child brain development. International Review of Neurobiology, 150, 17–40. https://www.sciencedirect.com/science/article/pii/S0074774219301199
- Lautarescu, A., Craig, M. C., & Glover, V. (2020). Prenatal stress: Effects on fetal and child brain development. International Review of Neurobiology, 150, 17–40. https://doi.org/10.1016/bs.irn.2019.11.002
- Leonardi, N., & Van De Ville, D. (2015). On spurious and real fluctuations of dynamic functional connectivity during rest. Neuroimage, 104, 430–436. https://www.sciencedirect.com/science/article/pii/S1053811914007496 https://doi.org/10.1016/j.neuroimage.2014.09.007
- Loomans, E. M., van Dijk, A. E., Vrijkotte, T. G. M., van Eijsden, M., Stronks, K., Gemke, R. J. B. J., & Van den Bergh, B. R. H. (2013). Psychosocial stress during pregnancy is related to adverse birth outcomes: Results from a large multi-ethnic community-based birth cohort. European Journal of Public Health, 23(3), 485–491. https://doi.org/10.1093/eurpub/cks097
- Lord, L.-D., Expert, P., Atasoy, S., Roseman, L., Rapuano, K., Lambiotte, R., Nutt, D. J., Deco, G., Carhart-Harris, R. L., Kringelbach, M. L., & Cabral, J. (2019). Dynamical exploration of the repertoire of brain networks at rest is modulated by psilocybin. NeuroImage, 199, 127–142. https://doi.org/10.1016/j.neuroimage.2019.05.060
- O’Connor, T. G., Monk, C., & Fitelson, E. M. (2014). Practitioner review: Maternal mood in pregnancy and child development – implications for child psychology and psychiatry. Journal of Child Psychology and Psychiatry, and Allied Disciplines, 55(2), 99–111. https://doi.org/10.1111/jcpp.12153
- O’Donnell, K. J., Glover, V., Barker, E. D., & O’Connor, T. G. (2014). The persisting effect of maternal mood in pregnancy on childhood psychopathology. Development and Psychopathology, 26(2), 393–403. https://www.cambridge.org/core/article/persisting-effect-of-maternal-mood-in-pregnancy-on-childhood-psychopathology/E13852533BAB5648488004E36BA24C75 https://doi.org/10.1017/S0954579414000029
- Posner, J., Cha, J., Roy, A. K., Peterson, B. S., Bansal, R., Gustafsson, H. C., Raffanello, E., Gingrich, J., & Monk, C. (2016). Alterations in amygdala-prefrontal circuits in infants exposed to prenatal maternal depression. Translational Psychiatry, 6(11), e935-8–e935. https://doi.org/10.1038/tp.2016.146
- Pulli, E. P., Kumpulainen, V., Kasurinen, J. H., Korja, R., Merisaari, H., Karlsson, L., Parkkola, R., Saunavaara, J., Lähdesmäki, T., Scheinin, N. M., Karlsson, H., & Tuulari, J. J. (2019). Prenatal exposures and infant brain: Review of magnetic resonance imaging studies and a population description analysis. Human Brain Mapping, 40(6), 1987–2000. https://doi.org/10.1002/hbm.24480
- Qiu, A., Anh, T. T., Li, Y., Chen, H., Rifkin-Graboi, A., Broekman, B. F. P., Kwek, K., Saw, S.-M., Chong, Y.-S., Gluckman, P. D., Fortier, M. V., & Meaney, M. J. (2015). Prenatal maternal depression alters amygdala functional connectivity in 6-month-old infants. Translational Psychiatry, 5(2), e508–e508. https://doi.org/10.1038/tp.2015.3
- Rajasilta, O., Häkkinen, S., Björnsdotter, M., Scheinin, N. M., Lehtola, S. J., Saunavaara, J., Parkkola, R., Lähdesmäki, T., Karlsson, L., Karlsson, H., & Tuulari, J. J. (2022). Maternal psychological distress associates with alterations in resting-state low-frequency fluctuations and distali functional connectivity of the neonate prefrontal cortex. The European Journal of Neuroscience, 57(2), 242–257.
- Rajasilta, O., Tuulari, J. J., Björnsdotter, M., Scheinin, N. M., Lehtola, S. J., Saunavaara, J., Häkkinen, S., Merisaari, H., Parkkola, R., Lähdesmäki, T., Karlsson, L., & Karlsson, H. (2020). Resting-state networks of the neonate brain identified using independent component analysis. Developmental Neurobiology, 80(3–4), 111–125. https://doi.org/10.1002/dneu.22742
- Savva, A. D., Mitsis, G. D., & Matsopoulos, G. K. (2019). Assessment of dynamic functional connectivity in resting-state fMRI using the sliding window technique. Brain and Behavior, 9(4), e01255. https://doi.org/10.1002/brb3.1255
- Scheinost, D., Kwon, S. H., Lacadie, C., Sze, G., Sinha, R., Constable, R. T., & Ment, L. R. (2016). Prenatal stress alters amygdala functional connectivity in preterm neonates. NeuroImage. Clinical, 12, 381–388. https://doi.org/10.1016/j.nicl.2016.08.010
- Schultz, D. H., Ito, T., Solomyak, L. I., Chen, R. H., Mill, R. D., Anticevic, A., & Cole, M. W. (2018). Global connectivity of the fronto-parietal cognitive control network is related to depression symptoms in the general population. Network Neuroscience, 3(1), 107–123. https://doi.org/10.1162/netn_a_00056
- Serpeloni, F., Radtke, K. M., Hecker, T., Sill, J., Vukojevic, V., de Assis, S. G., Schauer, M., Elbert, T., & Nätt, D. (2019). Does prenatal stress shape postnatal resilience? An epigenome-wide study on violence and mental health in humans. Frontiers in Genetics, 10(MAR), 269. https://doi.org/10.3389/fgene.2019.00269
- Soe, N. N., Wen, D. J., Poh, J. S., Chong, Y.-S., Broekman, B. F., Chen, H., Shek, L. P., Tan, K. H., Gluckman, P. D., Fortier, M. V., Meaney, M. J., & Qiu, A. (2018). Perinatal maternal depressive symptoms alter amygdala functional connectivity in girls. Human Brain Mapping, 39(2), 680–690. https://doi.org/10.1002/hbm.23873
- Stark, E. A., Cabral, J., Riem, M. M. E., Van IJzendoorn, M. H., Stein, A., & Kringelbach, M. L. (2020). The power of smiling: The adult brain networks underlying learned infant emotionality. Cerebral Cortex, 30(4), 2019–2029. https://doi.org/10.1093/cercor/bhz219
- Thomason, M. E., Hect, J. L., Waller, R., & Curtin, P. (2021). Interactive relations between maternal prenatal stress, fetal brain connectivity, and gestational age at delivery. Neuropsychopharmacology, 46(10), 1839–1847. Available from https://doi.org/10.1038/s41386-021-01066-7
- Vohryzek, J., Deco, G., Cessac, B., Kringelbach, M. L., & Cabral, J. (2020). Ghost attractors in spontaneous brain activity: recurrent excursions into functionally-relevant BOLD phase-locking states. Frontiers in Systems Neuroscience, 14, 20. https://doi.org/10.3389/fnsys.2020.00020
- Walsh, K., McCormack, C. A., Webster, R., Pinto, A., Lee, S., Feng, T., Krakovsky, H. S., O’Grady, S. M., Tycko, B., Champagne, F. A., Werner, E. A., Liu, G., & Monk, C. (2019). Maternal prenatal stress phenotypes associate with fetal neurodevelopment and birth outcomes. Proceedings of the National Academy of Sciences of the United States of America, 116(48), 23996–24005. https://doi.org/10.1073/pnas.1905890116
- Wang, Y., Tao, F., Zuo, C., Kanji, M., Hu, M., & Wang, D. (2019). Disrupted resting frontal–parietal attention network topology is associated with a clinical measure in children with attention-deficit/hyperactivity disorder. Frontiers in Psychiatry, 10(MAY), 300. https://doi.org/10.3389/fpsyt.2019.00300