Abstract
This study investigates the differences in hip biomechanics for subjects following a total hip arthroplasty (THA), through the lateral approach (LA) and posterior approach (PA), to those with no pathology (NP). The principal component analysis was performed on two kinematic and two kinetic waveforms (subject-based characteristics) from level gait to identify salient portions of the waveforms for comparison between the subject cohorts. These were classified to identify the differences between post-THA and non-pathological cohorts. The primary technique exposited in the THA analysis is classification and ranking belief simplex (CaRBS). Within the analysis, from the configuration of a CaRBS model, there is discussion on the model fit and contribution of the subject-based characteristics. Where appropriate, comparisons to the CaRBS model are made with the results from a logistic regression (LR) analysis. In terms of model fit, using CaRBS, 24 out of 27 LA/PA subjects (88.89%) and 13 out of 16 NP subjects (81.25%) were correctly classified as exhibiting either post-THA or NP hip functional characteristics during level gait, combining to 86.05% classification accuracy, compared with 81.40% classification accuracy when using LR.
1. Introduction
Total hip arthroplasty (THA) is routinely performed to effectively relieve the pain and disability associated with hip osteoarthritis (Ritter et al. Citation1995). This study determines whether differences in gait exist between subjects with a THA and those with no pathology (NP), using kinematic and kinetic subject-based characteristics in this classification problem.
The classification and ranking belief simplex (Beynon Citation2005a, Citation2005b; Beynon et al. Citation2006), known as CaRBS, is a novel technique for analysing the data where there may be imprecision, inexactness and uncertainty in its information content (Beynon et al. Citation2006). The ability of the CaRBS technique to work with the notion of uncertain reasoning is due to its mathematical rudiments being based on the Dempster–Shafer theory (Dempster Citation1967; Shafer Citation1976). It therefore intuitively lends itself to the analysis of large amounts of data generated from a motion analysis data-set, which is both corroborating and conflicting in nature.
Despite the continued success of a THA, the hip recovered biomechanical function during level gait does not necessarily return to what is typically quantified as normal (Madsen et al. Citation2004; Whatling et al. Citation2008). There are numerous surgical approaches to THA, each compromising different muscles and static constraints surrounding the hip. For this reason, the surgical approach used may affect both the biomechanics and stability of a total hip replacement. Studies have shown that lateral surgical access to the hip joint weakens the hip abductors, affecting the mechanical ability of the abductor mechanism to control the hip and pelvis in the frontal plane (Baker and Bitounis Citation1989; Dijk et al. Citation2009), whereas posterior surgical access compromises the joint capsule and external rotator muscle group, affecting the posterior and lateral stability of the hip joint (Gore et al. Citation1982). It is important to establish whether this is an influential factor on patient recovery as there is currently no consensus on the best surgical approach (Gore et al. Citation1982).
Two principal surgical approaches are the McFarland–Osborne direct lateral approach (LA; McFarland and Osborne Citation1954) and the Moore (southern exposure) posterior approach (PA; Moore Citation1959). In an earlier study by Whatling et al. (Citation2008), the motion analysis of level gait revealed that subjects following the PA used a greater range of pelvic obliquity (motion of their pelvis in the frontal plane), and larger frontal plane powers and moments were measured at the hip, as compared to those following the LA. Subjects following the LA were also found to have a significantly lower range of motion (ROM) in the sagittal plane for their operative hip as compared to their non-operative hip. A significant challenge to the clinical use of gait information is the successful analysis of the data (Chau Citation2001). A common difficulty is not only the vast amount of data yielded but its variability which can be difficult to interpret subjectively.
The aims of this study are to (1) demonstrate the use of the nascent CaRBS technique in assessing the gait characteristics to discern between subject groups associated with THA function (LA and PA) and NP; (2) investigate the level of model fit using the CaRBS model by comparing with the results from a logistic regression (LR) analysis, a technique commonly used in gait-based studies (Archer et al. Citation2006; Chang et al. Citation2007) and (3) quantify and visually represent the contribution of each subject-based characteristic to the CaRBS analysis.
2. Methods
The differences in non-pathological and THA hip gait biomechanics were investigated from the motion analysis data using principal component analysis (PCA), CaRBS and LR. The selection of gait characteristics and analyses is described below.
2.1 Motion analysis
Biomechanical gait data collected using 3D motion analysis techniques (Qualisys, Sweden; Bertec Corp and Visual3D, C-motion, Inc.) were used in the analyses (Whatling et al. Citation2008). In this study, 14 subjects had received THA via the McFarland–Osborne – direct LA and 13 subjects via the Moore – southern exposure PA. Sixteen hips with NP were assessed, forming a control subject cohort.
The following four kinematic and kinetic subject-based characteristics, sagittal plane hip rotation, pelvic obliquity rotation, frontal plane power at the hip during stance and frontal plane moment at the hip during stance, were used in the analysis since discrete measures from them were found to be significantly different for THA (LA or PA) and NP function during level gait, determined using independent sample t-tests (Whatling et al. Citation2008). A summary of these significant differences, identified in Whatling et al. (Citation2008), is next reported:
1. | Sagittal plane hip rotation: sagittal plane hip ROM was significantly greater for the NP subject cohort (46.94 ± 5.73°) compared to the LA subject cohort (28.72 ± 6.67°), PA subject cohort (33.88 ± 7.12°) and combined LA and PA subject cohorts (31.21 ± 7.25°). | ||||
2. | Pelvic obliquity rotation: pelvic frontal ROM was significantly greater for the NP subject cohort (6.88 ± 3.29°) compared to the LA subject cohort (4.32 ± 1.08°), PA subject cohort (5.03 ± 1.64°) and combined LA and PA subject cohorts (4.66 ± 1.40°). | ||||
3. | Frontal plane power at the hip during stance: hip frontal power at 50% stance was significantly different for the NP subject cohort (0.20 ± 0.12 W/kg) compared to the LA subject cohort (0.83 ± 0.71 W/kg), PA subject cohort (0.16 ± 0.12 W/kg) and combined LA and PA subject cohorts (0.12 ± 0.10 W/kg). Peak frontal power during the stance phase of gait was significantly greater for the NP subject cohort (0.75 ± 0.31 W/kg) compared to the LA subject cohort (0.39 ± 0.20 W/kg), PA subject cohort (0.56 ± 0.23 W/kg) and combined LA and PA subject cohorts (0.47 ± 0.23 W/kg). | ||||
4. | Frontal plane moment at the hip during stance: peak hip frontal moment in stance was significantly greater for the NP subject cohort (0.97 ± 0.15 Nm/kg) compared to the LA subject cohort (0.70 ± 0.24 Nm/kg), PA subject cohort (0.89 ± 0.20 Nm/kg) and combined LA and PA subject cohorts (0.79 ± 0.24 Nm/kg). |
Using discrete measures from kinematic and kinetic subject-based characteristics is common practice in gait analysis studies (Madsen et al. Citation2004; Whatling et al. Citation2008). However, there is a danger in discarding valuable temporal information. Therefore, PCA (Jones et al. Citation2008) was performed on the kinematic and kinetic waveforms from the combined LA, PA and NP subject cohorts to represent these subject-based characteristics in a discrete form while retaining temporal information. The first PC from each waveform was used as an input to CaRBS, termed here, C1, C2, C3 and C4, one for each of the four subject-based characteristics. Details of the waveforms for each gait characteristic are graphically reported in Figure and described in Table .
Figure 1 Waveforms for (a) hip extension/flexion; (b) pelvic obliquity; (c) frontal plane power at the hip and (d) frontal plane moment at the hip. Principal components C1,…,C4, are highlighted by the grey regions on each waveform (the NP (std) is NP +1 and − 1 std, and NP (2std) is NP +2 and − 2 std).
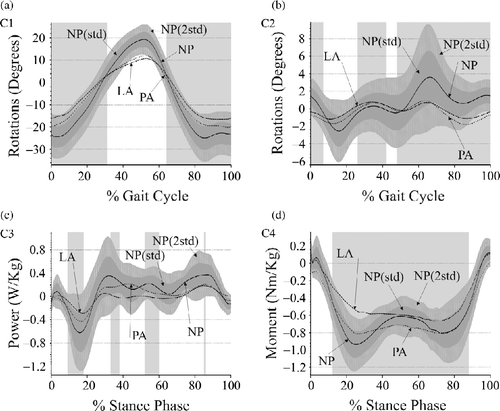
Table 1. Descriptions of PCs reflecting the subject-based characteristics C1, C2, C3 and C4.
The PCs were interpreted by determining the periods of the gait cycle they represent. The relationship between the magnitude of each PC and the original waveform was determined through the examination of the waveforms corresponding to the 5th and 95th percentiles of the first PC scores. The PCs represent the portions of the kinematic and kinetic waveforms where the largest differences exist between the LA, PA and NP subject cohorts. C1 highlights the portions of waveform where the hip is in the greatest flexion. C2 represents portions of the stance phase and the whole of the swing phase, where there is a notable difference in pelvic obliquity between the LA, PA and NP subject cohorts. Four regions of the frontal plane power waveform are represented by C3. This includes the peak power, previously identified as a significant variable in Whatling et al. (Citation2008). The majority of the frontal plane moment during stance phase is represented by C4, including peak values, which were previously identified as being significant in the comparison of THA and NP function (Whatling et al. Citation2008).
2.2 CaRBS and logistic regression methods
The details of the CaRBS technique are presented in the Appendix (along with the description of Dempster–Shafer theory upon which it is structured). For examples of the incumbent calculations forming part of the configuration process, see the studies of Beynon (Citation2005a) and (Citation2005b). Here, emphasis is on the results from the configuration of a CaRBS model using the previously described THA data-set. Importantly, since the CaRBS technique undertakes binary classification, the two surgical types considered are: having had surgery (LA or PA; in Appendix x = {LA, PA}) and NP (¬x = {NP}).
From the Appendix, the configuration process includes the construction of characteristic body of evidences (BOEs) m j,i (·), which describe, for the jth subject, the association evidence evident in the ith characteristic (one of the C1,…,C4 subject-based characteristics). For an individual subject, the evidence contained in the respective characteristic BOEs (one for each characteristic describing them) is combined to form a subject BOE m j (·) (made up of a triplet of mass values, m j ({LA, PA}), m j ({NP}) and m j ({LA, PA, NP})). It is the subject BOEs that contain the predicted association information that discerns (classifies) subjects to their appropriate surgical types, in this case, surgery ({LA, PA}), NP ({NP}) as well as a level of concomitant ignorance ({LA, PA, NP}).
To benchmark the level of model fit exhibited by the configured CaRBS model, results are briefly presented using LR, a technique previously used in gait-based research (Archer et al. Citation2006; Chang et al. Citation2007). For this analysis, where there is a binary dependent variable (DV), the numerical values, DV = 1 and DV = 0, were assigned to the subjects known to be associated with having had surgery (using LA or PA) or NP, respectively. In this study, the notion of model fit is in terms of classification accuracy.
2.3 Contribution of subject-based characteristics
The contribution of each subject-based characteristic used in discerning the association of subjects based on having had surgery or NP was investigated.
In the CaRBS analysis, the elucidation of this contribution initially uses the average characteristic BOEs (defined in Appendix), which accrue the level of evidence from subject-based characteristics to certain equivalence classes of subjects (known to have had LAs or or PAs to surgery ({LA, PA}) or NP ({NP})). The BOEs evaluated are graphically reported using simplex plots (see Beynon Citation2005a, Citation2005b).
With respect to the LR analysis also undertaken on the THA data-set, the contribution of the subject-based characteristic is measured through the LR coefficients.
3. Results
The results from CaRBS and LR analyses of the PCs are given below. The contributions of each characteristic PC to the classifications are also detailed.
3.1 CaRBS and logistic regression analyses of THA and NP data-sets
In the CaRBS analysis, post the configuration process (see Appendix for technical details), the positioning of the predicted association of a subject in a simplex plot (a subject BOE represented by a simplex coordinate), to the LAs/PAs to surgery and NP, can be undertaken for each of the 43 subjects considered, see Figure .
Figure 2 Simplex plot-based representation of final subject BOEs, post configuration of CaRBS model, for (a) LA, PA and (b) NP surgical types.
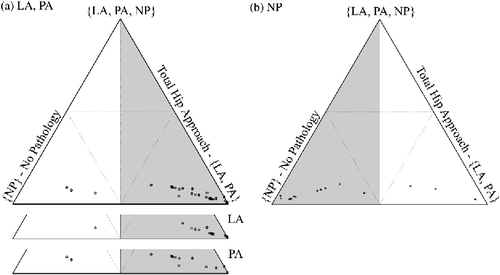
From Figure , 24 out of 27 LA/PA subjects (88.89%) and 13 out of 16 NP subjects (81.25%) were correctly classified as exhibiting either post THA or NP hip functional characteristics during level gait. This combines to a total of 86.05% classification accuracy. The main simplex plots (equilateral triangles) in Figure (a),(b) partition the presentation of the subjects' subject BOEs between those known to be associated with having LAs or PAs to surgery (a) and NP (b), where each subject BOE is labelled with a circle and a cross, respectively. The subject BOEs are positioned either side of the vertical dashed lines in the simplex plots in Figure , based on their simplex coordinates. The correct side is denoted by the grey-shaded regions.
For further elucidation, in Figure (a), two sub-domains are presented below the simplex plot showing the final subject BOEs (via their simplex coordinates), separately, for those subjects known to have had LA and PA surgery. That is, while the full 27 subjects associated with LA or PA were considered collectively when configuring the CaRBS model (binary classification), the simplex plot sub-domains reported allow an inspection of how well the separate LA and PA subjects were appropriately classified. A visual inspection of the simplex coordinates in the simplex plots in Figure shows that 13 out of 14 of LA (92.86%) and 11 out of 13 of PA (84.62%) were correctly classified. In Figure (a), the positions of the correctly classified LA subjects are closer to the {LA, PA} vertex than those of the PA subjects correctly classified. This indicates a greater ability to discern subjects associated with surgery through the LA from those with NP, than for those associated with the PA to surgery.
In the LR analysis, 24 out of 27 LA/PA subjects (88.89%) and 11 out of 16 NP subjects (68.75%) were correctly classified combining to a total of 81.40% classification accuracy. These results show, in this case, that the CaRBS analysis has performed better in terms of modelling fit.
3.2 Contribution of subject-based characteristics
In the CaRBS analysis, the associated average characteristics are presented in Figure (average characteristic BOEs exist only in the grey-shaded sub-domain shown – where characteristic BOEs exist).
Figure 3 Description of levels of contribution of subject-based characteristics based on average characteristic BOEs.
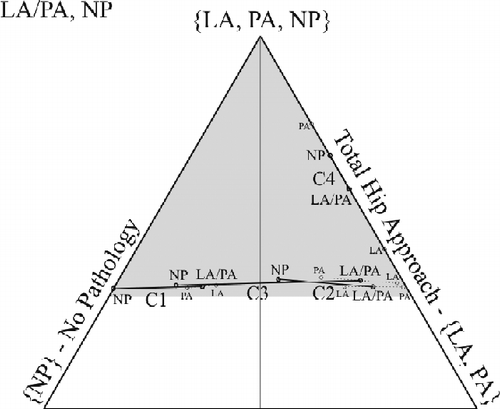
In Figure , each simplex coordinate labelled ‘LA/PA’ and ‘NP’ represents the average characteristic BOEs associated with the subject-based characteristics C1,…,C4 (labelling the line joining them), from subjects with LAs or PAs to surgery and NP, respectively.
There are two positional issues to consider when viewing the results in Figure (and considered in conjunction with each other):
1. | Vertical distance from the {LA, PA, NP} vertex: the further the distance away (down) from the {LA, PA, NP} vertex the average characteristic BOE is, the less ignorance there is associated with the evidence from that individual subject-based characteristic in the overall associations of subjects to {LA, PA} and {NP} (so more relevance). | ||||
2. | Horizontal distance between LAs/PAs to surgery and NP labelled simplex coordinates associated with a subject-based characteristic: the horizontal distance between the two points considers the level of ambiguity of the responses made between the groups of different subjects (more distance between them infers less ambiguity). |
Based on the position of the characteristic BOEs associated with the subject-based characteristics, there is a noticeable difference, in terms of contribution, between the C1 (sagittal plane hip rotation), C2 (frontal plane pelvic rotation), C3 (frontal plane hip power) subject-based characteristics and the C4 (frontal plane hip moment) subject-based characteristic. That is, based on the issues described earlier, the three subject-based characteristics, C1, C2 and C3, have less ignorance associated with them. The subject-based characteristic, C4, exhibits the most ignorance and ambiguity in its evidence.
Figure also displays the constituent average characteristic BOEs, namely based only on considering LA or PA-associated subjects. That is, from each of the points labelled LA/PA, there are dotted lines going off to two further points, separately labelled LA and PA, identifying the associated average characteristic BOEs of LA and PA subjects only, respectively.
Beyond the association accuracy (Figure ) and relative contribution (Figure ), more specific information on the contribution of the four subject-based characteristics, C1,…,C4, can be accrued using the CaRBS technique (from the configuration of a CaRBS model). A graphical elucidation can be made showing the specific level of evidential belief, contained in a characteristic BOE (m j,i (·)), a subject-based characteristic value offers to a subject's association to lateral or posterior approaches to surgery (m j,i ({LA, PA})) and NP (m j,i ({NP})) (and ignorance m j,i ({LA, PA, NP})), made by combining stages (a) and (b) in Figure in the Appendix, for each of the general characteristic BOEs (m j,C1…C4(·)), see Figure .
Figure 4 Contribution graphs for characteristic values in terms of their characteristic BOEs, for C1, C2, C3 and C4 (post configuration of CaRBS model, for LA, PA surgical types and NP).
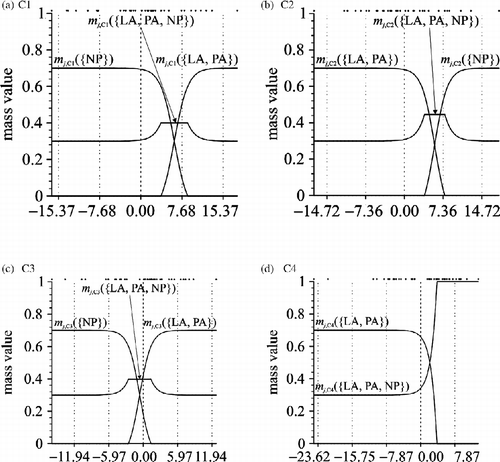
To summarise the contribution (refer to Table ) exhibited by the characteristic C1, there is a positive (negative) association of this characteristic with a subject having had hip replacement surgery (NP). That is, decreased hip flexion during early stance and late swing phase during gait is more indicative of THA than of NP, supporting the findings of Madsen et al. (Citation2004) and Whatling et al. (Citation2008).
Similar inference can be gained from the other three subject-based characteristics C2,…,C4, where the directions of contribution are: C2 – negative (positive) to surgery (NP), C3 – positive (negative) to surgery (NP) and C4 – negative (positive) to surgery (ignorance – surgery or NP). The inference for the subject-based characteristic C4 is different to that for the other subject-based characteristics, whereas the characteristic value increases over its domain: from the minimum near − 24.00 there is decrease in belief towards surgery (m j,C4({LA, PA})) and balancing increase in ignorance (m j,C4({LA, PA, NP})). However, from a value near 3.80 and above, there is only total ignorance in the evidence this subject-based characteristic offers (m j,C4({LA, PA, NP}) = 1.00).
In Figure , the four graphs give information on the contribution of the characteristic BOEs constructed (based on their respective four control variables k i , ϑ i , A i and B i found during the configuration of the CaRBS model, see Appendix. In each graph, the range of the respective subject-based characteristic (C1…C4) is shown over the minimum to maximum values present amongst the 43 subjects considered. Across the top of each graph are the small points showing the individual subject-based characteristic values taken by the 43 subjects. The vertical dashed lines shown depict the mean values of the characteristic, and other dotted vertical lines are successive values depicting the multiples of standard deviations away from the individual characteristic means. In each graph, up to three lines (nonlinear) are shown, depicting the values of mass values, m j,C1…C4({LA, PA}), m j,C1…C4({NP}) and m j,C1…C4({LA, PA, NP}), present in a characteristic BOE.
To further illuminate, the case of the subject-based characteristic, C1, is considered. As the C1 characteristic value increases from its identified minimum of − 15.37 to near − 2.00 there is constant belief m j,C1({NP}) in the association of a subject to {NP} with the remainder assigned to concomitant ignorance (mass value m j,C1({LA, PA, NP})).Footnote3 Beyond the characteristic value − 2.00, up to near 3.50, there is a decrease in the level of belief m j,C1({NP}), with a balancing increase in the level of concomitant ignorance m j,C1({LA, PA, NP}). Over the sub-domain 3.50 up to near 8.00, the level of concomitant ignorance remains constant, but there is a decrease in belief m j,C1({NP}), with an increasing belief in m j,C1({LA, PA}) – in the association of a subject to either LAs or PAs to surgery. Beyond 8.00, there is no belief in m j,C1({NP}) ( = 0), with an increase in m j,C1({LA, PA}) and balancing decrease in m j,C1({LA, PA, NP}).
With respect to the LR analysis also undertaken on the THA data-set, the contribution of the subject-based characteristic is measured through the LR coefficients, see Table (noting, as stated previously, the numerical-DV values DV = 1 and DV = 0 were assigned to the subjects known to be associated with having had surgery (using LA or PA) or NP, respectively).
Table 2. Subject-based characteristic contribution results from LR analysis (coefficients a1,…,a4 associated with subject-based characteristics C1,…,C4, respectively – making up the logistic expression Logit(DV) = a0+a1 × C1+a2 × C2+a3 × C3+a4 × C4).
From the LR analysis, the most significant factor associated with the post THA gait was the frontal plane power at the hip during stance (C3) which with a positive coefficient is shown to be positively associated with THA. This agrees with the CaRBS analysis. The other subject-based characteristics C1 (sagittal plane hip rotation), C2 (frontal plane pelvic rotation) and C4 (frontal plane hip moment) show the same directions of association to those found from the CaRBS analysis (compare with evidence in Figure and surrounding discussion). Similar to the CaRBS analysis, the salient measures in the frontal plane may be indicative of abductor muscle dysfunction and weakness in the surgical subject cohort (Baker and Bitounis Citation1989; Dijk et al. Citation2009).
4. Discussion
PCs were calculated to represent the salient regions of kinematic and kinetic waveforms in a discrete form while retaining temporal information. The first PC from each waveform accounts for the greatest variance, which was found to exist during hip flexion, pelvic obliquity during part of the stance and all of the swing phase, frontal plane power during parts of the stance phase and moment during the majority of the stance phase.
When examining the regions of the waveforms represented by the PCs used in the CaRBS model (Figure ), a greater difference is observed between the LA and NP waveforms compared to the PA and NP waveforms for C1, C3 and C4, where the LA subjects use a reduced sagittal plane hip ROM, reduced frontal plane power and moment. For C2, pelvic obliquity is similar for both surgical groups throughout the gait cycle, with a slightly greater difference observed in the swing phase of gait between the PA and NP cohorts, compared to the LA and NP cohorts. These findings add strength to the different levels of classification accuracy exhibited by the different LA and PA subject cohorts, as shown in Figure . This supports the findings of Whatling et al. (Citation2008), where the differences in hip function between PA and NP cohorts were less clearly defined as compared to between LA and NP cohorts. Madsen et al. (Citation2004) also found that subjects following the LA exhibited a gait pattern deviating from NP whereas several subjects following the PA exhibited NP gait patterns.
Inspection of the constituent average characteristic BOEs in Figure shows that for the subject-based characteristics, C1, C3 and C4, the LA points are nearer the {LA, PA} vertex than the respective PA points, indicating that these subject-based characteristics offered evidence that enabled the LA subjects to be more correctly discerned from the NP subjects than that for the PA subjects. For the case of the subject-based characteristic C2, with the PA point nearer the {LA, PA} vertex than the respective LA point, its evidence enabled the PA subjects to be more discerned from the NP subjects than the LA subjects were. Interestingly, for C4, the short distance between the LA/PA and NP average characteristic BOEs initially indicates a small range in the frontal plane hip power between the NP and LA/PA cohorts. However, when examining the average characteristic BOE for the LA and PA cohorts separately, there is a large variability in the frontal hip moment between the LA and PA cohorts. The average C4 characteristic BOE for the PA cohort is closer to the average NP BOE but has a high ignorance. The average characteristic BOE for the LA cohort is further from the average NP and has less ignorance.
From the CaRBS analysis, the contribution of the subject-based characteristics is measured through the respective characteristic BOEs, see Figure . The most significant factors associated with the post THA gait were the PC characteristics related to hip flexion (C1), pelvic obliquity (C2) and frontal plane power (C3). The salient measures in the frontal plane, i.e. reduced pelvic obliquity and frontal plane power and moments, may be indicative of abductor muscle dysfunction and weakness (Baker and Bitounis Citation1989; Dijk et al. Citation2009) in the surgical subject cohort (subjects associated with LA or PA). For frontal plane moments (C4), the level of ignorance reaches 1.00 for high values where no association can be made to either cohort and the majority of the contribution implies that as the frontal moment reduces the subject has undergone surgery. From Figure (b), it can be seen that during the swing phase of the gait cycle, the position of the pelvis was either level or falling below the horizontal position on the unsupported side for the THA sample, indicating abductor weakness and Trendelenburg gait (Hardcastle and Nade Citation1985). Fourteen subjects from the surgical subject cohort received the LA to surgery which may influence these variables since this surgical approach compromises the abductor mechanism. Figure (d) illustrates that patients following the LA approach to THA had reduced moment acting at the hip in the frontal plane compared to both PA and NP. The moment waveform for both LA and PA lacks the double trough identifiable in the NP frontal plane moment waveforms. During the two characteristic regions of the gait cycle in Figure (a) for C1, NP subjects use a greater hip flexion (and a greater ROM) than the surgical cohorts. A reduced sagittal plane hip ROM has been reported for the subjects that have undergone THA surgery by Madsen et al. (Citation2004) and Whatling et al. (Citation2008).
5. Conclusion
This study has demonstrated the use of the CaRBS technique employed in the analysis of hip replacement. It has been used to classify the subjects who have undergone THA surgery using LA or PA as compared to the subjects with NP. The use of PCs to determine subject-based characteristics and their subsequent CaRBS analysis have demonstrated that frontal plane power, pelvic obliquity and sagittal hip rotation are important in determining the changes in hip function associated with THA surgery. This was also true when using LR, although this approach does not allow a visual representation of the contributions of the characteristics.
The findings found that reduced hip flexion (C1), pelvic obliquity (C2), frontal plane hip power (C3) and frontal plane hip moment (C4) are indicative of subjects following THA. Examining the classification of LA and PA subjects separately revealed a greater range of functional ability of subjects following the PA to surgery, confirming the findings of Madsen et al. (Citation2004) and Whatling et al. (Citation2008). This study has shown the ability of the CaRBS technique to classify the data from the motion analysis and to visualise the contributions of each variable and how each contribution relates to the original biomechanical waveform. Thus, this technique provides a useful biomechanical tool for the analysis of complex data.
Acknowledgements
This work was supported by Arthritis Research UK [grant number 18461].
Additional information
Notes
3. The term remainder (or balancing – see later) is employed here since across a subject-based characteristic domain the sum of the mass values shown must equal one.
References
- Archer , KR , Castillo , RC , MacKenzie , EL and Bosse , MJ . 2006 . Gait symmetry and walking speed analysis following lower–extremity trauma . Phys Ther , 86 ( 12 ) : 1630 – 1640 .
- Baker , A and Bitounis , V . 1989 . Abductor function after total hip replacement: an electromyographic and clinical review . J Bone Joint Surg Br , 71 : 47 – 50 .
- Beynon , MJ . 2005a . A novel technique of object ranking and classification under ignorance: an application to the corporate failure risk problem . Eur J Operat Res , 167 : 493 – 517 .
- Beynon , MJ . 2005b . Optimizing object classification under ambiguity/ignorance: application to the credit rating problem . Int J Intell Syst Account Finance Manag , 13 : 113 – 130 .
- Beynon , MJ , Jones , L and Holt , CA . 2006 . Classification of osteoarthritic and normal knee function using three-dimensional motion analysis and the Dempster–Shafer theory of evidence . IEEE Trans Syst Man Cybern A Syst Hum , 36 ( 1 ) : 173 – 186 .
- Chang , A , Hurwitz , D , Dunlop , D , Song , J , Cahue , S , Hayes , K and Sharma , L . 2007 . The relationship between toe-out angle during gait and progression of medial tibiofemoral osteoarthritis . Ann Rheum Dis , 66 ( 10 ) : 1271 – 1275 .
- Chau , T . 2001 . A review of analytical techniques for gait data. Part 1: fuzzy, statistical and fractal methods . Gait Posture , 13 ( 1 ) : 49 – 66 .
- Dempster , AP . 1967 . Upper and lower probabilities induced by a multiple valued mapping . Ann Math Statist , 38 : 325 – 339 .
- Dijk , CM , Bimmel , R and Haddad , FS . 2009 . Surgical approaches in primary total hip arthroplasty – pros and cons . Orthopaedics Trauma , 23 ( 1 ) : 27 – 34 .
- Fan , H-Y and Lampinen , J . 2003 . A Trigonometric mutation operation to differential evolution . J Global Opt , 27 : 105 – 129 .
- Gore , D , Murray , M , Sepic , S and Gardner , G . 1982 . Anterolateral compared to posterior approach in total hip arthroplasty: differences in component positioning, hip strength and hip motion . Clin Orthop Relat Res , 165 : 180 – 187 .
- Hardcastle , P and Nade , S . 1985 . The significance of the trendelenburg test . J Bone Joint Surg Br , 67 ( 5 ) : 741 – 746 .
- Jones , L , Holt , CA and Beynon , MJ . 2008 . Reduction, classification and ranking of motion analysis data: an application to osteoarthritic and normal knee function . Comput Methods Biomech Biomed Engin , 11 ( 1 ) : 31 – 40 .
- Madsen , MS , Ritter , MA , Morris , HH , Meding , JB , Berend , ME , Farrs , PM and Vardoxis , VG . 2004 . The effect of total hip arthroplasty surgical approach on gait . J Orthop Res , 22 : 44 – 50 .
- McFarland , B and Osborne , G . 1954 . Approach to the hip: a suggested improvement on Kocher's method . J Bone Joint Surg Br , 36 : 364 – 367 .
- Moore , AT . 1959 . “ The self locking Vitallium prosthesis in femoral neck fractures: a new low posterior approach (the southern exposure) ” . In Instructional Course Lectures , Vol. 16 , 309 – 321 . St. Loius : The C.V. Mosby Co . The American Academy of Orthopaedic Surgeons
- Ritter , MA , Albohm , MJ , Keating , EM , Faris , PM and Meding , JB . 1995 . Comparative outcomes of total joint arthroplasty . J Arthroplasty , 10 ( 6 ) : 737 – 741 .
- Safranek , RJ , Gottschlich , S and Kak , AC . 1990 . Evidence accumulation using binary frames of discernment for verification vision . IEEE Trans. Robot Autom , 13 ( 4 ) : 405 – 417 .
- Shafer , GA . 1976 . Mathematical theory of evidence , Princeton : Princeton University Press .
- Whatling , GM , Dabke , HV , Holt , CA , Jones , L , Madete , J , Alderman , PM and Roberts , P . 2008 . Objective functional assessment of total hip arthroplasty following two common surgical approaches: the posterior and direct lateral approaches . Proc Inst Mech Eng H J Eng Med , 222 ( 6 ) : 897 – 905 .
Appendix
This appendix offers a description of the CaRBS technique (Beynon Citation2005a, Citation2005b; Beynon et al. Citation2006), employed in the analysis of the THA data-set (where appropriate the introduced terminology will be described using the language used in the analysis in the main text).
Formally, Dempster–Shafer theory is based on a finite set of p elements Θ = {s
1,s
2,…,s
p
}, collectively called a frame of discernment (Θ). A mass
value is a function m: 2Θ → [0, 1] such that m(∅) = 0(∅) – the empty set) and (2Θ – the power set of Θ). Any proper subset s of the frame of discernment Θ, for which m(s) is non-zero, is called a focal element and the concomitant mass value represents the exact belief in the proposition depicted by s. The notion of a proposition here being the collection of the hypotheses is represented by the elements in a focal element. The collection of mass values (and the focal elements) associated with a piece of evidence is called a BOE.
In the context of the CaRBS technique, it is concerned with the binary segmentation of objects (subjects o j , 1 ≤ j ≤ n 0) to a hypothesis x (surgery – LA, PA) and not-the-hypothesis ¬x (NP), and a level of concomitant ignorance, based on the subject-based characteristic values (v i , 1 ≤ i ≤ n V ). For a single subject (R j ) and a characteristic value (v i ) in CaRBS the associated evidence is formulated in a characteristic BOE, defined m j,i (·), and is made up of the mass values, m j,i ({x}) and m j,i ({¬x}), which denote the levels of exact belief in the association of the object to x and ¬x, and m j,i ({x, ¬x}) the level of concomitant ignorance. In the case of m j,i ({x, ¬x}), its association with the term ignorance is because this mass value is unable to be assigned specifically to either x or ¬x (an unknown distribution exists in the allocation of this mass value to x and ¬x).
From Safranek et al. (1990), and used in CaRBS, the described triplet of mass values in a characteristic BOE is given by the expressions (for a subject-based characteristic value v):
Figure A1 Graphical representation of an intermediate stage in CaRBS, for a characteristic value v to be transformed into a characteristic BOE and subsequent representation as a simplex coordinate in a simplex plot. Adapted from Beynon 2005a, p. 498, copyright (2004), with permission from Elsevier.
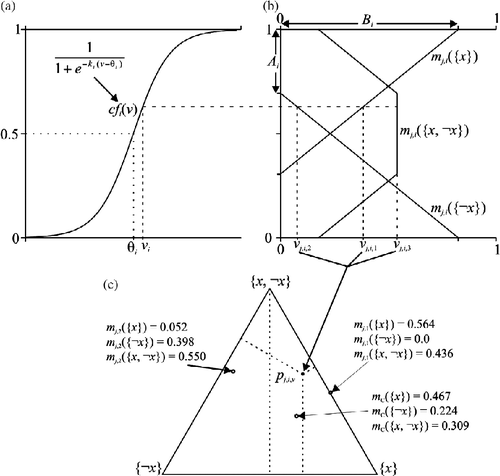
Figure (a),(b) shows the process by which a characteristic value v is re-scaled into a confidence value cf i (v) (over the domain 0 to 1), and then transformed into a BOE, here a characteristic BOE m j,i (·), made up of a triplet of mass values: m j,i ({x}), m j,i ({¬x}) and m j,i ({x, ¬x}). The characteristic BOE m j,i (·) is then able to be represented as a single simplex coordinate p j,i,v in a simplex plot (Figure (c)). That is, a point p j,i,v exists within an equilateral triangle such that the least distance from p j,i,v to each of the sides of the equilateral triangle is in the same proportions (ratios) to the values, v j,i,1 (m j,i ({x})), v j,i,2 (m j,i ({¬x})) and v j,i,3 (m j,i ({x, ¬x})).
The set of characteristic BOEs {m
j,i
(·), i = 1,…,n
0}, associated with an individual subject characteristic, can be combined using Dempster's combination rule into a subject BOE, denoted by m
j
(·). This combination rule, denoted by (on two BOEs
and
), is defined by
With the objects (subjects) known to be associated with either x or ¬x, a configured CaRBS model can be constructed, with respect to the intended optimisation of the required segmentation (to x or ¬x). The effectiveness of such a configured CaRBS model is governed by the values assigned to the incumbent control variables k i , θ i , A i and B i , i = 1,…,n P (see Figure ). This configuration process is defined as a constrained optimisation problem (using standardised response values), solved here using trigonometric differential evolution (TDE – Fan and Lampinen 2003), using an objective function (OB), which, from Beynon (Citation2005b), is defined by
To gauge the relevance (quality) of the individual survey questions in the optimum segmentation of objects (respondents), the average characteristic BOE is introduced. As its name suggests, this BOE is simply the average of the characteristic BOEs associated with an equivalence class of objects (E(x) or E(¬x) in this case). The general average characteristic BOE, associated with a characteristic v i and equivalence class of subjects E(R), defined am i,R (·), is given by