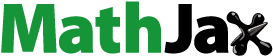
1. Introduction
Body segment inertia parameters (BSIP) are necessary parameters to perform dynamic analysis of human movement. However, as they cannot be directly measured in a non-invasive way, they are classically estimated using anthropometric tables (AT, e.g. Dumas et al. Citation2007). However, these tables are usually not adapted to atypical population (children, elderly, obese, individual with prostheses, etc.) that are classically of interest.
One option to estimate personalized BSIP consists in using identification techniques based on a dynamic model of the body and experimental data about a motion and external reaction forces. Previous studies showed the possibility to estimate “base parameters”, i.e. linear relation between segmental inertial parameters. Although using these base parameters allowed reconstructing the external forces (Hansen et al. Citation2014), they cannot be used directly to perform analysis at the joint levels (e.g. inverse dynamics). Nevertheless, these base parameters could be used to personalize the BSIP estimated from AT. Although promising, this idea has only been evaluated in two recent studies (Jovic et al. Citation2016 and Bonnet et al. Citation2016). However, these studies either presented results for masses only (Jovic et al. Citation2016), or used a complex procedure to guide long lasting (7 min) exciting motions (Bonnet et al. Citation2016). This study thus aims at evaluating the possibility to use identification techniques to evaluate segmental BSIP in a classical human movement analysis framework.
2. Methods
2.1. Experimental data
Nine subjects took part to this experiment: 3 males with a standard BMI (23.4 ± 1.2 kg/m²), and 3 males and 3 females with a larger BMI (33.6 ± 4.2 kg/m²). Subjects were equipped with 51 skin markers, whose 3D-trajectories were recorded at 100 Hz by an optoelectronic system (Vicon©). Ground reaction forces were collected at 1 kHz using a forceplate (Bertec©). Subjects performed several motions, including an “exciting motion”, i.e. 60 s of recording during which the subjects were instructed to move freely all of theirs segments. Joint angles were estimated using a multibody kinematics optimization approach. Velocities and accelerations were obtained by numerical differentiation and filtering (Butterworth recursive filters). Experiments were approved by the national ethical committee.
2.2. Biomechanical model
The human body was represented by a 17 segments rigid body model including feet, shanks, thighs, pelvis, trunk, head, clavicles (massless connecting rods), arms, forearms and hands. Dimensions were estimated from markers placed on anatomical landmarks. BSIP of segments () were estimated from anthropometric tables (Dumas et al. Citation2007).
2.3. Identification of base parameters
The unactuated part of the system’s dynamics can be written as (Ayusawa et al. Citation2013): , with: ϕ the vector of BSIP, i.e. 10 inertial parameters for each segment: Mass, 1st moments of mass (Mass time position of the CoM), moments and products of inertia;
the external forces and torques, expressed in the base (pelvis) reference frame;
the regressor matrix, function of the joint (
) and base (
) positions, velocities and accelerations. Its literal form of
was computed using the software Symoro+(Khalil & Creusot Citation1997).
Considering experimental values of ,
,
and their derivatives at each image, it led to:
As the matrix is not full rank, the system was further reduced to
, where
are linear combinations of ϕ. It was then solved using a Moore-Penrose pseudo inverse:
. Confidence in the identification of the base parameters was assessed using the relative standard deviations σ\ (Ayusawa et al. Citation2013). An estimate of the fitting’s quality is obtained by calculating
, the mean residual between experimental (
) and reconstructed forces (
). It can be compared to ɛTA, the mean residual between experimental (
) and reconstructed forces (
) using the BSIP from anthropometric tables (
).
2.4. From base parameter to segmental BSIP
Segmental values of BSIP (ϕ) were estimated by optimization: ϕ were as close as possible from the reference values obtained by anthropometric tables () while constrained by values of
. These constraints were passed as penalty and weighted according to the values of σ\. Only the most reliable relations (σ\<5%) were considered. Additional constraints ensured that masses remained positive. It ended-up in the following quadratic problem, solved using Matlab dedicated solver (quadprog):
In this study, no additional constraints nor bounds were used to ensure the coherence of BSIP values.
3. Results and discussion
Overall, the reconstructed forces were in correct agreement with the experimental values. Using identified base parameters led to a better fit (
), i.e. it improved the dynamics consistency. In most cases, and for both BMI groups, the identified segmental values of BSIP
were coherent when compared to the values from the anthropometric tables
, although a very simple exciting procedure used (simple instructions, short duration) compared to those previously proposed (Bonnet et al. Citation2016).
Figure 1. Locations of the segment’s CM along the longitudinal axis (Y axis of the ISB-local coordinate systems) obtained from both anthropometric tables and identification for a typical subject. Differences on other axes were below 5 mm.
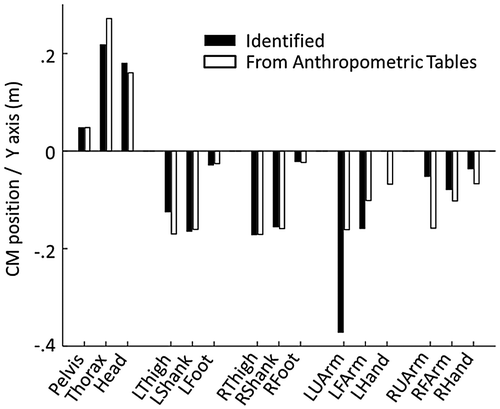
However, values of were very sensitive to quality of the fitting. Unrealistic BSIP were obtained from movements with larger values of
(see example in Table ). These errors were usually localized on few BSIPs. It typically happened when the frequency content varies much during the identification movements, where the use of Butterworth filters with constant cut-off frequencies led to inaccuracy in the velocity-accelerations estimates, i.e. incorrect regressor matrix
. This issue could be tackle by: (1) Adding bounding constraints on
in the optimization problem to prevent irrelevant values; (2) Using advanced filtering algorithms.
Table 1. Example of a correct vs irrelevant case.
A future step will be to perform an actual validation of these estimated BSIP. A promising idea (Bonnet et al. Citation2016) would be to evaluate if identification techniques could detect controlled changed in BSIP (e.g. adding a mass at a specific location) or BSIP of a known object (e.g. prosthesis).
4. Conclusions
This study provided encouraging results showing that relevant segmental BSIP can be obtained using identification techniques and a simple exciting motion procedure. Still, identified BSIP are very sensitive to the quality of the experimental data. Future tracks were identified to obtain 1/consistently coherent results and 2/actual validation.
Acknowledgements
Bilateral project JSPS-MAEDI & Sakura n°34192RL.
References
- Ayusawa K, Venture G, Nakamura Y. 2013. Identifiability and identification of inertial parameters using the underactuated base-link dynamics for legged multibody systems. Int J Robot Res. 33:446–468.
- Bonnet V, Fraisse P, Crosnier A, Gautier M, González A, Venture G. 2016. Optimal exciting dance for identifying inertial parameters of an anthropomorphic structure. IEEE Trans Robot. 32:823–836.10.1109/TRO.2016.2583062
- Dumas R, Chèze L, Verriest JP. 2007. Adjustments to McConville and Young et al. body segment inertial parameters. J Biomech. 40:543–553.10.1016/j.jbiomech.2006.02.013
- Hansen C, Venture G, Rezzoug N, Gorce P, Isableu B. 2014. An individual and dynamic body segment inertial parameter validation method using ground reaction forces. J Biomech. 47:1577–1581.10.1016/j.jbiomech.2014.03.004
- Jovic J, Escande A, Ayusawa K, Yoshida E, Kheddar A, Venture G. 2016. Humanoid and human inertia parameter identification using hierarchical optimization. IEEE Trans Robot. 32:726–735.10.1109/TRO.2016.2558190
- Khalil W, Creusot D. 1997. SYMORO+: a system for the symbolic modeling of robots. Robotica. 15:153–161.10.1017/S0263574797000180