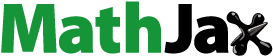
Keywords:
1. Introduction
The influence of footwear and orthopaedic treatments on the foot is usually measured through body segments kinematic analysis and/or plantar pressure.
To perform a more complete biomechanical analysis, an internal point of view containing the soft tissue deformation and the bone motion is used to study the internal behavior of the foot. In this kind of foot study, the precise motion of the bones is a convenient input for calculation of ligament and soft tissue deformation.
Foot motion capture is usually done through multiple techniques, either external (stereo-correlation, motion capture) or internal (weight bearing computed tomography (CT) scan, X-Ray fluoroscopy) (Morio et al. Citation2009; Colin et al. Citation2014; Ito et al. Citation2015).
As the 3D position of each bone is complex to obtain, even more so with shod foot and specific to the patient, calculating each bone position in the most reliable and automatic way is essential to perform internal patient-specific analyses. The objective of this work is to analyze the 3D kinematic of the foot internal structure during static loading cases to see the influence of the shoe on the bone positions. We present here a complete methodological framework based on CT scans to automatically segment and register the load bearing foot bones. The method is tested on 5 cadaveric feet.
2. Methods
2.1. Cadaveric experimentations
A specific horizontal compression bench that can be used inside the CT scanner was designed (pictures are available in supplementary data). It is composed of the frame, a load cell and a TekScan pressure map.
Five cadaveric feet underwent a series of loading configurations. Each foot is placed first in its neutral flat position; then two angular variations are considered: plantar/dorsal flexion, inversion/eversion.
In each configuration, the applied load and plantar pressure are continually recorded and CT scans are captured at specific loads. Three load magnitudes are considered (see ).
Table 1. Experimental conditions applied to bare and shod feet.
Experiments were carried out firstly on the naked feet, then wearing a sport shoe.
In total 24 scanners were done for each foot
Each scanner, done from the feet to the tibial plateau, lasted 28 seconds due to the choice to obtain a fine voxel resolution of 0.78 x 0.78 x 1.00 [mm].
2.2. Bones registration
The evaluation of each bone position requires the segmentation of the CT images. In the case of the foot, it means 30 bones. Different semi-manual procedures exist but reveal themselves time consuming due to the difficulty to treat “polluting” voxels connecting the different clusters of voxel associated with a bone, preventing the finding of distinct voxels groups corresponding to each bone.
In each case, i.e., for each foot, the first step consists in a classical semi-automatic segmentation of one CT scan images and the 3D reconstruction of the bones. Here, the chosen CT scan is the naked unloaded case.
All the other 3D images from the loaded CT scans are treated in an automatic way: a simple threshold isolates all the bones in a single skeleton mask. Then, the distance between each unloaded bone and the loaded skeleton is minimized using a variant of the Iterative Closest Point (ICP) algorithm based on the Sequential Least SQuares Programming (SLSQP).
The problem is formulated as the following nonlinear unconstrained least-squares problem:
Each point of the bone point set
points, corresponds to the closest point
of the skeleton point set.
With the eulerian angles rotation matrix expressed in function of the angle in each direction
and
the translationnal vector expressed in function of the translation in each direction
3. Results and discussion
Three of the five feet were processed. One was discarded because of its unusual stiffness, and another will not be commented here because of load mismatch between naked and shod situations.
The registration was successfully obtained, the mean minimal distance between the bone registered and the skeleton is in average equal to 0.28 mm, with a standard deviation of 0.09 mm.
Iterations of the first minimization, corresponding to the foot segment optimization done prior to each bone optimization, as the calcaneus last optimization are shown in supplementary data.
In the transverse plane, the articulations angles exhibiting a significant difference between shod and bare foot are the CalTavNav/CalTavCub as the CubM5PP5 (angles presented in supplementary data).
Because the shod foot acquisitions being made after all barefoot acquisitions completed, the stiffness of the cadaveric feet is potentially reduced due to the previous foot manipulations, which can facilitate the foot skeleton motion during the shod acquisition and can play a role in the articulation angles observed. Prior manipulations and repetition of the acquisitions can insure comparison between shod and bare foot without potential cadaveric foot stiffness evolution during manipulation. The same problem would not be encountered in vivo.
Observations are made on static cases that differ from moving cases, but still provide an interesting perspective of how the subject foot responds to a loading.
4. Conclusions
We realized experimentation with 5 cadaveric feet naked and shod, which permitted us to analyze footwear influences according to different foot positions with different loadings. To arrive to these results, we developed a semi-automatic segmentation technique by using an optimization method to quickly isolate each bone on all the 22 loading configurations scanned. This registration approach has been tested on cadaveric feet loaded in different static configurations and gave good results while accelerating the outcome obtaining.
Even though this technique was done on cadaveric feet, it can be used on in vivo feet with adapted test bed. Future work will take advantage of this present method to calculate ligaments deformations and soft tissue strain with Finite Element Model.
Additional information
Funding
References
- Colin F, Horn Lang T, Zwicky L, Hintermann B, Knupp M. 2014. Subtalar joint configuration on weightbearing CT scan. Foot Ankle Int. 35(10):1057–1062.
- Ito K, Hosoda K, Shimizu M, Ikemoto S, Kume S, Nagura T, Imanishi N, Aiso S, Jinzaki M, Ogihara N. 2015. Direct assessment of 3D foot bone kinematics using biplanar X-ray fluoroscopy and an automatic model registration method. J Foot Ankle Res. 8(1):21
- Morio C, Lake M. J, Gueguen N, Rao G, Baly L. 2009. The influence of footwear on foot motion during walking and running. J Biomech. 42(13):2081–2088.