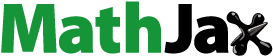
1. Introduction
Endovascular therapies have developed significantly in recent years (Wilbring et al. Citation2016). This rise is joined with increasingly sophisticated innovations (coils, balloons, or endoprosthesis for instance) which need to navigate through vascular structures. Catheters and flexible guidewires carry out the navigation. The major difficulty comes from vascular tortuosities, leading to a lack of control for the surgeons who handle such devices. Our global research project aims at developing a numerical tool dealing with the navigation of a specific active guidewire made of SMA (Shape Memory Alloy) into the aorta. For the sake of generalization, we aim to simulate a large cohort of patients, requiring reduced order modelling. The present paper deals with the active guidewire modelling associated with a non-intrusive a posteriori reduction model method named HOPGD for High Order Proper Generalized Decomposition (Lu et al. Citation2018).
2. Methods
2.1. Numerical model
On a first approach, we use a simple 2 D beam model to represent the active guidewire. As can be seen in , two wires (Ø 0.2 mm) are attached to an 8 cm length central beam (Ø 0.5 mm) via springs (stiffness ) and rigid links at the end. The behaviour of materials is linear elastic with stiffer wires (Young modulus
for the wires against
for the central beam). The mesh is made of a 1 mm Hughes-Liu beam elements discretization. We took into account the shape memory effect of SMA by applying a negative coefficient of thermal expansion to the wires. Thus, heating the wires causes them to shrink, allowing the beam to bend in a multi-curve way. One end of the central beam is fixed. Simulations are performed in Ls-Dyna (Livermore Software Technology Corporation (LSTC), CA., USA, 1976).
2.2. Model reduction by HOPGD
In order to cope with the parameter multiplicity and to prepare the navigation simulation into patient-specific vasculature models, we set up a model reduction procedure using HOPGD for the active guidewire. From precomputed snapshots obtained with the model described above, the HOPGD is used to seek separated representation of solutions. A relaxation method accelerates the global scheme to produce a multi-parametric computational vademecum. We decided to apprehend the HOPGD method by considering three parameters described in the chart ().
Table 1. Parameters and extrema values for the HOPGD method.
A dense grid with a coarse discretization of snapshots fed the HOPGD, each parameter taking its minimum, intermediate and maximum value alternatively. They correspond to the green dots in . In order to validate the reduced model, we consider eight sets of parameters that are at the centers of the eight sub-domains of the grid described by the sub-cubes in ; for each set of parameters, we compute the reference result in LS-DYNA and the corresponding result using HOPGD method. If we note
and
the matrix containing the reference and the reduced model results respectively, we compute the relative error with the matrix norm as follows:
3. Results and discussion
One of the precomputed snapshot is illustrated in . The elapsed time for this simulation is 31 minutes and 12 seconds using six Intel Core i7 2.60 GHz cores.
Using the HOPGD, the computed time for each set of parameters is about seconds (six Intel Core i7 2.60 GHz cores). With this method, we found a minimum error of 3% in the blue subdomain and a maximum error around 15% in the red subdomain depicted in , which is already rather satisfying given the very limited number of snapshots. However, obtaining a large error in a subdomain means the latter should be fed with a denser grid of snapshots to improve the interpolation between snapshots.
4. Conclusions
We obtained promising results for the HOPGD method in a simple 2 D beam case. Henceforth, our purpose is to use a more precise model for the active guidewire taking into account specificities inherent to SMA (Lagoudas Citation2008) and to develop a patient-specific endovascular navigation model. Then, these data will be considered to feed the HOPGD method in order to build an accurate numerical abacus.
Additional information
Funding
References
- Lagoudas DC. 2008. Shape memory alloys: modelling and engineering applications. College Station, TX: Texas A&M University.
- Lu Y, Blal N, Gravouil A. 2018. Adaptive sparse grid based HOPGD: towards a non‐intrusive strategy for constructing space‐time welding computational vademecum. Int J Numer Methods Eng. 114(13):1438–1461.
- Wilbring M, Rehm M, Ghazy T, Amler M, Matschke K, Kappert U. 2016. Aortic arch mapping by computed tomography for actual anatomic studies in times of emerging endovascular therapies. Ann Vasc Surg. 30:181–191.