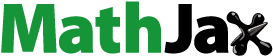
1. Introduction
Anthropometry databases have been collected in armies (US, Australian, Civilian: ANSUR I, II) for product design and sizing such as clothes, work environments and vehicles. However, anthropometry only does not furnish mechanical information to design systems. Their use is generally reduced to the sizing specifications of these systems. Using such databases to generate more relevant biomechanical specifications can be a real asset for prototyping. For example, defining the population-based articular range of motions or segment lengths can be relevant for the design of assistive devices such as exoskeletons, or for carrying devices such as bags. Indeed, anthropometrics can be used to scale segments lengths of biomechanical models as it has already been done from cadavers.
The current study aims at generating population-based full-body kinematical models of the French soldier, based on an anthropometric measurements database of the French army personnel.
2. Materials and methods
2.1. Anthropometric database
The scientifically sampled working data set ABCD from a French military personnel survey contains 1230 subjects, 459 females and 771 males respectively, belonging to the General Directorate of Armament of the French ministry of the army. In the database, the following information is stored for each subject: demographics (e.g. Age, sex, ethnicity) and 122 anthropometric measurements. These measurements were done on the torso, upper limbs, lower limbs, the head, hands and feet. For this study, a subset of 26 key measurements was selected for their low ratio standard deviation over mean (<0.1) and their potential for scaling. Indeed, these measurements are representative of the segments lengths and widths of the subject. These measurements included: height of right and left anterior superior iliac spine, height of right and left great trochanter, height of the middle patella, distance from great trochanter to lateral femoral condyle, height of popliteal fossa, distance from lateral femoral condyle to lateral malleolus, bitrochanteric distance, maximal foot length, height vertex sat on a seat, C7 height sat on a seat, acromial height sat on a seat, biacromial distance, pelvis width, anteroposterior thorax thickness, distance from elbow epicondyle to 3rd metacarpal head, distance from posterior plan to the tip of the hand, distance from acromion to epicondyle, distance from epicondyle to radial styloid, functional upper limb reachable height, height, C7 height, acromion height, manubrium height and completed by the mass of the subject. These measurements were done in three joint configurations: standing position arms up, standing position arms down, sitting position with straight horizontal arms.
2.2. Dimension reduction and clustering
Data was standardized for principal component analysis (PCA). The purpose was to identify the main features responsible for anthropometric variability in the population. Kaiser criterion was applied to select the number of principal components (PCs).
A k-means clustering approach was applied to the selected PCs with the Euclidian distance (Ding and He Citation2004) with a maximum of 10000 iterations and with 1000 new initial cluster centroid positions to ensure convergence to the global optimum. The number of clusters was chosen with a subjective interpretation of the mean of the silhouettes to assess the clustering quality (Kaufman and Rousseeuw Citation1990). Measurements of the centroids of clusters were used to generate canonical kinematical models.
2.3. Biomechanical model scaling
A biomechanical model with 18 rigid segments (pelvis, lower trunk, upper trunk, head, clavicles, arms, forearms, hands, thighs, shanks and feet) was used. They were linked by 17 joints equivalent to 41 degrees of freedom (3 for the pelvis/lowertrunk joint, 3 for the lower trunk/upper trunk joint, 3 for the neck, 3 for each the upper trunk/clavicles joint, 3 for each shoulder, 2 for each elbow, 2 for each wrist, 3 for each hip, 1 for each knee and 2 for each ankle). A total of 23 anatomical landmarks (including the ground) were placed in the segment-fixed frames of the model according to the sites of measurements of the database.
Each segment length of this model was initialised by applying an homothetic coefficient which is the ratio of the subjects' height over the height of the generic model. Generic values, i.e. segment lengths and anatomical landmarks were ‘isometrically scaled’ ensuring a good initial guess. Then, an optimisation scheme was applied to scale the model with the anthropometric measurements
corresponding to each selected centroid. Each
correspond to one of the 25 measurements. The modelled distances
between segment-fixed anatomical landmarks were written as symbolic distance functions of segment homothetic coefficients vector
and joint angle vector
The joint angle vector could take three possible values referring to three joint configurations. A joint configuration
was assigned to each of the 25 measurements
Adapted from (Puchaud et al. Citation2019), it yielded to the following minimisation problem, optimising segment homothetic coefficients using interior-point method:
(1)
(1)
Where and
are the centroids and modelled distances,
is the number of measurements,
is the number of rigid segments. The optimisation is stopped when the variation between two iterations is less than 0.1%.
3. Results and discussion
According to the Kaiser criterion, three PCs accounted for 85.3% of the variability in the data. Modes of variation was observed in the PCs. The 1st component was mostly correlated to vertical height measurements and mass. The 2nd component was correlated to the ‘widths’ such as bitrochantic or pelvis width, anteroposterior chest diameter and mass. The 3rd component was correlated to three sitting measurements and femoral condyle to external malleolus distance. For ranging from 2 to 3, the clusters had a reasonable structure. The means of the silhouettes were between 0.51 and 0.70. Above three clusters, at least one of the clusters presented a weak structure, i.e. the means of the silhouettes were below 0.51. The number of cluster chosen for this study was
For more details on centroids see . Optimisations reduced the root mean square errors (RMSE) between modelled distances and centroids’ distances. For each clusters from 1 to 3, the initial RMSE were 47 mm, 45 mm and 43 mm. After optimisation, the RMSE were respectively 23 mm, 24 mm and 25 mm.
Table 1. Number of subjects per clusters and centroids values for a few measurements.
In this paper a subset of ABCD database of the French army has been presented with a numerical approach to propose population-based canonical models using, PCA and k-means clustering and an optimisation-based scaling method. This method allows to consider for 2 D measurements to scale 3D full-body kinematical models. The use of the mass in the PCA was relevant because height and width measurements have been found to be correlated to the mass, in the 1st and 2nd PCs. In the present study, the choice of rely on a subjective criterion, however in virtual prototyping this criterion could have been relied on an economic criterion for design. The redundancy of the 2D anthropometric measurements has been used in the optimisation-based scaling to scale the 3D, full-body kinematical models, being useful to avoid the use of 3D complicated measurements. Finally, these generated models could be useful to reduce the number of representative models in contrast to (Miehling Citation2019), for product design such as vehicles, exoskeletons or backpacks. Our method may be completed with maximal torque databases to specify the biomechanical properties of our canonical models, but these databases take time to be collected with dynamometers. As a perspective, these models could be used with statistical motion models or motion synthesis for product design.
Disclosure statement
No potential conflict of interest was reported by the authors.
References
- Ding C, He X. 2004. K-means clustering via principal component analysis. In: Proceedings, Twenty-First Int. Conf. Mach. Learn (ICML 2004); p. 225–232. Available from: https://icml.cc/Conferences/2004/proceedings/papers/262.pdf.
- Kaufman L, Rousseeuw PJ. 1990. Partitioning Around Medoids (Program PAM). In: Finding groups in data: an introduction to cluster analysis. New York: John Wiley & Sons, Ltd; p. 68–125
- Miehling J. 2019. Musculoskeletal modeling of user groups for virtual product and process development. Comput Methods Biomech Biomed Eng. 22(15):1209–1218.
- Puchaud P, Sauret C, Muller A, Bideau N, Dumont G, Pillet H, Pontonnier C. 2019. Accuracy and kinematics consistency of marker-based scaling approaches on a lower limb model: a comparative study with imagery data. Comput Methods Biomech Biomed Eng. 23(3):114–125.