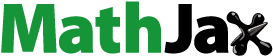
Abstract
Margin of stability (MOS) is one of the essential indices for evaluating dynamic stability. However, there are indications that MOS was affected by body height and its application in identifying factors on dynamic stability other than body height is restricted. An inverted pendulum model was used to simulate human walking and investigate the relevance between MOS and body height. Eventually, a height-independent index in dynamic stability assessment (named as Angled Margin of Stability, AMOS) was proposed. For testing, fifteen healthy young volunteers performed walking trials with normal arm swing, holding arms, and anti-normal arm swing. Kinematic parameters were recorded using a gait analysis system with a Microsoft Kinect V2.0 and instrumented walkway. Both simulation and test results show that MOS had a significant correlation with height during walking with normal arm swing, while AMOS had no such significant correlation. Walking with normal arm swing produced significantly larger AMOS than holding arms and anti-normal arm swing. However, no significant difference showed up in MOS between normal arm swing and holding arms. The results suggest that AMOS is not affected by body height and has the potential to identify the variations in dynamic stability caused by physiological factors other than body height.
Introduction
Falling poses a significant threat to human safety and leads to substantial pressure on the healthcare system (Yeoh et al. Citation2013). Fall risks are closely related to human dynamic stability (Toebes et al. Citation2012), which is affected by various factors such as age (Huang et al. Citation2008), neuromuscular diseases (Allum et al. Citation1994), and walking posture (Collins et al. Citation2009). For effective interventions to address fall risks, it is crucial to understand the influence of these factors on dynamic stability. For this purpose, various methods have been developed to quantify walking stability in a computationally and physiologically correct manner (Hurmuzlu and Basdogan Citation1994; Dingwell et al. Citation2000; Riva et al. Citation2013).
In early work, it was considered that as long as the vertical projection of the whole body center of mass (COM) is within the base of support (BOS), body balance or stability is maintained (Shumway-Cook and Woollacott Citation1995; Winter Citation1995). Later, it was noticed that besides the spatial location, the sway velocity of COM should also be accounted for in assessing body stability (Pai and Patton Citation1997; Iqbal and Pai Citation2000). Accordingly, the ‘position of the extrapolated center of mass’ (Xcom) and margin of stability (MOS) were declared (Hof et al. Citation2005). Xcom is derived from the position and velocity of the whole-body COM. MOS is defined as the minimum horizontal distance from Xcom position to the boundaries of BOS. Larger MOS is associated with higher stability (Hof et al. Citation2005; Buurke et al. Citation2019). Since the concept of MOS was put forward, it has been widely used in the quantitative evaluation of human dynamic stability (Young et al. Citation2012; Beltran et al. Citation2014; Siragy et al. Citation2020).
However, it is acknowledged that body height affects the swing amplitude of COM (Hegeman et al. Citation2007), step length (Samson et al. Citation2001) as well as step width (Donelan et al. Citation2001), and consequently the estimation of Xcom and BOS. It is reasonable to speculate that MOS is affected by body height and the height differences among individuals will increase inter-subject variability of MOS. And it will further lead to bias in studying the physiological or pathological factors other than body height on dynamic stability. For a reliable assessment of dynamic stability, the influence of body height should be controlled. The literature showed that for different gait parameters, we need to adopt different methods to remove the influence of body size, such as scaling stride lengths by dividing body height (Simon et al. Citation2017), scaling walking velocity by dividing L1/2, L is leg length (Hof Citation1996; Carty and Bennett Citation2009). The specific method needs to be determined by the relationship between body size and gait parameters. However, there is currently a lack of quantitative research on the effect of body height on MOS, thereby methods to exclude the effect of body height.
In order to test the influence of body height on MOS, and thereby explore the possibility of eliminating such interference in the assessment of dynamic stability, an inverted pendulum model for body sway was applied, and eventually, a height-independent index in dynamic stability assessment (named as Angled Margin of Stability, AMOS) was proposed. Theoretically, the model was utilized to simulate normal walking activity and investigate the relevance between MOS, AMOS, and body height. Also, experiments under different walking postures were conducted to verify the findings.
Methods
Simulation model
A well-known inverted pendulum model (Winter Citation1995), which considers the relationship between the trajectories of the center of pressure (COP) and COM, was used to simulate human gait. By the model, the effect of body height on COM and foot placement was investigated, and finally, the relationships between MOS, AMOS, and body height were quantified. As shown in , the body is modeled as a single mass m supported by a massless leg with length PL which is put on the ground at COP, somewhere under the foot (Hof et al. Citation2007).
Figure 1. (A) Schematic diagram of the inverted pendulum model. PL is the pendulum length; m is whole body mass; com is the vertical projection of COM on the ground plane; COP is the position of center of pressure. (B) The trajectory of COM and the position of COP for inverted pendulum walking in AP direction. SL is step length; orange straight lines, right leg in ground contact; blue straight lines, left leg in ground contact. copy0 is the position where the right foot contact with the ground; comy0 and vy0 are initial COM position and velocity, respectively (C) In ML direction, SW is step width; COPxL and COPxR are the positions where the right and left foot contact with the ground, respectively; comx0 and vx0 are initial COM position and velocity, respectively. (D) Xcom is the projection of XCOM on the ground plane, h is the height of XCOM, BOSb is BOS boundary, s is the line from XCOM to BOSb, θ is the angle between s and h, F is the minimum external force that makes the body unstable.
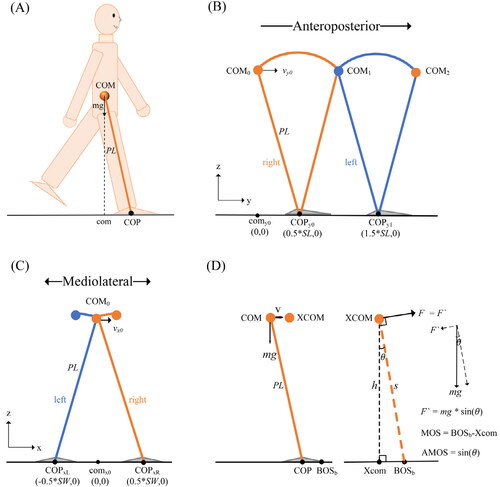
The motion equation of the inverted pendulum model is described as:
(1)
(1)
Where com is the vertical projection of COM on the ground plane; COP is the position of center of pressure; is the eigenfrequency of the inverted pendulum,
is the acceleration of gravity; PL is the pendulum length, approximately 0.575 times body height (Pai and Patton Citation1997). The simulation was designed on idealized settings:
The pivot point is ankle joint center of the supporting leg (Kagawa et al. Citation2007).
COP keeps constant until the next stride (McGrath et al. Citation2015).
The projection of ankle joint center is regarded as COP, ignoring the height of ankle joint center.
The double-support time is set as zero (Hof Citation2008).
The solution of Equationequation (1)(1)
(1) in a single step gait time can be described as:
(2)
(2)
(3)
(3)
The hyperbolic sine and cosine functions:
T is step time; v(t) is COM velocity on the ground plane at time t; COP0, com0, and v0 are COP position, COM position, and velocity at the initial time, which is defined as the moment when the right foot contacts ground. We need to determine these initial values to fully describe com(t) and v(t). In AP direction, comy0 is the initial COM position as the origin of coordinate, COPy0 is the initial COP position; according to the periodicity and symmetry of normal gait (Ankarali et al. Citation2015), we can get COPy0 = SL/2, SL is stride length; because COM velocity at the initial time and end time of single-step are equal, we can get the initial COM velocity
Finally, we can get the trajectory of COM and COP for normal gait in AP direction (see ).
The motion equations above can also be applied in mediolateral (ML) direction by setting corresponding initial values of the equations. The initial COM position comx0 is the origin of coordinate, the initial COP position COPx0 = SW/2; SW is step width; the initial COM velocity Finally, the trajectory of COM and COP in ML direction (see ) can be obtained.
As shown, based on the whole-body center of mass position (COM) and horizontal velocity (v), the extrapolated center of mass (XCOM) can be introduced:
(4)
(4)
The projection of XCOM on the ground plane is denoted as Xcom, MOS is defined as the distance from Xcom to BOS boundary (BOSb) which roughly equals the area of the foot placement (Hof et al. Citation2005).
(5)
(5)
As shown in , the simulated BOSb is related to the size of the foot and COP, where the projection of ankle joint center is regarded as COP. Assuming that in the AP direction the distance between the ankle joint to the upper edge of the foot is 0.81 times foot length (Winter Citation1990) and in the ML direction, the ankle joint center is at the middle of the foot.
Figure 2. Positional relationship between COP and BOS boundary (BOSb) in foot model. COP is the projection of ankle joint center. (A) In ML direction, BOSb1 and BOSb2 are the left and right edges of the foot, respectively. (B) In AP direction, BOSb1 and BOSb2 are the lower and upper edges of the foot, respectively.
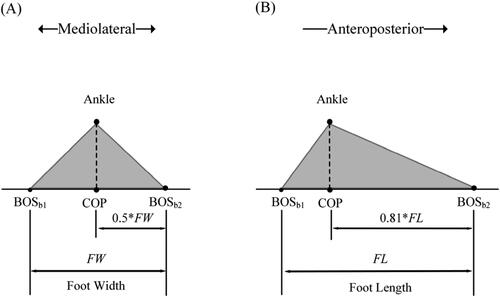
So, the simulated BOSb in AP direction can be estimated as:
(6)
(6)
And the simulated BOSb in ML direction can be estimated as:
(7)
(7)
Where FL is foot length, FW is foot width.
In AP direction, the mean value of MOS in step time T is given by:
(8)
(8)
Combine Equationequations (2) (3)(3)
(3) and the values of COPy0, comy0, and vy0, the mean MOS value in the AP direction can be simplified as:
(9)
(9)
Similarly, we can get the mean value of MOS in the ML direction:
(10)
(10)
From the above derivation, we can see that the mean values of MOS are determined by pendulum length, step width, step length, step time, foot length and foot width. All these parameters are related to body height (H). The pendulum length is about 0.575*H (Winter Citation1990; Pai and Patton Citation1997), the step length (SL) can be estimated by 0.42*H during normal walking (Jasuja et al. Citation1997), the leg length (LL) is about 0.53*H (Drillis et al. Citation1964) and the preferred step width (SW) is 0.13*LL (Donelan et al. Citation2001), the preferred walking velocity (V) is (England and Granata Citation2007; Martin and Schmiedeler Citation2014), and the step time T = SL/V. The foot width (FW) and length (FL) of adults are estimated at 0.055*H and 0.152*H, respectively (Drillis et al. Citation1964). With these typical gait parameters, the effect of body height on MOS is quantified as:
(11)
(11)
This indicates that the mean value of MOS has a linear relationship with body height. Normalizing MOS with body height may be an alternative way to remove the influence of body height. However, it needs to be noted that this linear relationship is obtained with normal gait under ideal conditions. In practice, such as for abnormal gait, this linear relationship may be mostly invalid, and the normalization is not applicable. Therefore, it is crucial to propose a novel solution to exclude the influence of body height.
The principle of AMOS
Previous research suggested that the dimensionless numbers method can be used to decrease the inter-subject variability (Hof Citation1996; Pierrynowski and Galea Citation2001). For instance, the step length SL is not given in length units, meters or inches, but as a ratio SL’ = SL/L, where L can be some characteristic length like body height. The angle is already a dimensionless parameter. Evoked by this, we proposed a novel dynamic stability evaluation parameter, named Angled Margin of Stability (AMOS):
(12)
(12)
Where s is the distance from XCOM to BOS boundary, h is the height of XCOM, θ is XCOM and BOS boundary inclination angle. The mean value of AMOS in step time T can be obtained with the equation:
(13)
(13)
An illustrative description of AMOS is shown in . We can explain the physical meaning of AMOS from a mechanical point of view. As shown in , the body is in a stable state. If we want to break this stable state (making XCOM rotate around BOS boundary), we need to provide an external force. The minimum external force is:
(14)
(14)
EquationEquation (14)(14)
(14) shows that the minimum external force required to make the body unstable is proportional to AMOS. The larger AMOS, the greater the external force required, indicating that the system is more stable, which gives AMOS a reasonable physical explanation.
Participants
In addition to model simulation, we also performed walking trials. Fifteen healthy young adults (12 males and 3 females, aged 26.9 ± 3.4 years, weight 70 ± 13.1 kg, height 172.9 ± 6.0 cm, BMI 23.3 ± 3.3) participated in walking trials at normal pace speed. None of the participants had gait related injuries or disorders. Approval of this study was provided by the ethics committee at the Zhejiang Hospital. All participants were recruited from Zhejiang University, including students and technicians who gave informed consent and agreed to participate in the study.
Tests
All participants completed the trials with three different walking postures: normal arm swing (Normal), hold arms to the body (Hold), and anti-normal arm swing (Anti-normal); the latter two were abnormal walking postures, as illustrated in . All trials were completed by the home developed gait analysis system. It consists of a motion sensor of Microsoft Kinect V2.0, instrumented walkway, and gait analysis software which was custom developed using C# (MS Visual Studio 2019). The gait analysis software was used to integrate the joint data and the footprint data obtained by Kinect and the instrumented walkway, respectively. The experiment setup is shown in . The subject walked on the instrumented walkway about 3 meters long, and the Kinect was placed at the front of the subject, at a height of 0.9 meters and 1.5 meters from the proximal end of the walkway. The Kinect can obtain the position of 25 joints of human body with a frame rate of 30 fps (Muller et al. Citation2017). With the mass distribution of the human body (Paolo Citation1996), the position of COM is estimated (Yeung et al. Citation2014). The instrumented walkway was used to record the track of walking feet by arrays of pressure sensors under a matt, with a spatial resolution of 1 cm and a sampling rate of 30 fps. Accordingly, BOS was computed. The boundary of BOS is defined by the outermost point of footprint of the leading foot. MOS and AMOS are calculated by Equationequations (5)(5)
(5) and Equation(12)
(12)
(12) , respectively. For every subject, each walking posture trial was repeated five times to acquire averaged MOS and AMOS values. The averaged values were then applied for statistics.
Statistical analysis
Pearson correlation analysis was adopted to assess the relationship between body height and stability parameters (MOS and AMOS) during walking with three different postures both in AP and ML directions. The coefficient of variation (CV) was used to determine inter-subject variability. Considering MOS in ML direction is more suitable than that in AP direction for dynamic stability assessment (Bruijn et al. Citation2013) MOS and AMOS in ML direction were investigated to compare the walking stability between normal and abnormal postures. The paired t-test was used and a p-value below 0.05 was considered significant. The data processing and analysis were performed using MATLAB R2019a (MathWorks) and GraphPad Prism 7 (GraphPad Software).
Results
Correlation analysis of MOS and body height
The model simulation results show that MOS has a linear relationship with body height under normal gait condition (). The magnitude of MOS increases with body height both in AP and ML direction. When H was set as 172.9 cm (the average body height of the participants) in the model, the simulated MOS value in AP and ML direction was −18.8 cm and 9.5 cm, respectively.
Figure 5. The relationship between MOS, AMOS and body height during normal gait in model simulation. (A) MOS in AP direction decreases with body height. (B) AMOS in AP direction remains unchanged with body height. (C) MOS in ML direction increases with body height. (D) AMOS in ML direction remains unchanged with body height.
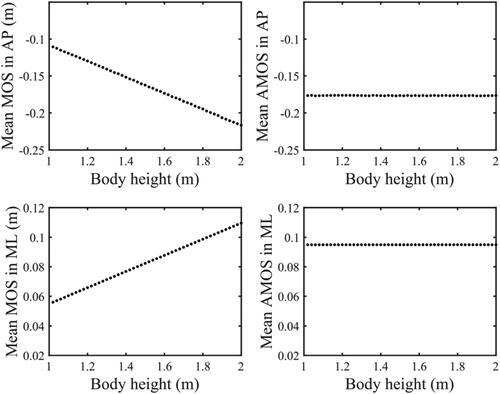
For walking tests, the measured data in normal arm swing condition also yielded a linear regression between MOS and body height as in AP direction, and
in ML direction. The averaged MOS in AP and ML direction was −17.1 cm and 9.8 cm, respectively. As shown in , in normal arm swing condition, MOS had a significant correlation with body height both in AP (r = -0.55, p < 0.05) and ML direction (r = 0.64, p < 0.05). Meanwhile, in hold arms condition, MOS had a significant correlation with body height only in ML direction (r = 0.57, p < 0.05). In anti-normal arm swing, there was no significant correlation between MOS and body height either in AP or in ML direction.
Table 1. Summary of the correlation analysis between MOS, AMOS, and body height in ML and AP directions under three different walking postures.
Walking stability in normal and abnormal postures
To reveal the influence of body height on the assessment of walking stability, the acquired parameters were compared between normal and abnormal postures in ML direction. As shown in , MOS and AMOS in normal walking condition were the highest, and they decreased constantly in hold arm and anti-normal condition. shows that MOS in normal arm swing (0.098 ± 0.024 m) was significantly larger (p < 0.05) than anti-normal arm swing (0.083 ± 0.025 m). There was no significant difference in MOS between normal arm swing and hold arms condition (0.094 ± 0.027 m). shows that AMOS in normal arm swing condition (0.108 ± 0.021) is significantly larger (p < 0.05) than hold arms condition (0.100 ± 0.026) and anti-normal arm swing condition (0.092 ± 0.027). Coefficient of variation (CV) was used to characterize inter-subject variability. As shown in , in normal arm swing condition and hold arms condition, the CV of AMOS is smaller than that of MOS both in AP and ML direction. In anti-normal arm swing condition, the CV of AMOS and MOS are very close in either AP or ML direction. For more details, the repeatability analysis for five repeated trials including intra-class correlation coefficients (ICC1,1) as well as mean and standard deviation are present in . The ICC1,1 values in ML and AP directions were all greater than 0.9 under three different walking postures.
Figure 6. The acquired parameters (A) MOS, (B) AMOS were compared and analyzed among normal and abnormal walking postures in ML direction. Normal: walking with normal arm swing; Hold: hold arms (no arm swing); Anti: walking with anti-normal arm swing; *: significant differences (p < 0.05).
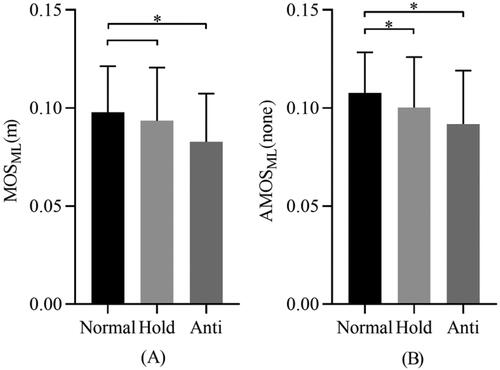
Table 2. Summary of the intra-group coefficient of variation in ML and AP directions under three different walking postures.
Table 3. Repeatability analysis over five repeated trials. Intra-class correlation coefficients (ICC1,1) and mean and standard deviation in ML and AP directions under three different walking postures.
Furthermore, the gait parameters, such as velocity, step length and width are given in . It shows that gait velocity and step length were affected by walking postures, compared with normal walking condition, holding arm resulted in significantly lower gait velocity while anti-normal produced longer step length.
Table 4. Summary of gait parameters under three different walking postures.
Discussion
The feasibility of Microsoft Kinect in estimating COM
Microsoft Kinect V2.0 is a kind of marker-less motion capture system which can track body joints displacements in real-time. Promising results by Microsoft Kinect with respect to gait analysis have been published (Geerse et al. Citation2015; Eltoukhy, Kuenze, et al. Citation2017; Eltoukhy, Oh, et al. Citation2017; Napoli et al. Citation2017). In this study, Microsoft Kinect V2.0 associated the instrumented walkway to obtain COM and BOS during walking, and in turn to estimate MOS and AMOS.
It was noticed that Kinect-based system had systematic errors and measurement noise (Otte et al. Citation2016). The measurement noise was mostly due to the interference of infrared in the test environment, in particular from the sunlight. Since our trials were conducted indoor, the influence of measurement noises on the Kinect application was largely avoided.
In the published work, the Kinect system produced highly correlated measurement of COM during standing (Ferraris et al. Citation2019) and forward walking (Danilov et al. Citation2016). Although the systemic errors of the Kinect system in the present study was not accurately assessed, the main results and conclusions were not influenced, as like the possible systemic errors did not hinder the wide application of the Kinect-based motion capture system in clinical practice. The work included the assessment of postural instability in Parkinson’s disease (Ferraris et al. Citation2019), investigation of human body locomotion dynamics (Danilov et al. Citation2016), classification of walking gait features in ASD children (Zakaria et al. Citation2019), prediction of short-term risk of falls in dementia groups (Mehdizadeh et al. Citation2021), and the gait analysis in the children with cerebral palsy (Ma et al. Citation2021), etc.
The validation of MOS and AMOS estimation by tests and the model
The estimated MOS from model simulation and experiments are consistent both in ML direction (9.5 cm vs. 9.8 cm) and AP direction (-18.8 cm vs. −17.1 cm) during normal walking. Furthermore, they highly agree with the reported study, i.e., about 7 to 12 cm in ML direction (Beltran et al. Citation2014; Mummolo et al. Citation2016; Magnani et al. Citation2020; Siragy et al. Citation2020; Mezher et al. Citation2021) and about −20 to −10 cm in AP direction (Magnani et al. Citation2020; Iwasaki et al. Citation2021). In addition, the estimated AMOS from model simulation and experiments coincided both in ML direction (0.095 vs. 0.108) and AP direction (-0.177. vs −0.182) during normal walking. This demonstrates that the model simulation, experimental design as well as the parameters calculation in the present work are reasonable and effective.
The influence of body height on MOS
The relationship between MOS and body height was verified both by model simulation and walking tests. Such relationship was consistent both in AP direction ( vs.
) and ML direction (
vs.
) during normal walking. These findings support the hypothesis that the estimation of MOS is affected by body height during normal walking. The influence of body height on MOS in the ML direction seems smaller than that in the AP direction. This is because the swaying of the human body in the ML direction during walking is modest in comparison with that in the AP direction. The correlation between MOS and body height shows positive in ML direction but negative in AP direction. This is due to the fact that in AP direction the walking velocity makes Xcom exceed COP and thus MOS value negative (Bruijn et al. Citation2013), while in ML direction Xcom lies in COP and MOS value keeps positive (Hof et al. Citation2007).
To eliminate the influence of body height on MOS, one initial solution might be the normalization of body height. However, the walking trials in abnormal conditions did not produce a clear correlation between body height and MOS. The rhythmic body movement and the posture control were altered during abnormal walking, and eventually, the movement of COM was interfered (Collins et al. Citation2009). Thereby, during abnormal walking the body motion does not comply with the inverted pendulum model constantly, and the linear relationship between MOS and body height is uncertain.
The experimental results show that in hold arms condition, the correlation between MOS and body height was significant in ML direction but not significant in the AP direction. In anti-normal arm swing condition, neither in the AP nor in the ML direction there shows a significant correlation between MOS and body height. These suggest that the coherence between body height and MOS does not present a unanimous relationship, but varies with walking posture. Thus, in practice, it is not reliable to normalize MOS with body height and for assessing dynamic stability a body height independent indicator is essential.
The availability of AMOS in walking stability assessment
The proposed assessment indicator AMOS has a definite meaning. It is proportional to the minimum external force required to make the human body unstable. In the simulation, the results show that AMOS is independent of body height. In agreement with the simulation, it was verified in the experiments that there was no significant correlation between AMOS and body height under walking postures either in the AP or in the ML direction. These findings indicate that AMOS can be used as an index independent of body height.
The walking trials with three different arm swing conditions further validated the ability of AMOS in walking stability assessment and verified its advantages over MOS. In the tests, walking with holding arm caused a decrease in MOS and AMOS, and anti-normal arm swing led to further decline. The reduction of MOS and AMOS indicate lower stability during abnormal walking (Hof et al. Citation2005; Buurke et al. Citation2019). It is consistent with the literature that walking with normal arm swing is more stable than abnormal postures (Ortega et al. Citation2008). Upper and lower limbs coordinated movement is crucial in maintaining stability during walking. However, in hold arm swing condition, the COM undergoes large lateral oscillations and further leads to small MOS, indicating the difficulty of walking in such posture (Shibukawa et al. Citation2001). In this case, more metabolically expensive mechanism is required in walking due to the reduced body stability (Ortega et al. Citation2008).
It is noticed that both MOS and AMOS exhibit a significant decrease in an extreme abnormal condition, like anti-normal arm swing. This can be interpreted by that while the upper and lower limbs move on the same side, the COM also shifts to this side of the body, resulting in the reduction of the distance between Xcom and BOS boundary and further the magnitude of MOS and AMOS. However, no significant difference shows between MOS in normal and holding arm walking posture. In contrast, AMOS with normal arm swing is significantly larger than that with holding arm as well as anti-normal arm swing. These results suggest that AMOS is capable to recognize the influence of typical abnormal walking postures, while MOS fails to distinguish the impact of posture on walking stability in some cases.
The analysis of inter-subject CV provided clues about the different performances of MOS and AMOS. The CV was often used to characterize inter-subject variability (Gidal et al. Citation2000). The statistics show that in normal walking gait, compared with AMOS, MOS produced larger CV in AP (0.173 vs. 0.138) and ML (0.242 vs. 0.194) direction, respectively. It hints that the difference in body height aggravates the inter-subject gait variability, which weakens the ability of MOS in the application. These results support that superior to MOS, AMOS is reliable to assess dynamic stability, owing to its independence on body height.
Limitations
It should be pointed out that there are a number of limitations in the present work. The model simulation was based on ideal settings and the experiments were only conducted on a normal group without gaiting deficits. In the further study, complex algorithms should be explored for the model to mimic abnormal gaiting, and the subjects diagnosed with body stability problems should be recruited in the experiments.
Conclusion
It is essential to assess the dynamic stability; however, the effect of body height reduces the ability of MOS to recognize the influence of physiological or pathological factors on dynamic stability. The present work proposed a new indicator AMOS for dynamic stability assessment. The simulations and experiment results demonstrate that AMOS is not affected by body height, and has the potential to provide a reliable measure for research and clinical application.
Statement of human studies
This study was reviewed and approved by the ethical review boards of Zhejiang Hospital (2021108 K).
Acknowledgment
The authors would like to thank Prof. Dr. Lili Liu from the Faculty of Applied Health Sciences, University of Waterloo for her helpful suggestions.
Disclosure statement
No potential conflict of interest was reported by the author(s).
Additional information
Funding
References
- Allum JH, Honegger F, Schicks H. 1994. The influence of a bilateral peripheral vestibular deficit on postural synergies. J Vestibular Res: Equilibr Orientation. 4(1):49–70.
- Ankarali MM, Sefati S, Madhav MS, Long A, Bastian AJ, Cowan NJ. 2015. Walking dynamics are symmetric (enough). J R Soc Interface. 12(108):20150209.
- Beltran EJ, Dingwell JB, Wilken JM. 2014. Margins of stability in young adults with traumatic transtibial amputation walking in destabilizing environments. J Biomech. 47(5):1138–1143.
- Bruijn SM, Meijer OG, Beek PJ, van Dieen JH. 2013. Assessing the stability of human locomotion: a review of current measures. J R Soc Interface. 10(83):20120999.
- Buurke TJW, Lamoth CJC, van der Woude LHV, Hof A, den Otter R. 2019. Bilateral temporal control determines mediolateral margins of stability in symmetric and asymmetric human walking. Sci Rep. 9(1):10.
- Carty CP, Bennett MB. 2009. The use of dimensionless scaling strategies in gait analysis. Hum Mov Sci. 28(2):218–225.
- Collins SH, Adamczyk PG, Kuo AD. 2009. Dynamic arm swinging in human walking. Proc R Soc B-Biol Sci. 276(1673):3679–3688.
- Danilov I, Gabbasov B, Afanasyev I, Magid E. 2016. Zmp trajectory from human body locomotion dynamics evaluated by kinect-based motion capture system. In: International Conference on Computer Vision Theory and Applications, SCITEPRESS.
- Dingwell JB, Cusumano JP, Sternad D, Cavanagh PR. 2000. Slower speeds in patients with diabetic neuropathy lead to improved local dynamic stability of continuous overground walking. J Biomech. 33(10):1269–1277.
- Donelan JM, Kram R, Kuo AD. 2001. Mechanical and metabolic determinants of the preferred step width in human walking. Proc R Soc B-Biol Sci. 268(1480):1985–1992.
- Drillis R, Contini R, Bluestein M. 1964. Body segment parameters. Artif Limbs. 8(1):44–66.
- Eltoukhy M, Kuenze C, Andersen MS, Oh J, Signorile J. 2017. Prediction of ground reaction forces for Parkinson's disease patients using a kinect-driven musculoskeletal gait analysis model. Med Eng Phys. 50:75–82.
- Eltoukhy M, Oh J, Kuenze C, Signorile J. 2017. Improved kinect-based spatiotemporal and kinematic treadmill gait assessment. Gait Posture. 51:77–83.
- England SA, Granata KP. 2007. The influence of gait speed on local dynamic stability of walking. Gait Posture. 25(2):172–178.
- Ferraris C, Nerino R, Chimienti A, Pettiti G, Cau N, Cimolin V, Azzaro C, Priano L, Mauro A. 2019. Feasibility of home-based automated assessment of postural instability and lower limb lmpairments in Parkinson's disease. Sensors. 19(5):1129.
- Geerse DJ, Coolen BH, Roerdink M. 2015. Kinematic validation of a multi-Kinect v2 instrumented 10-meter walkway for quantitative gait assessments. Plos One. 10(10):e0139913.
- Gidal BE, Radulovic LL, Kruger S, Rutecki P, Pitterle M, Bockbrader HN. 2000. Inter- and intra-subject variability in gabapentin absorption and absolute bioavailability. Epilepsy Res. 40(2–3):123–127.
- Hegeman J, Shapkova EY, Honegger F, Allum JHJ. 2007. Effect of age and height on trunk sway during stance and gait. J Vestibular Res. 17(2–3):75–87.
- Hof AL. 1996. Scaling gait data to body size. Gait Posture. 4(3):222–223.
- Hof AL. 2008. The 'extrapolated center of mass' concept suggests a simple control of balance in walking. Hum Mov Sci. 27(1):112–125.
- Hof AL, Gazendam MGJ, Sinke WE. 2005. The condition for dynamic stability. J Biomech. 38(1):1–8.
- Hof AL, van Bockel RM, Schoppen T, Postema K. 2007. Control of lateral balance in walking - Experimental findings in normal subjects and above-knee amputees. Gait Posture. 25(2):250–258.
- Huang SC, Lu TW, Chen HL, Wang TM, Chou LS. 2008. Age and height effects on the center of mass and center of pressure inclination angles during obstacle-crossing. Med Eng Phys. 30(8):968–975.
- Hurmuzlu Y, Basdogan C. 1994. On the measurement of dynamic stability of human locomotion. J Biomech Eng. 116(1):30–36.
- Iqbal K, Pai YC. 2000. Predicted region of stability for balance recovery: motion at the knee joint can improve termination of forward movement. J Biomech. 33(12):1619–1627.
- Iwasaki T, Okamoto S, Akiyama Y, Inagaki T, Yamada Y. 2021. Kinematic gait stability index highly correlated with the margin of stability: concept and interim report. In: 2021 IEEE/SICE International Symposium on System Integration (SII), IEEE.
- Jasuja OP, Harbhajan S, Anupama K. 1997. Estimation of stature from stride length while walking fast. Forensic Sci Int. 86(3):181–186.
- Kagawa T, Fukuda H, Uno Y. 2007. A kinematic and dynamic analysis on orthotic gait of paraplegics. Elect Eng Jpn. 161(3):10–21.
- Ma Y, Mithraratne K, Wilson N, Zhang Y, Wang X. 2021. Kinect v2-based gait analysis for children with cerebral palsy: validity and reliability of spatial margin of stability and spatiotemporal variables. Sensors. 21(6):2104.
- Magnani RM, Bruijn SM, van Dieën JH, Vieira MF. 2020. Head orientation and gait stability in young adults, dancers and older adults. Gait Posture. 80:68–73.
- Martin AE, Schmiedeler JP. 2014. Predicting human walking gaits with a simple planar model. J Biomech. 47(6):1416–1421.
- McGrath M, Howard D, Baker R. 2015. The strengths and weaknesses of inverted pendulum models of human walking. Gait Posture. 41(2):389–394.
- Mehdizadeh S, Sabo A, Ng K-D, Mansfield A, Flint AJ, Taati B, Iaboni A. 2021. Predicting short-term risk of falls in a high-risk group with dementia. J Am Med Dir Assoc. 22(3):689–695.e681.
- Mezher C, Siragy T, Nantel J. 2021. Increased arm swing and rocky surfaces reduces postural control in healthy young adults. Front Bioeng Biotechnol. 9:645581.
- Muller B, Ilg W, Giese MA, Ludolph N. 2017. Validation of enhanced kinect sensor based motion capturing for gait assessment. Plos One. 12(4):e0175813.
- Mummolo C, Park S, Mangialardi L, Kim JH. 2016. Computational evaluation of load carriage effects on gait balance stability. Comput Methods Biomech Biomed Eng Online. 19(11):1127–1136.
- Napoli A, Glass S, Ward C, Tucker C, Obeid I. 2017. Performance analysis of a generalized motion capture system using microsoft kinect 2.0. Biomed Signal Process Control. 38:265–280.
- Ortega JD, Fehlman LA, Farley CT. 2008. Effects of aging and arm swing on the metabolic cost of stability in human walking. J Biomech. 41(16):3303–3308.
- Otte K, Kayser B, Mansow-Model S, Verrel J, Paul F, Brandt AU, Schmitz-Hubsch T. 2016. Accuracy and reliability of the kinect version 2 for clinical measurement of motor function. Plos One. 11(11):e0166532.
- Pai YC, Patton J. 1997. Center of mass velocity-position predictions for balance control. J Biomech. 30(4):347–354.
- Paolo DL. 1996. Adjustments to Zatsiorsky-Seluyanov's segment inertia parameters. J Biomech. 29(9):1223–1230.
- Pierrynowski MR, Galea V. 2001. Enhancing the ability of gait analyses to differentiate between groups: scaling gait data to body size. Gait Posture. 13(3):193–201.
- Riva F, Bisi MC, Stagni R. 2013. Orbital stability analysis in biomechanics: a systematic review of a nonlinear technique to detect instability of motor tasks. Gait Posture. 37(1):1–11.
- Samson MM, Crowe A, de Vreede PL, Dessens JAG, Duursma SA, Verhaar HJJ. 2001. Differences in gait parameters at a preferred walking speed in healthy subjects due to age, height and body weight. Aging (Milano). 13(1):16–21.
- Shibukawa M, Sugitani K, Hong R, Kasamatsu K, Suzuki S, Ninomija SP. 2001. The relationship between arm movement and walking stability in bipedal walking. In: 2001 Conference Proceedings of the 23rd Annual International Conference of the IEEE Engineering in Medicine and Biology Society, IEEE.
- Shumway-Cook A, Woollacott MH. 1995. Motor control: Theory and practical applications. Baltimore: Williams & Wilkins.
- Simon AL, Lugade V, Bernhardt K, Larson AN, Kaufman K. 2017. Assessment of stability during gait in patients with spinal deformity-A preliminary analysis using the dynamic stability margin. Gait Posture. 55:37–42.
- Siragy T, Mezher C, Hill A, Nantel J. 2020. Active arm swing and asymmetric walking leads to increased variability in trunk kinematics in young adults. J Biomech. 99:109529.
- Toebes MJP, Hoozemans MJM, Furrer R, Dekker J, van Dieen JH. 2012. Local dynamic stability and variability of gait are associated with fall history in elderly subjects. Gait Posture. 36(3):527–531.
- Winter DA. 1990. Biomechanics and motor control of human movement. New York: Wiley.
- Winter DA. 1995. Human balance and posture control during standing and walking. Gait Posture. 3(4):193–214.
- Yeoh HT, Lockhart TE, Wu XF. 2013. Non-fatal occupational falls on the same level. Ergonomics. 56(2):153–165.
- Yeung LF, Cheng KC, Fong CH, Lee WCC, Tong KY. 2014. Evaluation of the Microsoft Kinect as a clinical assessment tool of body sway. Gait Posture. 40(4):532–538.
- Young PMM, Wilken JM, Dingwell JB. 2012. Dynamic margins of stability during human walking in destabilizing environments. J Biomech. 45(6):1053–1059.
- Zakaria NK, Md Tahir N, Jailani R. 2019. Classification of walking gait features using markerless-based approach in ASD children. Int J Electr Electron Syst Res. 15:28–34.