ABSTRACT
Artificial intelligence (AI) has breached creativity research. The advancements of creative AI systems dispute the common definitions of creativity that have traditionally focused on five elements: actor, process, outcome, domain, and space. Moreover, creative workers, such as scientists and artists, increasingly use AI in their creative processes, and the concept of co-creativity has emerged to describe blended human–AI creativity. These issues evoke the question of whether creativity requires redefinition in the era of AI. Currently, co-creativity is mostly studied within the framework of computer science in pre-organized laboratory settings. This study contributes from a human scientific perspective with 52 interviews of Finland-based computer scientists and new media artists who use AI in their work. The results suggest scientists and artists use similar elements to define creativity. However, the role of AI differs between the scientific and artistic creative processes. Scientists need AI to produce accurate and trustworthy outcomes, whereas artists use AI to explore and play. Unlike the scientists, some artists also considered their work with AI co-creative. We suggest that co-creativity can explain the contemporary creative processes in the era of AI and should be the focal point of future creativity research.
Introduction
Creativity is usually considered an interactive process where actors create novel outcomes as part of different domains in varying environments (Amabile, Citation1996; Boden, Citation2004; Csikszentmihalyi, Citation1997; Csikszentmihalyi & Sawyer, Citation2014; Fischer, Giaccardi, Eden, Sugimoto, & Ye, Citation2005). Many definitions combine some or all of the elements of an actor, process, outcome, domain, and space, which we call the five elements of creativity. However, creativity remains a disputed concept (e.g., Puryear & Lamb, Citation2020; Runco & Jaeger, Citation2012). Numerous definitions exist and evolve across academic debates. Some focus on humans, whereas others consider creativity to be distributed beyond humans into artifacts and the environment (Glaveanu & Kaufman, Citation2019) or rooted in systems formed of creative individuals, audiences, and domains (Csikszentmihalyi, Citation2014). Today, the surge of artificial intelligence (AI) across various creative occupations, such as science and arts, has brought a novel aspect into these debates, and scholars are increasingly scrutinizing the implications of AI in creativity (Daniele & Song, Citation2019; Fujita, Citation2018; Mazzone & Elgammal, Citation2019). We contribute to this research by studying computer scientists and new media artists who use AI in their work. In this paper, we aim to examine whether creativity requires redefinition in the era of AI.
We define AI as a computational system demonstrating behavior that would be considered intelligent when performed by humans – for example, learning, problem-solving, and reasoning (Kaplan & Haenlein, Citation2019; Russell & Norvig, Citation2010). The increasing use of AI raises several important conceptual, empirical, and real-life needs to revisit the definitions of creativity (Esling & Devis, Citation2020). AI has become more common in creative work because it performs well in tasks that are difficult for humans (Kaplan & Haenlein, Citation2019, p. 20), such as tirelessly analyzing and extracting big data, predicting outcomes, and even creating new content (Anantrasirichai & Bull, Citation2022). For instance, AI can imitate artistic styles (Elgammal, Liu, Elhoseiny, & Mazzone, Citation2017), compose music (Choi, Fazekas, & Sandler, Citation2016), or create dance moves in real time (Berman & James, Citation2018). Among the increased use of AI, these achievements have raised critical questions regarding AI’s potential to influence creativity, creation processes, and the audience’s experience of creativity (Daniele & Song, Citation2019; Miller, Citation2020).
Moreover, there is a need to bring forward a debate for including AI related creativity into the sphere of current creativity research (cf. Cropley, Citation2020; Garrido, Citation2021). Some AI methods are considered to be creative either on their own (Colton & Wiggins, Citation2012; Daniele & Song, Citation2019; Fujita, Citation2018) or together with a human. The latter is called co-creativity, where the creativity of humans and AI blends (Davis, Citation2013, p. 10). In this paper, we delve into research on co-creativity and reveal a surprising lack of empirical studies outside aesthetic art domains and for studies comparing multiple domains. Furthermore, co-creativity remains mostly unattended within social scientific creativity research and requires more focus on the everyday creative processes of people. However, co-creativity is almost exclusively studied in computer science and in prearranged settings (see Kantosalo & Riihiaho, Citation2019; Karimi, Rezwana, Siddiqui, Maher, & Dehbozorgi, Citation2020; Lin, Guo, Chen, Yao, & Ying, Citation2020). To develop creativity-supporting AI, we must understand how the novel AI technologies are used in everyday creative processes. People who work with AI are the experts in such technologies and in the role of AI in their own creativity. Revealing expert perspective requires empirical support that expands the concept of co-creativity research beyond computer science.
Thus, this study answers the need to revisit the definitions of creativity in the era of AI. To examine the role of AI in the everyday creation processes, we focus on computer science and new media arts that are both creative domains (cf. Florida, Citation2014) where people increasingly use AI in their work (Fujita, Citation2018; Jeon, Fiebrink, Edmonds, & Herath, Citation2019). As Miller (Citation2020, p. 2) notes, the field of AI ‘fuses’ science and art – here, computer science represents the domain where AI emerges from, and new media arts include some of the pioneers who apply AI in art. A known example is Harold Cohen’s painting tool AARON, one of the first projects to elicit dialogue between computer science and arts (Zylinska, Citation2020). At the same time, scientists and artists have distinct audiences and create different outputs: academic knowledge and artwork. Thus, we see that the two domains are imperative to understand creativity in the era of AI, as the scientists and artists can provide critical perspectives and interesting variety. The present paper examines whether creativity requires redefinition in the era of AI. We ask the following: (i) How do scientists and artists define creativity? (ii) Are the definitions of creativity different between scientists and artists? If yes, how? (iii) What is the role of AI in the creation process of scientists and artists? Our data consist of interviews with 52 Finland-based participants (26 computer scientists and 26 new media artists), which was assessed using a qualitative content analysis approach.
According to our key results, the scientists and artists recognize all five elements of creativity (i.e., actor, process, outcome, domain, and space) in their definitions. Scientists often define creativity via an outcome that, in their domain, should be valuable and novel knowledge. Artists focus on the creativity process with a personal perspective and often see that all outcomes have intrinsic value. When we examined the creative processes in the context of AI, we found that AI is approached differently between the two domains. For scientists, AI was a capable but limited tool, whereas the artists more often recognized a co-creative, playful relationship with it. The present study demonstrates how the understanding of creativity may change in the era of AI. We encourage the efforts to develop AI that augments human creativity rather than replaces it. Clarifying the concept of co-creativity is very necessary to research and better understand the limitations and potential of the human–AI relationship our near future.
This paper is structured as follows. First, we introduce relevant theoretical literature on creativity research through the five elements of creativity and focus on the creative contexts of science and arts. Second, we define AI, reflect on how it is implemented in creative work, and further elucidate the concept of co-creativity. Third, we present the data that we obtained from the interviews and analyzed with qualitative content analysis methodology. Subsequently, we explore the creativity definitions provided by computer scientists and new media artists, and further examine how they see the role of AI as part of their creativity process. Finally, we discuss the implications of this study along with those of previous research. The paper concludes with limitations and directions for future research.
What is creativity? Building the framework
Five elements of creativity
In general, many definitions of creativity incorporate aspects of the following five elements: actor, process, outcome, domain, and space, particularly the first three (Amabile, Citation1996; Boden, Citation2004; Csikszentmihalyi, Citation1997; Rhodes, Citation1961). visualizes the further analysis of each element, where we present them as “doings” (i.e., processes) or “beings” (i.e., actor and outcome) that all exist within space and time (). According to Runco and Jaeger (Citation2012, p. 92), the standard definition of creativity is twofold and includes the concepts of originality (or novelty) and effectiveness (or usefulness). These terms have appeared in several creativity definitions since the 1950s (Puryear & Lamb, Citation2020; Runco & Jaeger, Citation2012). We present some of the well-known definitions of creativity in . The same definitions are commonly applied in AI-related research as well (Esling & Devis, Citation2020; Jordanous, Citation2016).
Figure 1. A simplified conceptualization of the elements in the definitions of creativity. The creative actor is at the core of the figure. As a result of the creative process, they produce an outcome that is evaluated as creative (or not creative) in the domain. All elements exist in space and time.
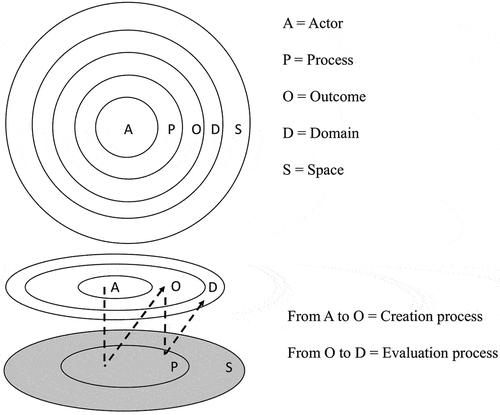
Table 1. Definitions of human creativity commonly applied in AI-related research.
The first element of creativity, actor, is most often considered a human with abilities to produce unique ideas and behavior (Glaveanu & Kaufman, Citation2019). The creative actor has been used to define creativity; for instance, Guilford (Citation1950, p. 444) described creativity as “the abilities that are most characteristic of creative people.” Research exploring “big C” concentrates on exceptionally creative geniuses, whereas “little c” research identifies the everyday creativity of a given individual (Simonton, Citation2013, p. 71; Stein, Citation1987, p. 420). However, the human-centered views of an actor have been challenged by the distributed and posthuman creativity theories that emphasize the interaction between humans and “nonhumans” – for example, artifacts and technologies. We argue that AI can also be considered an actor from these perspectives. From a distributed perspective, creativity is scattered beyond the (human) actor into artifacts and the environment (Glaveanu & Kaufman, Citation2019, pp. 15–21; Meusburger, Funke, & Wunder, Citation2009; Roudavski & McCormack, Citation2016). Similarly, from a posthuman perspective, knowledge and creativity are co-constituted with artifacts or technologies (Ihde, Citation1990; Latour, Citation2013; Rose, Citation2017). In his critical work, Ihde (Citation1990) argues that technologies mediate the human world. Here, humans experience the world with and through technology, which means that technology enables certain actions – for instance, AI enables the scientist to analyze large sets of data. We further apply Hayles’s theory (Citation2017, pp. 31–32) to demonstrate that AI is a cognizer (i.e., an actor that can autonomously pursue a goal). It differs from non-cognizer (i.e., a non-autonomous artifact such as a pen). Thus, researching AI from these perspectives is critical because it is a novel technology that can make decisions and change the process it participates in (cf. Mazzone & Elgammal, Citation2019).
The second element is the process of creation, where thoughts and actions are further developed to produce novel outcomes or ideas (Lubart, Citation2001). Creativity scholars have successfully identified several cognitive skills that are present in the creative process. One of the most critical is divergent thinking ability because it allows humans to imagine novel ideas and explore multiple solutions to solve a problem (Runco & Acar, Citation2012). Divergent thinking is followed by convergent thinking, where different thoughts come together in a structural manner, landing upon a single solution to a problem. Creativity is often conceptualized within the context of problem finding and problem-solving (Runco, Citation1994). Creative insight is considered to be the moment when a problem or solution is identified (e.g., Csikszentmihalyi & Sawyer, Citation2014). Today, AI can be used in tasks that require divergent and convergent thinking or problem finding and solving. Humans and AI can thus take distinct roles and complement each other in the co-creative process (Kantosalo & Toivonen, Citation2016). AI can, for instance, interactively inspire the human in a creative sketching task (Lin et al., Citation2020).
The third element is the creative outcome, which is the result of the creation process. Some outcomes are intangible (e.g., theories, skills) and others are tangible (e.g., paintings, songs, software). The definition of creative outcome as new, surprising, and valuable often traces back to Margaret Boden’s well-known work (Boden, Citation2004). Boden also distinguishes two types of creativity via the outcome; psychological (P-creativity) and historical (H-creativity) (Boden, Citation2004, p. 2). P-creativity occurs when an actor produces an outcome that is novel for themselves but not necessarily to the world. H-creativity represents a revolutionary outcome that is novel in the entire human history. However, evaluation of the originality and value of an outcome is an ongoing debate in creativity research. Creative AI further contests the issues regarding novelty, autonomy, and authorship, as AI’s creativity is often evaluated via the outcome it produces (Browne, Citation2022).
The fourth element of creativity is domain. Csikszentmihalyi (Citation2014) uses domain to represent a broad culture such as painting or logic. In this paper, we apply a more specific model by Baer and Kaufman (Citation2005), who explain domains in a three-step hierarchy: general thematic areas (e.g., arts), domains (e.g., visual arts), and microdomains (e.g., painting). However, the concept of domain remains disputed; for instance, scholars have long debated between domain-general and domain-specific views on creativity (Baer, Citation2010; Kaufman, Citation2012; Sternberg, Citation2009). In the domain-general perspective, creative individuals share similar abilities across all domains (e.g., Baer, Citation2010). Thus, certain skills required for creativity, such as personality, openness to new experiences, or willingness to take risks, could be domain-general (Kaufman, Citation2012; Sternberg, Citation2009). Others maintain that all humans are creative and that creativity can be further encouraged in everyone through creative teaching (Lucas, Citation2001). On the contrary, the domain-specific approach argues there are differences in creativity between domains, meaning that certain traits are prone to distinct domains (Baer, Citation2010; Sternberg, Citation2009). Here, creativity must be learned domain-specifically across various domains to foster creative skills (Baer, Citation2013). Similarly, AI is developed domain-specifically because it “must be trained using data having statistics and characteristics typical of the particular application domain under consideration” (Anantrasirichai & Bull, Citation2022, p. 593).
The fifth element is space. Various scholars have presented the connection of creativity and environment (e.g., Amabile, Conti, Coon, Lazenby, & Herron, Citation1996; Jordanous, Citation2016; Meusburger et al., Citation2009; Rhodes, Citation1961). Space is less common in the definitions of creativity than the other elements; however, we argue that creativity is inherently a spatial process (Hautala & Jauhiainen, Citation2014). The creative process includes the mobility of humans and artifacts (Hautala & Jauhiainen, Citation2019), and the process is distributed between the creator, environment, society, and space (Botella et al., Citation2013, p. 162). Space is not only a container or passive environment for creativity but a network of actors, artifacts, and environments that interact in the creation processes (Hautala & Jauhiainen, Citation2019; Jones, Citation2009, p. 491). Space in the era of AI is less restricted by physical mobilities and environments that can be overcome with digital tools and spaces. Recent research has also demonstrated that the opportunities for co-creativity between humans and AI-related technologies increase in situations with less control in spaces such as robot laboratories (Hautala & Jauhiainen, Citation2022).
Science and arts as the context of creativity
To research creativity in the era of AI, the present paper focuses on how computer scientists and new media artists view AI within the creative process. Both computer science and new media arts can be considered creative domains (cf. Florida, Citation2014), and an increasing number of scientists and artists are using AI in their work (Fujita, Citation2018; Jeon et al., Citation2019). The domains belong to the broader themes of science and art, where creative expression is required (Baer & Kaufman, Citation2005; Florida, Citation2014). Despite the fact that science and arts have sometimes been distinguished as two separate cultures (e.g., Snow, Citation1993), many contemporary scholars have noted that they developed codependently over time (Bullot, Seeley, & Davies, Citation2017). Science has influenced artistic innovations, whereas artistic culture has leveraged scientific discoveries. The history of both domains has celebrated many “creative geniuses,” from Pablo Picasso to Albert Einstein. Creativity can occur on the boundaries of these domains (Klausen, Citation2014), redefine such boundaries, or even produce new domains (Hautala & Ibert, Citation2018, p. 1689).
Scientists and artists have similarities in their creativity processes and as contexts of creativity (Root-Bernstein & Root-Bernstein, Citation2004). Both are found to have common, domain-general characteristics. For example, openness and intelligence support creativity in both domains (Batey & Furnham, Citation2006; Feist, Citation1998). To make certain outcomes possible, scientists and artists need to defy one’s own thinking, the audience, and the current status quo – or zeitgeist – of society (Sternberg, Citation2018a, p. 10). Workers in both domains need enough suitable resources, knowledge of methods, existing outcomes, and criteria of evaluation to produce creative outcomes. However, some studies have argued that creativity in science and arts is domain specific to some extent (Glaveanu et al., Citation2013; Lubart & Guignard, Citation2004). Agnoli, Corazza, and Runco (Citation2016) suggest that self-efficacy and the ability to solve problems by insight are predictors of scientific achievement, whereas personality and divergent-thinking abilities are predictors of artistic creativity. Ego strength (i.e., general emotional stability) often supports the creativity of artists but not of scientists (Kirsch, Lubart, & Houssemand, Citation2014).
In both science and arts, outcomes can be evaluated by their creativity. The recognition of peers, editors, funders, curators, and audiences is critical for a person to be considered a professional scientist or artist (Marnin-Distelfeld & Dorchin, Citation2020). Thus, creative achievement is constructed through social processes because it involves the interaction between actors in groups, domains, and wider networks (Csikszentmihalyi & Sawyer, Citation2014; Fischer et al., Citation2005; Mazzone & Elgammal, Citation2019). For example, in science, the creativity of a scientific article is often considered in the scientific peer-review process. In arts, the creativity of artworks is evaluated by both other artists and the audience.
However, the creative outcomes also present some of the key differences in creativity between science and arts. In science, the key outcomes are peer-reviewed publications that are evaluated in the domain (Simonton, Citation2004). The publications must meet the requirements of the domain; for instance, Sternberg (Citation2018b, p. 212) argues that academic journals and funding agencies often value incremental creativity (i.e., small steps that advance the field). Revolutionary discoveries are rare because they require the defiance of oneself, the state of the art in the domain, and the societal zeitgeist (Sternberg, Citation2018a). Arts, on the contrary, are more imaginative and connected to the impulse to create and express (Glaveanu et al., Citation2013). Evaluating the creativity of artistic outcomes (e.g., paintings, poems) appears to be more ambivalent as opposed to science because there are no set requirements for artistic creativity or value (Grant, Citation2018). Thus, artistic creativity relates to the idea of play. Play is a voluntary activity (e.g., Huizinga, Citation1938/1955/1955) that has special meaning and value (Lundman, Citation2012). Huizinga (Citation1938/1955/1955) describes how play happens in a “magic circle” that can break the rules of reality (i.e., what is impossible in real life is possible in play) (Lundman, Citation2012). Here, artists engage with their audience in the magic circle of make-believe when presenting their piece of art (Root-Bernstein, Citation2014). However, playfulness is not exclusive to arts; scientists can also participate in playful activities and emotions when they, for instance, imagine future research (e.g., Salen & Zimmerman, Citation2003).
Creativity with artificial intelligence
AI: simulating human intelligence in machines
Before we delve into creativity with AI, we must first understand what AI is. The first “intelligent machines” were designed nearly a century ago by mathematicians to simulate human thought (e.g., problem-solving, logic) (Mitchell, Citation2019, pp. 16–18). The term AI was coined in Dartmouth in 1956, when pioneer scientists were to develop a “fully intelligent machine.” However, intelligence has proven difficult to replicate because we do not fully understand what intelligence is or how to simulate it (Hawkins, Citation2021; Mitchell, Citation2019). Many agree that intelligence is a combination of different skills, such as information processing, motoric skills, and reasoning (Boden, Citation2016). The ability to learn is essential to obtain these skills (Hawkins, Citation2021, p. 134). AI can process information and learn, too; however, whether it can possess knowledge (Hautala, Citation2021; Hautala & Jauhiainen, Citation2022) or consciousness (Hawkins, Citation2021) is a question that remains unanswered. The intelligence of an AI has traditionally been measured with the Turing test: an AI passes the test if the human evaluator is unable to distinguish the AI from a human (Pease & Colton, Citation2011). AI can indeed outperform humans in certain tasks, such as analyzing massive amounts of data, and thus appears to be intelligent. However, a so-called general AI that combines various skills is yet to be developed.
Since its founding, AI has been divided into several subfields: machine learning, machine vision, and natural language processing (Russell & Norvig, Citation2010, p. 2). Today’s AI is relatively narrow in scope; it is applied in specific tasks such as industrial robotics or face recognition (Kaplan & Haenlein, Citation2019). The most important branch leading the current surge of AI is deep learning based on artificial neural networks (ANNs) (Boden, Citation2016, p. 6; Hawkins, Citation2021, p. 119). The ANNs mimic the network of the human brain, and some have argued they could be a step toward the general AI (Boden, Citation2016, p. 79). However, the current ANN models are simple compared to the neural networks of the human brain, and human-like intelligence remains purely speculative (Hawkins, Citation2021). The recent developments in ANNs, along with the availability of data, have nevertheless led to significant results, from programs that understand spoken language to self-driving cars. Importantly, ANNs have been critical in computer scientists’ attempts to model creativity, which has been a challenge for AI thus far (Toivonen & Gross, Citation2015).
From independently creative AI to co-creativity of humans with AI
Developing AI that performs creative tasks or augments the creativity of humans has intrigued scientists for decades (Colton & Wiggins, Citation2012). Computational creativity, a subfield of computer science aimed at developing creative AI, has recently gained traction due to the advancement in neural networks and deep learning techniques. Computational creativity has two major perspectives that we distinguish as “independently creative AI” and “co-creative AI.”
The first perspective focuses on developing AI that simulates human-level creativity (Colton & Wiggins, Citation2012). For instance, machine learning techniques can be used to classify neural markers of divergent thinking ability (Stevens & Zabelina, Citation2020) or problem-solving (Colin, Belpaeme, Cangelosi, & Hemion, Citation2016). Novel neural networks are used in systems that create, for example, paintings (Elgammal et al., Citation2017), sketches (Karimi et al., Citation2020), or compositions (Choi et al., Citation2016). Sometimes, AI is considered an artist, such as Shimon the musician (Dvorsky, Citation2017) or the painter software The Painting Fool (Colton, Citation2012). The creativity of AI is usually evaluated via an outcome (Ritchie, Citation2007, p. 69); a known method is the Lovelace test (Bringsjord, Bello, & Ferrucci, Citation2001). However, such tests fail to consider the originality and effectiveness of the outcome (Gioti, Citation2020, p. 29; Pease & Colton, Citation2011). Moreover, some research has suggested that human evaluators tend to be negatively biased toward art generated by AI, as opposed to art made by humans (Ragot, Martin, & Cojean, Citation2020). People might lack understanding of AI, or creativity might be perceived as a uniquely human trait. The idea of independently creative AI means a “competitive relationship” between humans and AI and evokes questions as to whether AI could replace humans as a creative actor (Gioti, Citation2020, p. 25).
The abilities of AI in creativity processes are very different from those of a human. Humans mobilize their skills into a versatile spatial creation process and, for example, respond to their culture, create meaning, move in different environments, engage in social interaction, build tools, or test new ideas. Although AI can simulate some of these skills, it cannot combine creative skills like humans can (Hertzmann, Citation2018, p. 19). In creative tasks, goals and inputs are also difficult to know beforehand, which is a problem for AI (Gioti, Citation2020, p. 30). Current AI lacks identity, feelings, the ability to give meaning to the outcomes it creates, or reflect “the lived experience of the human” (Mazzone & Elgammal, Citation2019, p. 8). As the programming requires a human actor, the concept of a fully autonomous AI is challenged (Daniele & Song, Citation2019). Thus, many oppose the idea of an “AI author” (e.g., Browne, Citation2022; Hertzmann, Citation2018; Hong & Curran, Citation2019).
The second perspective of computational creativity focuses on developing AI that is co-creative with humans. Human–AI co-creativity aims to blend the creativity of humans and AI in an interactive process “on a shared task in real time” (Karimi et al., Citation2020, p. 22). Such AI is capable of interacting with humans, learning, and adapting its functions in real time, and this interaction is also known as “human in the loop” (Chung, Citation2021). Thus, some consider it “an equal creative partner” to humans (Berman & James, Citation2018, p. 257) or a tool that can support the creativity of a human (Kantosalo & Toivonen, Citation2016). Research has shown that AI is capable of generating new ideas and inspiration for humans, providing knowledge that enhances humans’ creative abilities, overcoming fixated thinking and “blank canvas paralysis,” and inspiring individuals by presenting sketches of varying similarity (Kantosalo & Toivonen, Citation2016; Karimi et al., Citation2020; Maher, Citation2012).
Compared with the long history of creativity research, the human–AI co-creativity research is young, dominated by computer science, and empirically thin (Davis, Citation2013, p. 10). Most empirical research is focused on testing co-creative models in pre-organized settings within art-related domains, including design (Karimi et al., Citation2020; Lin et al., Citation2020), music (Gioti, Citation2020), writing (Kantosalo & Riihiaho, Citation2019), and dance (Berman & James, Citation2018; Fabiano et al., Citation2017). Moreover, creativity is often vaguely defined in the co-creativity research (). Sometimes, activity within any “creative domain,” such as dance, theater, or writing, is considered creative (Berman & James, Citation2018). When creativity is defined, it is often done via Boden’s (Citation2009) criteria of novelty, surprise, and value (Canaan, Menzel, Togelius, & Nealen, Citation2018; Gioti, Citation2020) or via combination, exploration, or transformation (Karimi et al., Citation2020). Also, Csikszentmihalyi’s (Citation1997) concept of flow, (i.e., state of complete concentration with the activity at hand) is applied. Here, AI helps humans reach the flow (Davis, Citation2013). Others define creativity as open-ended playful or improvisational interaction (Piplica, DeLeon, & Magerko, Citation2012) and searching (Kantosalo & Toivonen, Citation2016). Sometimes, all interactive action where humans and computers influence each other while doing a creative task is considered creativity (Fabiano et al., Citation2017, p. 1).
Table 2. Definitions of human-AI co-creativity.
In this study, we bring human-AI co-creativity to the center of creativity research. We interviewed computer scientists and new media artists who work with AI because they are the experts in such technologies and in the role of AI in their own creativity. In the following section, we introduce the materials and methods of this paper in more detail.
Materials and methods
Participants
To study creativity in the era of AI, we interviewed 52 Finland-based computer scientists (26) and new media artists (26) who created with or developed AI. The scientists consisted of doctoral students (eight), postdoctoral researchers or senior lecturers (seven), assistant or associate professors (eight), and professors (five). All scientists worked in universities and were part of research groups. Their work included research and, in some cases, teaching. The scientists represented the domains of computer science in which they worked – for example, machine learning, data science, data mining, or natural language processing. In these domains, they applied and developed varying AI techniques. We selected the participants by recognizing key AI-focused research groups from five universities in Finland. From these research groups, we aimed to select researchers at varying career stages, including group leaders (i.e., a professor or associate professor), postdoctoral researchers, and PhD students. We approached the scientists by e-mail, and those who were available and willing participated. Most researchers in these groups were men (n = 20), which is common in the computer science field (Sax et al., Citation2017).
Out of the 26 artists, 13 worked full-time as professional artists, whereas the rest had miscellaneous professions – for example, in artistic research, art education, or programming. Eighteen of them had formal art education. Their main domain can be deemed new media art, or arts that involve applying or developing novel mediating tools (Biggs, Citation2009). Some artists were also involved in other domains, such as the performing arts, music, or the visual arts, but they all had experience in new media art. The AI methods that the artists used include, for instance, machine vision, machine learning, and neural networks. Such methods are common in AI-based art (Elgammal et al., Citation2017; Gatys, Ecker, & Bethge, Citation2016). Participants who were at the intersection of science and the arts were classified as artists when they primarily identified themselves as such. The artists were selected via an online search, and more artists were included through a snowball technique (Tracy, Citation2019, p. 136). The artists comprehensively represented the AI-related artists of Finland, as the field is not very large. Only three artists were women, sharing similar demographics to those in computer science.
Data and methods
We collected the research data in the spring and summer of 2020. We used the semi-structured thematic interview method, where each interview has the same question pattern but the interviewer can ask additional questions during the interview if needed (Adams, Citation2015, p. 493). We chose this method because it accommodates an open discussion and supplemental questions. Due to the COVID-19 outbreak, all interviews but four were arranged via a videoconferencing tool. We understand that online interviewing brings about certain issues that must be considered (see, e.g., Lee & Hollister, Citation2020). To ensure the ethical integrity of the research, we provided an exhaustive information sheet regarding the research to the participants and acquired an informed consent form from each informant. All informants were ensured anonymity. The research plan received approval from the Ethics Committee for Human Sciences at the University of Turku.
Each interview was recorded and transcribed. The lengths of the interviews varied between 30 and 80 minutes, with the average interview being 50 minutes long. In the interviews, we asked the participants questions regarding AI and creativity, and we let them describe their personal creative processes. The informants were introduced to the topic of co-creativity and were asked to share their personal experiences, or in the absence of such experiences, they were asked to share examples of human–AI co-creativity that they were familiar with. We paid attention to how the informants described AI, particularly in relation to creative experiences and processes. We also focused our attention on the role that AI reportedly played in the participants’ work.
We examined the interviews via a content analysis, which is a common qualitative analysis method used to summarize and describe key results from large amounts of data (Krippendorff, Citation2018, pp. 24–27). To answer research question one (How do scientists and artists define creativity), we identified the key elements of creativity from the interviewees’ definitions of creativity. One definition usually included several elements. Each definition was coded (i.e., assigned a label that accurately described the definition); this approach is known as a conventional content analysis (Hsieh & Shannon, Citation2005, p. 1279). To answer research question two (Are the definitions of creativity different between artists and scientists? If yes, how), we compared the definitions to identify similarities and differences between the definitions of creativity that the scientists and artists provided. We then performed a similar analysis to answer research question three (What is the role of AI in the creation process of artists and scientists). Through this question, we discovered how the participants defined AI and described creation processes with AI. In the next section, we present the results of our study in greater detail.
Creativity from the perspectives of scientists and artists who work with AI
Creativity definitions
In the definitions of the interviewees, all five elements of creativity (i.e., actor, process, outcome, domain, and space) were present. The actor, process, and outcome elements were the most common elements, whereas domain and space were included less often. Most definitions included multiple elements ().
Table 3. Definitions of creativity provided by the scientists (S) and artists (A).
The actor was mostly referred to as human with such terms as “myself,” “we,” “human,” “artist,” and “user.” Most actors recognized that any human can be creative. More than a quarter (n = 14) of the participants specifically defined creativity as a human trait, or “what makes us human” (Artist 9). Artists particularly used themselves as examples of creative actors and emphasized a strong interest in making art as well as exploring new ideas (Glaveanu et al., Citation2013). A few scientists and artists called AI a creative actor.
Definitions of creativity as a process included mentions of specific abilities, such as the ability to invent or create new outcomes (n = 22), the ability to combine existing information (n = 14), the ability to find a solution or solve a problem (n = 13), and the ability to explore new ideas or concepts (n = 12). Similar concepts are widely recognized in research on creativity (e.g., Boden, Citation2004; Csikszentmihalyi & Sawyer, Citation2014; Runco, Citation1994; Runco & Acar, Citation2012). Thirteen interviewees defined creativity as “finding” or “searching” for possible new ideas, methods, or approaches, particularly at the beginning of a new research or art project. These are examples of the divergent thinking process (Runco, Citation1994; Runco & Acar, Citation2012). Some artists also included the environment and nonhuman objects, including AI, in the process.
The outcome element included examples of ideas, concepts, art pieces, or inventions. The most common outcome attribute was novelty. For some, an incremental step in everyday life (“little c”) was enough, whereas others described famous innovations or art pieces (“big C”) (Simonton, Citation2013, p. 71).
Things that perhaps already existed in the world but [that] had not [ever] been combined before. (Artist 19)
The act [of creating] something new. It might be tangible or intangible […]. You can create a new type of airplane, […] you can create a new piece of music. (Scientist 13)
Of all of the elements, domain and space were mentioned the least often in the definitions of creativity and appeared to be rather implicit. Creativity processes happen in relation to domain and space (Glaveanu et al., Citation2013; Lubart & Guignard, Citation2004). Moments of interaction with peers and the environment enhance creativity (e.g., Csikszentmihalyi & Sawyer, Citation2014; Fischer et al., Citation2005) and intertwine the process with space (Amabile et al., Citation1996; Hautala & Jauhiainen, Citation2014). For example, Artist 12 described creativity as one’s existence “in active space.” Interestingly, the scientists more often than the artists brought up domain through discussing the domain-specific evaluations of the outcomes. Some artists discussed domain from the perspective of social interaction and cooperation.
Creativity is […] coming up with something novel – that the community considers novel. (Scientist 11)
Creativity is born within the human community […], it emerges from the cooperation with others. (Artist 6)
As these definitions demonstrate, similar elements of creativity are valued across the domains of computer science and the new media arts (cf. Baer, Citation2010). Moreover, many participants recognized the affinities between the domains, particularly via AI. For example, Artist 23 noted that developing AI and machine learning models requires creativity and stated that “it is a big creative effort to transfer data into a form that a machine learning model can use.” Likewise, Scientist 17 explained that “creating a [computer] program or writing a paper is mostly artistic work.” However, the results also revealed certain differences that point to the domain specificity of creativity.
Differences in the creativity definitions: scientists and knowledge, artists and play
Scientists and artists have key differences in their definitions of creativity, realized through the elements of process and outcome. The differences are further connected to the idea of play in artists’ creativity process, whereas the scientific creativity process is aimed at producing outcomes approved as knowledge. The artists often defined creativity from their personal perspectives on the creation process, which they described based on feelings, practices, past experiences, and exploration. Artists’ creativity is a state of being: “you live in this flow where artistic work and thought is present all the time. It is a part of life” (Artist 12). Notably, many artists described this process as play and also used other play-related concepts, such as spontaneity, surprise, freedom, boredom, idleness, and boldness, to break the rules of reality (cf. Lundman, Citation2012).
[Creativity is] taking a playful approach [toward] everything, being curious, enthusiastic, and not afraid of failure. It’s courage to seize the moment and […] to try things that seem impossible at first. (Artist 3)
The sample included six artists who, in addition to artistic work, had experience with conducting science. They provided useful insights into the differences between creativity in science and the arts:
The scientific process is somewhat gradual because creativity must be verified. The methods [in the arts and science] are similar, meaning that you develop an interest in a phenomenon and begin to research it. The tools are, of course, different. (Artist 7)
The scientists described the creativity process as one in which the outcome – which must meet the demands of knowledge in the domain – is considered early on. Decisions and actions in the creative process must be justified, explained, and verified, and creativity must occur within the rules of the domain. For instance, Scientist 3 found it most creative when they were “thinking outside of the box and trying things no one else has done.” At the same time, they recognized that the results are “too different compared [with] the mainstream to get them published in the top journals.” For example, defying the zeitgeist is challenging (Sternberg, Citation2018a). In some cases, however, the rules can prompt a different approach to creativity:
Certain laws of computation and mathematics set the rules that cannot be broken, but in a way, there are infinite possibilities to utilize those rules. […] the process where we invent new things […] is undeniably creative thinking. (Scientist 20)
Outcome was mentioned in 72 summarized definitions of creativity (), and the scientists stated 60% of these. A total of 10 scientists (38% of all scientists) but no artists mentioned the definition of creativity as an “outcome that is useful and/or valuable” (). Novelty and value are very often used attributes in the creativity research (Boden, Citation2004; Puryear & Lamb, Citation2020), but in this sample, they existed mostly in the definitions of scientists. On the contrary, some artists challenged the idea that creativity is justified through the usefulness and value of creative acts or outcomes. For them, it is not common to measure creativity through external factors – for example, with benefit or merit (Grant, Citation2018). Instead, creativity is considered to have “intrinsic value” (Artist 22).
Roles of AI in creativity in science and arts
So far, the results have demonstrated how artists and scientists who create with AI define creativity. Next, we connect creativity with the role of AI and answer research question three, which concerns the role of AI in artists’ and scientists’ creation processes. In general, the scientists and artists described AI as a method, technology, and tool that learns or evolves (14 scientists, six artists). Opinions about the intelligence of AI were multifaceted. Many participants note that AI, or a certain function of AI, “is or at least appears intelligent” (Scientist 19), as it “imitates the intelligent behavior of humans” (Artist 6). Here, many interviewees differentiated between appearing intelligent and being “truly” intelligent, arguing that AI often fails the latter: “[AI has] no intelligence in a real sense […], it’s mimicking intelligent behavior” (Scientist 25). On that note, many participants speculated whether “general AI” is possible (Boden, Citation2016, pp. 21–22):
I don’t believe [independently creative AI] is going to be achievable, but we can always provoke, […] and that’s part of the fun of doing the artist’s work. (Artist 16)
The issue of intelligence relates to the question of whether AI can be creative. Twelve interviewees found the question of AI’s creativity to be difficult and answered it ambiguously. Twenty-four interviewees considered that AI can be creative, either by itself (n = 12) or (only) with a human (n = 12). Most agreed that the answer depends on how creativity is defined or what type of AI is used. For instance, if an audience considers an outcome generated via AI to be creative – for example, it passes the Lovelace test (Bringsjord et al., Citation2001) – it can be called creative. Some scientists had personally worked on AI that mimicked the human creativity process:
[I] prefer to think of what aspects of human creativity can be done by computers and then [think] about how to go about that. (Scientist 4)
However, the participants also pointed out how creative action is challenging for AI. Sixteen participants doubted or opposed the idea that AI can be creative. For instance, Scientist 2 explained that the self-evaluation of a machine is mostly superficial, and “the machine can, for example, evaluate the phonetics of a poem, but it cannot understand the meaning [of the poem].” Some participants noted how AI lacks crucial components for creativity, such as intention, motivation, self-evaluation, and the ability to interpret results or recognize discovery (see also, Davis, Citation2013; Hawkins, Citation2021; Mitchell, Citation2019). Here, the concept of consciousness comes into play – particularly the question of whether AI can exhibit it:
I think that the lack of [the] ability to recognize a discovery makes AI not creative. (Scientist 11)
If self-consciousness is related to creativity, then I don’t think AI can be creative. (Artist 8)
Thus, the role of humans is critical when one is analyzing and interpreting the AI-produced outcomes. As Scientist 22 described, “[AI] can only calculate what they have in terms of data, but humans can also think beyond the data.”
The limitations of independently creative AI direct the focus toward co-creative AI. The majority (65%) of those who answered the question of whether AI can be creative supported this notion, as many described the process of creation with AI from the perspective of co-creativity. Some emphasized the “human-in-the-loop” (Chung, Citation2021) relationship, where, for instance, “a human gives an example that an AI then follows and occasionally asks for the human’s opinion” (Scientist 5). Many participants who dismissed AI as independently creative agreed that it can support the creative process. For instance, Scientist 25 noted that if the creative process is understood as coming up and testing new ideas, “[AI] is essential for the testing.”
However, everyday work with co-creative AI divided opinions between the scientists and the artists. Many scientists emphasized that they did not use co-creative AI, and many contemplated whether such AI would be useful in science. Hence, most dismissed the idea of working with AI co-creatively: “for me, [the idea of creating things together with AI] sounds romanticized” (Scientist 20). Artists, most of whom use creative AI methods, were instead more open to the idea of working together with AI. Several of them recognized co-creativity in their own work. Take, for example, Artist 19, who explained that using AI “is co-creativity because you create things that cannot exist without that tool.” Moreover, as Artist 8 pointed out, AI does not have to be “intelligent” to participate in the process, and the artist can nevertheless “find new interesting possibilities [with AI].” This is in alignment with posthuman perspectives, when a creative outcome is “co-constructed” in a mutual interaction between the artist and AI (Ihde, Citation1990; Latour, Citation2013; Rose, Citation2017).
AI as a trusted or playful companion in science and arts
Scientists and artists have described various ways in which AI has enhanced their creative processes. For instance, AI has helped with exploring new possibilities, testing models, verifying hypotheses, creating new outcomes, or, in general, “making the lives of humans easier” (Artist 6). In a further analysis of the role of AI in co-creativity processes, an interesting difference between scientists and artists emerges. This difference is further linked to the differences between artists’ and scientists’ definitions of creativity – namely, science and knowledge (Simonton, Citation2004; Sternberg, Citation2018b), as well as the arts and play (Glaveanu et al., Citation2013; Lundman, Citation2012; Glaveanu et al., Citation2013). The core of co-creativity is the interaction between humans and AI (Davis, Citation2013), which we describe as a trusted companion in science and a playful companion in the arts.
For scientists, AI needs to be a “trusted companion” that works properly and does not make mistakes. Achieving this requires the careful development of AI, which Scientist 13 described as planting seeds in a pot and then watering and nurturing them – “the tool […] rises from the pot when it is ready.” Although most scientists in this sample did not recognize a co-creative relationship with AI in their own work, they mentioned several ways in which AI enhanced their work or made it easier: “we use machines and algorithms to analyze [data] because they do it very quickly and correctly, and there’s less [of a] chance of mistakes” (Scientist 22). With their trust in AI, they can verify hypotheses and create justified and explainable knowledge, although some found the idea of malfunctioning AI to be intriguing:
It would be entertaining to show the results we get when the models produce something silly […] to show all [of] the things the machine can do if it does not work. (Scientist 16)
For artists, AI can be a playful companion that can produce surprising and interesting results. What scientists would deem an AI error may be a source of a new idea for an artist. For instance, Artist 14 described their experience with errors during programming:
It’s a classic thing in coding […]. You had a certain goal you wanted to reach, but then you made a mistake, and you look at it and think, ‘Oh, this is much better than I had in mind. Let’s follow it. (Artist 14)
For some artists, the unexpected results embody the creativity of AI: “the digital errors or the little faulty parts are absolutely a part of [AI’s] creativity” (Artist 21). They deliberately aimed for unanticipated outcomes by testing systems with different settings and parameters. Here, AI enhances the artistic creative process by prompting out-of-the-box thinking and completely new ideas. Thus, the artists were more prone to recognizing co-creativity with AI in their work as opposed to the scientists. We explore these results further, along with the implications and limitations of this study, in the following discussion section.
Discussion and conclusions
Co-creativity with AI – the new focal point of creativity research
The present paper contributes to the need to revisit the definitions of creativity in the era of AI. Although creativity is a disputed concept, the definitions center on five elements – actor, process, outcome, domain, and space – across academic debates. Recently, scholars have scrutinized AI’s potential to influence creativity and creation processes (Daniele & Song, Citation2019; Miller, Citation2019). Whether we should understand creativity from a perspective that is human centered, AI centered, or in between (co-creativity) is a discussion we bring into the Creativity Research Journal. Whereas the creativity research is increasingly complemented by AI-related studies (Daniele & Song, Citation2019; Fujita, Citation2018; Mazzone & Elgammal, Citation2019), the concept of co-creativity is still rather unknown in the field. Co-creativity – for example, blending the creativity of humans and AI in an interactive process (Karimi et al., Citation2020, p. 22) – is mainly studied within computer science. Such studies mostly utilize pre-arranged empirical settings and rarely dispute the definitions of creativity (see Kantosalo & Riihiaho, Citation2019; Karimi et al., Citation2020; Lin et al., Citation2020).
Our pioneering empirical study focused on the use of AI in computer scientists’ and new media artists’ everyday creativity processes. Computer science and new media art are considered to be creative domains that have a long history together (Bullot et al., Citation2017), and they are at the forefront of developing and pioneering AI (Fujita, Citation2018; Jeon et al., Citation2019). However, the domains also have distinct features (Florida, Citation2014; Kaufman, Citation2012), particularly in terms of outcome and audience. Thus, scientists and artists offer interesting variety in their views in the context of creativity, and furthermore with AI. We present a comparative setting of the two domains, with data consisting of interviews with 52 Finland-based computer scientists and new media artists.
The definitions of creativity from the participants of this study adhered to the ones known in creativity research, as all five creativity elements (actor, process, outcome, domain and space) were present. The scientists and artists had similar descriptions regarding the actor and process elements. However, the outcome element revealed key differences between the two groups. Several scientists affiliated outcome with usefulness and value, whereas many artists opposed the idea of certain criteria for outcomes or creativity in general. The results highlight the different demands of the two domains. Scientists need to meet the strict rules of the domain to produce new knowledge (Sternberg, Citation2018a). Artists, on the contrary, can express themselves without requirements from the domain (Glaveanu et al., Citation2013; Grant, Citation2018). Next, we examine the results in the context of AI and co-creativity, as we present the three main contributions of this paper.
AI challenges the elements of creativity
As the first contribution, we show that the understanding of some creativity elements may change in the era of AI (see also, Daniele & Song, Citation2019; Miller, Citation2020). Whereas the creative actor is often synonymous to the human in creativity research, nearly half of the participants considered that AI can be creative, too. Here, views on the creative process and its outcome, as well as how AI matches the creative process and outcome criteria, influence the understanding of a “creative actor.” For instance, some participants noted that machines can simulate the creative process or parts of it, such as divergent thinking (Stevens & Zabelina, Citation2020) or problem-solving (Colin et al., Citation2016). Furthermore, many common definitions of creativity (Boden, Citation2004; Puryear & Lamb, Citation2020) focus on novel and valuable outcomes, which AI arguably can produce. Therefore, AI can be included within these definitions, and it challenges the understanding of human-centered creativity.
On the other hand, creative AI causes tension with the human-centered perceptions on creativity. The lack of certain human traits was a critical reason why one-third of the participants opposed the idea of creative AI. For instance, scientists and artists are needed to develop and program AI that cannot act independently, and AI’s lack of intention or motivation was also mentioned (cf. Mazzone & Elgammal, Citation2019). Moreover, although AI can “pass the Lovelace test” (Bringsjord et al., Citation2001) – for example, create outcomes that the audience deems creative – it cannot give meaning to such work. Thus, for many participants, acting consciously is an essential requirement that AI does not meet. This also indicates that, for some, creativity is inherently a human experience (Ragot et al., Citation2020).
Regarding domain, the results show how AI is used differently in two distinct domains, computer science and the new media arts. Computer scientists need precise AI models to create justified and trustworthy knowledge. Meanwhile, new media artists use AI and its mistakes playfully to create interesting and surprising artwork. However, we also support Miller’s (Citation2020) argument that AI fuses science and the arts. Some computer scientists considered developing AI to be artistic work, and many new media artists said they needed computer science-related skills to use AI. Moreover, both groups included people who had collaborated in multi-domain projects where scientists and artists worked together with AI. Such collaboration might break the boundaries of domain-specific requirements and consequently open doors for new ideas and innovation.
Co-creativity explains creativity in the era of AI
As the second contribution, we argue that the concept of co-creativity should be incorporated into the creativity research in the era of AI (e.g., Kantosalo & Toivonen, Citation2016). In the results, the artists recognized co-creativity in their work. Although the scientists were more opposed to calling the AI they used co-creative, they also identified various ways in which they used AI in their creative processes. The participants used AI to test data, verify hypotheses, explore solutions, and create new outcomes, among other tasks. Moreover, as AI cannot work intentionally or consciously, humans are required to interpret, develop, and create meaning for the outcomes that AI produces. In these occasions, AI mediates the human experience (Ihde, Citation1990), and human–AI interaction is realized “on a shared task in real time” (Karimi et al., Citation2020, p. 22).
Thus, the results, as well as previous research (cf. Anantrasirichai & Bull, Citation2022), demonstrate how AI is increasingly used in various stages of the creative process. The concept of independently creative AI remains disputed; this turns the question from independently creative AI to co-creativity, that is, how and when AI can best contribute to the creativity process. Co-creativity brings together the distributed, process and posthuman perspectives of creativity (Glaveanu & Kaufman, Citation2019; Meusburger et al., Citation2009; Roudavski & McCormack, Citation2016) and thus explains AI’s role in contemporary creative processes. This also highlights co-creativity as a temporal and spatial process; in some moments, human–AI co-creativity emerges, and along the process, the distribution of co-creativity changes. Such a process is also spatial, which in this empirical study was an implicit element of co-creativity.
Implications, limitations, and directions for future research
Finally, as the third contribution, we present two suggestions to the developers of (co-)creative AI. We support efforts to develop co-creative AI that augments human creativity instead of replacing it (Anantrasirichai & Bull, Citation2022, p. 589). We found various examples of human–AI interaction that could be deemed co-creative. However, in this sample, the scientists did not recognize a co-creative relationship with AI in their work, whereas many artists noted that they worked co-creatively with AI. This demonstrates how co-creativity is understood as an interaction with a specific type of AI, and most of the current co-creative AI is developed in the field of arts. However, we suggest that AI be developed in a co-creative direction in other domains, too, considering the domain-specific demands as well as the uniqueness of everyday creative processes. For example, based on our results, AI needs to be transparent and trustworthy in science, and it must be playful and surprising in the arts. We encourage collaboration with social scientific creativity research to understand co-creativity from distributed and posthuman perspectives as well. In the future, co-creativity can expand to describe creative human–AI interaction on a more comprehensive level.
When studying a novel phenomenon with little preexisting empirical research, a qualitative study is a justified choice. For a qualitative study, the present paper included vast empirical material. Our results can be applied to these domains internationally and, to some extent, beyond the domains to cover quantitative science and the digital arts. However, as a limitation of this study, we note that each participant has a unique creative process. The participants also use different types of AI, which might influence how they perceive co-creativity with AI. Moreover, certain pressing issues in the current AI-related research, such as ethical and legal questions, or the impact of AI on medicine, decision-making, or the labor market were beyond the scope of this research.
With these limitations in mind, we turn to future research. This study can be a stepping stone for a broader study of the use of AI in various domains. For instance, a survey could be used to estimate the influence of co-creativity on the skills required in the future labor market, organization, and management (Su, Togay, & Côté, Citation2021), or to study data bias and ethical issues (Kaplan & Haenlein, Citation2019). Ethical issues are relevant from the perspective of co-creativity as well (Müller, Citation2021). For instance, bias behind AI technology can be harmful for science, where the results must be trustworthy and transparent. In terms of AI-related ethics in the arts, the authorship and autonomy of AI is a greatly debated issue (Browne, Citation2022). We argue that co-creativity can explain the complex human–AI relationship and support artists in this matter, as it acknowledges the roles of both humans and AI in the process and does not strip artist of their creative share.
Concluding words
In conclusion, should creativity be redefined in the era of AI? Our answer is twofold. On the one hand, no. Scientists and artists who have long worked with AI still define creativity via the same five elements (actor, process, outcome, domain, and space) that adhere to the common creativity definitions. Moreover, the concept of creative AI remains disputed, and humans remain central in the creative tasks. On the other hand, considering how AI is increasingly used in creative processes, we argue that yes – creativity in the era of AI must be revised to co-creativity. The future possibilities of human–AI co-creativity are endless, and we are only beginning to explore them. This requires a shift from human-centered creativity studies to co-creativity research that explains the co-constituted, complex, and spatial process between humans and AI.
Disclosure statement
No potential conflict of interest was reported by the author(s).
Data availability statement
The anonymized and transcribed interview data will be opened for public access via the AILA research portal of the University of Tampere, Finland (www.fsd.uta.fi/en) after the research project is finished.
Additional information
Funding
References
- Adams, W.C. (2015) Conducting Semi-Structured Interviews. In J. S. Wholey, H. P. Harty, & K. E. Newcomer (Eds.), Handbook of Practical Program Evaluation (pp. 492–505). Hoboken, NJ: Jossey-Bass.
- Agnoli, S., Corazza, G. E., & Runco, M. A. (2016). Estimating creativity with a multiple-measurement approach within scientific and artistic domains. Creativity Research Journal, 28(2), 171–176. doi:10.1080/10400419.2016.1162475
- Amabile, T. M. (1996). Creativity in context: Update to the social psychology of creativity. New York, NY: Westview Press.
- Amabile, T. M., Conti, R., Coon, H., Lazenby, J., & Herron, M. (1996). Assessing the work environment for creativity. Academy of Management Journal, 39(5), 1154–1184. doi:10.5465/256995
- Anantrasirichai, N., & Bull, D. (2022). Artificial intelligence in the creative industries: A review. Artificial Intelligence Review, 55, 89–656. doi:10.1007/s10462-021-10039-7
- Baer, J. (2010). Is creativity domain-specific? In J. C. Kaufman & R. J. Sternberg (Eds.), Cambridge Handbook of Creativity (pp. 321–341). New York, NY: Cambridge University Press.
- Baer, J. (2013). Teaching for creativity: Domains and divergent thinking, intrinsic motivation, and evaluation. In M. Gregerson, J. Kaufman, & H. Snyder (Eds.), Teaching creatively and teaching creativity (pp. 175–181). Springer.
- Baer, J., & Kaufman, J. C. (2005). Bridging generality and specificity: The amusement park theoretical (APT) model of creativity. Roeper Review, 27(3), 158–163. doi:10.1080/02783190509554310
- Batey, M., & Furnham, A. (2006). Creativity, intelligence, and personality: A critical review of the scattered literature. Genetic, Social, and General Psychology Monographs, 132(4), 355–429. doi:10.3200/MONO.132.4.355-430
- Berman, A., & James, V. (2018). Learning as performance: Autoencoding and generating dance movements in real time. In A. Liapis, J. J. R. Cardalda, & A. Ekárt (Eds.), International Conference on Computational Intelligence in Music, Sound, Art and Design (pp. 256–266). Springer. doi:10.1007/978-3-319-77583-8.
- Biggs, S. (2009). New media: The “first word in art? In H. Smith & E. T. Dean (Eds.), Practice-led research, research-led practice in the creative arts (pp. 66–83). Edinburgh University Press.
- Boden, M. A. (2004). The creative mind: myths and mechanisms (2nd ed.). New York, NY: Routledge.
- Boden, M. A. (2009). Computer models of creativity. AI Magazine, 30(3), 23–34. doi:10.1609/aimag.v30i3.2254
- Boden, M. A. (2016). AI: Its nature and future. Oxford University Press.
- Botella, M., Glaveanu, V., Zenasni, F., Storme, M., Myszkowski, N., Wolff, M., & Lubart, T. (2013). How artists create: Creative process and multivariate factors. Learning and Individual Differences, 26, 161–170. doi:10.1016/j.lindif.2013.02.008
- Bray, L., & Bown, O. (2016). Applying core interaction design principles to computational creativity. In F. Pachet, A. Cardoso, V. Corruble, & F. Ghedini (Eds.), Proceedings of the Seventh International Conference on Computational Creativity (ICCC 206) (pp. 93–97). Paris, France: Sony CSL.
- Bringsjord, S., Bello, P., & Ferrucci, D. (2001). Creativity, the turing test, and the (better) Lovelace test. Minds and Machines, 11(1), 3–27. doi:10.1023/A:1011206622741
- Browne, K. (2022). Who (or What) Is an AI Artist? Leonardo, 55(2), 130–134. doi:10.1162/leon_a_02092
- Bullot, N. J., Seeley, W. P., & Davies, S. (2017). Art and science: A philosophical sketch of their historical complexity and codependence. The Journal of Aesthetics and Art Criticism, 75(4), 453–463. doi:10.1111/jaac.12398
- Canaan, R., Menzel, S., Togelius, J., & Nealen, A. (2018). Towards game-based metrics for computational co-creativity. In C. Browne, M. H. M. Winands, J. Liu, & M. Preuss (Eds.), Proceedings of the 2018 IEEE Conference on Computational Intelligence and Games (CIG’18) (pp. 482–489). Maastricht, The Netherlands.
- Choi, K., Fazekas, G., & Sandler, M. (2016). Text-based LSTM networks for automatic music composition [Paper presentation]. In 1st Conference on Computer Simulation of Musical Creativity (CSMC 16), Huddersfield, United Kingdom. arXiv:1604.05358
- Chung, N. C. (2021). Human in the loop for machine creativity. In 9th AAAI Conference on Human Computationand Crowdsourcing (HCOMP 2021), Virtual conference.arXiv:2110.03569.
- Colin, T. R., Belpaeme, T., Cangelosi, A., & Hemion, N. (2016). Hierarchical reinforcement learning as creative problem solving. Robotics and Autonomous Systems, 86, 196–206. doi:10.1016/j.robot.2016.08.021
- Colton, S. (2012). The painting fool: Stories from building an automated painter. In J. McCormack & M. d’Inverno (Eds.), Computers and creativity (pp. 3–38). Springer.
- Colton, S., & Wiggins, G., A. (2012). Computational creativity: The final frontier? In L. De Raedt, C. Bessiere, D. Dubois, P. Doherty, P. Frasconi, F. Heintz, & P. Lucas (Eds.), Proceedings of the 20th European Conference on Artificial Intelligence (ECAI) (pp. 21–26). Amsterdam, The Netherlands: IOS Press. doi:10.3233/978-1-61499-098-7-21
- Cropley, A. (2020). Creativity-focused technology education in the age of industry 4.0. Creativity Research Journal, 32(2), 184–191. doi:10.1080/10400419.2020.1751546
- Csikszentmihalyi, M. (1997). Creativity: Flow and the psychology of discovery and invention. New York, NY: HarperPerennial.
- Csikszentmihalyi, M. (2014). Society, culture, and person: A systems view of creativity. In M. Csikszentmihalyi (Ed.), The systems model of creativity (pp. 47–61). Dordrecht, The Netherlands: Springer. doi:10.1007/978-94-017-9085-7_4
- Csikszentmihalyi, M., & Sawyer, K. (2014). Creative insight: The social dimension of a solitary moment. In M. Csikszentmihalyi (Ed.), The systems model of creativity (pp. 73–98). Dordrecht, The Netherlands: Springer. doi:10.1007/978-94-017-9085-7_7
- Daniele, A., & Song, Y. (2019). AI art = human. In V. Conitzer, G. K. Hadfield, & S. Vallor (Eds.), Proceedings of the 2019 AAAI/ACM Conference on AI, Ethics, and Society (pp. 155-161). New York, NY: Association for Computing Machinery. doi:10.1145/3306618.3314233
- Davis, N. M. (2013). Human-computer co-creativity: Blending human and computational creativity. In G. Smith & A. Smith (Eds.), The Doctoral Consortium at AIIDE 2013 (pp. 9–12). The AAAI Press.
- De Garrido, L. (2021). Conceptual design of a creative artificial intelligence system based on the neurocognitive bases of human creativity in the brain. Creativity Research Journal, 1–22. doi:10.1080/10400419.2021.2005309
- Dvorsky, G. (2017). This artificially intelligent robot composes and performs its own music. Gizmodo. Accessed 1 March 2022. https://gizmodo.com/this-artificially-intelligent-robot-composes-and-perfor-1796093082
- Elgammal, A., Liu, B., Elhoseiny, M., & Mazzone, M. (2017). Can: Creative adversarial networks, generating” art” by learning about styles and deviating from style norms. In A. Goel, A. Jordanous, & A. Pease (Eds.), Proceedings of the 8th International Conference on Computational Creativity (ICCC’17) (pp.96–103). Georgia Institute of Technology.
- Elliott, A. (2019). The culture of AI: Everyday life and the digital revolution. Abingdon, UK: Routledge.
- Esling, P., & Devis, N. (2020). Creativity in the era of artificial intelligence. In JIM Conference 2020. Journées d’Informatique Musicale, Strasbourg, France. doi:10.48550/arXiv.2008.05959
- Fabiano, F., Pelikan, H. R., Pingen, J., Zissoldt, J., Catala, A., & Theune, M. (2017). Designing a co-creative dancing robotic tablet [Paper presentation]. In The 6th International Workshop on Computational Creativity, Concept Invention, and General Intelligence (C3GI 2017), Madrid, Spain.
- Feist, G. J. (1998). A meta-analysis of personality in scientific and artistic creativity. Personality and Social Psychology Review, 2(4), 290–309. doi:10.1207/s15327957pspr0204_5
- Fischer, G., Giaccardi, E., Eden, H., Sugimoto, M., & Ye, Y. (2005). Beyond binary choices: Integrating individual and social creativity. International Journal of Human-Computer Studies, 63(4–5), 482–512. doi:10.1016/j.ijhcs.2005.04.014
- Florida, R. (2014). The rise of the creative class, revisited. NewYork, NY: Hachette.
- Fujita, M. (2018). AI and the future of the brain power society: When the descendants of Athena and Prometheus work together. Review of International Economics, 26(3), 508–523. doi:10.1111/roie.12310
- Gatys, L. A., Ecker, A. S., & Bethge, M. (2016). Image style transfer using convolutional neural networks. In Proceedings of the IEEE Conference on Computer Vision and Pattern Recognition Las Vegas, NV, USA. (pp. 2414–2423). doi:10.1109/CVPR.2016.265
- Gioti, A. (2020). From artificial to extended intelligence in music composition. Organised Sound, 25(1), 25–32. doi:10.1017/S1355771819000438
- Glaveanu, V. P., & Kaufman, J. C. (2019). A historical perspective. In J. Kaufman R. & Sternberg (Eds.). Cambridge, UK: The Cambridge Handbook of Creativity (2nd ed.). Cambridge University Press. doi:10.1017/9781316979839.
- Glaveanu, V., Lubart, T., Bonnardel, N., Botella, M., de Biaisi, P., Desainte-Catherine, M., … Mouchiroud, C. (2013). Creativity as action: Findings from five creative domains. Frontiers in Psychology, 4, 1–14. doi:10.3389/fpsyg.2013.00176
- Grant, J. (2018). Creativity as an artistic merit. In B. Gaut & M. Kieran (Eds.), Creativity and philosophy (pp. 333–349). Abingdon, UK: Routledge.
- Guilford, J. P. (1950). Creativity. American Psychologist, 5(9), 444–454. doi:10.1037/h0063487
- Hawkins, J. (2021). A thousand brains - a new theory of intelligence. New York, NY: Basic Books.
- Hautala, J. (2021). Can robots possess knowledge? Rethinking the DIK (W) pyramid through the lens of employees of an automotive factory. Humanities and Social Sciences Communications, 8(1), 1–10. doi:10.1057/s41599-021-00893-9
- Hautala, J., & Ibert, O. (2018). Creativity in arts and sciences: Collective processes from a spatial perspective. Environment and Planning A, 50(8), 1688–1696. doi:10.1177/0308518X18786967
- Hautala, J., & Jauhiainen, J. S. (2014). Spatio-temporal processes of knowledge creation. Research Policy, 43(4), 655–668. doi:10.1016/j.respol.2014.01.002
- Hautala, J., & Jauhiainen, J. S. (2019). Creativity-related mobilities of peripheral artists and scientists. GeoJournal, 84(2), 381–394. doi:10.1007/s10708-018-9866-3
- Hautala, J., & Jauhiainen, J. S. (2022). Co-creating Knowledge with Robots: System, Synthesis, and Symbiosis. Journal of the Knowledge Economy, 1–21.
- Hayles, N. K. (2017). Unthought: The power of the cognitive nonconscious. University of Chicago Press. doi:10.7208/chicago/9780226447919.001.0001
- Hertzmann, A. (2018). Can computers create art? Arts, 7(2), 18. doi:10.3390/arts7020018
- Hong, J., Curran, N. M., Harandi, M., Zhou, Z., Pietikäinen, M., & Zhao, G. (2019). Artificial intelligence, artists, and art: Attitudes toward artwork produced by humans vs. artificial intelligence. ACM Transactions on Multimedia Computing, Communications, and Applications (TOMM), 15(2s), 1–16. doi:10.1145/3326337
- Hsieh, H., & Shannon, S. E. (2005). Three approaches to qualitative content analysis. Qualitative Health Research, 15(9), 1277–1288. doi:10.1177/1049732305276687
- Huizinga, J. (1938/1955). Homo ludens: A study of the play element in culture. Boston, MA: Beacon Press.
- Ihde, D. (1990). Technology and the lifeworld. Bloomington, IN: Indiana University Press.
- Jeon, M., Fiebrink, R., Edmonds, E. A., & Herath, D. (2019). From rituals to magic: Interactive art and HCI of the past, present, and future. International Journal of Human-Computer Studies, 131, 108–119. doi:10.1016/j.ijhcs.2019.06.005
- Jones, M. (2009). Phase space: Geography, relational thinking, and beyond. Progress in Human Geography, 33(4), 487–506. doi:10.1177/0309132508101599
- Jordanous, A. (2016). Four PPPPerspectives on computational creativity in theory and in practice. Connection Science, 28(2), 194–216. doi:10.1080/09540091.2016.1151860
- Kantosalo, A., & Riihiaho, S. (2019). Experience evaluations for human–computer co-creative processes–planning and conducting an evaluation in practice. Connection Science, 31(1), 60–81. doi:10.1080/09540091.2018.1432566
- Kantosalo, A., & Toivonen, H. (2016). Modes for creative human-computer collaboration: Alternating and task-divided co-creativity. In A. Cardoso, V. Corruble, & F. Ghedini (Eds.), Proceedings of the Seventh International Conference on Computational Creativity (pp. 77–84). Paris, France.
- Kaplan, A., & Haenlein, M. (2019). Siri, Siri, in my hand: Who’s the fairest in the land? On the interpretations, illustrations, and implications of artificial intelligence. Business Horizons, 62(1), 15–25. doi:10.1016/j.bushor.2018.08.004
- Karimi, P., Rezwana, J., Siddiqui, S., Maher, M. L., & Dehbozorgi, N. (2020). Creative sketching partner: An analysis of human-AI co-creativity. In Proceedings of the 25th International Conference on Intelligent User Interfaces, 221–230. Cagliary, Italy. doi:10.1145/3377325.3377522
- Kaufman, J. C. (2012). Counting the muses: Development of the Kaufman domains of creativity scale (K-DOCS). Psychology of Aesthetics, Creativity, and the Arts, 6(4), 298. doi:10.1037/a0029751
- Kirsch, C., Lubart, T., & Houssemand, C. (2014). Creative personality: The importance of an autonomous working style. In E. Xeni (Ed.), Creativity in educational research and practice (pp. 123–140). Brill. doi:10.1163/9781848883086_012
- Klausen, S. H. (2014). Interdisciplinarity and creativity. In E. Shiu (Ed.), Creativity research. an interdisciplinary and multidisciplinary research handbook (pp. 31–50). London: Routledge.
- Krippendorff, K. (2018). Content analysis: An introduction to its methodology (4th ed.). Thousand Oaks, CA: Sage publications.
- Lundman, R. (2012). Kaupunki leikkikenttänä [City as a Playground]. Alue Ja Ympäristö, 41(1), 3–13.
- Latour, B. (2013). Reassembling the social. An introduction to actor-network-theory. Journal of Economic Sociology, 14(2), 73–87. doi:10.17323/1726-3247-2013-2-73-87
- Lee, J., & Hollister, J. M. (2020). Internet-mediated research in the age of social distancing: Methodological reflections and recommendations from two online research projects. Journal of Korean Library and Information Science Society, 51(2), 319–353. doi:10.16981/kliss.51.2.202006.319
- Lin, Y., Guo, J., Chen, Y., Yao, C., & Ying, F. (2020). It is your turn: Collaborative ideation with a co-creative robot through sketch. In Proceedings of the 2020 CHI Conference on Human Factors in Computing Systems. New York, NY: Association for Computing Machinery.
- Lubart, T. I. (2001). Models of the creative process: Past, present and future. Creativity Research Journal, 13(3–4), 295–308. doi:10.1207/S15326934CRJ1334_07
- Lubart, T., & Guignard, J. (2004). The generality-specificity of creativity: A multivariate approach. In R. J. Sternberg, E. L. Grigorenko, & J. L. Singer (Eds.), Creativity: From potential to realization (p. 43–56). Washington, DC: American Psychological Association. doi:10.1037/10692-004
- Lucas, B. (2001). Creative teaching, teaching creativity and creative learning. In A. Craft, A. Craft, & A. Craft (Eds.), Creativity in education (pp. 35–44). Continuum.
- Maher, M. L. (2012). Computational and collective creativity: Who’s being creative? In M. L. Maher, K. Hammond, A. Pease, R. Pérez y Pérez, D. Ventura & G. Wiggins (Eds.), Proceedings of the Third International Conference on Computational Creativity (pp. 67–71). Dublin, Ireland: University College Dublin.
- Marnin-Distelfeld, S., & Dorchin, U. (2020). “I am not an artist, I make art”: Amateurish artists in Israel and the sense of creativity. Creativity Studies, 13(1), 64–86. doi:10.3846/cs.2020.9907
- Mazzone, M., & Elgammal, A. (2019). Art, creativity, and the potential of artificial intelligence. Arts, 8(1), 26. doi:10.3390/arts8010026
- Meusburger, P., Funke, J., & Wunder, E. (2009). Introduction: The spatiality of creativity. In P. Meusburger, J. Funke, & E. Wunder (Eds.), Milieus of creativity (pp. 1–10). Springer. doi:10.1007/978-1-4020-9877-2_1
- Miller, A. I. (2019). The Artist in the Machine: The World of AIPowered Creativity. Cambride, MA: Mit Press.
- Miller, A. I. (2020). Creativity in the Age of AI: Computers and artificial neural networks are redefining the relationship between art and science. American Scientist, 108(4), 244–249. doi:10.1511/2020.108.4.244
- Mitchell, M. (2019). Artificial intelligence: A guide for thinking humans. New York, NY: Penguin UK.
- Müller, V. C. (2021). Ethics of artificial intelligence and robotics. In E. N. Zalta (Ed.), The Stanford encyclopedia of philosophy. Accessed 1 March 2022 https://plato.stanford.edu/archives/sum2021/entries/ethics-ai
- Nieves, J., & Osorio, J. (2013). The role of social networks in knowledge creation. Knowledge Management Research & Practice, 11(1), 62–77. doi:10.1057/kmrp.2012.28
- Pease, A., & Colton, S. (2011). On impact and evaluation in computational creativity: A discussion of the Turing Test and an alternative proposal. In D. Kazakov & G. Tsoulas (Eds.), Proceedings of AISB ‘11: computing and philosophy (pp. 15–22). Society for the Study of Artificial Intelligence and Simulation of Behaviour.
- Pereira, F. C. (2007). Creativity and artificial intelligence: A conceptual blending approach. Berlin, Germany: Walter de Gruyter.
- Piplica, A., DeLeon, C., & Magerko, B. (2012). Full-Body gesture interaction with improvisational narrative agents. In Y. Nakano, M. Neff, A. Paiva, & M. Walker (Eds.), Intelligent virtual agents 2012. Lecture notes in computer science (Vol. 7502, pp. 514–516). Springer. doi:10.1007/978-3-642-33197-8_63
- Puryear, J. S., & Lamb, K. N. (2020). Defining creativity: How far have we come since plucker, beghetto, and dow? Creativity Research Journal, 32(3), 206–214. doi:10.1080/10400419.2020.1821552
- Ragot, M., Martin, N., & Cojean, S. (2020). Ai-generated vs. human artworks. a perception bias towards artificial intelligence? In Extended abstracts of the 2020 CHI conference on human factors in computing systems (pp. 1–10). New York, NY.
- Rhodes, M. (1961). An analysis of creativity. The Phi Delta Kappan, 42(7), 305–310.
- Ritchie, G. (2007). Some empirical criteria for attributing creativity to a computer program. Minds and Machines, 17(1), 67–99. doi:10.1007/s11023-007-9066-2
- Root-Bernstein, M. (2014). Inventing imaginary worlds: From childhood play to adult creativity across the arts and sciences. Lanham, MD: Rowman & Littlefield.
- Root-Bernstein, R., & Root-Bernstein, M. (2004). Artistic scientists and scientific artists: The link between polymathy and creativity. In R. J. Sternberg, E. L. Grigorenko, & J. L. Singer (Eds.), Creativity: From potential to realization (p. 127–151). Washington, DC: American Psychological Association. doi:10.1037/10692-008
- Rose, R. (2017). Posthuman agency in the digitally mediated city: Exteriorization, individuation, reinvention. Annals of the American Association of Geographers, 107(4), 779–793. doi:10.1080/24694452.2016.1270195
- Roudavski, S., & McCormack, J. (2016). Post-anthropocentric creativity. Digital Creativity, 27(1), 3–6. doi:10.1080/14626268.2016.1151442
- Runco, M. A. (1994). Problem finding, problem solving, and creativity. Norwood, NJ: Greenwood Publishing Group.
- Runco, M. A., & Acar, S. (2012). Divergent thinking as an indicator of creative potential. Creativity Research Journal, 24(1), 66–75. doi:10.1080/10400419.2012.652929
- Runco, M. A., & Jaeger, G. J. (2012). The standard definition of creativity. Creativity Research Journal, 24(1), 92–96. doi:10.1080/10400419.2012.650092
- Russell, S., & Norvig, P. (2010). Artificial intelligence: A modern approach (3rd ed.). Harlow, UK: Pearson.
- Salen, K., & Zimmerman, E. (2003). Rules of play: Game design fundamentals. Cambridge, MA: MIT Press.
- Sax, L. J., Lehman, K. J., Jacobs, J. A., Kanny, M. A., Lim, G., Monje-Paulson, L., & Zimmerman, H. B. (2017). Anatomy of an enduring gender gap: The evolution of women’s participation in computer science. The Journal of Higher Education, 88(2), 258–293. doi:10.1080/00221546.2016.1257306
- Simonton, D. K. (2004). Creativity in science: Chance, logic, genius, and zeitgeist. Cambridge, UK: Cambridge University Press.
- Simonton, D. K. (2013). What is a creative idea? little-c versus big-C creativity. In J. Chan & K. Thomas (Eds.), Handbook of Research on Creativity. Cheltenham, UK: Edward Elgar. doi:10.4337/9780857939814.00015
- Snow, C. P. (1993). The two cultures (revised edition ed.). Cambridge, UK: Cambridge University Press.
- Stein, M. I. (1987). Creativity research at the crossroads: A 1985 perspective. In S. G. Isaksen (Ed.), Frontiers of creativity research: Beyond the basics (pp. 417–427). Buffalo, NY: Bearly Ltd.
- Sternberg, R. J. (2009). Domain-generality versus domain-specificity of creativity. In P. Meusburger, J. Funke, & E. Wunder (Eds.), Milieus of creativity (pp. 25–38). Spinger.
- Sternberg, R. J. (2018a). A triangular theory of creativity. Psychology of Aesthetics, Creativity, and the Arts, 12(1), 50. doi:10.1037/aca0000095
- Sternberg, R. J. (2018b). Evaluating merit among scientists. Journal of Applied Research in Memory and Cognition, 7(2), 209–216. doi:10.1016/j.jarmac.2018.03.003
- Stevens, C. E., Jr, & Zabelina, D. L. (2020). Classifying creativity: Applying machine learning techniques to divergent thinking EEG data. NeuroImage, 219, 116990. doi:10.1016/j.neuroimage.2020.116990
- Su, Z., Togay, G., & Côté, A. M. (2021). Artificial intelligence: A destructive and yet creative force in the skilled labour market. Human Resource Development International, 24(3), 341–352. doi:10.1080/13678868.2020.1818513
- Toivonen, H., & Gross, O. (2015). Data mining and machine learning in computational creativity. Wiley Interdisciplinary Reviews: Data Mining and Knowledge Discovery, 5(6), 265–275.
- Tracy, S. J. (2019). Qualitative research methods: Collecting evidence, crafting analysis, communicating impact. Hoboken, NJ: John Wiley & Sons.
- Wyse, L. (2019). Mechanisms of artistic creativity in deep learning neural networks. In K. Grace, M. Cook, D. Ventura, M. L. Maher (Eds.), Proceedings of the Tenth International Conference on Computational Creativity (ICCC’19) (pp. 116–123). Charlotte, UK: Association for Computational Creativity.
- Zylinska, J. (2020). AI art. London, UK: Open Humanities Press.