Abstract
Total phosphorus (TP) concentrations are known to be a significant factor influencing fish populations in Minnesota lakes. Consequently, a primary focus of the Minnesota Department of Natural Resources to address fish habitat in lakes across the state has been to determine relationships between TP concentrations and watershed conditions in Minnesota lakes. Because phosphorus concentrations in Minnesota lakes vary widely corresponding to differences in geomorphology, nutrient criteria were established by the Minnesota Pollution Control Agency for specific ecoregions. To refine these relationships in Minnesota lakes managed for fishing, we gathered mean summer epilimnetic TP concentrations on 1330 natural lakes to identify where agricultural and urban development have elevated phosphorus levels. Random forest, regression tree, and generalized additive models were used to model spatial variation in lake phosphorus concentrations across Minnesota. Key landscape variables known to influence TP concentrations in lakes, including lake depth and watershed size, were used as explanatory variables in these models, along with agricultural and urban development quantified for lake watersheds from the National Land Cover Dataset. These models explained up to 60% of the variation in TP in lakes across the state and showed a critical benchmark of anthropogenic land use disturbance at 40%, that once exceeded could significantly alter TP levels and consequently fish populations. This information should be useful for fish managers to prioritize conservation efforts and to set appropriate fish population goals.
Identifying how lakes are impacted by human activities is critical for resource agencies responsible for protecting and enhancing aquatic habitats. Aquatic habitat in lakes is highly influenced by phosphorus and significantly affects the composition of fish communities. Coldwater fish communities are especially vulnerable to eutrophication from increased phosphorus concentrations. Hypolimnetic oxygen concentrations decline as lake productivity increases (Nürnberg Citation1995). Decreases in hypolimnetic oxygen concentrations have direct negative effects on fish that physiologically require oxygenated cold water to survive, grow, and reproduce. Cultural eutrophication has caused declines in many European and North American coldwater fish populations (Hasler Citation1947, Tammi et al. Citation1999). Latta (Citation1995) reported that cisco (Coregonus artedii) have been extirpated in at least 14% of Michigan lakes, primarily from eutrophication. Also, fish populations in lakes are highly influenced by plant cover, which is largely determined by water quality and trophic status (Hoyer and Canfield Citation1996, Crosbie and Chow-Fraser Citation1999, Cross and McInerny Citation2005). Direct manipulations of plant communities in lakes are difficult to accomplish compared to managing the riparian and watershed influences affecting plant communities (Crowder et al. Citation1996). Consequently, efforts aimed at managing fish populations need to account for phosphorus levels as an important factor in formulating strategies for protecting and restoring fish habitat. Protection is viewed as the most cost effective strategy when applied to watersheds where human activities have not already significantly elevated phosphorus levels; otherwise, watershed restoration may be required at levels dictated by anthropogenic landscape disturbances.
Minnesota is fortunat to have many natural lakes with desirable water quality that are important to lake users (Krysel et al. Citation2003). These lakes deliver important ecosystem services such as fishing, boating, and swimming, as well as providing high quality habitats for diverse and important plant and animal communities. These ecosystem services are an important part of the state's economy. For example, in 2001 anglers spent $1.3 billion on fishing-related expenses in Minnesota (USFWS Citation2002). Continued delivery of these highly valued ecosystem services requires identification of processes such as land use practices that threaten the water quality that forms the basis of those services.
General patterns of phosphorus levels in Minnesota lakes have been described by Moyle (Citation1956), Heiskary et al. (Citation1987), and Omernik et al. (Citation1988), and other studies have shown increases in phosphorus concentrations associated with human land use activities. Urbanization and agriculture are the 2 most important contributors to water quality degradation in north temperate lakes (Carpenter et al. Citation1998, Drake and Pereira Citation2002). Nonpoint sources contribute 84% of the phosphorus discharged into United States surface waters (Carpenter et al. Citation1998) and 69% in Minnesota (Barr Engineering Citation2004). Cultural eutrophication in Minnesota lakes has occurred primarily in the Eastern Temperate Forests and Great Plains ecoregions and not in the Northern Forest ecoregion (Ramstack et al. Citation2004). Conversion of prairie and hardwood forests to agriculture after European settlement corresponded with large increases in lake phosphorus levels between 1800 and 1970 according to fossil diatom evidence in lake sediment cores (Umbanhowar et al. Citation2003, Ramstack et al. Citation2004). Urbanization, especially in the Minneapolis–St. Paul metropolitan area, also increased phosphorus concentrations in Eastern Temperate Forest ecoregion lakes after European settlement. While Brezonik (Citation2002) found evidence that shoreline development may be affecting water quality in Minnesota lakes, Johnston and Shmagin (Citation2006) found it mostly restricted to shallow lakes in highly urbanized areas.
Modeling the effects of land use on the delivery of phosphorus to a lake has been the focus of research for a number of years (Reckhow and Simpson Citation1980, Soranno et al. Citation1996, Alexander et al. Citation2004). For individual lakes, the export coefficient model of Reckhow and Simpson (Citation1980) has become a standard method for predicting phosphorus loading from watershed land use information (Endreny and Wood Citation2003) and has been successfully used by the Minnesota Pollution Control Agency (Heiskary and Wilson Citation2005). Even so, there is little information at larger statewide scales assessing the impact of human land use disturbances on lake nutrient levels. Empirical models incorporating watershed land use at a regional or statewide scale, such as those developed by Jones et al. (Citation2008) for midcontinent reservoirs and by Soranno et al. (Citation2008) for Michigan lakes, appear promising; however, data and model constraints limit the direct transferability of these models to lakes in other areas. More recently, Catherine et al. (Citation2010) related land use and catchment characteristics to the eutrophication status of French lakes using novel modeling techniques, including generalized additive models (GAM) and random forest (RF) that capture nonlinear relationships and interactions among predictors. Nonlinear modeling techniques are particularly useful for identifying threshold response levels common in ecological systems such as lake ecosystems. The objective of this study was to develop empirical spatial models at statewide and regional scales that identify effects of land use disturbance on total phosphorus in fishing lakes managed in Minnesota.
Methods
To identify land use disturbance impacts on lake phosphorus levels we assembled data on Minnesota Department of Natural Resources (MNDNR)-managed fishing lakes that had available mean summer total phosphorus (TP) data; TP is considered the best measurement for quantifying phosphorus in lakes, and it strongly relates to lake trophic status and algal biomass (Clark et al. Citation2010). We then characterized effects of land use disturbance on TP levels using empirical models derived using RF, classification and regression tree analysis (RTA), GAM, and linear modeling (LM). Lake and watershed factors used in previous empirical models at various scales were employed as covariates along with variables describing anthropogenic land use disturbance to predict TP levels in Minnesota lakes managed for fishing. Empirical approaches are particularly suited for broad spatial scale studies that seek information for directing management effort from a statewide or regional perspective.
Table 1 Akaike information criteria (AIC), Bayesian information criteria (BIC), and adjusted R2 for 7 individual one-way analysis of variance models predicting log10 mean summer TP (ppb) in 1330 Minnesota study lakes using different regionalization schemes as a categorical predictor variable. EPA ecoregions are described by Omernik (Citation1987), Ecological Classification System (ECS) is described by Cleland et al. (Citation1997), and soil landforms by Cummins and Grigal (Citation1981).
Study lakes and watershed attributes
We obtained data for 1330 lakes widely distributed across Minnesota that represent a broad range of physiographic regions (). All study lakes have a surface area ≥40 ha, at least one MNDNR fish population survey, and watersheds located completely within the Minnesota border. Lake surface area and maximum lake depths (zmax) were obtained from the MNDNR fish survey database and are based on MNDNR bathymetric surveys verified and updated with aerial imagery (MNDNR Citation2011). We used geographic lake center points () to assign ecoregion and physiographic schemes () to the study lakes. Much of the influence of climate, land cover, and geomorphology are characterized by the Ecological Land Classification (ECS) system (http://www.dnr.state.mn.us/ecs/index.html). According to Cleland et al. (Citation1997) the landscape scale (ECS landform units) characterizes much of the influence terrestrial processes would have on aquatic systems. The Omernik Environmental Protection Agency (EPA) ecoregion classification is very similar; however, these ecoregional units are delineated with more consideration of land cover based on contemporary land use (Omernik Citation1987, Citation2004) instead of on native plant communities weighted more heavily with the ECS system. We also analyzed soil landscape units identified by Cummins and Grigal (Citation1981), which are based on Minnesota geologic history and soil characterizations.
Figure 1 Distribution of 1330 study lakes across Minnesota shown with 3 major EPA level I ecoregions represented in Minnesota (Omernik Citation1987; Northern Forests, Eastern Temperate Forests, and Great Plains). The inset shows the U.S. distribution of the same 3 ecoregions (cross-hatched areas denote other ecoregions).
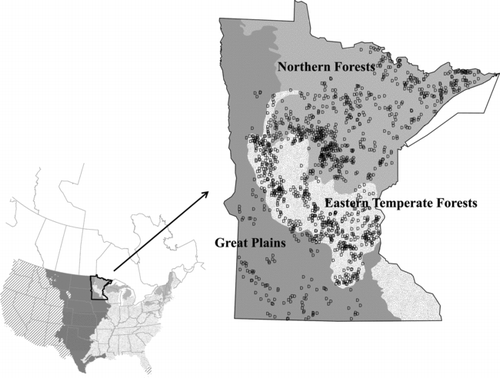
Watersheds were delineated using GIS to derive hydrologically corrected digital elevation models and flow networks guided by field input to verify accuracy and processing errors (http://www.dnr.state.mn.us/watersheds/lakeshed_project.html). Hydrologic units nested in a multilevel, hierarchical drainage system define lake watersheds and fold into the national standards defining the watershed boundary dataset (MNDNR Citation2011). Because significant contributing sources of TP can occur outside the direct contributing catchments (Heathwaite Citation2010), we used lake watersheds defined as the sum of all hydrologic units contributing directly to that lake or upstream.
Anthropogenic land use disturbances and other key watershed factors (in addition to physiographic region) identified by published studies as affecting lake TP levels were summarized by lake watershed. First, we calculated the ratio of watershed area to lake surface area (W:L), a variable that reflects the amount of runoff and flushing affecting each lake. Soils with parent material formed by glacial outwash are usually well drained. We used maps developed by Cummins and Grigal (Citation1981) and digitized by MNDNR (Citation2011) to identify areas with glacial outwash, which we summarized as a percent of the total watershed for each lake. We summarized land use categories assigned by the National Land Cover Database (Homer et al. Citation2004) as a percent of the total watershed area for each lake. This was a logical choice because the database is well studied and widely available and because the time frame was a good match for our TP data. NLCD classes 21, 22, 23, and 24 were used to represent development; 31 to represent mining; 41, 42, 43, and 52 to represent forest, and 82 to represent cropped agriculture. Anthropogenically disturbed land use was calculated as the sum of developed, mining, and cultivated agriculture land use. Finally, we assigned each lake watershed US Geological Survey (USGS) SPARROW estimates (Smith et al. Citation1997) of TP yield (kg/km2/y) from animal production derived for hydrologic unit code (HUC) level 8 units.
Total phosphorus
We assembled data on mean summer TP (ppb) in the epilimnion collected from 1993 to 2005 for Minnesota lakes. For most lakes we retrieved data from the EPA STORET, which was most often collected by the Minnesota Pollution Control Agency. These data were supplemented with TP data from samples collected during MNDNR lake surveys with chemical analyses performed by the Minnesota Department of Agriculture Laboratory using standard methods. Potential outliers were identified by manually examining extreme values. In addition, a parameter that measured the relative influence of each observation on a lake mean was calculated using , where xi
is the individual observation,
is the lake mean, and n is the number of samples for the lake. Observations with large relative influences were manually examined, and obvious errors were omitted from the analysis.
Table 2 Summary statistics (left) and Pearson's correlation matrix (right) calculated with the complete set of 1330 Minnesota lakes used in this study for mean lake total phosphorus (TP in ppb); maximum lake depth (m); watershed:lake area ratio (W:L); percent of watershed classified as outwash, agriculture, development, forest, disturbed (agriculture+development+mining); and USGS SPARROW estimates of TP yield from animal production (kg/km2/y).
Data analysis
Our initial analysis involved calculating summary statistics and examining histograms to assess the distribution of the dependent variable (TP) and various predictor variables. Consequently, for all subsequent analysis we used log10 transformation of TP. Prior to correlation analysis and linear modeling we used arcsine transformations on land cover proportions and log10 transformations on zmax and W:L to improve normality. A matrix of Pearson's correlation coefficients was calculated to reveal relationships of predictors with TP and among each other.
Statewide empirical modeling
We developed predictive models of TP using zmax, W:L, proportion of watershed with disturbed land use, proportion with outwash soils, and EPA Omernik level I ecoregions as predictor variables. These predictor variables were selected based on Soranno et al. (Citation2008) and by availability of data for Minnesota lakes. The predictor variables were relatively independent of each other except for a weak inverse correlation between land use disturbance and zmax and a stronger inverse correlation between land use disturbance and forest cover, a key descriptor variable relating to differences among Omernik ecoregions. We employed both RF and GAM procedures at the statewide scale because they excel in predictive accuracy and are sufficiently robust for analyzing multimodal and nonlinear relationships expected as a consequence of the high landscape diversity and agricultural and urban nutrient sources (Brieman Citation2001, Olden et al. Citation2008, and Catherine et al. Citation2010). Nonlinear modeling techniques were also desirable because we were specifically interested in the premise that specific thresholds of human disturbance exist that when exceeded lead to a rapid acceleration of TP levels. An inflection point in a curvilinear gradient between disturbance and TP provides the most obvious solution to identifying a possible threshold. To identify possible threshold levels of disturbance we plotted the specific response of land use disturbances on TP after accounting for the other predictors.
Both RF and GAM were implemented in R (R Development Core Team Citation2011) on the same statewide dataset. The GAM procedure fits the dependent variable using nonparametric smoothing curves fit to each variable, similar to doing a multiple linear regression with curvilinear functions. For GAM calculations we used the mgcv package, which automatically selects degrees of freedom for each smooth term in the model by minimizing the generalized cross validation score. The GAM models were run using a Gaussian distribution and default settings. We used the GAM plot function to examine the contribution of each component's smooth function on TP and to evaluate the statistical significance of each smooth term based on the null hypotheses that each smooth term is zero, similar to probability values of terms that would be calculated in a general linear model.
The RF procedure is based on aggregating a collection of regression trees. The RTA procedure is explained in more detail in the regional analysis section (below), but in essence it explains variation in the dependent variable by evaluating successive binary splits of each predictor variable resulting in a model analogous to a binary key. We used the random Forest package (Liaw and Wiener Citation2010) to create RF models, a collection of regression trees that are averaged using a bootstrapping technique (Liaw and Wiener Citation2002, Prasad et al. Citation2006). After several trial runs we determined the procedure would operate best by growing 1000 trees (ntree = 1000) and using 3 randomly selected variables at each split (try = 3). Two measures of relative importance were obtained using the RF procedure that identifies the most relevant predictors. One measure of variable importance was calculated as the percent of prediction error (MSE) for each predictor on an aggregation of bootstrap samples (Liaw and Wiener Citation2010). The second measure was the total decrease in node impurities from splitting on the variable, averaged over all trees measured by residual sum of squares (Liaw and Wiener Citation2010). For this measure, predictors that appear the most times at split points have the most reduction of impurity and are most important. For both measures of variable importance, larger values are indicative of greater model contributions. Partial dependence plots were used to examine the behavior of individual predictors on mean lake phosphorus with the effects of the other predictor variables removed. Additionally, we used GIS to compare the spatial distribution and autocorrelation of both RF and GAM model residuals (predicted minus observed).
Table 3 Contribution of predictor variables in generalized additive (GAM) and random forest (RF) statewide empirical models of TP in Minnesota lakes. Two importance factors are provided for each RF predictor; percent mean square error (%MSE) and increase in node purity. The contribution of each GAM predictor is shown with F ratio.
Regional modeling
We sought further insight by modeling at a regional scale because post hoc inspection of the geographic distribution of residuals from the statewide model were spatially autocorrelated, which arises from the influence of land use disturbance that likely varies with differences in resiliency among regional landscapes (Heathwaite Citation2010). By definition, much of the spatial variation in geomorphology (glacial history, deposition of parent material, topography), climate, and plant communities is already captured in various regionalization schemes (ecoregions). Ecoregions have been shown to be useful in accounting for variation in phosphorus and other water quality variables (Heiskary et al. Citation1987, Cheruvelil et al. Citation2008). In addition to EPA Omernik level I regions used for the empirical statewide model, we evaluated other regionalization schemes to account for geographical variation in TP. A series of one-way analyses of variance was used to assess the fit to TP with 7 regionalization schemes (). An optimal regionalization scheme was then selected based on comparisons among models using adjusted R2, Akaike information criteria (AIC), and Bayesian information criteria (BIC) values. Furthermore, we once again used GIS to inspect the spatial distribution and autocorrelation of residuals resulting from each model as a measure of suitability.
Next, we employed the optimal regionalization scheme with other natural lake and watershed factors (zmax, W:L, and outwash) to classify lakes by regional baseline TP levels. Establishing regional baseline TP levels resulting from natural factors allowed us to parse out effects of land use disturbance that we were specifically interested in modeling. To classify regional baseline TP conditions in lakes we used RTA applied to the complete statewide dataset using the rpart package implemented in R (R Development Core Team. Citation2011). The complexity parameter for pruning the tree in rpart was set to 0.02, essentially stopping model building once splits did not account for more than 2% of the variation in the dependent variable. We also reran the analysis using the partition decision procedure in JMP 9.0 (SAS Citation2010), which uses slightly different splitting criteria as a check on model reliability. The analysis was validated using a 10-fold cross validation analysis, which involved dividing the original data into 10 subsets and comparing model best fit (R2) for these 10 subsets to the original model (SAS Citation2010).
Following RTA classification we used GAM and LM to determine the specific influence of individual land use disturbance variables on TP. The same GAM methodology specified for the statewide model was also used for the regional disturbance modeling. Separate models were run for each RTA lake class and also collectively with RTA class added along with disturbance variables as predictors. Disturbance variables used in the models were proportion agriculture, proportion urbanized development, and animal agriculture TP yield (kg/km2/y). Because some of the disturbance variables seemed to influence TP linearly, we also modeled TP using same disturbance variables in a linear model implemented with the R lm module (The R Development Core Team Citation2011) to add statistical rigor.
Results
Our set of 1330 Minnesota lakes varied considerably in physical characteristics and watershed attributes (). Mean summer epilimnetic TP ranged from 4 to 722 ppb, zmax ranged down to 64 m, and W:L ranged from <2:1 to >5000:1. Individual anthropogenic land use disturbances ranged from more than 90% of the watershed in heavily urbanized and agricultural settings, to near zero in forested settings.
Predictor variables were often correlated and related to TP (). Among the 3 nonanthropogenic disturbance variables (zmax, W:L, and outwash), zmax was the most correlated with TP. Lakes with lower TP tended to be deeper and have watersheds containing higher amounts of glacial outwash. W:L area ratio had only a weak positive correlation with TP. Among anthropogenic disturbance variables, percent disturbance had the highest correlation with TP. Because land use variables are all proportions of the same area they are highly correlated. Also, there was a strong positive correlation between the contribution of agricultural animal phosphorus yield and cultivated land use and negative correlation with forested land use.
Statewide empirical modeling
RF and GAM models using EPA level I ecoregions, zmax, W:L, outwash, and land use disturbance explained 60.1 and 59.7% of the variation in TP among Minnesota lakes, respectively. Both modeling procedures also showed similar influences of the predictor variables on TP, with zmax and disturbed land use being most influential (; ). A curvilinear relationship between TP and both zmax and disturbed land use is very evident by a precipitous drop in lake TP between 2 and 10 m zmax and land use disturbance less than approximately 40% of watershed area ().
Figure 2 The influence of individual predictor variables on log10 mean summer TP (ppb) based on random forest (RF; left column) and generalized additive models (GAM; right column) in Minnesota lakes. The dependent axis for the RF generated plots shows predicted TP and for the GAM model it shows the deviation from the overall mean log10 TP of all 1330 study lakes (1.52). Dashed lines represent 1 SE deviation from the model fit.
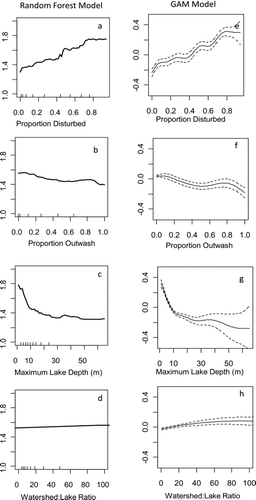
Regional modeling
Our evaluation of regional schemes showed that EPA level IV ecoregions were most optimal for explaining variation in TP levels among Minnesota lakes. The level IV ecoregion scheme had the highest R2 along with the lowest AIC and BIC scores compared to all other regionalization schemes analyzed with one-way ANOVA models (). Furthermore, post hoc analysis revealed the use of level IV ecoregions resulted in the least amount of spatial autocorrelation.
Using RTA, 5 groups of lakes were classified with homogeneous baseline TP levels (). This classification accounted for 55% of the variation in TP levels among lakes using only EPA level IV ecoregions and zmax. The classification distinguished between deep and shallow lakes in forested and prairie/agricultural regions along with a subunit comprised of “transitional” ecoregions prone to contain shallow lakes with higher TP.
Figure 4 Classification tree model of baseline log10 mean summer TP (ppb) in Minnesota lakes predicted using EPA level IV ecoregions and maximum lake depth as predictors. Boldface type denotes node mean log10 TP. Inset graph shows a progressive reduction in the complexity parameter R2 in the model with each split of the dataset.
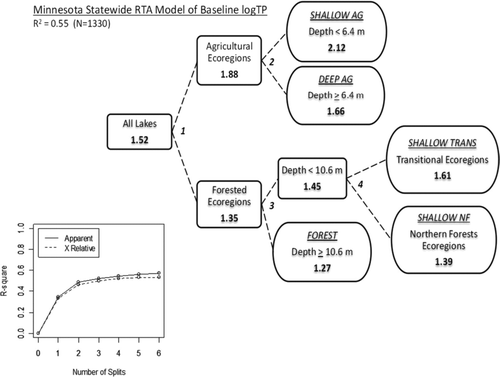
Table 4 Statistical probability levels of the contribution of each of the 3 disturbance predictor variables used in generalized additive models (GAM) of log10 mean summer TP (ppb) within each ecoregion-depth class (); deep-forest (DF), shallow-forest (SF), shallow transition ST), deep-agriculture (DA), shallow-agriculture (SA). Sample size (N) and R2 listed for each model.
Once baseline TP levels were established with RTA ecoregion–depth classification, we identified specific responses in TP resulting from watershed land use disturbances. GAM models of TP using disturbance variables (agriculture, development, and animals) as predictors within individual RTA ecoregion–depth classes explained from 7.4 to 31.3% of the variation in TP beyond baseline levels (). With all classes combined, a threshold level of approximately 40% was found with both development and agriculture ( and ). Because few lakes have watersheds that exceed 40% development, the sample size was insufficient within lake ecoregion–depth classes to precisely model a TP response across a broad range of development; however, ecoregion–depth classes differed in their response to agricultural disturbance. Phosphorus levels in agricultural and transitional regions elevated rapidly in response to agricultural land use, while the response in forested regions was much less, especially in deeper lakes (). Agricultural animal production was associated with elevated TP levels statewide once animal phosphorus yields exceeded approximately 50 kg/km2/y (), but too few lakes had watersheds with animal P loading >50 kg/km2/y to discern differences among individual ecoregion–depth classes.
Our optimal linear model, specified as TP∼lake class *proportion agriculture + lake class*proportion urban + animal phosphorus yield, provided a similar fit as the GAM model to the response of TP to land use disturbance (LM R2 = 0.60, AIC = 192.0, BIC = 103.7; GAM R2 = 0.60, AIC = 118.5, BIC = 246.3). The linear model confirmed significant effects of all three disturbance variables and statistically significant differences between all ecoregion–depth classes except between the 2 deep lake classes (). The model also confirmed significant differences in the response of TP to agricultural land use between forested and agricultural/transitional regions, confirming the trends shown in GAM plots ().
Figure 5 The influence of disturbance variables (a) development, (b) animals, and (c) agriculture on TP conditions in Minnesota lakes from generalized additive models. Model results for agriculture land use effects within individual ecoregion–depth classes () are depicted in d–h. The dependant axis shows deviation from the overall log10 mean summer TP (ppb) listed in parenthesis for each class. Dashed lines represent 1 SE deviation from the model fit.
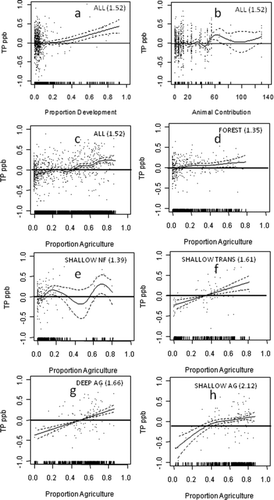
Discussion
Our empirical modeling showed TP to have a curvilinear relationship with watershed disturbance in Minnesota lakes. TP concentrations increased steadily as watershed disturbance increased to around 40%, then increased significantly at greater watershed disturbances. The 40% land use disturbance value could provide a valuable benchmark for guiding land use management in the watersheds of lakes in Minnesota and possibly for other North American lakes in similar ecoregions. Lakes with watersheds <40% land use disturbance would be good candidates for protection, while lakes with more disturbance might need restoration. The relationship between TP and land use disturbance may also be valuable for lake managers considering other alternatives. For example, the increase in TP associated with 40% land use disturbance might be unacceptably large for high water quality lakes with undisturbed watersheds. Alternatively, a goal of 40% land use disturbance might be unrealistically low for lakes with highly disturbed watersheds located in areas with intensive urbanization or agriculture. The curvilinear response of TP to watershed disturbance should allow lake managers to develop realistic goals for protection and restoration.
Predictors used in our model seem to behave similarly to the hydrogeomorphic-land use multiple regression model developed by Soranno et al. (2008; TP∼mean depth+ percent outwash+water color+ percent agriculture and urban), although our model reflected curvilinear responses over a greater range of TP. Furthermore, we determined that agriculture is the most prevalent form of land use disturbance affecting TP levels in Minnesota lakes and is primarily confined within agricultural and transitional regions. Consequently, Minnesota forest region lakes are relatively undisturbed by watershed land use practices except for the few that have relatively high levels of development. These findings agree with results of sediment diatom analysis that show contemporary TP levels in northern lakes and forest (NLF) ecoregion lakes unchanged from a period prior to European settlement (Heiskary et al. Citation2004, Ramstack et al. Citation2004). Conversely, diatom-inferred TP levels in western corn belt plain (WCP) and central hardwood forest (CHF) ecoregion lakes (comparable to our agricultural and transition region lakes) were significantly higher at present than during pre-European settlement. Many other studies have also shown increases in TP in lakes related to the intensity of agricultural cultivation (Umbanhowar 2003, Jones et al. Citation2004, Soranno et al. Citation2008, Taranu and Gregory-Eaves Citation2008) and determinations of higher phosphorus exports with agricultural land as opposed to forested land (Endreny and Wood Citation2003).
Table 5 Linear model of the response of log10 mean summer TP to land use disturbance (percent developed land use, percent agricultural land use, and animal phosphorus contribution) and ecoregion–depth classes; deep-forest (DF), shallow-forest (SF), shallow transition ST), deep-agriculture (DA), shallow-agriculture (SA).
Although TP is lower in deeper lakes than shallow lakes, we still found evidence of elevated TP levels in deeper lakes at agricultural land use >40%. This evidence is inconsistent with sediment diatom studies showing TP levels unchanged from the pre-European era in 5 dimictic lakes located in the heavily agricultural WCP (Ramstack et al. Citation2004, Heiskary et al. Citation2004); however, the higher TP response we observed in agricultural lakes could be due to the large sample size of lakes (216), including many located outside the WCP in agricultural areas located in the CHF ecoregion. Nonetheless, we found deep lakes to have a lower response rate of TP to agriculture land use than shallow lakes. These findings are consistent with numerous lake phosphorus models reviewed by Brett and Benjamin (Citation2008) in which resiliency to external phosphorus loading increases with depth. Shallow lakes are more likely to have higher TP because of significantly higher levels of internal phosphorus loading resulting from resuspension and bioturbation. Furthermore, Johnston and Shmagin (Citation2006) indicate that deeper lakes in Minnesota are more likely to tap into regional groundwater regimes, which would lead to lower TP levels.
Our results confirm a nonlinear relationship between land use disturbance and TP that differs in magnitude among ecoregions and zmax. Consequently, RF and GAM methods yielded optimal predictive models for describing a land use disturbance threshold. Close agreement between GAM and RF models along with relatively high precision added confidence in our conclusions regarding the effects of land use disturbance on TP. In a similar study of French lakes, Catherine et al. (Citation2010) also found RF and GAM to provide superior predictive models of eutrophication status using environmental factors. In their analysis, RF models performed only slightly better than GAM models, which they attributed to the ability of RF to handle interactions between predictors in addition to multimodality.
We found mapping and analyzing the geographic distribution of model residuals combined with GIS overlays of additional explanatory variables useful for evaluating spatial coincidence of possible covariates. Despite a relatively high level of predictive accuracy, significant levels of spatial autocorrelation were evident in residuals from the statewide empirical model that directed us to incorporating finer resolution ecoregion units in subsequent models. Ecoregion units, by accounting for spatial differences in geomorphology (including lake morphology), climate, soils, and plant cover, grouped lakes into more homogenous units with respect to phosphorus and improved model accuracies and goodness of fit. Furthermore, individual models unique for each ecoregion showed how TP responds to different landscape influences. For our purposes, the EPA ecoregion scheme performed better than the ECS scheme. The EPA ecosystem classification scheme was formulated to characterize influences on water body chemistry and places emphasis on hydrology (Omernik et al. Citation2000). ECS ecoregions are designed to delineate differences in terrestrial native plant communities and forest cover as opposed to water bodies (Cleland et al. Citation1997, Omernik et al. Citation2000).
Our state and regional scale model fits into the continuum of lake models that range from broad-scale (continental and beyond) and highly empirical to lake specific and highly mechanistic. Relying on our models to accurately predict precise responses for an individual lake is not advisable. Our study lakes were selected opportunistically based on availability of TP assessments in an attempt to get the broadest range of variability of disturbance possible, so there could be a bias toward larger and more “socially significant” lakes. Because our study was limited to lakes >40 ha, we advise caution when making conclusions about lakes with smaller surface areas. As a consequence of the broader spatial scale of our analysis, the models of this study did not take into account many of the mechanistic factors known to influence TP in specific lakes. Managers needing to direct efforts on specific lakes and watersheds would be advised to use more mechanistic models such as BATHTUB (e.g., James et al. Citation2002) or the Soil and Water Assessment Tool (SWAT; Neitsch et al. Citation2002).
Our analyses provides direct evidence of land use disturbances elevating TP in Minnesota lakes to levels that significantly affect fish community structure and function in Minnesota lakes (Schupp and Wilson Citation1993, Heiskary and Wilson Citation2008). We recommend that lake managers consider thresholds of land use disturbance on lake TP levels in efforts to protect and enhance fish habitat in Minnesota lakes.
Acknowledgments
We thank the many contributions made by Minnesota DNR and PCA staff involved with the collection and maintenance of extensive datasets. We also benefited from comments and statistical guidance provided by Donald Pereira and David Staples and from reviews provided by Barry Moore and Rich Wildman. This research was funded in part by the Federal Aid in Sport Fish Restoration program.
References
- Alexander , R B , Smith , R A and Schwarz , G E . 2004 . Estimates of diffuse phosphorus sources in surface waters of the United States using a spatially referenced watershed model . Water Sci Technol. , 49 ( 3 ) : 1 – 10 .
- Barr Engineering . 2004 . “ Detailed assessment of phosphorus sources to Minnesota watersheds ” . St. Paul , MN : Report to the Minnesota Pollution Control Agency .
- Breiman , L. 2001 . Statistical modeling: the two cultures . Stat Sci. , 16 ( 3 ) : 199 – 231 .
- Brett , M T and Benjamin , M M . 2008 . A review and reassessment of lake phosphorus retention and the nutrient loading concept . Freshw Biol. , 53 : 194 – 211 .
- Brezonik , PL. 2002 . “ Cumulative impacts of development in lakes in the north central hardwoods forest ecoregion of Minnesota: and exploratory study ” . Minneapolis , MN : University of Minnesota Water Resources Center Technical Report 144 .
- Carpenter , S R , Caraco , N F , Correll , D L , Howarth , R W , Sharpley , A N and Smith , V H . 1998 . Nonpoint pollution of surface waters with phosphorus and nitrogen . Ecol Appl. , 8 : 559 – 568 .
- Catherine , A , Mouillot , D , Escoffier , N , Bernard , C and Troussellier , M . 2010 . Cost effective prediction of the eutrophication status of lakes and reservoirs . Freshw Biol. , 55 : 2425 – 2435 .
- Cheruvelil , K S , Soranno , P A , Bremigan , M T , Wagner , T and Martin , S L . 2008 . Grouping lakes for water quality assessment and monitoring: The roles of regionalization and spatial scale . Environ Manage. , 41 ( 3 ) : 425 – 440 .
- Clark , B J , Paterson , A M , Jeziorski , A and Kelsey , S . 2010 . Assessing variability in total phosphorus measurements in Ontario lakes . Lake Reservoir Manage. , 26 : 63 – 72 .
- Cleland , D T , Avers , P E , McNab , W H , Jensen , M E , Bailey , R G , King , T and Russell , W E . 1997 . “ National Hierarchical Framework of Ecological Units ” . Edited by: Boyce , M S and Haney , A . 181 – 200 . New Haven , CT : Yale University Press . Ecosystem management applications for sustainable forest and wildlife resources
- Crosbie , B and Chow-Fraser , P . 1999 . Percentage land use in the watershed determines the water and sediment quality of 22 marshes in the Great Lakes basin . Can J Fish Aquat Sci. , 56 : 1781 – 1791 .
- Cross , T K and McInerny , M C . 2005 . Spatial habitat dynamics affecting bluegill abundance in Minnesota bass-panfish lakes . N Am J Fish Manage. , 25 : 1052 – 1066 .
- Crowder , A A , Smol , J P , Dalrymple , R , Gilbert , R , Mathers , A and Price , J . 1996 . Rates of natural and anthropogenic change in shoreline habitats in the Kingston Basin, Lake Ontario . Can J Fish Aquat Sci. , 53 ( S1 ) : 121 – 135 .
- Cummins , J F and Grigal , D F . 1981 . “ Legend to map soils and land surfaces of Minnesota 1980 ” . St. Paul , MN : University of Minnesota Department of Soil Science Soil Series No. 110 . Miscellaneous Publication 11
- Drake , M T and Pereira , D L . 2002 . Development of a fish-based index of biotic integrity for small inland lakes in central Minnesota . N Am J Fish Manage. , 22 : 1105 – 1123 .
- Endreny , T A and Wood , E F . 2003 . Watershed weighting of export coefficients to map critical phosphorus loading areas . J Am Water Resour As. , 39 : 165 – 181 .
- Hasler , AD. 1947 . Eutrophication of lakes by domestic drainage . Ecology. , 28 : 383 – 395 .
- Heathwaite , AL. 2010 . Multiple stressors on water availability at global to catchment scales: understanding human impact on nutrient cycles to protect water quality and water availability in the long term . Freshw Biol. , 55 : 241 – 257 .
- Heiskary , S A , Swain , E B and Edlund , M B . 2004 . “ Reconstructing historical water quality in Minnesota lakes from fossil diatoms ” . St. Paul , MN : Minnesota Pollution Control Agency Environmental Bulletin Number 4 . [cited 4 May 2012]. Available from http://www.pca.state.mn.us/index.php/view-document.html?gid=11453
- Heiskary , S A , Wilson , C B and Larsen , D P . 1987 . Analysis of regional patterns in lake water quality: using ecoregions for lake management in Minnesota . Lake Reservoir Manage. , 3 : 337 – 344 .
- Heiskary , S A and Wilson , C B . 2005 . “ Minnesota lake water quality assessment report: developing nutrient criteria ” . St. Paul , MN : Minnesota Pollution Control Agency . [cited 4 May 2012]. Available from http://www.pca.state.mn.us/index.php/view-document.html?gid=6503
- Heiskary , S A and Wilson , C B . 2008 . Minnesota's approach to lake nutrient criteria development . Lake Reservoir Manage. , 24 : 282 – 297 .
- Homer , C , Huang , C , Yang , L , Wylie , B and Coan , M . 2004 . Development of a 2001 National Land Cover Database for the United States . Photogram Eng Rem S. , 70 : 829 – 840 .
- Hoyer , M V and Canfield , D E Jr . 1996 . Largemouth bass abundance and aquatic vegetation in Florida Lakes: an empirical analysis . J Aquat Plant Manage. , 34 : 23 – 32 .
- James , W F , Barko , J W , Eakin , H L and Sorge , P W . 2002 . Phosphorus budget and management strategies for an urban Wisconsin lake . Lake Reservoir Manage , 18 : 149 – 163 .
- Johnston , C A and Shmagin , B A . 2006 . “ Scale issues in lake-watershed interactions: Assessing shoreline development impacts and water clarity ” . Edited by: Wu , J , Jones , K B , Li , H and Loucks , O L . 297 – 313 . Netherlands : Springer . Scaling and uncertainty analysis in ecology: methods and applications
- Jones , J R , Knowlton , M F , Obrecht , D V and Cook , E A . 2004 . Importance of landscape variables and morphology on nutrients in Missouri reservoirs . Can J Fish Aquat Sci. , 61 : 1503 – 1512 .
- Jones , J R , Knowlton , M F and Obrecht , D V . 2008 . Role of land cover and hydrology in determining nutrients in mid-continent reservoirs: implications for nutrient criteria and management . Lake Reservoir Manage. , 26 : 1 – 9 .
- Krysel , C , Boyer , E M , Parson , C and Welle , P . 2003 . “ Lakeshore property values and water quality: Evidence from property sales in the Mississippi Headwaters Region ” . Walker , MN : Mississippi Headwaters Board . [cited 4 may 2012]. Available from http://www.friendscvsf.org/bsu_study.pdf
- Latta , WC. 1995 . “ Distribution and abundance of the lake herring (Coregonus artedii) in Michigan ” . Lansing , MI : Michigan Department of Natural Resources Fisheries Division . Research Report Number 2014
- Liaw , A and Wiener , M . 2002 . Classification and Regression by randomForest . R News. , 2 ( 3 ) : 18 – 22 .
- Liaw , A and Wiener , M . 2010 . “ Breiman and Cutler's random forests for classification and regression version 4.6–2 ” . [cited 4 May 2012]. Available from http://cran.r-project.org/web/packages/randomForest/randomForest.pdf
- Moyle , JB. 1956 . Some aspects of the chemistry of Minnesota surface waters and wildlife management . J Wildlife Manag. , 20 : 303 – 320 .
- [MNDNR] Minnesota Department of Natural Resources . 2011 . MNDNR data deli; [cited 4 May 2012]. Available from http://deli.dnr.state.mn.us/index.html
- Neitsch , S L , Arnold , J G , Kiniry , R R , Williams , J R and King , K W . 2002 . “ Soil and water assessment tool theoretical documentation ” . College Station , TX : Texas Water Resources Institute . Report TR-191
- Nürnberg , GK. 1995 . The anoxic factor, a quantitative measure of anoxia and fish species richness in central Ontario lakes . Trans Am Fish Soc. , 124 : 677 – 686 .
- Olden , J D , Lawler , J J and Poff , N L . 2008 . Machine learning methods without tears: a primer for ecologists . Q Rev Biol. , 83 : 171 – 193 .
- Omernik , JM. 1987 . Ecoregions of the continuous United States . Ann Assoc Am Geogr. , 77 : 118 – 125 .
- Omernik , JM. 2004 . Perspectives on the nature and definitions of ecological regions . Environ Manage. , 34 : 27 – 38 .
- Omernik , J M , Chapman , S S , Lillie , R A and Dumke , R T . 2000 . Ecoregions of Wisconsin . T Wis Acad Sci. , 88 : 77 – 103 .
- Omernik , J M , Larsen , D P , Rohm , C M and Clarke , S E . 1988 . Summer total phosphorus in lakes: A map of Minnesota, Wisconsin, and Michigan, USA . Environ Manage. , 12 : 815 – 825 .
- Prasad , A M , Iverson , L R and Liaw , A . 2006 . Newer classification and regression tree techniques: bagging and random forests for ecological prediction . Ecosystems. , 9 : 181 – 99 .
- R Development Core Team . 2011 . “ R: A language and environment for statistical computing. R Foundation for Statistical Computing ” . Vienna , , Austria [cited 29 October 2012]. Available from http://www.R-project.org/
- Ramstack , J M , Fritz , S C and Engstrom , D R . 2004 . Twentieth century water quality trends in Minnesota lakes compared with presettlement variability . Can J Fish Aquat Sci. , 61 : 561 – 576 .
- Reckhow , K H and Simpson , J T . 1980 . A procedure using modeling and error analysis for the prediction of lake phosphorus concentration form land use information . Can J Fish Aquat Sci. , 37 : 1439 – 1448 .
- SAS . 2010 . “ JMP 9 modeling and multivariate methods ” . Cary , NC : SAS Institute Incorporated .
- Schupp , D and Wilson , B . 1993 . Developing lake goals for water quality and fisheries . Lakeline. , 13 ( 4 ) : 18 – 21 .
- Smith , R A , Schwarz , G E and Alexander , R B . 1997 . Regional interpretation of water-quality monitoring data . Water Resour Res. , 33 : 2781 – 2798 .
- Soranno , P A , Cheruvelil , K S , Stevenson , R J , Rollins , S L , Holden , S W , Heaton , S and Torng , E K . 2008 . A framework for developing ecosystem-specific nutrient criteria: integrating biological thresholds with predictive modeling . Limnol Oceanogr. , 53 : 773 – 787 .
- Soranno , P A , Hubler , S L , Carpenter , S R and Lathrop , R C . 1996 . Phosphorus loads to surface waters: a simple model to account for spatial pattern of land use . Ecol Appl. , 6 : 865 – 878 .
- Taranu , Z E and Gregory-Eaves , I . 2008 . Quantifying relationships among phosphorus, agriculture, and lake depth at an inter-regional scale . Ecosystems. , 11 : 715 – 725 .
- Tammi , J , Lappalainen , A , Mannio , J , Rask , M and Vuorenmaa , J . 1999 . Effects of eutrophication on fish and fisheries in Finnish lakes: a survey based on random sampling . Fish Manag Ecol. , 6 : 173 – 186 .
- Umbanhowar , C E Jr , Engstrom , D R and Bergman , E C . 2003 . Reconstructing eutrophication and phosphorus loading for Lake Volney, Minnesota: combining lake sediments and land-use history to establish ‘natural’ baselines for management and restoration . Lake Reserv Manage. , 19 : 364 – 372 .
- [USFWS] United States Fish and Wildlife Service . 2002 . “ 2001 National Survey of Fishing, Hunting, and Wildlife-Associated Recreation ” . Washington , DC