Abstract
Aquatic habitat in littoral environments can be affected by residential development of shoreline areas. We evaluated the relationship between macrophyte richness metrics and shoreline development to quantify indicator response at 2 spatial scales for Minnesota lakes. First, the response of total, submersed, and sensitive species to shoreline development was evaluated within lakes to quantify macrophyte response as a function of distance to the nearest dock. Within-lake analyses using generalized linear mixed models focused on 3 lakes of comparable size with a minimal influence of watershed land use. Survey points farther from docks had higher total species richness and presence of species sensitive to disturbance. Second, between-lake effects of shoreline development on total, submersed, emergent-floating, and sensitive species were evaluated for 1444 lakes. Generalized linear models were developed for all lakes and stratified subsets to control for lake depth and watershed land use. Between-lake analyses indicated a clear response of macrophyte richness metrics to increasing shoreline development, such that fewer emergent-floating and sensitive species were correlated with increasing density of docks. These trends were particularly evident for deeper lakes with lower watershed development. Our results provide further evidence that shoreline development is associated with degraded aquatic habitat, particularly by illustrating the response of macrophyte richness metrics across multiple lake types and different spatial scales.
The increasing presence of homes on lake shorelines and the associated alteration of aquatic habitat have been extensively documented in the last 20 years (Bryan and Scarnecchia Citation1992, Christensen et al. Citation1996, Radomski and Goeman Citation2001, Jennings et al. Citation2003, Gaeta et al. Citation2011). The potential effects of shoreline development are of concern because littoral areas in freshwater lakes represent critical habitat, such as nursery and spawning areas for fish (Reed and Pereira Citation2009), structure for aquatic invertebrates (Tolonen et al. Citation2001, Jurca et al. Citation2012), and production areas for waterfowl (Lauridsen et al. Citation1993, Knapton and Petrie Citation1999). Macrophyte-dominated littoral areas also promote the maintenance of a clear-water stable state by improving water clarity through sediment retention, filtering nutrients, and dampening physical effects of wave action (Scheffer et al. Citation1993). Residential development of shoreline areas is often accompanied by reduced aquatic vegetation and changes in water quality as homeowners alter nearshore areas to create more desirable shoreline property (Christensen et al. Citation1996, Radomski and Goeman Citation2001, Jennings et al. Citation2003, Marburg et al. Citation2006). The potential effects of shoreline development may be most pronounced near docks as homeowners clear vegetation and woody structure for access (Lepore Citation2013). These activities are often regulated by resource agencies that legally permit homeowners to remove vegetation within a given area, either by physical or chemical means. Permitted activities are typically nonselective toward individual species, although highly visible growth forms (e.g., emergent and floating-leaf or tall submersed species) may be particularly vulnerable given their prominence in nearshore areas (Radomski and Goeman Citation2001, Lepore Citation2013). A greater understanding of the effects of residential development at different spatial scales and on the structure of macrophyte communities could greatly improve the protection of shoreline habitat.
The potential effects of shoreline development can be evaluated with biological indicators responsive to anthropogenic stressors and also have ecological significance within the larger community. Aquatic macrophytes, in particular, are responsive to the effects of shoreline development (Radomski and Goeman Citation2001, Jennings et al. Citation2003, Keville Citation2013, Lepore Citation2013) and provide critical ecological services (Scheffer et al. Citation1993, Jeppesen et al. Citation1998). A variety of techniques have been developed in recent years that show the utility of macrophytes as biological indicators (Beck et al. Citation2010, Radomski and Perleberg Citation2012). Species richness may be the most practical indicator because it can be determined with relatively minimal effort and is responsive to changes in lake condition (Rorslett Citation1991, Sass et al. Citation2010, Radomski and Perleberg Citation2012). Biological indicators may also be responsive to environmental changes at multiple spatial scales (Niemi and McDonald Citation2004).
Most evaluations of shoreline development have focused on a relatively small number of lakes within a limited geographical area and have not extensively evaluated biological indicators or community response in different spatial contexts (e.g., Bryan and Scarnecchia Citation1992, Reed and Pereira Citation2009). Cumulative and incremental alterations of the shoreline at individual sites may induce lake-wide changes in environmental condition (Jennings et al. Citation2003, Scheuerell and Schindler Citation2004). Evaluations of shoreline development should consider large sample sizes that capture a gradient of different lake types in addition to site-level comparisons to evaluate the range of indicator responses to shoreline development at different scales. Predictive models describing the effects of shoreline development should also consider confounding factors that influence macrophyte community composition, such as the effects of watershed-based stressors or depth-based mediation of trophic state (Cross and Jacobson Citation2013). Macrophyte communities may indicate declines in submersed species richness related to eutrophication (Søndergaard et al. Citation2013), whereas emergent and floating-leaf species may be more affected by shoreline development (Radomski and Goeman Citation2001, Jennings et al. Citation2003, Keville Citation2013, Lepore Citation2013). The interactive effects of multiple stressors, as well as natural variation in community response, could be evaluated as a basis for prioritizing stressor mitigation activities.
The goal of this study was to evaluate the response of littoral macrophyte assemblages to shoreline development by considering appropriate indicators and different spatial scales. We characterized the response of macrophyte richness metrics within and between lakes by evaluating spatial scales at which shoreline development may significantly alter community composition. The richness of macrophyte growth forms, such as the number of emergent and floating-leaf species, were evaluated for their potential utility as biological indicators. Richness measurements were our sole measure of community response because of the ability to evaluate patterns across multiple lake types. First, we evaluated within-lake effects of shoreline development on 3 lakes using the response of richness metrics at individual survey points to distance from the nearest dock. Richness measurements were used for the within-lake analyses to maintain comparability with the second analysis. Second, we evaluated between-lake effects of shoreline development on a set of 1444 lakes that captured a gradient of anthropogenic stressors and limnological characteristics. For all analyses, docks and other artificial structures on the water (hereafter docks) were used as proxy measures of the intensity of shoreline development on ecosystem function. Although docks may locally inhibit plant growth through reduced light availability directly underneath a structure (Burdick and Short Citation1999), the primary effects of shoreline development may be caused by land alteration in riparian areas or direct removal of vegetation by homeowners (Keville Citation2013). Dock density can be correlated with riparian area development (e.g., lawns or vegetation removal) or recreational activity and may be a useful indicator of shoreline alteration (Radomski et al. Citation2010, Wehrly et al. Citation2012).
Methods
Within-lake effects of dock
Within-lake effects of docks on macrophyte richness metrics were evaluated using surveys for 3 mesotrophic lakes near Saint Paul, Minnesota: Christmas (Hennepin County) and Jane and Square (Washington County). Each lake is similar in size (∼100 ha), maximum depth (∼20 m), and species composition (primarily hardwater flora; Moyle Citation1956). Secchi depth measurements based on 10-year averages of summer samples were 6 m for Christmas, 4 m for Jane, and 5 m for Square (MNDNR Citation2013). Percent littoral area of each lake is approximately 29% for Christmas, 68% for Jane, and 32% for Square (MNDNR Citation2013). Point-intercept surveys (Madsen Citation1999) were conducted in late summer 2008 for Christmas and Jane lakes and late summer 2006 for Square Lake. Macrophytes were sampled using a double-sided rake in a grid of evenly spaced points in the littoral zone. The littoral zone was defined as the area (or percentage) of the lake where macrophytes were expected to grow (i.e., euphotic zone). Sample points were added during the survey if this area did not adequately cover the true littoral zone, although additions were minimal. Point density was approximately 7.8 points per littoral hectare (pts/ha) for Christmas and 8.1 pts/ha for both Jane and Square lakes, which was approximately 40 m between points. The point density in each lake was sufficient to obtain an accurate estimate of species richness (see Fig. 5 in Beck et al. Citation2010) and was considered adequate sampling effort for point intercept surveys (Mikulyuk et al. Citation2010). The side of the boat for each rake toss was consistent, and one toss sampled 1 m2. The rake was retrieved after reaching the lake bottom at each point. Depth and species presence/absence were measured at each survey point (; ). Coefficients of conservatism (C, from Milburn et al. Citation2007) were assigned to all species, and those with C values >7 were considered sensitive to disturbance. C values range from 0 to 10 and describe a generic measure of sensitivity as the estimated probability that a plant is likely to occur in a landscape believed to be relatively unaltered from presettlement conditions (Nichols Citation1999). The distance of each point to the nearest dock was quantified as a primary measure of the potential effects of shoreline development (; ).
Table 1 Summary of variables used in within-lake analyses for survey points (n = 1007) in 3 lakes. Response variables for each model included richness or presence of total, submersed, and sensitive species. Distance to the nearest dock and depth at each point were used as explanatory variables.
Figure 1 Illustration of approach used to evaluate the potential within-lake effects of docks using Jane lake (Washington County). Size of each survey point is proportional to species richness. Lake depth (m) is indicated by bathymetric shading. Docks are indicated along the shoreline with dashed lines indicating the distance and direction to the nearest dock for each point (color figure available online).
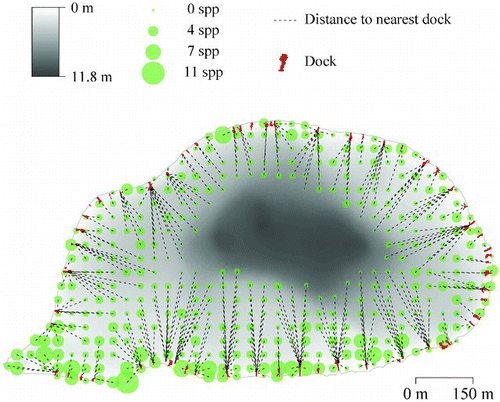
Generalized linear mixed models (GLMM) were used to determine whether the distance to the nearest dock had a significant effect on macrophyte response variables for each survey point (Zuur et al. Citation2009). Models also included depth at each point as an explanatory variable to account for direct and indirect effects on macrophytes. GLMM were used to model linear fixed effects of predictors and a random lake effect. The advantage of this approach is that between-lake variation among richness metrics are excluded from the variance component, and the primary effects of distance to nearest dock may be more easily distinguished. Model selection used an approach where the optimal random component of each model was determined (random intercept and slope, random intercept, no effect), followed by identification of the optimal structure of fixed effects (chapter 5 in Zuur et al. Citation2009). Backward stepwise selection based on minimum Akaike Information Criterion (AIC) was used to identify the most parsimonious models (Akaike Citation1973).
Total and submersed species richness and sensitive species presence/absence at each point were evaluated as response variables for 3 different models. Richness of emergent and floating species was not evaluated because few species of these growth forms were observed in the study lakes. Total and submersed species richness were evaluated as Poisson random variables (logarithmic link). Survey points rarely had more than one sensitive species; therefore, sensitive species richness was evaluated as presence/absence using a binomial random variable (logarithmic odds link). Models that evaluated submersed species richness included total species richness as an offset variable to account for different total richness at each point (Fox and Weisberg Citation2011). Submersed species richness was highly correlated with total species richness, and we considered an evaluation of the relative proportion of total species richness attributed to submersed species to be more informative than models that did not include an offset. None of the offset variables had parameter estimates significantly different from one.
Three linear mixed-effects models were developed (one for each response variable) that included explanatory variables as additive and interactive effects for distance of each point to the nearest structure and point depth, a random lake effect (as a categorical predictor), and an offset variable for total species richness when appropriate. The general notation for a mixed effects model of a Poisson-distributed response variable is (following Zuur et al. Citation2009):
where μ i is the expected value of the response variable Y (richness metric) for observation i, determined as a function of fixed effects (q variables) and a random effect (for one variable with j levels, varying as a function of predictor Z). The vector η defines the associated parameters (α, β1, …, β q ) for the fixed effects (distance and depth), and a and b define the parameters for the random component not included in the mean response (indicating a random intercept and slope model).
The potential correlation between point depth and distance to nearest structure may prevent the use of statistical models to describe the precise effects of shoreline development within lakes. For example, Pearson's product-moment correlations between depth and distance were significant for Jane (r = 0.82) and Square (r = 0.58) lakes; however, preliminary analyses suggested this relationship may not substantially influence the results. Initial models were developed that focused on points close to shore to remove the confounding effect of depth. Initial results were similar using only close survey points as compared to using all survey points. Thus, we have developed models using all survey points based on the assumption that the correlation between depth and distance was insufficient to influence the results.
Model residuals were examined to assess patterns, variance, and outliers. Additionally, model performance was evaluated using the R2 value of the linear fit between the observed and fitted values (pseudo-R2 ; Tuerlinckx et al. Citation2006, Edwards et al. Citation2008). Effects plots (Fox and Weisberg Citation2011) were used to explain the specific effects of relevant explanatory variables for specific models. Effects plots allow an interpretation of the effects of a single explanatory variable (usually high-order terms) on a response by calculating the fitted values of an estimated model across the range of values for the variable while holding all other variables constant.
Finally, spatial correlation among survey points for each lake was evaluated to determine whether a correlation structure was required for model development (e.g., Dale et al. Citation2002, Perry et al. Citation2002). Point intercept surveys use a systematic grid of evenly spaced points to sample the presence/absence of species at each point. The potential for similar measurements of species richness between points that occur near one another may require modeling techniques that control for spatial autocorrelation. Moreover, the surveys for each of the lakes had point densities of approximately 8 pts/ha, indicating that spatial correlation may be likely. The residuals of a best-fitting model for each response variable were examined for each lake using spherical semivariograms (Bivand et al. Citation2008). Evidence for spatial correlation among survey points was determined by comparing the semivariogram model for each lake to bootstrapped null models created by calculating semivariance from randomized residual values (n = 1000 randomizations; Bivand et al. Citation2008). Semivariogram models that differed from the null models were considered evidence for spatial correlation. All analyses were conducted using R statistical software (RDCT Citation2013).
Between-lake effects of docks
Between-lake effects of docks relative to macrophyte richness metrics were evaluated using surveys from the Minnesota Department of Natural Resources (MNDNR). The dataset included macrophyte surveys for 1444 lakes throughout Minnesota (), collected in early July to late August from 1992 to 2003 (Reschke et al. Citation2006). Surveys were conducted using a modified belt transect method with transects perpendicular to the shoreline and extending to the maximum depth of plant growth (MNDNR Citation1993). Transect number varied with lake size (10–50), with larger lakes having more transects. Macrophyte species within 10 feet of each side of the boat were visually identified and categorized by growth form and sensitivity as in the previous section. Macrophytes were sampled with a double-sided rake when closer inspection was needed (MNDNR Citation1993). Species presence and absence were recorded for each transect to obtain a lake-wide measure of species richness.
Figure 2 Locations of 1444 lakes used to evaluate effects of shoreline development between lakes. Locations are color-coded to indicate groups used in the stratified analyses: (1) shallow lakes with low watershed development (n = 439); (2) deep lakes with low watershed development (n = 414); (3) shallow lakes with high watershed development (n = 352); and (4) deep lakes with high watershed development (n = 239). Point size represents the estimated dock density as a continuous variable from 0 to 31.2 docks/km of shoreline (color figure available online).
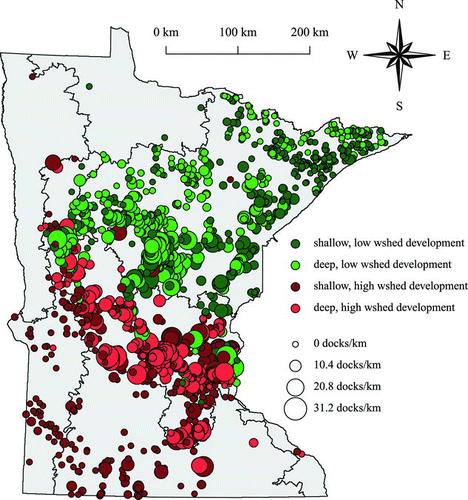
Generalized linear models (GLM) were used to evaluate potential effects of docks between lakes (Venables and Ripley Citation2002, Zuur et al. Citation2009). Four response variables at each lake were examined: total, submersed, emergent and floating-leaf, and sensitive species richness. Emergent and floating-leaf species (hereafter emergent-floating) may be useful indicators of shoreline development because of preferential removal by homeowners (Radomski and Goeman Citation2001, Jennings et al. Citation2003). All response variables were modeled using a Poisson distribution (log-link). Models that evaluated submersed, emergent-floating, and sensitive species included total species richness (log-transformed) as an offset variable, as described in the previous section.
Explanatory variables included dock density (docks/km of shoreline, log-transformed), surface area (ha, log-transformed), maximum depth (m, log-transformed), trophic state index (TSI), and the proportion of watershed land use as urban, agricultural, or forested (). These variables represent natural lake characteristics and human-induced stressors hypothesized to influence aquatic plant assemblages. Although additional variables could help explain variability among macrophyte richness metrics (e.g., alkalinity), the current variables were chosen based on data availability. Dock density was obtained using image analysis techniques to extract dock polygons from high-resolution aerial photos (Beck et al. Citation2013). Surface area and maximum depth were obtained from Geographical Information System shapefiles that described lake bathymetry (MNDNR Citation2012). TSI data were determined primarily from water transparency measurements (MNPCA Citation2012) and methods in Carlson (Citation1977) such that:
Table 2 Summary of variables used in between-lake analyses for all lakes (n = 1444). Response variables for each analysis included total, submersed, emergent-floating, and sensitive species richness. All other variables represent explanatory variables.
Model development for each of the 4 response variables followed a backward stepwise selection approach, including all second-order interactions between variables with AIC selection (Akaike Citation1973, Venables and Ripley Citation2002, Fox and Weisberg Citation2011). Models were validated as described earlier. Model performance was evaluated using the explained deviance, calculated as the difference between the null and the residual deviance, divided by the null deviance (Zuur et al. Citation2009). Lastly, effects plots were used to illustrate the specific effects of explanatory variables on response variables for relevant models.
Species richness is affected by natural and anthropogenic factors that act across different spatial contexts (Rorslett Citation1991, Sass et al. Citation2010). We used 2 approaches for model development to account for the influence of multiple variables. First, we evaluated the entire dataset of 1444 lakes and included all explanatory variables. Second, we developed models using stratified subsets of the entire dataset to control for lake depth and watershed development (). Stratification of the dataset produced 4 groups of lakes: (1) shallow lakes with low watershed development (n = 439); (2) deep lakes with low watershed development (n = 414); (3) shallow lakes with high watershed development (n = 352); and (4) deep lakes with high watershed development (n = 239). A maximum depth of 10 m was used as a threshold because glacial lakes with maximum depths >10 m are typically dimictic, whereas shallower lakes are polymictic. This threshold describes lakes with different rates of internal nutrient loading caused by sediment resuspension during mixing (Cross and Jacobson Citation2013). The development threshold was defined as the summed percentage of watershed land use as urban and agriculture, such that lakes with >25% had developed watersheds, and lakes <25% had undeveloped watersheds. This threshold is used by MNDNR to identify lake protection/restoration priorities (T. Cross, MNDNR, Jan 2013, pers. comm.). Depth and land use were not used as explanatory variables in the models for the stratified datasets. Twenty models were developed: 16 for the 4 stratified subsets (4 response variables per subset) and 4 for the entire dataset (one for each response variable). Models were identical to Equationequation 1 except random effects were not included.
Results
Within-lake effects of docks
Data summary and model performance
Of the 43 species identified in the 3 study lakes used to evaluate within-lake effects, 28 were submersed and 3 were sensitive (Potamogeton friesii, P. robbinsii, and P. strictifolius). Species richness was 31 for Jane Lake, 29 for Christmas Lake, and 24 for Square Lake. Mean total species richness at each point for all lakes was 2.7, with values ranging from 0 to 11; submersed species was 2.3 ranging from 0 to 10; and sensitive species was 0.3 ranging from 0 to 2 ().
Performance of GLMM varied with pseudo-R2 values from 0.24–0.90 (). Models with the lowest performance evaluated total species richness (pseudo-R2 , 0.24). Models for total species richness also exhibited a partial prediction bias toward higher estimated fitted values relative to observed, as indicated by slopes <1 (0.92). Models that evaluated submersed species indicated exceptional model performance (pseudo-R2 , all 0.90), although performance was only attributed to random components related to lake effects. Models that evaluated sensitive species exhibited moderate performance relative to the other analyses (pseudo-R2 , 0.42). Analyses of spatial dependence indicated that similarity among survey points as a result of spatial correlation was negligible (). Semivariance of residual values by lake overlapped with null estimates of semivariance based on randomized residuals. Spatial correlation within lakes was not observed for any of the 3 models.
Table 3 Optimal GLMMs for within lake analyses to evaluate effects of shoreline development on macrophyte richness metrics (see Fig. 1). Response variables were total and submersed species richness and sensitive species presence/absence. Each model included a random intercept for the categorical lake variable (aj for lake j in Equationequation 1, not shown).
Figure 3 Estimates of semivariance for residuals at survey points for the 3 lakes (Christmas, Jane, Square) used in the within-lake analyses. Estimates were based on residual values for the best model describing total species richness (). Larger points indicate calculated semivariance, whereas smaller points and intervals indicate estimates of mean semivariance and 95% confidence obtained from 1000 randomizations of the residual values. Lines indicate the best estimate of the semivariogram for each lake using a spherical model. Similar results were obtained for submersed and sensitive species.
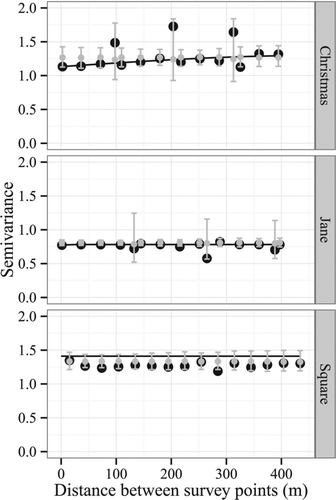
Effects of shoreline development
Evaluation of random effects indicated that a random intercept for each lake was appropriate. The optimal structure of fixed effects varied for each response variable. Models that evaluated total species richness and sensitive species presence/absence included distance to nearest dock as a significant variable, including significant interactions with depth (). For submersed species, no response variables were significant as fixed effects. Distance to the nearest dock was positively related to total species richness and sensitive species presence/absence. Conversely, depth was negatively related to total species richness and positively related to sensitive species presence/absence (). The association of total species richness and sensitive species presence/absence with distance to nearest dock is most evident at shallow depths, with effects observed at distances up to 100 m (similar for sensitive species). Relationships among shoreline development variables () were confirmed by visual assessment of effects plots (not shown).
Between-lake effects of docks
Data summary and model performance
Of the 188 species identified in all lakes used to evaluate between-lake effects, 75 were submersed, 58 emergent-floating, and 43 sensitive. A mean of 20.5 species was found in each lake, and richness ranged from 1 to 64 (). Approximately 50% of the species at each lake were submersed, 33% were emergent-floating, and <15% were sensitive.
Model performance varied for all lakes and stratified subsets of lakes, in addition to variable performance among macrophyte response metrics. The highest performing model was total species richness in all lakes (0.46 explained deviance), and the lowest performing model was emergent-floating species richness in deep lakes with low development (0.05 explained deviance). Analyses that evaluated all lakes generally outperformed models that evaluated subsets of lakes. Additionally, model performance among different response variables indicated that performance decreased sequentially for total (0.27 mean explained deviance among all models), sensitive (0.21), submersed (0.17), and emergent-floating species richness (0.09). These trends were generally consistent regardless of dataset.
Effects of shoreline development
Dock density was included as a significant main effect or interaction in the optimal model for every analysis ( –), excluding sensitive species for shallow lakes with high development () and submersed species for deep lakes with high development (). Models that included dock density as both a main effect and interaction were emergent-floating and sensitive species for all lakes () and total species for lakes with low watershed development ( and ). All other models included dock density only as a main effect. Models included significant main effects for surface area, TSI, and PC1 for total species richness; surface area and TSI for submersed species; TSI and PC1 for emergent-floating species; and surface area, maximum depth, and TSI for sensitive species. Significant interactions were observed among explanatory variables not related to docks.
Table 4 Optimal GLMs for between lake analyses to evaluate effects of shoreline development on macrophyte richness metrics for all lakes (n = 1444). Values indicate parameter estimates and standard errors. Response variables included total, submersed, emergent-floating, and sensitive species richness. Model development for each of the response variables followed a backward stepwise selection approach that began with all explanatory variables and their second-order interactions. Nonsignificant parameters for fixed effects may be included if interactions were observed (Fox and Weisberg Citation2011).
Table 5 Optimal GLMs for between lake analyses to evaluate effects of shoreline development on macrophyte richness metrics for shallow lakes with low watershed development (n = 439). Values indicate parameter estimates and standard errors. Response variables included total, submersed, emergent-floating, and sensitive species richness. Model development for each of the response variables followed a backward stepwise selection approach that began with all explanatory variables and their second-order interactions. Nonsignificant parameters for fixed effects may be included if interactions were observed (Fox and Weisberg Citation2011).
Table 6 Optimal GLMs for between lake analyses to evaluate effects of shoreline development on macrophyte richness metrics for deep lakes with low watershed development (n = 414). Values indicate parameter estimates and standard errors. Response variables included total, submersed, emergent-floating, and sensitive species richness. Model development for each of the response variables followed a backward stepwise selection approach that began with all explanatory variables and their second-order interactions. Nonsignificant parameters for fixed effects may be included if interactions were observed (Fox and Weisberg Citation2011).
Table 7 Optimal GLMs for between lake analyses to evaluate effects of shoreline development on macrophyte richness metrics for shallow lakes with high watershed development (n = 352). Values indicate parameter estimates and standard errors. Response variables included total, submersed, emergent-floating, and sensitive species richness. Model development for each of the response variables followed a backward stepwise selection approach that began with all explanatory variables and their second-order interactions. Nonsignificant parameters for fixed effects may be included if interactions were observed (Fox and Weisberg Citation2011).
Table 8 Optimal GLMs for between lake analyses to evaluate effects of shoreline development on macrophyte richness metrics for deep lakes with high watershed development (n = 239). Values indicate parameter estimates and standard errors. Response variables included total, submersed, emergent-floating, and sensitive species richness. Model development for each of the response variables followed a backward stepwise selection approach that began with all explanatory variables and their second-order interactions. Nonsignificant parameters for fixed effects may be included if interactions were observed (Fox and Weisberg Citation2011).
Dock density generally had a positive effect on total and submersed species richness and a negative effect on emergent-floating and sensitive species richness among subsets of lakes. The specific effects were intensified with decreasing watershed development, however, such that dock density was significantly related to every richness metric for lakes with low watershed development ( and ). Dock effects were marginally higher with increasing lake depth, such that analyses comparing richness metrics in the low watershed development category had slightly stronger relationships with dock density in deeper lakes. Effects plots for deep lakes with low watershed development confirmed a strong association of dock density with richness metrics. The relationship of dock density with total species richness was only relevant for small lakes, with the trend decreasing with increasing lake size. Effects of shoreline development as indicated by effects plots (not shown) were most pronounced up to 5–7 docks/km of shoreline. No interactions were evident for dock density with the other richness metrics in deep lakes with low watershed development.
Discussion
Interpretation of within- and between-lake effects
Research focused on characterizing the effects of shoreline development has consistently described a negative relationship between development and the quality of aquatic habitat (Bryan and Scarnecchia Citation1992, Christensen et al. Citation1996, Radomski and Goeman Citation2001, Gaeta et al. Citation2011). Our analyses corroborate previous research by quantifying a negative response of macrophytes to shoreline development. Specifically, we found that (1) macrophyte richness metrics at individual survey points within lakes indicate predictable responses to distance from the nearest dock, and (2) macrophyte richness metrics between lakes indicate predictable responses to increasing gradients of dock density. The statistical significance of additional covariates that were expected to influence macrophyte assemblage composition indicates the importance of evaluating multiple lake characteristics when developing models with ecological relevance. Although these conclusions generally support previous research, our use of a large dataset and previously unevaluated data on dock location and density greatly increases the confidence in interpretation of effects of shoreline development on aquatic habitat. No other studies to our knowledge have evaluated a comparable dataset using spatially referenced dock data.
The response of macrophyte richness metrics within-lakes indicated significant effects of shoreline development on aquatic habitat. Survey points farther from docks were more likely to have higher species richness and contain sensitive species; however, distance was not related to submersed species richness and contradicts the between-lake analyses that suggested shoreline development may promote higher submersed species richness. Similar studies that examined shoreline development at different spatial scales have produced comparable results. For example, the abundance of submersed vegetation may be related to anthropogenic stressors in the watershed but not in shoreline areas, whereas emergent and floating-leaf vegetation may be related to watershed land use and shoreline development (Jennings et al. Citation2003). Although Jennings et al. (Citation2003) evaluated abundance rather than richness and measured shoreline development as residential housing density, the similarity with our analyses provides evidence that macrophytes are responsive indicators of human-induced changes at different spatial scales.
The relationship of total species richness and sensitive species presence/absence with distance to the nearest dock suggests that shoreline development may have far-reaching effects on littoral habitat. Few studies have quantified the potential range of influence that shoreline development may have on aquatic habitat (Radomski et al. Citation2010). The relationships we found suggest that the effects of shoreline development may extend tens of meters from a dock. Although the confidence intervals in the effects plots are expansive, a continuous decline in total species richness and sensitive species presence/absence was observed across the range of distances, with dramatic declines closer to docks. The significant interaction of distance to dock with depth suggests that the response may only be relevant for littoral areas at shallow depths. This interaction is not trivial and suggests that littoral habitat in shallow areas on undeveloped shoreline may have greater species richness, whereas the opposite may be true for areas near docks. Similar results were observed by Keville (Citation2013) and Lepore (Citation2013) in evaluations of habitat in shallow areas at developed and undeveloped sites.
The between-lake analyses indicated that shoreline development can have lake-wide, cumulative effects on macrophyte assemblages. Emergent-floating and sensitive species richness was negatively associated with increasing shoreline development, whereas total and submersed species richness was positively associated. Our analysis of macrophyte richness metrics considered different growth forms as diagnostic of shoreline development. The response of emergent-floating species to nearshore development suggests that vulnerability to shoreline development could be explained by depth. Emergent and floating-leaf species colonize shallow areas of lakes and are often preferentially removed by homeowners due to shoreline proximity (Radomski and Goeman Citation2001).
The positive association of total and submersed species richness with shoreline development in the between-lake analyses was unexpected and warrants additional research; however, alteration of community structure, regardless of the form of the response, may establish a basis for the development of biological standards. Accordingly, deviation of richness metrics from reference conditions in response to anthropogenic stressors could be used to potentially designate lakes as altered, stressed, or impaired (e.g., Davies and Jackson Citation2006). The observed effects of shoreline development may also be an artifact of the data rather than an ecological relationship; however, the model that evaluated all lakes indicated a negative interaction between lake size and dock density such that the positive association between total species richness and dock density decreased for larger lakes. Thus, larger lakes may be more heavily influenced by shoreline development, and a subsequent decrease in total species richness may actually occur. Alternatively, smaller lakes may exhibit a positive association with shoreline development as a result of nutrient limitation. We observed a slight positive association of lake trophic state with lake size, possibly because the smaller lakes in our dataset may be more nutrient limited. Shoreline development in small lakes may increase nutrient inputs and create more favorable growing conditions for macrophytes. Morever, the relationship of trophic state with species richness may not be linear, such that a unimodal distribution is observed with species richness maximized at moderate nutrient loading (Connell Citation1978). Deep lakes with low watershed development are observed in the northern portion of the state where oligotrophy is more common. Shoreline development may increase species richness in lakes with naturally depauperate plant communities as a result of increased nutrients (Borman et al. Citation2009). Consequently, a more detailed exploration of nonlinear response of macrophyte richness metrics could be potentially informative, such as the inclusion of polynomial terms in existing models or the use of additive smoothing models.
The between-lake effects of shoreline development also varied among different lake types stratified by depth and watershed development. Specifically, shoreline development exhibited the strongest relationships with macrophyte richness metrics for deep lakes that had relatively undeveloped watersheds, with the effects more pronounced in smaller lakes. Our large dataset necessitated the stratification of analyses to control for depth and watershed development based on relationships with trophic state. The finding that macrophyte assemblages in lakes with low watershed development may be more vulnerable to shoreline development suggests watershed characteristics are perhaps larger determinants of macrophyte composition as compared to more localized effects originating from shoreline areas. Alternatively, lakes with high watershed development may be more culturally eutrophic, and the effects of shoreline development may be masked. Watershed development may also be related to hydrologic alterations, such that lakes with highly developed watersheds have more variable hydrology from agricultural or municipal water withdrawal or increased stormwater runoff from impervious surfaces. Emergent-floating species that colonize shallow areas could be more vulnerable than other growth forms to variable hydrology (Moore et al. Citation2012).
Integration of information from both spatial scales could facilitate protection or restoration of shoreline habitat. We suggest that shallow areas and small, deep lakes with low watershed development may be particularly vulnerable to effects of shoreline development. Habitat management could focus on lakes that fit these criteria, in addition to an evaluation of habitat at shallow depths. In the context of our models, effects plots indicated that macrophytes may be affected up to 100 m from a dock, such that one dock could potentially affect 200 m of shoreline. The between-lake results suggest increasing dock density up to 25 docks/km of shoreline could potentially affect whole-lake communities, although the most dramatic changes were observed up to 5–7 docks/km; however, relatively poor model performance (e.g., low R 2, large confidence intervals) suggests that the proposal of specific guidelines for management requires additional research. We present the above information as preliminary information stated within the constraints of the analyses.
Several limitations of our data and analyses should be noted. First, we only evaluated the response of macrophyte richness metrics, whereas other metrics may provide additional information about shoreline development. Most studies evaluating macrophyte response to shoreline development examined relative abundance, biomass, or frequency measurements (Radomski and Goeman Citation2001, Jennings et al. Citation2003, Keville Citation2013, Lepore Citation2013). These metrics may be more useful indicators of shoreline development related to vegetation removal, such that a change in frequency or abundance at the lake-wide scale may be observed, whereas total species richness may not indicate an effect. More specifically, vegetation may be absent at developed sites, but the same species could colonize undeveloped areas without indicating a lake-wide change in richness. Our results indicate that littoral areas farther from structures are more likely to have higher species richness. Furthermore, the between-lake analyses indicated a response of richness metrics at the whole-lake scale, which suggests that a combined and cumulative impact of site-level alterations could induce community-wide changes. Additional research could examine the precise mechanisms of declines of diversity at the whole-lake scale as explained by site-level alterations in frequency and abundance, particularly in relation to species richness. Observed changes in species richness may actually reflect lake-wide changes in abundance and eventual extirpation of individual species within a particular lake. The precise implications for fish and invertebrates should also be examined to determine the extent to which changes in abundance and richness interact to affect multiple trophic levels.
Second, our within-lake analyses evaluated specific lake types. The 3 lakes are small and mesotrophic with relatively small, wooded watersheds and do not characterize the range of lake types throughout the state. Emergent and floating-leaf species were also not observed at sufficient frequencies of occurrence to allow an examination of the relationship with shoreline development in the 3 study lakes. However, mean dock density for all lakes in the ecoregion is approximately 4 docks/km shoreline (Beck et al. Citation2013), and the study lakes have dock densities approximately 3–4 times the average (Christmas 15 docks/km, Jane 17 docks/km, Square 12 docks/km). The low frequency occurrence of emergent-floating vegetation suggests that these species may have been locally extirpated from the study lakes as a result of development.
Third, the explanatory variables used in the between- and within-lake analyses represent a limited set of lake characteristics. Other variables such as shoreline heterogeneity, alkalinity, or watershed size are likely related to richness metrics. For within-lake analyses, bottom substrate, wind exposure, and littoral slope also influence macrophyte distribution. A primary focus of our analysis was the characterization of the effects of shoreline development while maintaining analytical simplicity. The limited set of explanatory variables was considered most relevant for the analyses. Inclusion of additional explanatory variables could improve predictive performance of the models, although we suspect conclusions would generally remain unchanged.
Lastly, the data we evaluated in the between-lake analyses potentially contain biases that could influence interpretation of the results. Surveys were conducted over a period of 10 years, with the latest survey completed in 2003, whereas dock data were obtained from aerial photos taken in 2008. The estimated effects of shoreline development may be conservative given that macrophyte surveys were completed several years prior to the aerial photos. Although data describing temporal trends in development are lacking, shoreline development has generally been considered to be increasing since the 1990s. Additionally, the macrophyte data from Reschke et al. (Citation2006) do not represent a random sample of lakes. Large lakes with managed fisheries are surveyed more often. Misidentification of species is also common, particularly for rare species.
Conclusions and management implications
Our analyses indicate that shoreline development may significantly affect individual sites within lakes and whole-lake macrophyte assemblages. Shallow, littoral areas farther from docks were more likely to have higher species richness and presence of sensitive species, although these results may only apply to small, mesotrophic lakes with undeveloped watersheds. Deeper lakes with low watershed development were particularly vulnerable, such that more shoreline development was associated with fewer emergent-floating and sensitive species. Accordingly, site-level impacts via removal of vegetation may have cumulative lake-wide effects on richness metrics, as well as alter the distribution of species within lakes.
We emphasize that management could focus on the protection of riparian areas and discouragement of aquatic vegetation removal to prevent negative effects of shoreline development. Our analysis using docks as proxy indicators of these stressors indicates that specific control of docks to mitigate shoreline development may be ineffective because docks may not be the primary cause of habitat degradation. The objective of the analysis was to illustrate effects of shoreline development at different spatial scales and to determine structural response of macrophyte communities using richness-based metrics. Accordingly, stewardship of shoreline areas for the protection of water quality and aquatic habitat may focus on minimizing activities that are the primary cause of environmental degradation. Advocating for the preservation of emergent-floating leaf species and minimum maintenance of riparian lawns, rather than an exclusive focus on docks, may be the most effective approach to habitat protection.
The economic implications of shoreline management are apparent; reduced aquatic habitat may have negative effects on lake fisheries, water quality, or residential property values. A precautionary principle may be best because habitat management on lakes with high dock densities may be ineffective. Consistent trends in macrophyte response to shoreline development could also be interpreted in the context of other anthropogenic stressors, particularly watershed land use, which may mask potential effects of shoreline development at the whole-lake scale. Management of degraded macrophyte communities may be most effective if water quality issues are addressed prior to site-level restoration.
Acknowledgments
Thanks to Timothy Cross, Frank Wilhelm, and 4 anonymous reviewers for providing insightful comments that have improved the manuscript, Sanford Weisberg and James Forester for statistical guidance, and Donna Perleberg for providing statewide transect data. Don Pereira and Bruce Wilson provided general project guidance. Funding was provided by the Minnesota Department of Natural Resources using Clean Water Legacy funds appropriated by the Minnesota Legislature and a graduate school fellowship provided to MW Beck from the University of Minnesota. The use of trade names or products does not constitute endorsement by the US Government.
†The Unit is jointly sponsored by the US Geological Survey, the University of Minnesota, the Minnesota Department of Natural Resources, the US Fish and Wildlife Service, and the Wildlife Management Institute.
References
- Akaike , H. 1973 . Information theory and an extension of the maximum likelihood principle. In: Petrov BN, Csaki F, editors. Second international symposium on information theory , 267 – 281 . Budapest , , (Hungary) : Akademiai Kiado .
- Beck , M W , Hatch , L K , Vondracek , B and Valley , R D . 2010 . Development of a macrophyte-based index of biotic integrity for Minnesota lakes . Ecol Indic. , 10 ( 5 ) : 968 – 979 .
- Beck , M W , Vondracek , B , Hatch , L K and Vinje , J . 2013 . Semi-automated analysis of high-resolution aerial images to quantify docks in glacial lakes . ISPRS J Photogramm Remote Sens. , 81 : 60 – 69 .
- Bivand , R S , Pebesma , E J and Gómez-Rubio , V . 2008 . Applied spatial data analysis with R , New York : Springer . NY
- Borman , S C , Galatowitsch , S M and Newman , R M . 2009 . The effects of species immigrations and changing conditions on isoetid communities . Aquat Bot. , 91 ( 3 ) : 143 – 150 .
- Bryan , M D and Scarnecchia , D L . 1992 . Species richness, composition, and abundance of fish larvae and juveniles inhabiting natural and developed shorelines of a glacial Iowa lake . Environ Biol Fishs. , 35 ( 4 ) : 329 – 341 .
- Burdick , D M and Short , F T . 1999 . The effects of boat docks on eelgrass beds in coastal waters of Massachusetts . Environ Manage. , 23 ( 2 ) : 231 – 240 .
- Carlson , RE. 1977 . A trophic state index for lakes . Limnol Oceanogr. , 22 ( 2 ) : 361 – 369 .
- Christensen , D L , Herwig , B R , Schindler , D E and Carpenter , S R . 1996 . Impacts of lakeshore residential development on coarse woody debris in north temperate lakes . Ecol Appl. , 6 ( 4 ) : 1143 – 1149 .
- Connell , JH. 1978 . Diversity in tropical rain forests and coral reefs . Science. , 199 : 1302 – 1310 .
- Cross , T K and Jacobson , P C . 2013 . Landscape factors influencing lake phosphorus concentrations across Minnesota . Lake Reserv Manag. , 29 ( 1 ) : 1 – 12 .
- Dale , M RT , Dixon , P , Fortin , M J , Legendre , P , Myers , D E and Rosenberg , M S . 2002 . Conceptual and mathematical relationships among methods for spatial analysis . Ecography. , 25 ( 5 ) : 558 – 577 .
- Davies , S P and Jackson , S K . 2006 . The biological condition gradient: A descriptive model for interpreting change in aquatic ecosystems . Ecol App. , 16 ( 4 ) : 1251 – 1266 .
- Edwards , L J , Muller , K E , Wolfinger , R D , Qaqish , B F and Schabenberger , O . 2008 . An r2 statistic for fixed effects in the linear mixed model . Stat Med. , 27 ( 29 ) : 6137 – 6157 .
- Fox , J and Weisberg , S . 2011 . An R companion to applied regression , Thousand Oaks : SAGE Publications . CA
- Gaeta , J W , Gaurascio , M J , Sass , G G and Carpenter , S R . 2011 . Lakeshore residential development and growth of largemouth bass (Micropterus salmoides): a cross-lakes comparison . Ecol Freshw Fish. , 20 ( 1 ) : 92 – 101 .
- Homer , C , Dewitz , J , Fry , J , Coan , M , Hossain , N , Larson , C , Herold , N , McKerrow , A , VanDriel , J N and Wickham , J . 2007 . Completion of the 2001 National Land Cover Database for the conterminous United States . Photogramm Eng Remote Sens. , 73 ( 4 ) : 337 – 341 .
- Jennings , M J , Emmons , E E , Hatzenbeler , G R , Edwards , C and Bozek , M A . 2003 . Is littoral habitat affected by residential development and land use in watersheds of Wisconsin lakes? . Lake Reserv Manag. , 19 ( 3 ) : 272 – 279 .
- Jeppesen , E , Søndergaard , M , Søndergaard , M and Christoffersen , K, . 1998 . The structuring role of submerged macrophytes in lakes, Ecological Studies , Vol. 131 , New York : Springer . editors., NY
- Jurca , T , Donohue , L , Laketic , D , Radulovic , S and Irvine , K . 2012 . Importance of the shoreline diversity features for littoral macroinvertebrate assemblages . Fundam Appl Limnol. , 180 ( 2 ) : 175 – 184 .
- Keville , J. 2013 . Effects of residential shoreline development on near shore aquatic habitat in Minnesota lakes. [master's thesis] , [St. Paul , (MN)] : University of Minnesota .
- Knapton , R W and Petrie , S A . 1999 . Changes in distribution and abundance of submerged macrophytes in the inner bay at Long Point, Lake Erie: implications for foraging waterfowl . J Great Lakes Res. , 25 ( 4 ) : 783 – 798 .
- Lauridsen , T L , Jeppesen , E and Andersen , F O . 1993 . Colonization of submerged macrophytes in shallow fish manipulated lake Vaeng: impact of sediment composition and waterfowl grazing . Aquat Bot. , 46 ( 1 ) : 1 – 15 .
- Lepore , J. 2013 . Local and cumulative influences of docks on littoral habitat structure. [master's thesis] , [St. Paul , (MN)] : University of Minnesota .
- Madsen , JD. 1999 . Point intercept and line intercept methods for aquatic plant management. US Army Engineer Center , Vicksburg , (MS) : APCRP Technical notes collection . Technical Report TN APCRP-M1-02
- Marburg , A E , Turner , M G and Kratz , T K . 2006 . Natural and anthropogenic variation in coarse wood among and within lakes . J Ecol. , 94 ( 3 ) : 558 – 568 .
- Mikulyuk , A , Hauxwell , J , Rassmussen , P , Knight , S , Wagner , K I , Nault , M E and Ridgely , D . 2010 . Testing a methodology for assessing plant communities in temperate inland lakes . Lake Reserv Manag. , 26 ( 1 ) : 54 – 62 .
- Milburn , S A , Bourdaghs , M and Husveth , J J . 2007 . Floristic quality assessment for Minnesota wetlands. St , Paul , (MN) : Minnesota Pollution Control Agency . report wq-bwm2-01
- [MNDNR] Minnesota Department of Natural Resources . 1993 . Manual of instructions for lake survey. St , 147 Paul , (MN) : Special publication .
- [MNDNR] Minnesota Department of Natural Resources . 2012 . The DNR Data Deli; [cited 25 Jan 2013] . Available from: http://deli.dnr.state.mn.us/index.html
- [MNDNR] Minnesota Department of Natural Resources . 2013 . LakeFinder website; [cited 20 May 2013] . Available from: http://www.dnr.state.mn.us/lakefind/index.html
- [MNPCA] Minnesota Pollution Control Agency . 2012 . Minnesota Pollution Control Agency, surface water data submittal . review, and reports; [cited 25 Jan 2013]. Available from: http://www.pca.state.mn.us/index.php/water
- Moore , M JC , Romano , S P and Cook , T . 2012 . Synthesis of Upper Mississippi River system submersed and emergent aquatic vegetation: past, present, and future . Hydrobiologia. , 640 ( 1 ) : 103 – 114 .
- Moyle , JB. 1956 . Relationships between the chemistry of Minnesota surface waters and wildlife management . J Wildl Manag. , 20 ( 3 ) : 303 – 320 .
- Nichols , SA. 1999 . Floristic quality assessment of Wisconsin lake plant communities with example applications . J Lake Reserv Manag. , 15 ( 2 ) : 133 – 141 .
- Niemi , G J and McDonald , M E . 2004 . Application of ecological indicators . Annu Rev Ecol Evol Syst. , 35 : 89 – 111 .
- Perry , J N , Liebhold , A M , Rosenberg , M S , Dungan , J , Miriti , M , Jakomulska , A and Citron-Pousty , S . 2002 . Illustrations and guidelines for selecting statistical methods for quantifying spatial pattern in ecological data . Ecography. , 25 ( 5 ) : 578 – 600 .
- Radomski , P , Bergquist , L A , Duval , M and Williquett , A . 2010 . Potential impacts of docks on littoral habitats in Minnesota lakes . Fisheries. , 35 ( 10 ) : 489 – 495 .
- Radomski , P and Goeman , T J . 2001 . Consequences of human lakeshore development on emergent and floating-leaf vegetation abundance . North Am J Fish Manag. , 21 ( 1 ) : 46 – 61 .
- Radomski , P and Perleberg , D . 2012 . Application of a versatile aquatic macrophyte integrity index for Minnesota lakes . Ecol Indic. , 20 : 252 – 268 .
- [RDCT] R Development Core Team . 2013 . R: a language and environment for statistical computing, v2.11.1 , Vienna : R Foundation for Statistical Computing . Austria
- Reed , J R and Pereira , D L . 2009 . Relationships between shoreline development and nest site selection by black crappie and largemouth bass . N Am J Fish Manag. , 29 ( 4 ) : 943 – 948 .
- Reschke , C , Host , G E and Johnson , L B . 2006 . Evaluation of DNR aquatic vegetation surveys: data summaries and comparative analysis , Duluth , (MN) : Natural Resources Research Institute, University of Minnesota, Minnesota Department of Natural Resources .
- Rorslett , B. 1991 . Principal determinants of aquatic macrophyte richness in northern European lakes . Aquat Bot. , 39 ( 1-2 ) : 173 – 193 .
- Sass , L L , Bozek , M A , Hauxwell , J A , Wagner , K and Knight , S . 2010 . Response of aquatic macrophytes to human land use perturbations in the watersheds of Wisconsin lakes, U . S.A. Aquat Bot. , 93 : 1 – 8 .
- Scheffer , M , Hosper , S H , Meijer , M L , Moss , B and Jeppesen , E . 1993 . Alternative equilibria in shallow lakes . Trends Ecol Evol. , 8 ( 8 ) : 275 – 279 .
- Scheuerell , M D and Schindler , D E . 2004 . Changes in the spatial distribution of fishes in lakes along a residential development gradient . Ecosystems. , 7 ( 1 ) : 98 – 106 .
- Søndergaard , M , Phillips , G , Hellsten , S , Kolada , A , Ecke , F , Mäemets , H , Mjelde , M , Azzella , M M and Oggioni , A . 2013 . Maximum growing depth of submerged macrophytes in European lakes . Hydrobiologia. , 704 ( 1 ) : 165 – 177 .
- Tolonen , K T , Hamalainen , H , Holopainen , I J and Karjalainen , J . 2001 . Influences of habitat type and environmental variables on littoral macroinvertebrate communities in a large lake system . Hydrobiologia. , 152 ( 1 ) : 39 – 67 .
- Tuerlinckx , F , Rijmen , F , Verbeke , G and DeBoeck , P . 2006 . Statistical inference in generalized linear mixed models: a review . Brit J Math Stat Psy. , 59 : 225 – 255 .
- Venables , W N and Ripley , B D . 2002 . Modern applied statistics with S. 4th ed , New York : Springer . NY
- Wehrly , K E , Breck , J E , Wang , L and Szabo-Kraft , L . 2012 . Assessing local and landscape patterns of residential shoreline development in Michigan lakes . Lake Reserv Manag. , 28 ( 2 ) : 158 – 169 .
- Zuur , A F , Ieno , E N , Walker , N J , Saveliev , A A and Smith , G M . 2009 . “ Mixed effects models and extensions in ecology with R ” . New York : Springer . NY