ABSTRACT
June-Wells M, Gallagher F, Hart B, Malik V, Bugbee G. 2016. The relative influences of fine and landscape scale factors on the structure of lentic plant assemblages. Lake Reserv Manage. 32:116–131.
The process of plant community assembly has long been a topic of debate among ecologists. Aquatic plant assemblages and their structure may be the result of a series of abiotic and biotic filters that include transport (i.e., physical movement of species), water chemistry, sediment chemistry, basin structure, and competitive interactions. The influences of transport and water chemistry have been well investigated, but many questions persist about the interrelationships among water and sediment variables, including their combined influences on the structure of the aquatic plant assemblage. To understand how these abiotic conditions interact, we sampled 750 points in 30 lakes. Using these data, a split canonical correspondence design was used to evaluate the relative influences of regional and fine-scale conditions in structuring the plant assemblage. Additionally, multiple logistic regressions were employed to determine the individual species’ abiotic preferences. The results suggest that the plant assemblage structure is principally determined by the lake-sediment characteristics. Sediment variables explained 28% of the total species-data variance and water chemistry accounted for 9% (total variance explained = 46%; 9% due to intercorrelation of water/sediment characteristics). There were also strong species–environmental relationships; nonnative species showed distinct correlations with sediment pH and lake water conductivity, which may be useful in lake management initiatives such as risk assessment and nonnative species monitoring. Finally, and as expected, the Shannon diversity index was strongly related to depth and light.
Assembly theory views plant communities as aggregates of species present in an area due to their relationships with environmental conditions, successful recruitment, and competitive ability (Weiher and Keddy Citation1995, Wilson Citation1999). Plant assemblage structure and the inherent oscillations of species vary with abiotic conditions and disturbances but may result in an assemblage composed of species best suited to the local environment (Weiher and Keddy Citation1995, Wilson Citation1999). In this study, we examined the composition of rooted aquatic plant assemblages and the associated abiotic filters to better understand the mechanisms that structure these assemblages in lentic systems.
In 2009, Capers et al. (Citation2009a) examined the influences of lake location, water chemistry conditions, and the dispersal abilities of species on the structure of these assemblages. The relative contributions of lake location and environmental conditions in that study were equal, suggesting interplay between randomness (i.e., transport/colonization) and the water chemistry preferences of species. That study was conducted in Connecticut where abiotic water conditions and lake location co-vary linearly over longitudinal scales (Deevey Citation1940, Frink and Norvell Citation1984, Canavan and Siver Citation1995). In addition, Connecticut is a small state with a total surface area of 5543 km2, likely too small to deter colonization through anthropogenic transport mechanisms (Johnstone et al. Citation1985). Therefore, abiotic filters are probably more important in determining the distribution of aquatic macrophyte species and the structure of these assemblages.
The concept that abiotic factors impact the distribution of plant species and the structure of these assemblages is not new. In the latter part of the 20th century, Tilman (Citation1981) conducted a convincing examination of environmental variation on competitive interactions among species. His work with algae suggested a significant contribution of resource availability to the outcome of competitive interactions and, ultimately, the relative abundances of each species (Tilman Citation1981, Citation1987, Citation2004). Further significant investigation in the realm of plant community assembly and dynamics occurred in the 1990s with the presentation of community assembly under a variety of environmental conditions (Keddy Citation1992, Weiher and Keddy Citation1995). These initiatives argue that the structural nature of plant assemblages is a result of the environmental conditions that form the assemblages (Weiher and Keddy Citation1995, Wilson Citation1999).
Our current understanding of environment–plant relationships in lentic systems stems from the influential research of Kadono (Citation1982a, Citation1982b) and Hellquist (Citation1980) on the distribution of Potamogeton species. These authors showed that the species distribution of this genus relates to the spatial distribution of environmental conditions. Overall, contemporary research suggests that sediment structure (Barko and Smart Citation1986), water chemistry (Hellquist Citation1980, Jackson and Charles Citation1987, Jacobs and MacIsaac Citation2009, Thum and Lennon Citation2010, June-Wells et al. Citation2013), lake size, elevation, and water clarity (Lacoule and Freeman 2006) all influence the structure of aquatic plant assemblages. By including the influence of transport (Johnstone et al. Citation1985, Capers et al. Citation2009a, Citation2009b) in the assessment of lentic plant assemblages, a more malleable theory of aquatic plant community assembly emerges.
The objective of this study was to evaluate the relative influences of water and sediment characteristics on the structure of aquatic plant assemblages. In addition, we included the influences of lake and watershed size, light, bottom slope, and in-lake variations of sediment characteristics. We tested the following traditional hypotheses that concern lentic plant community assembly: (1) that light is a principal variable in explaining all plant assemblage structural features, (2) that diversity is consistently greatest in shallow waters, (3) the more novel idea that geomorphic characteristics describe a significantly greater portion of species data variance when compared to water chemistry, and (4) that in-lake sediment characteristics significantly contribute the distributions of species in lakes.
Materials and methods
We selected 30 Connecticut lakes to analyze the distribution of aquatic macrophyte species and the structure of these assemblages. Lakes were chosen based on the following criteria: (1) surface area >40 ac (>16.19 ha), (2) maximum depth >5 m, and (3) plant community richness ≥13 species, a number based on the mean number of species per lake in the state (The Connecticut Agricultural Experiment Station Database). Overall, the study lakes had areas inside and outside of the littoral zone (as established for Connecticut lakes; i.e., 0.1–5.0 m) and encompassed a wide variety of sizes. All samples were taken between 1 July and 1 September 2011 and 2012. Although there was some variation between years, the large number of samples, careful attention to variance in the analyses, and the use of randomization techniques afforded acceptable confidence limits.
In Connecticut, the eastern section, including the coastal slope, is primarily crystalline in nature (Deevey Citation1940, Canavan and Siver Citation1995) and weather slowly, adding little dissolved solutes to the lake waters. The bedrock of the central and western portions of Connecticut is sedimentary or metamorphosed limestone (Deevey Citation1940, Canavan and Siver Citation1995), which are more soluble in nature and contribute to high specific conductance and alkalinity; these lakes were considered hardwater for the purposes of this study (hardwater = >25 mg/L). The study lakes were distributed throughout all geological formations present within the state.
In each lake, 25 random georeferenced points were established at depths ranging from 0.1 to 5.0 m. These points were organized by depth; 5 depth classes were established (0–1, 1–2, 2–3, 3–4, and 4–5 m), and each class contained 5 points. In addition, no 2 points were closer than 50 m apart. Finally, the lakes’ plant communities were not actively managed during this study.
Table 1. Species encountered during this study.
Table 2. Conditions used in canonical analyses including water and soil variables. Average point diversity is the 25-point average of Shannon diversity index from each.
At each point, the boat was anchored and oriented perpendicular to the nearest shoreline. Slope of the lake bottom was calculated by measuring depth at the front and rear of the boat (a span of 4 m). Sediment samples were taken at the front of the vessel using an Ekman Dredge and stored in 500 mL containers and kept at 3 C until processed in the lab. Sample pH and specific conductance (herein referred to as conductivity) at 25 C were evaluated in a 1:1 by volume sediment/DI-H2O slurry using a Fisher Accumet meter. Organic content was examined by drying the sample at 60 C for 24 h, weighing, and then determining the loss of mass following ignition at 400 C for 24 h. Light reaching the bottom at each point was calculated using the following formula:
(1)
where Iz = intensity at depth z, e is Euler's number, k is the attenuation coefficient of light in water (1.7) multiplied by the summer Secchi depth recorded in the deepest site within each lake, z is the depth, and Io is the solar constant (340 w/m2).
Water samples were taken at a depth of 0.5 m in the area of maximum lake depth and stored at 3 C until analyzed for alkalinity (buffering capacity), conductivity, pH, and total phosphorus. Conductivity and pH were measured with a Fisher-Accumet AR20 meter. Alkalinity was quantified by titration and expressed as mg/L CaCO3. The titrant was 0.16N H2SO4 with an end point of pH 4.5 (Eaton et al. Citation1995). Total phosphorus analysis was conducted on acid-stabilized (H2SO4) lake water using the ascorbic acid and potassium persulfate digestion method (Eaton et al. Citation1995). Within the target lakes, aqueous conductivity has been shown to have a 90% correlation with calcium concentration, and aqueous phosphorus exhibits a 75% correlation with total nitrogen (Frink and Norvell Citation1984). Finally, these lakes are generally phosphorus limited; therefore, we were confident that our parameters were the most critical.
The plant assemblage was sampled using a grapple tied to a 20 m line. At each point, 4 grapple tosses (2 to each side of the boat) were conducted by throwing the grapple parallel to the nearest shore out to the full length of the line. Plant species were identified on site, and species abundances were described using a rank abundance technique by 2 independent observers. Species were given a rating of 1 for rare, 2 for present but not abundant, 3 for abundant but not dominant, 4 for dominant, or 5 for dense monoculture. Point diversity was calculated using Shannon diversity index (H’):
(2)
where pi is the fraction of the community each species represents, S is the total lake richness, and N is the total abundance at each point (Magurran Citation1988). Specimens of each sampled species were preserved and retained in the CAES Herbarium.
Data treatment
Because one midsummer water sample was taken for each lake and 25 points were used to evaluate the sediment/basin characteristics and the plant community, it was necessary to unite these 2 data matrices using the lake averages of the measured sediment/basin variables (note: median values were tested and no difference in the analyses were found). Furthermore, to characterize the in-lake variation of the variables, the standard deviations of the raw values (n = 25) were divided by their mean (i.e., the coefficient of variation). These metrics represent the average sediment/basin conditions and how much they vary throughout the waterbody.
To construct the species matrix, we created 2 variables for each species from the raw data. The first variable, total rank abundance of each species in each lake, was calculated by taking the sum of the individual species ranks at all 25 points in each lake (max = 125). The second variable constructed from those data, frequency of occurrence of each species, was calculated by taking the sum of all presence/absence data for each species at all 25 points (max = 25) in each lake. These 2 metrics are important in characterizing the plant assemblage because the first represents the abundance of each species and the second describes whether each species is clustered or diffusely distributed throughout the waterbody.
The process resulted in 3 different data matrices, each 30 rows (lakes) in length. The “Water Chemistry” matrix included lake size (ac), watershed area (mi2), and the water chemistry variables alkalinity (mg/L), conductivity (μS/cm), pH, and total phosphorus (μg/L). Lake size and watershed area were included in that matrix because the relationship between the 2 has a distinct impact on water chemistry (Søndergaard Citation2007). The “Sediment/Basin” matrix included light reaching the bottom (w/m2), bottom slope (%), soil pH, soil conductivity (μS/cm2), soil organic content (%), and the coefficient of variation for each of the aforementioned variables. The “Plant Community” matrix included abundances/frequencies for each of the 41 (species with n > 5) encountered species and the 25-point average of Shannon diversity index from each lake.
Statistical analysis
We used a partial CCA design (Borchard et al. Citation1992, Legendre and Legendre Citation1998) to estimate the amount of species-data variance that could be attributed to water and sediment characteristics, including lake/watershed size and light/bottom slope. To partition the variance explained by these 2 sets of variables, we conducted 3 separate analyses. First, both water and sediment/basin matrices were used individually as independent variables. The analyses were then repeated with the other set of variables as covariates (Borchard et al. Citation1992, Legendre and Legendre Citation1998). The proportion of variance explained by each analysis was calculated as the sum of all constrained eigenvalues from the significant canonical axes divided by the total inertia. Variance components were described as follows: total water chemistry variation including any sediment/basin component [W], total sediment/basin variation including any water component [S], total water chemistry variation excluding any sediment/basin component [W|S], and total sediment/basin variation excluding any water component [S|W]. We calculated the variance attributed to the correlation between water and sediment/basin components as [W] − [W|S] and the total proportion of variance explained as [W] + [S|W] (Borchard et al. Citation1992, Legendre and Legendre Citation1998). All analyses were conducted with a forward selection process (α = 0.05) using a Monte-Carlo permutation test to examine the influences of each variable under the reduced model. Biplots were constructed using Hill's scaling.
To describe the relationships among sediment/basin characteristics and the individual species in-lake distributions, multiple logistic regressions were used. The species dataset was converted to a species presence/absence matrix (n = 750) and overlaid with the sediment/basin dataset. Using that dataset, individual species multiple-logistic regressions were run with a forward selection process and 499 permutations. Thirty species were encountered 15 or more times and were analyzed as described earlier. The sediment/basin variables included in these analyses were sediment conductivity, bottom slope, sediment organic matter, sediment pH, and light reaching the bottom.
Finally, we used linear regression to examine the relationship between the individual point assessment of Shannon diversity index (n = 750) to light and depth to determine how point diversity relates to water clarity and the physical features of a lake. All analyses were conducted in R using the STATS and VEGAN packages.
Results
Environmental effects on aquatic plant community structure
Forty-one species were encountered during this initiative and were present at 5 or more points; 11 were not present at 15 or more points (). Our preliminary data assessment elucidated a significant correlation between light reaching the bottom and depth (ρ > 0.60); therefore, depth was removed from multivariate analyses. No other variables exhibited strong correlations ().
Water chemistry variables accounted for 18% of the total species-data variance when sediment/basin variables were not included as covariates [W]. The first 2 canonical axes were found to be significantly different when all 6 axes were compared (). Axes 1 and 2 accounted for 13 and 6% of the variance, respectively. Alkalinity and conductivity were found to have the greatest individual relationships with species data, but conductivity provided the best fit when all variables were evaluated together (axis 1, 2; inset correlations = 0.83, 0.23; conditional λ = 0.03; and ). The other water chemistry variables did not improve the fit of the model appreciably.
Table 3. Water chemistry CCA without covariates.
Table 4. Water chemistry CCA with sediment/basin covariates.
Sediment/basin variables accounted for 36% of the total species-data variance when water chemistry variables were not included as covariates [S]. The first 4 canonical axes were found to be significantly different and independent when all 10 axes were compared (). Axes 1, 2, 3, and 4 accounted for 15, 9, 7, and 5% of the variance, respectively. Sediment pH, organic matter, and conductivity exhibited the strongest individual relationships with those species data. When all environmental variables were considered together, those same variables provided the best fit, but the hierarchy changed to: (1) sediment pH, (2) sediment conductivity, and (3) sediment organic matter (). Sediment pH exhibited inset correlations of 0.79, 0.02, 0.14, and 0.07 with the first 4 canonical axes (conditional λ = 0.01; and ). Sediment conductivity had inset correlations with axes 1, 2, 3, and 4 of 0.70, 0.27, −0.38, and 0.09, respectively (conditional λ = 0.01). Sediment organic matter had inset correlations of 0.02, −0.68, −0.22, and −0.39 with the first 4 canonical axes (conditional λ = 0.01; and ). The remaining sediment/basin variables, including light, did not significantly improve the fit of the multivariate model. Finally, variation in sediment/basin characteristics did not appreciably affect the model.
Table 5. Sediment/basin CCA without covariates.
Table 6. Sediment/basin CCA with water chemistry covariates.
When sediment/basin variables were included in the evaluation of water chemistry influences on aquatic plant community structure [W|S], the total variance explained was reduced to 9% (). Furthermore, the amount of variance explained by sediment/basin variables [S|W] was reduced to 28% when water chemistry variables were entered as covariates (). Therefore, the total variance that can be attributed to the interrelationships among water and sediment/basin ([W] – [W|S]) variables was 9 percent (). The total variance explained by these 2 sets of variables ([W] + [S|W]) was 46% (). Variable influence hierarchies did not change for either analysis.
Table 7. Description of variance partitioning.
A CCA biplotconstructed with water chemistry variables shows a distinct separation of species along environmental gradients. The western side of the biplot contains species that exhibit relationships with softwater systems, and the eastern side contains species that show a preference for hardwater systems (). Notably, the 4 nonnative species encountered during this study show distinct differences in their relationships with water chemistry variables. Both the frequency and abundance of Myriophyllum spicatum and Potamogeton crispus exhibit strong positive relationships with lake water conductivity/alkalinity; conversely, Myriophyllum heterophyllum and Cabomba caroliniana show negative correlations with lake conductivity/alkalinity (), suggesting that M. spicatum and P. crispus are distributed in hardwater systems whereas M. heterophyllum and C. caroliniana are distributed in softwater systems. A few other examples of species positively associated with water conductivity and alkalinity are Ceratophyllum demersum, Potamogeton perfoliatus, and Potamogeton illinoensis. Finally, examples of species related to softwater systems include many of the Utricularia species, Eriocaulon aquaticum, and Gratiola aurea ().
Figure 1. Water chemistry CCA biplots. Both species variables were analyzed together but were separated for viewing simplicity. The figures with species abbreviations of “.ab” and “.fr” indicate that the plots represent species abundances and frequencies, respectively.
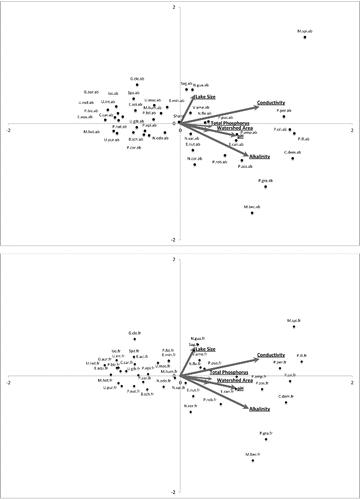
Plotting species data against sediment/basin variables again shows a distinct separation among species along environmental gradients. The eastern side of the biplot is associated with sediments of high conductivity and pH whereas the western side is characteristic of sediments low in pH and conductivity (). The northern sections of the biplot represent sediments with low organic content, and the southern portion is representative of sediments with high organic content. Species separate along these 3 gradients and, unexpectedly, light did not have a strong influence in the primary axes; it becomes important in axis 4, accounting for 5% of the variance (). Interestingly, the 4 nonnative species (i.e., M. spicatum, P. crispus, M. heterophyllum, and C. caroliniana) separate in the same way as described for water chemistry (). Myriophyllum spicatum and P. crispus show strong positive associations with sediment pH and conductivity whereas M. heterophyllum and C. caroliniana exhibit negative relationships with these variables. Additionally, Potamogeton perfoliatus, Potamogeton amplifolius, Megalodonta beckii, and Potamogeton illinoensis are a few examples of species positively related to sediment pH and conductivity. On the other side of the pH/conductivity spectrum are many of the Utricularia species, Eleocharis acicularis, Gratiola aurea, and Potamogeton bicupulatus. A few species show positive correlations with sediment organic content, including Potamogeton robbinsii, Ceratophyllum demersum, and Potamogeton gramineus. Finally, a few species that exhibit negative relationships with sediment organic content include Potamogeton pusillus, Elatine minima, and Isoetes spp. ().
Individual species relationships to environmental conditions
When each species was analyzed individually using the raw sediment/basin dataset (n = 750) and multiple logistic regression, 22 species were strongly related to sediment pH, 18 were significantly affected by light, 13 by sediment organic content, 11 by bottom slope, and 7 were significantly affected by sediment conductivity (). Furthermore, we found that the nonnative species had similar relationships compared to those obtained during the lake-wide sediment/basin plant assemblage structure analysis. Cabomba caroliniana exhibited a significant negative relationship with sediment pH (p < 0.001) and a previously undetected positive relationship with sediment organic matter (p = 0.02). Myriophyllum heterophyllum also showed a strong negative correlation with sediment pH (p < 0.001). Myriophyllum spicatum was strongly and positively associated with sediment pH (p < 0.001) and conductivity (p < 0.001), and positively and negatively related to light and bottom slope, respectively. Potamogeton crispus was not encountered the minimum number of 15 times and was not analyzed with this method.
Table 8. Individual species' logistic regression and specific variable influences.
When the Shannondiversity index (H’) was regressed against light and depth, both variables’ relationships were significantly different from random (p < 0.001 for both). Depth was a factor that explained a higher degree of data variance (r2 = 0.42; ) compared to light (r2 = 0.27, ). The relationships of light and depth with H’ were positive and negative, respectively.
Discussion
Our results confirm previous work that water and sediment/basin characteristics are sets of complimentary factors that structure aquatic plant assemblages (Alahuhta et al. Citation2013). However, our results indicate that local sediment characteristics correlate well with species composition and probably act as the principle factor determining the structure of lentic plant assemblages. We found that sediment/basin variables explained 28% of the total species-data variance, and that the variance was accounted for primarily by sediment pH, conductivity, and organic content. Water chemistry accounted for an additional 9% of the variance in species data, and aqueous conductivity and pH were responsible for the explanatory power in that CCA model. Overall, 46% of the species data variance was explained by these 2 sets of data (9% due to the correlation of the 2 environmental datasets), resulting in a powerful description of environmental influences on aquatic plant assemblage structure.
Our results also indicate that the majority of individual species in-lake distributions are affected by sediment pH/light availability and associated covariates. Although light was an important factor on an individual species distribution level, it was not highly significant in the whole community analysis. Instead, the structure of the assembly as a whole requires that light be present (i.e., important in the fourth canonical axis), but that assemblage structure is primarily determined by sediment/water pH and conductivities. Despite that finding, light was important to the individual in-lake distribution of many of the encountered species, as was expected. Growth forms of aquatic plant species vary widely, and light was principally important for species such as Brasenia schreberi, Elatine minima, Eleocharis acicularis, Eriocaulon aquaticum, Nuphar variegata, Nymphaea odorata, Potamogeton natans, and Sagittaria graminea, which are generally recognized as shallow-water species. Furthermore, sediment pH was found to be important for 22 aquatic macrophyte species, including the nonnative species encountered during this study. Although Potamogeton crispus was not encountered with a frequency allowing logistic analyses, C. caroliniana, M. heterophyllum, and M. spicatum exhibited significant relationships with sediment pH; Cabomba caroliniana and M. heterophyllum exhibited significant negative relationships with sediment pH; and M. spicatum was positively related to sediment pH. These results (including these species relationships with water chemistry) enhance/confirm previously reported findings for Connecticut's nonnative aquatic macrophyte/environmental relationships (June-Wells et al. Citation2013). Ultimately, these findings suggest that the overall assemblage structure is a result of lake-wide environmental properties whereas the in-lake distribution of individual species is directly related to small-scale variations in sediment features (i.e., pH) and light.
Lake-wide diversity (average point diversity) did not exhibit any strong relationships with the measured environmental variables; however, when diversity was analyzed on a point-by-point basis, significant relationships with light and depth were detected. Light and depth are highly correlated, but depth provided a greater degree of fit during model development. As expected, these results suggest that more species are present and evenly contributing to the assemblage in shallow areas of each lake (i.e., high-light areas). It is tempting to decidedly attribute this in-lake community feature to the abundance of light, but it may be a result of an unstable area present in all lakes. Whether this result is due to higher levels of disturbance from wave/ice action and/or from exposure during times of low precipitation is unclear because these measures were not included in these analyses; however, this finding does suggest that most of the plant species diversity is located in the top 1–2 m of water depth.
A theoretical overview
The process that assembles and organizes plant communities is still a matter of debate in the field of aquatic biology (Clements Citation1916, Gleason Citation1926, Weiher and Keddy Citation1995, Gotelli and McCabe Citation2002). However, we can estimate that at least 3 levels of organization would comprise a comprehensive hypothesis of aquatic plant community assembly. These levels can be interpreted as filters (Whitaker and Woodwell 1971) that constrain or promote the presence and abundances of aquatic macrophyte species in an assemblage. The first filter can be described as “transport of the local species pool,” a constraint/promoter defined by the physical movement of species throughout the region. Mechanisms of transport for aquatic plant species vary, but for a species to become a part of a plant community, it must first be present in the region and undergo transport through natural or anthropogenic processes (Gotelli and Simberloff Citation1987, Gibson et al. Citation1999).
Our analyses suggest that aquatic plant species are tightly coupled to environmental conditions, and these relationships characterize the second filter, the “abiotic filter,” which acts after transport of species to a waterbody. This study and other contemporary work in the field of aquatic plant community ecology suggest that species have distinct spatial responses that correlate with regional differences in water chemistry (Capers et al. Citation2009a, June-Wells et al. Citation2013). More precisely, carbon type/availability (Wetzel et al. Citation1984, 1985), conductivity (Kadono Citation1982a, Citation1982b, June-Wells et al. Citation2013), pH (Roberts et al. Citation1985, Jackson and Charles Citation1987, June-Wells et al. Citation2013), and nitrogen/phosphorus availability (Heegard et al. 2001, Gu and Hoyer 2005) are the important characteristics of the waterbody. These variables are distinctly related to the distribution of species and the overall assemblage composition where carbon type/availability, pH, and conductivity are thought to be most important. The first 2 filters lead to an incomplete understanding of aquatic plant assemblage structure because they are evaluated on a regional scale. Competition and niche theory are not landscape-scale phenomena; they occur at the smallest scales among individuals and populations in an assemblage where variation in the abiotic environment leads to the patchwork mosaics inherent to lentic plant assemblages. These local-scale effects may be the limiting factor in the effort to unite the multiscale initiatives of many researchers. The initiative in our study incorporates that variation and provides patterns relating to that scale.
The final filter affecting the composition and structure of aquatic plant assemblages is “competition in the local area” (Tilman Citation1981, Citation1987, Citation2004). Competition occurs among species populations at the smallest scales where expansion (i.e., species abundance) is dictated by a plant species ability to obtain resources, process carbon, and perform in a manner that excludes other species. Differences in species abilities to compete in the local area distinctly impact the structure of the local assemblage (Tilman Citation2004). Our results coincide with the idea that species have distinct environmental relationships resulting from enhanced competitive ability in the local abiotic environment; however, an in depth review of the contribution of competitiveness to the assemblage structure of the study lakes is beyond the scope of this initiative.
Conclusion
The results of this study suggest that assemblage structure and species distributions correlate most significantly (following transport) with water and sediment/basin characteristics. In addition, sediment/basin characteristics are probably more important because individual species exhibit strong relationships with specific sediment characteristics. Our results provide new data that will help unite the current plant assembly models describing regional species distributions and the local patchwork mosaic structure of aquatic plant assemblages. This study further suggests that, at least within the lentic environment, such models should use a deterministic approach based on strong abiotic filters.
A management perspective
Understanding the relationship among aquatic plant species and the abiotic environment allows researchers and lake managers to fully comprehend the functional properties of these assemblages and the larger community, including interactions among plants, fish, invertebrates, and amphibians. It also allows lake and resource management organizations to identify lakes at risk for nonnative species (i.e., water conductivity/sediment pH) and areas of species diversity within lakes. Our findings can be variously applied to private and conservation sectors by incorporating the principles of functional community assessments, including rigorous environmental valuation, allowing managers to monitor areas of lentic systems that favor colonization by nonnative species (i.e., sediment-data-driven plant community monitoring). Additionally, this initiative identifies the areas and conditions with the greatest assemblage diversity, which might call into the question the use of drawdown as a preferential plant management technique. More research is required to fully understand whether the areas of greatest diversity are functioning differently than areas dominated by a single species, but ecologists believe these areas contain a wider variety of interactions that constitute ecosystem function (Ogden et al. Citation2013, Valiente-Banuet and Verdú Citation2013). Therefore, these areas should probably be conserved when managing lentic systems because they may be areas where nutrients are cycled comprehensively, a more diverse set of insects reside, fish spawn or find refuge, amphibians feed and hide, and a more diverse range of interactions occur among microbes and other organisms. These assumptions exemplify the importance of functional assessments and targeted management because the need for responsible management approaches is crucial in this anthropogenic age.
Acknowledgments
We thank Jordan Gibbons for her assistance during this project, and a debt of gratitude is owed to Peter Morin for his consultation on statistical methodology.
Funding
This research was funded by the US Department of Agriculture under the Specific Cooperative Agreement 58-6629-2-205 and USDA Hatch CONH00768.
References
- Alahuhta J, Kanninen A, Hellsten S, Kari-Matti V, Kuoppala M, Hamalainen H. 2013. Environmental and spatial correlates of community composition, richness and status of boreal lake macrophytes. Ecol Indic. 32:172–181.
- Barko JW, Smart RM. 1986. Sediment-related mechanisms of growth limitation in submersed macrophytes. Ecology. 67:1328–1340.
- Borchard D, Legendre P, Drapeau P. 1992. Partialling out the spatial component of ecological variation. Ecology. 73:1045–1055.
- Canavan RW, Siver PA. 1995. Connecticut lakes: a study of the chemical and physical properties of fifty-six Connecticut lakes. New London (CT): Connecticut College Arboretum.
- Capers R, Selsky R, Bugbee GJ. 2009a. The relative importance of local conditions and regional processes in structuring aquatic plant communities. Freshwater Biol. 55:952–966.
- Capers RS, Selsky R, Bugbee GB, White JC. 2009b. Species richness of both native and invasive aquatic plants influenced by environmental conditions and human activity. Botany. 87:306–314.
- Clements FE. 1916. Plant succession: an analysis of development of vegetation. Washington (DC): Carnegie Institution of Washington.
- Deevey ES. 1940. Limnological studies in Connecticut: a contribution to regional limnology. Am J Sci. 238:717–741.
- Eaton AD, Clescenti LS, Greenberg AE. 1995. Standard methods for the examination of water and wastewater 19th ed.Washington (DC): American Public Health Association.
- Frink CR, Norvell WA. 1984. Chemical and physical properties of Connecticut lakes. New Haven (CT): Connecticut Agricultural Experiment Station.
- Gibson DJ, Ely JS, Collins SL. 1999. The core satellite hypothesis provides a theoretical basis for dominant, subordinate, and transient species. J Ecol. 87:1064–1067.
- Gleason HA. 1926. The individualistic concept of the plant association. Bull Torrey Bot Club. 53:7–26.
- Gotelli NJ, McCabe DJ. 2002. Species co-occurance: a meta-analysis of J.M. Diamond's assembly rules model. Ecology. 83:2091–2096.
- Gotelli NJ, Simberloff D. 1987. The distribution and abundance of tallgrass prairie plants: a test of the core-satellite hypothesis. Am Nat. 130:18–35.
- Gu B, Hoyer MV. 2005. Community structure and environmental conditions in Florida shallow lakes dominated by aquatic vegetation. Lake Reserv Manage. 21:403–410.
- Heegaard E, Birks HH, Gibson CE, Smith SJ, Wolfe-Murphy S. 2001. Species-environmental relationships of aquatic macrophytes in Northern Ireland. Aquat Bot. 70:175–223.
- Hellquist CB. 1980. Correlation of alkalinity and the distribution of Potamogeton in New England. Rhodora. 82:331–344.
- Jackson ST, Charles DF. 1987. Aquatic macrophytes in Adirondack (New York) Lakes: patterns of species composition in relation to environment. Can J Bot. 66:1449–1459.
- Jacobs MJ, MacIsaac HJ. 2009. Modelling spread of the invasive macrophyte Cabomba caroliniana. Freshwater Biol. 54:296–305.
- Johnstone M, Coffey BT, Howard-Williams C. 1985. The role of recreational boat traffic in interlake dispersal of macrophytes: a New Zealand case study. J Environ Manage. 20:263–279.
- June-Wells MR, Gallagher F, Gibbons J, Bugbee GJ. 2013. Water chemistry preferences of five non-native aquatic macrophyte species in Connecticut: a preliminary risk assessment tool. Lake Reserv Manage. 29:303–316.
- Kadono Y. 1982a. Distribution and habitat of Japanese Potamogeton. Tokyo Bot Mag. 95:63–76.
- Kadono Y. 1982b. Occurrence of aquatic macrophytes in relation to pH, alkalinity, calcium, chloride, and conductivity. Japn J Ecol. 32:39–44.
- Keddy PA. 1992. A Pragmatic approach to functional ecology. Funct Ecol. 6:621–626.
- Lacoul P, Freedman B. 2006. Relationships between aquatic plants and environmental factors along a steep Himalayan altitudinal gradient. Aquat Bot. 84:3–16.
- Legendre P, Legendre D. 1998. Numerical ecology, 2nd ed. Amsterdam (Netherlands): Elsevier.
- Magurran AE. 1988. Ecological diversity and its measurements. Princeton (NJ): Princeton University Press.
- Ogden L, Heynen N, Oslender U, West P, Kassam KA, Robbins P. 2013. Global assemblages, resilience, and earth stewardship in the anthropocene. Front Ecol Environ. 7:341–348.
- Roberts D, Singer R, Boyle CW. 1985. The submersed macrophyte communities of Adirondack lakes (NY, USA) of varying degrees of acidity. Aquat Bot. 21:21–235.
- Søndergaard M. 2007. Nutrient dynamics in lakes – with emphasis on phosphorus, sediment and lake restorations [dissertation]. [Aarhus (Denmark)]: University of Aarhus, National Environmental Research Institute. 276 p.
- Thum RA, Lennon JT. 2010. Comparative ecological niche models predict invasive spread of Variable Leaf Milfoil (Myriophyllum heterophyllum) and its potential imact on closely related native species. Biol Invasions. 12:133–143.
- Tilman D. 1981. Tests of resource competition theory using four species of Lake Michigan algae. Ecology. 62:802–815.
- Tilman D. 1987. On the meaning of competition and the mechanisms of competitive superiority. Funct Ecol. 1:304–315.
- Tilman D. 2004. Niche tradeoffs, neutrality, and community structure: a stochastic theory of resource competition, invasion, and community assembly. P Natl Acad Sci. 101:10854–10861.
- Valiente-Banuet A, Verdú M. 2013. Human impacts on multiple ecological networks act synergystically to drive ecosystem collapse. Front Ecol Environ. 8:408–413.
- Weiher E, Keddy P. 1995. The assembly of experimental wetland plant communities. Oikos. 73:323–335.
- Wetzel RG, Brammer ES, Forsberg C. 1984. Photosynthesis of submersed macrophytes in acidified lakes. I. Carbon fluxes and recycling of CO2 in Juncus bulbosus L. Aquat Bot. 19:329–342.
- Wetzel RG, Brammer ES, Lindstrom K, Forsberg C. 1985. Photosythesis of submersed macrophytes in acidified lakes. II. Carbon limitation and utilization of benthic CO2 sources. Aquat Bot. 22:107–220.
- Whittaker RJ, Woodwell GM. 1971. Evolution of natural communities. Ecosystem and structure. In: Weins JA, editor. Proceedings of the 21st Annual Biological Colloquium. Corvallis (OR): Oregon University Press. p. 137–159.
- Wilson J. 1999. Assembly rules in a plant community. In: Weiher E, Keddy P, editors. Ecological assembly rules: perspectives, advances, retreats. Cambridge (UK): Cambridge University Press.