Abstract
Cui Y, Zhu G, Li H, Luo L, Cheng X, Jin Y, and Trolle D. Modeling the response of phytoplankton to reduced external nutrient load in a subtropical Chinese reservoir using DYRESM-CAEDYM. Lake Reserv Manage. 32: 146--157.
The DYRESM-CAEDYM model was used to quantify the potential effects of reductions in external nutrient loads to the Shahe Reservoir in China, with an emphasis on effects on phytoplankton biomass. DYRESM-CAEDYM was calibrated using field data for a 2-year period and validated against a separate 1-year period. Simulated values for water temperature, dissolved oxygen, total nitrogen, total phosphorus, and chlorophyll a showed good agreement with field measurements. The model tended to underestimate peak values of inorganic nutrients such as ammonium, nitrate, and phosphate, with phosphate levels close to detection limits in both observed and simulated values. The model successfully captured seasonal phytoplankton succession, indicating spring peaks of diatom biomass and summer peaks of cyanophytes. Scenarios in which different reductions in external loading were applied suggest that simultaneous reductions in both external nitrogen and external phosphorus loading is more effective in controlling phytoplankton biomass than reducing input of either nutrient alone. Diatoms responded more strongly to load reductions in phosphorus input than nitrogen load, whereas cyanophytes showed the opposite pattern. A combined 25% reduction in the external loading of both nutrients could significantly decrease phytoplankton biomass in the reservoir below the 7 μg/L chlorophyll a required by drinking water standards, and the study indicated that DYRESM-CAEDYM can be a useful management tool for eutrophication control.
High nutrient loading to lakes generally leads to increased turbidity, extensive phytoplankton blooms, and loss of biodiversity. In past decades, major efforts have been made worldwide to improve the ecological status of lakes by reducing external nutrient load (Marsden Citation1989, Sas and Ahlgren Citation1989). In a contemporary study of multiple lakes, Jeppesen et al. (Citation2005b) demonstrated that reducing external total phosphorus (TP) load resulted in decreases in TP and chlorophyll a (Chl-a) concentrations and increases in Secchi depth (water transparency) in most cases. Model case studies from temperate lakes have indicated that the reduction in TP loading required for phytoplankton biomass to drop below acceptable levels proposed by the Water Framework Directive (WFD) requirements (summer average <6.5μg/L Chl-a) of the European Union are typically substantial (e.g., 40–50%; Søndergaard et al. Citation2005). Moreover, a reduction of 75% of total nitrogen (TN) input, could be necessary to achieve a worthwhile impact on phytoplankton biomass (e.g., Trolle et al. Citation2008a, Citation2008b). Therefore, although reducing external nutrient loads is a key component of mitigating eutrophication of lakes, quantifying the reductions required in individual lake systems is critical.
Water quality in the Shahe Reservoir in China's Jiangsu Province has been continuously threatened by fishery, tourism, and the development of organic agriculture since its completion in 1962. The main problem remains eutrophication and thus low water transparency, a decline in drinking water quality, and increased incidence of potentially toxic algal blooms (Zhang et al. Citation2005, Wu et al. Citation2006, Gao et al. Citation2009). Numerous measures have been taken to moderate the adverse effects of large-scale agricultural and tourism developments, such as creation of wetlands, rural domestic waste water treatment, sediment dredging, and manipulation of fish stocks (Li et al. Citation2013a, Zhu et al. Citation2013). These restoration measures were somewhat successful in some years, resulting in significant improvements in water quality and reduced incidence of algal blooms. However, a recent year by year increase in tea cultivation within the Shahe watershed, while bringing great economic benefit, has led to increased fertilizer use; the dose applied in tea gardens is approximately twice that used on rice paddies (Han et al. Citation2012). Furthermore, vegetation coverage in tea garden areas is generally low, decreasing to as little as 40% of surface area after harvest in April and May (Nie et al. Citation2013), leading to a high risk of erosion and transport of large quantities of nutrients to the Shahe Reservoir during rainfall events. Thus, there is a great risk that algal blooms will once again become a serious water pollution issue. Zhu et al. (Citation2013) recently noted that the top-down control of phytoplankton biomass in the Shahe Reservoir has already been exploited to its maximum potential by adjusting fish stock ratios, and that future improvements are likely to require bottom-up control of nutrient levels. Furthermore, recent increases in the frequency of extreme high temperature and precipitation events (Huang et al. Citation2011) have favoured algal bloom formation, and therefore further reductions in external nutrient load are required to counteract the deteriorating effects of climatic change.
Quantifying the external nutrient load reductions required for achieving desirable levels of, for example, maximum phytoplankton biomass is critical. Ecological models can be useful for predicting the effects of a reduced nutrient loading on lake water quality, and a large number and wide variety of models have been developed and applied to this purpose (Jørgensen Citation2010). The use of models in planning, designing, and testing management strategies has become commonplace since the 1980s.
The 1-dimensional (1D), vertically resolved, lake ecosystem model, the Dynamic Reservoir Simulation Model-Computational Aquatic Ecosystems Dynamic model (DYRESM-CAEDYM) developed by the University of Western Australia's Center for Water Research, has been applied successfully to several lake systems across the globe (Schladow and Hamilton Citation1997, Bruce et al. Citation2006, Burger et al. Citation2008, Trolle et al. Citation2011). The main objective of this study was to quantitatively assess the effects of decreased external nutrient loads on phytoplankton biomass in the Shahe Reservoir using DYRESM-CAEDYM.
Study site
The Shahe Reservoir is located in Liyang city in Jiangsu Province (), China, in the subtropical belt. The weather is influenced by a maritime monsoon climate and characterized by mild and humid conditions, with an average annual air temperature of 17.5 C and an average annual precipitation of 1149.7 mm, falling mainly from May to September. The reservoir has an area of 9 km2, a maximum water volume of 109 million m3, and average and maximum depths of 7 and 14 m, respectively. The Shahe Reservoir is one of the largest reservoirs in the Jiangsu hilly area and is important for both water supply and tourism. Three main rivers discharge into the reservoir, the Pingqiao, the Xiasong, and the Zhongtian. The lake catchment (146 km2) comprises forested hills (37.7%), cultivated land (23.7%), tea gardens (10.9%), and land put to other uses (Diao et al. Citation2013). External nutrient loads can be characterized as diffuse loading and point-source loading, with diffuse sources contributing a majority of the N and P loads from the catchment. Li et al. (Citation2013b) estimated that the TN loads from diffuse and point sources are 176.55 and 29.46 t/yr, respectively, and TP loads are 2.22 and 1.07t/yr, respectively. The phytoplankton community of the reservoir is dominated by cyanophytes and diatoms (Zhu et al. Citation2015).
Materials and methods
Model description
DYRESM is a 1D hydrodynamic model that resolves the vertical distribution of water temperature, salinity, and density in lakes and reservoirs (Gal et al. Citation2003, Yeates and Imberger Citation2004). DYRESM can be coupled to CAEDYM for investigations involving biological and chemical processes. CAEDYM is able to simulate carbon (C), N, P, Silica (Si), and dissolved oxygen (DO) cycles, including several size classes of inorganic suspended solids and potentially several phytoplankton groups specified by the user. DYRESM-CAEDYM has been widely used for long-term simulations of lake ecosystems (Bruce et al. Citation2006, Burger et al. Citation2008, Trolle et al. Citation2008a, Citation2008b, 2011, Gal et al. Citation2009).
The DYRESM-CAEDYM model was run at hourly time steps between January 2011 and December 2013 with daily averaged input data and daily output data (). Daily flow and meteorological data are required as input. Monitoring of inflow volumes and nutrient concentrations for this study was usually carried out monthly, so linear interpolations were performed to derive daily values. The model requires accurate data regarding water balance to be representative of the system being modeled, and these were initially derived from observed values for inflow and outflow, also taking into account potential evaporation. Any residual was treated as additional inflow volume. Six meteorological variables are required to calculate the vertical distribution of temperature, including cloud cover (fraction), shortwave radiation (W/m2), air temperature (C), vapor pressure (hPa), wind speed (m/s), and precipitation (m). Cloud cover and shortwave radiation data were acquired from a single meteorological station in Nanjing, located ∼120 km northwest of the Shahe Reservoir, and the other data were acquired from Liyang Station, near the Shahe Reservoir shoreline.
Table 1. DYRESM parameters file as used for the simulation period (2011–2013).
The CAEDYM input files contain biological and chemical model configuration and process rate parameters based on typical literature values (e.g., Schladow and Hamilton Citation1997, Westernhagen Citation2011). The complexity of the conceptual ecosystem model of CAEDYM is considerable and may include up to 7 different phytoplankton groups and 5 zooplankton groups, as well as fish and jellyfish. The conceptual biota model for this study was limited to include only the 2 dominant groups of phytoplankton in the Shahe Reservoir, cyanophytes and freshwater diatoms (Zhu et al. Citation2015). Phytoplankton growth in the model of the Shahe Reservoir is limited by light, temperature, P, N, and Si (for diatoms), and phytoplankton loss is attributed to respiration, mortality, and excretion. The light limitation function is described by an exponential response to incoming irradiance I, with Ik determining the slope of the light response curve (Webb et al. Citation1974):
(1)
For the diatom species, the Si limitation function is similar to that for N and P:
(2) where SiO2o is minimum concentration of Si at which growth ceases, and Ksia is the half-saturation constant for the effect of silica on the growth rate. The main output file is a NetCDF file, which may be visualized using the ARMSLite software package.
Model calibration and validation
In this study, the DYRESM-CAEDYM model was calibrated over a 2-year period (Jan 2011–Dec 2012) and validated for a separate 1-year period (Jan 2013–Dec 2013). There were originally 10 sampling sites distributed around the Shahe Reservoir (), but the water depth of sample site TM09 was often too shallow to sample. We collected surface water samples at the remaining 9 sites monthly from 2011 to 2013. Surface water samples were collected monthly to compare observations with the model predictions of temperature, DO, nutrients, chlorophyll a, and the 2 groups of phytoplankton. Additionally, in the deepest part of the study site, TM01, YSI probes were deployed at every 1 m depth to monitor the vertical distribution of water temperature and DO concentration. The concentration of PO4 was measured using a flow injection (Skalar SAN++, Delft, Netherlands) analyzer. Chlorophyll a concentration was measured by spectrophotometry at wavelengths of 665 and 750 nm, following extraction with hot 90% ethanol (Jespersen and Christoffersen Citation1987). Concentrations of nutrients were determined according to the textbook of Standard of Lake Eutrophication Survey of China (Jin and Tu Citation1990). Biomass (mg/L) of phytoplankton groups was estimated from empirical formulas after microscopy cell counts (Jin and Zhang Citation1995). Based on the proportion (%) of cyanophytes of the total phytoplankton biomass (derived from the cell counts), we estimated the cyanophyte concentration in Chl-a units (μg/L Chl-a) by multiplying the observed total Chl-a concentration by the biomass proportion of cyanophytes. An equivalent procedure was used to estimate diatom concentrations in Chl-a units.
Model parameters were adjusted manually according to a trial and error approach, with values constrained within ranges indicated by the literature (e.g., Schladow and Hamilton Citation1997, Özkundakci et al. Citation2011). Initial adjustments sought to maximize visual agreement between observed data and the model output, with further refinements achieved by optimizing model performance statistics (). Two commonly used statistical variables, represented by the coefficient of determination (R2) and the absolute relative error (RE), were selected to evaluate model performance. When evaluating the significance of correlations we used the Statistical Program for Social Sciences (SPSS) 19.0 software.
Table 2. Assigned values for the main parameters used in the calibration of DYRESM-CAEDYM for the Shahe Reservoir.
A series of nutrient load scenarios were set up to quantify the effect of external load reductions on Chl-a levels and on cyanophyte and diatom biomass. We designed 12 scenarios in which external loads of N, P, or both N and P (N+P) were reduced by 0, 25, 50, or 75% relative to each of the 3 baseline years from 2011 to 2013. To set up external load reduction scenarios, we reduced all different nutrient fractions (25%, 50%, and 75%) with the same proportion, including the daily concentration of nitrate (NO3), ammonium (NH4), labile particulate organic nitrogen (PONL), and labile dissolved organic nitrogen (DONL) in 3 inflows for N reduction. For P reduction, we reduced the daily concentration of phosphate (PO4), labile particulate organic phosphorus (POPL), and labile dissolved organic phosphorus (DOPL) in inflows. For N+P reduction, we reduced the daily concentration of NO3, NH4, PONL, DONL, PO4, POPL, and DOPL in 3 inflows. Tests for significant differences among different external load reduction scenarios were performed with a one-way ANOVA test in SPSS 19.0 software. Throughout the manuscript, we report nutrient concentrations in units of P and N, respectively, meaning, for example, that NO3 concentrations are equivalent to NO3-N.
Results
Calibration and validation
We implemented a water balance analysis from January 2011 to December 2013. After this calculation, the water level in the model remained almost constant over the calibration and validation period. Simulated water level clearly agreed well with the observed water level (), which provides confidence that the simulated water fluxes (comprised of inflows, outflows, and evaporation) were accurately balanced.
The surface water temperatures simulated by the model showed good agreement with observed data from Shahe Reservoir during both the calibration (2011–2012) and validation periods (2013; , ). DYRESM-CAEDYM reproduced water temperature accurately, with a high coefficient of determination and small relative error values during the calibration (R2 = 0.99, RE = 8.6%) and validation period (R2 = 0.96 RE = 14.7%). There was also good correspondence between bottom water temperatures output from the model and monitoring data in both the calibration (R2 = 0.83, RE = 19.8%) and the validation period (R2 = 0.94, RE = 18.2%), although some discrepancy existed between simulated and measured values for hypolimnion water temperature in 2012 ().
Table 3. Statistical variables for DYRESM-CAEDYM model performance for the Shahe Reservoir.
Figure 3. Comparison of model simulations (lines) against field measurements (circles) for temperature and dissolved oxygen in the surface (0 m) and bottom (11 m) waters of the Shahe Reservoir during the calibration period (solid lines, black circles) and validation period (dashed lines, hollow circles).
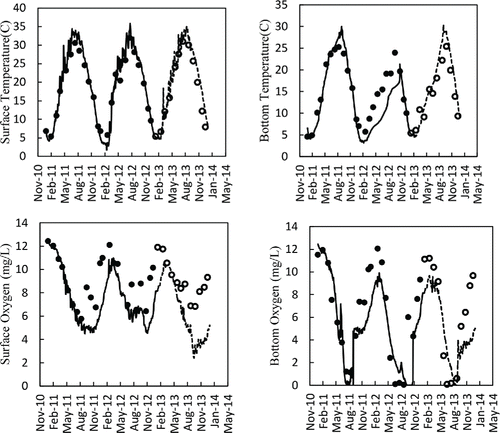
Comparison of simulated and observed data for surface DO yielded coefficient of determination values of 0.58 and 0.62 and RE values of 17.4 and 30.9%, respectively, indicating a good fit between model and field measurements during both the calibration and validation period. The simulations were able to predict the effects of stratification and sediment oxygen demand on bottom DO, which is gradually depleted until late summer when completely anoxic conditions are established (). Although the model also reproduced oxygen dynamics well, it tended to underestimate DO concentrations in the epilimnion when it peaked at the end of winter of 2013. There was generally good agreement between the hypolimnion DO values predicted by the model and those indicated in the monitoring data during the calibration period, yielding a high coefficient of determination value of 0.74 and small relative error value of 23.6%, respectively. However, the spring peak DO levels in the hypolimnion during the validation period were underestimated.
The surface TN concentrations yielded by the model simulation showed good agreement with measurements taken during both the calibration and validation period (), although peak values were somewhat underestimated during the validation period. The simulated values for NO3, however, were a relatively poor match for field data, reflecting the overall annual and seasonal variation seen during the calibration period but underestimating NO3 concentrations during the validation period (). The modeled values for NH4 generally showed annual and seasonal variations similar to those in observed data during both the calibration and the validation period, but again the model failed to simulate some of the peak values (). Simulation of TP during both the calibration and validation period was good, and the model successfully predicted seasonal and annual variations (). Both simulated and measured PO4 levels were low throughout both the calibration and validation period (), but the performance statistics were poor because the observed field concentrations were only marginally near detection limits (0.003 mg/L; Ma Citation2008) and thus yielded low R2 and large RE values.
Figure 4. Comparison of model simulations (lines) against field measurements (circles) for nutrients, and chlorophyll a in the surface (0 m) water of the Shahe Reservoir during the calibration period (solid lines, black circles) and validation period (dashed lines, hollow circles). The red line means the detection level of NO3 (0.01 mg/L) and PO4 (0.003 mg/L) concentration, respectively.
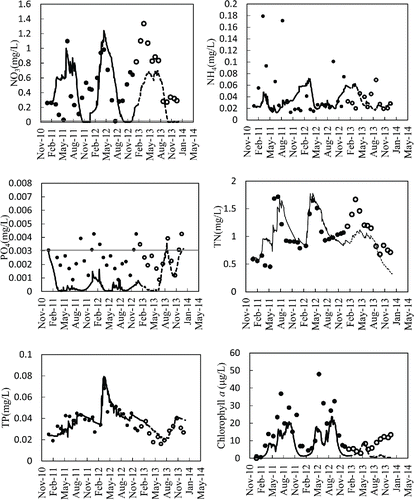
Model predictions for phytoplankton biomass included only cyanophytes and diatoms, which generally account for 70–80% of observed phytoplankton biomass in the Shahe Reservoir. Nevertheless, the model showed good agreement with field values of total Chl-a in terms of annual and seasonal variations during both the calibration and validation period (). The observed levels of Chl-a in 2013 were low, and the model successfully tracked this low trend through spring and summer of that year. In contrast, the model underestimated peak values of total Chl-a during the calibration period and winter values during the validation period. DYRESM-CAEDYM was generally able to capture the pattern of phytoplankton succession, including summer blooms of cyanophytes and interannual variability between 2011 and 2013 (). The model also successfully simulated the timing of diatom spring blooms and, in most cases, the annual and seasonal variation in their magnitude throughout the calibration and validation period (). However, the model tended to underestimate summer and autumn biomasses of diatoms during both the calibration and validation period.
Nutrient load reduction scenarios
As expected, the nutrient load scenarios all had an effect on predicted Chl-a concentrations in the Shahe Reservoir (). The model suggests that reducing external N loading will elicit an immediate response, which was more pronounced than that achieved by reducing only P load. A combined reduction of both external N and P loading reduction was shown to be most effective in reducing the Chl-a concentration, with average summer values declining from 10.22 to 6.40, 4.68, and 2.31 μg/L when simultaneously reducing both N and P inputs by 25, 50, and 75%, respectively. To meet the ecological quality recommendations of Zhang et al. (Citation2005), who suggested annual average Chl-a concentrations should be in the range of 4.1–10 μg/L or less, we set a water quality target of 7 μg/L for the Shahe Reservoir. The simulations indicated that such a target might be achieved with a combined reduction in both N and P load of 25%. The target might similarly be met by reducing N load alone by 50% or P load alone by 75%.
Figure 6. Simulated effects of reduced external nutrient loading (25%, 50% and 75%) on summer average surface total Chl-a, cyanophytes, and diatoms (based on data from 1 May to 30 September of each individual year between 2011 and 2013). Bars represent 95% confidence levels. Same letters denote there was no significant (P < 0.05) difference between them. Different letters denote there was a significant (P < 0.05) difference between them.
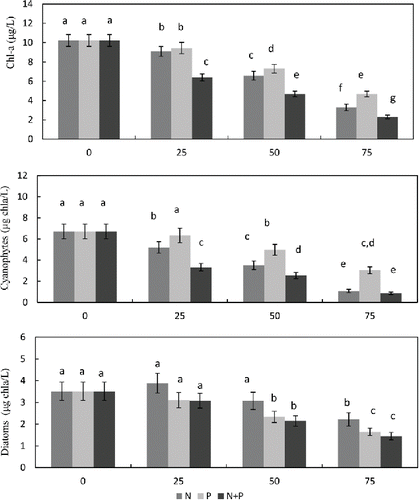
Simulated reductions in external N load had an immediate effect on cyanophyte biomass (). By contrast, reductions in P load produced no evident effect on cyanophytes biomass until reductions exceeded a threshold in the range of 50–75%. According to the model, average summer cyanophyte concentrations in the Shahe Reservoir might be decreased from 6.71 μg/L Chl-a to 5.20, 2.15, or 0.81 μg/L Chl-a by reducing external N load by 25, 50, or 75%, respectively. Moreover, at 75% external N reduction, cyanophyte levels declined to almost zero in the final year of the simulated period. By contrast, diatom biomass responded slowly when external N load was reduced, showing no reduction until loads declined by 50–75%. Diatom biomass even increased slightly when N loads were reduced by just 25%. Better results were achieved by reducing P loads (), but the most effective reductions in diatom biomass were achieved by reducing N+P loads. Average summer diatom concentrations in the Shahe Reservoir could be reduced from 3.51 μg/L Chl-a to 3.08, 2.14, and 1.45 μg/L Chl-a, as a result of reducing combined N+P loads by 25, 50, and 75%, respectively.
Discussion
Limitations of the model
R2 and RE showed good agreement between simulated values and field measurements for surface (R2 = 0.99, RE = 0.086) and bottom (R2 = 0.83, RE = 0.198) temperature, and the model was successful at reproducing the concentrations of DO and surface nutrients such as TN and TP during both the calibration and validation period. In particular, the model successfully captured the anoxic events in the hypolimnion during periods of stratification. Although the model was generally able to reproduce the timing and magnitude of simulated variables, there were some discrepancies between the model output and field measurements. Particularly, there was a poor fit between modeled and observed data for the inorganic nutrients PO4, NO3, and NH4. This finding maybe partially explained by the low concentrations of these compounds in the reservoir, which for PO4 remained only marginally around the nominal limits of detection (0.003 mg/L) by flow injection analysis for the majority of the study period. A low range of variation in observed data for inorganic nutrients leads naturally to a low covariance between observed and modeled data and low coefficients of determination (e.g., Trolle et al. Citation2011). The model generally underestimated PO4 concentrations, with observations generally higher during winter months, coinciding with periods of high runoff. In a similar modeling exercise for a temperate Danish lake, Trolle et al. (Citation2008b) adjusted sediment P release rates for each scenario to ensure they would equilibrate with the different external loads applied. Data to justify such an approach were not available in this study, but we were able to test the effect of reducing sediment P release to zero. This adjustment resulted in only minor changes to the PO4 and TP concentrations of the water column, suggesting that the significance of external loading exceeds that of internal loading by a considerable margin. The concentration of PO4 in the Shahe Reservoir is low, but organic forms of P can be mineralized to inorganic P to compensate for P deficiency of algae growth. Gao et al. (Citation2006) indicated that the P mineralization rate in Lake Taihu is fast, and the most rapid P turnover time is only a few minutes. Furthermore, local government adopted sediment dredging measures in 2007 to control water eutrophication, and internal loading is therefore low compared with the external loading. This prediction should be corroborated, however, by collecting bottom water samples for nutrient analysis in future research.
Another constraint of the model was underestimating the concentration of Chl-a. Biological variables are more difficult to simulate accurately than nonbiological variables due to the large number of known and unknown processes affecting variables such as biomass, timing of blooms, and the vertical distribution of each phytoplankton group. Although the model reproduced the magnitude and seasonal variation of phytoplankton biomasses during the simulation period, it could not capture the peak values occurring in summer and autumn during the calibration period. Furthermore, it also generally underestimated winter values of Chl-a. Note that the model only included cyanophytes and diatoms, which account for approximately 70–80% of the phytoplankton biomass in the reservoir; thus, Chl-a concentration, which is used as a proxy for total phytoplankton biomass, may be underestimated because some phytoplankton groups were simply not represented.
Although using a 1D model to simulate water quality in a reservoir has some limitations, the longitudinal gradients in Shahe Reservoir are generally minor. Nutrient concentrations could be somewhat higher in upstream than downstream. Based on our monitoring data, except for TM10 and TM8, the sample sites showed few differences. Nevertheless, the model should be considered as a management tool for decision making and technical support, but it remains a simplification of the real-world system.
Phytoplankton biomass responding to changes in nutrient loading
Chl-a and phytoplankton biomass in the Shahe Reservoir were used to evaluate the effects of external nutrient load reductions on the phytoplankton community. Some conceptual simplifications are unavoidable when systems are modeled in this way (Harris Citation1994), and the potential effects these simplifications may have on the outcome of various model scenarios must be considered (Özkundakci et al. Citation2011). No consideration was given to the potential effects of zooplankton, fish, or submerged macrophytes in the simulated ecosystem of our study, mainly because data for these elements were not available. Cascading effects from the top to the bottom of the food web may strongly influence water quality. For example, the proportion of piscivorous fish tends to increase with major reductions in average summer TP concentration, thus intensifying top-down control of benthi-planktivorous fish, reducing predation on zooplankton and thereby increasing grazing pressure on phytoplankton with a consequent decrease phytoplankton biomass (Jeppesen et al. Citation2005a, Citation2005b). Because higher trophic levels were absent from our simulations, modeled output describing the response of phytoplankton biomass to external load reductions may have been underestimated.
The model simulations indicate that water column Chl-a and cyanophyte concentrations may respond more rapidly to reductions in external N load than external P load. By contrast, diatom concentrations are more responsive to reduced external P than to N. One likely reason for these different response patterns is that N tends to be abundant during spring when diatoms grow but decreases rapidly during summer months when most of the cyanophyte growth takes place. Hence, the simulations suggest that primary production in the Shahe Reservoir is co-limited by both N and P. If focusing solely on annual or summer average Chl-a concentrations, reductions in N load may be an effective means of improving water quality; however, according to our simulations, the most effective control of phytoplankton biomass will be achieved through simultaneous reduction in both N and P inputs, providing useful information for setting management goals (e.g., Lewis and Wurtsbaugh Citation2008). A study by Özkundakci et al. (Citation2011) modeling the response of a highly eutrophic lake to reduced nutrients loading also found that reducing input of N alone was more effective than reducing only P load, but that simultaneous reduction in both N and P loads was most effective.
Implications for lake restoration and management
This study suggested that the control of external N loads may be a feasible means of restoring water quality. The most common form of N entering the reservoir is NO3 and dissolved organic N (Zhu et al. Citation2015). The major source of external N loading is agriculture, and common measures can be enacted including controls on fertilizer usage, the conversion of some areas to forms of agriculture that export fewer nutrients to waterways (e.g., forest or permanent grassland), the implementation of buffer strips alongside streams and lake shorelines, and the construction of wetlands to intercept and clean some of the lake inflows. In addition, overflow weirs are suggested to raise the water level and accelerate the denitrification process. External P loading is typically more strongly associated with point sources (Nielsen et al. Citation2013); however, particulate P is also an important form of P entering Shahe Reservoir. Therefore, sewage treatment and constructed wetlands approaches could be considered, although the efficiency of wetlands in removing P may be lower than for N (∼30% removal compared to 41%, respectively; Song et al. Citation2005). In addition, buffer strips are recommended to be constructed under the slope toe of tea gardens, which could effectively intercept P loss.
Although Chl-a and cyanophytes in the Shahe Reservoir tend to decrease more dramatically in response to reduced external N load than reduced P load, P should not be ignored in management interventions because N-fixing cyanobacteria may partly be able to compensate for reductions in external N load. This effect was not accounted for in our model simulations.
We consider a combined approach to simultaneously reduce both N and P loading to be the best choice for ensuring good ecological quality in the Shahe Reservoir. Even if such reductions are achieved, the ecosystem may still be resistant to change due to, for example, fish stock that diminishes the potential grazing effects of zooplankton on phytoplankton. In this case, further restoration measures such as sediment dredging, chemical treatment (for P flocculation), and fish removal may be necessary to drive the desired change from a turbid to a clear water regime. Nevertheless, reducing external nutrient loads will always be a prerequisite to such in-lake restoration measures. Özkundakci et al. (Citation2011) also stressed that the reduction of external nutrient loads must be prioritized in the long-term planning because a persistently abundant supply of nutrients will limit the longevity and efficacy of in-lake restoration methods targeting internal loading. Hence, reduced external loading is a principal requirement in lake restoration, at times supplemented with in-lake measures to accelerate the recovery process.
Conclusions
The 1D DYRESM-CAEDYM simulations carried out here demonstrate that the model is capable of reproducing the seasonally varying field observations of temperature, DO, nutrient levels, Chl-a concentration, and biomass values for 2 phytoplankton groups. Although the simulated results for NO3, NH4, and PO4 levels were not satisfactory, further efforts should aim to better account for lake sediment processes (and to collect bottom water samples for nutrient analysis to record any potential build-ups during stratification events) and possible top-down control effects on water quality and phytoplankton succession, both of which may increase the robustness of the model.
Diatoms responded more strongly to reductions in external P load than reduced N load, whereas cyanophytes showed the opposite pattern. For both types of phytoplankton, however, the most effective control was achieved by simultaneously reducing both N and P loads, suggesting that managers should adopt a combined approach. A 25% reduction of both N and P loads could decrease phytoplankton biomass below 7 μg/L in the Shahe Reservoir, thereby meeting targets for drinking water in China.
We found that a model such as DYRESM-CAEDYM can play an important role in planning strategy for reservoir management as well as aid in the scientific understanding of reservoir processes. We also found that further model improvements could increase the robustness of the model's predictive power.
Scenarios.xlsx
Download MS Excel (741.9 KB)Calibration_and_Validation.xlsx
Download MS Excel (772.5 KB)Acknowledgments
Special thanks to Visiting Prof. David P. Hamilton, University of Waikato, New Zealand, who helped the authors with calibrating the model. The authors would also like to thank Xiaoxia Han and Yujia Zhuang who supported the study by carrying out field and laboratory work at monthly intervals.
Funding
The study was supported by the Chinese National Science Foundation (51279194, 41301022), the International S&T Cooperation Program of China (ISTCP) project (2015DFG91980), the Taihu Pollution Control Office of Jiangsu Province (TH2014304) and the Shahe Administration Research Project.
References
- Bruce LC, Hamilton D, Imberger J, Gal G, Gophen M, Zohary T, Hambright KD. 2006. A numerical simulation of the role of zooplankton in C, N and P cycling in Lake Kinneret Israel. Ecol Model. 193:412–436.
- Burger DF, Hamilton DP, Pilditch CA. 2008. Modelling the relative importance of internal and external nutrient loads on water column nutrient concentrations and phytoplankton biomass in a shallow polymictic lake. Ecol Model. 211:411–423.
- Diao Y, Han Y, Li Z. 2013. Spatio-temporal change of tea plantation since 2000 and model-based prediction in the Tianmu Lake watershed. Lake Sci. 25:799–808.
- Gal G, Hipsey M, Parparov A, Wagner U, Makler V, Zohary T. 2009. Implementation of ecological modeling as an effective management and investigation tool: Lake Kinneret as a case study. Ecol Model. 220:1697–1718.
- Gal G, Imberger J, Zohary T, Antenucci J, Anis A, Rosenberg T. 2003. Simulating the thermal dynamics of Lake Kinneret. Ecol Model. 162:69–86.
- Gao G, Zhu G, Qin B, Chen J, Wang K. 2006. Alkaline phosphorus activity and the phosphorus mineralization rate of Lake Taihu. Sci China Ser D. 49:176–185.
- Gao Y, Zhu G, He R, Wang F. 2009. Variation of water quality and trophic state of Lake Tianmu, China. Environ Sci. 30:673–679.
- Han Y, Li H, Nie X, Xu X. 2012. Nitrogen and phosphorus budget of different land use types in hilly area of Lake Taihu Upper-River Basin. Lake Sci. 24:829–837.
- Harris GP. 1994. Pattern, process and prediction in aquatic ecology. A limnological view of some general ecological problems. Freshwater Biol. 32:143–160.
- Huang Y, Feng G, Dong W. 2011. Temporal changes in the patterns of extreme air temperature and precipitation in the various regions of China in recent 50 years. Acta Meteorol Sin. 69:125–136.
- Jeppesen E, Jensen JP, Søndergaard M, Lauridsen TL. 2005a. Response of fish and plankton to nutrient loading reduction in eight shallow Danish lakes with special emphasis on seasonal dynamics. Freshwater Biol. 50:1616–1627.
- Jeppesen E, Soendergaard M, Jensen JP, Havens KE, Anneville O, Carvalho L, Coveney MF, Deneke R, Dokulil MT, Foy B. 2005b. Lake responses to reduced nutrient loading–an analysis of contemporary long-term data from 35 case studies. Freshwater Biol. 50:1747–1771.
- Jespersen AM, Christoffersen K. 1987. Measurements of chlorophyll-a from phytoplankton using ethanol as extraction solvent. Arch Hydrobiol. 109:445–454.
- Jin X, Tu Q. 1990. Standard of lake eutrophication survey of China. Beijing (China): Environmental Science Publishing House. p. 69–75.
- Jin X, Zhang Z. 1995. Lakes of China. Bejing (China): Ocean Press.
- Jørgensen SE. 2010. A review of recent developments in lake modelling. Ecol Model. 221:689–692.
- Lewis WM, Wurtsbaugh WA. 2008. Control of lacustrine phytoplankton by nutrients: erosion of the phosphorus paradigm.Int Rev Hydrobiol. 93:446–465.
- Li H, Yang G, Nie X. 2013a. Reduction of nitrogen and phosphorus emission and zoning management targeting at water quality of lake or reservoir systems-practice in Lake Tianmu's water quality management. Lake Sci. 25:785–798.
- Li H, Zhu G, Chen W, Gao R, Nie X, Yu Z, Diao Y, Li X. 2013b. Current situation of good water quality reservoirs in hilly region of south-east China: protection practices of Tianmuhu Reservoir. Lake Sci. 25:775–784.
- Ma J. 2008. Development and application of the analytical technique and shipboard instrumentation for the determination of ultra-trace soluble reactive phosphorus in seawater[D]. Xiamen (China): Xiamen University.
- Marsden MW. 1989. Lake restoration by reducing external phosphorus loading: the influence of sediment phosphorus release. Freshwater Biol. 21:139–162.
- Nie X, Li H, Huang Q, Diao Y, Jiang J. 2013. Characteristics of nitrogen loss via runoff from typical land uses in hilly area of Tianmuhu watershed. Lake Sci. 25:827–835.
- Nielsen A, Trolle D, Me W, Luo L, Han B, Liu Z, Olesen JE, Jeppesen E. 2013. Assessing ways to combat eutrophication in a Chinese drinking water reservoir using SWAT. Mar Freshwater Res. 64:1–18.
- Özkundakci D, Hamilton DP, Trolle D. 2011. Modelling the response of a highly eutrophic lake to reductions in external and internal nutrient loading. New Zeal J Mar Fresh. 45:165–185.
- Sas H, Ahlgren I. 1989. Lake restoration by reduction of nutrient loading: expectations, experiences, extrapolations. Richarz, St. Augustin (Germany): Academia Verlag.
- Schladow SG, Hamilton DP. 1997. Prediction of water quality in lakes and reservoirs: Part II - Model calibration, sensitivity analysis and application. Ecol Model. 96:111–123.
- Søndergaard M, Jeppesen E, Jensen JP, Amsinck SL. 2005. Water Framework Directive: ecological classification of Danish lakes. J Appl Ecol. 42:616–629.
- Song Z, Wang R, Xi J, Han X, Yao C. 2005. Nitrogen and phosphrous removal efficiency and dynamic feature of constructed wetlands for sewage treatment. Chinese J Ecol. 24:648–651.
- Trolle D, Hamilton DP, Pilditch CA, DugganI C, Jeppesen E. 2011. Predicting the effects of climate change on trophic status of three morphologically varing lakes: implications for lake restoration and management. Environ Modell softw. 26:354–370.
- Trolle D, Jørgensen TB, Jeppesen E. 2008a. Predicting the effects of reduced external nitrogen loading on the nitrogen dynamics and ecological state of deep Lake Ravn, Denmark, using the Dyresm–Caedym Model. Limnologica. 38:220–232.
- Trolle D, Skovgaard H, Jeppesen E. 2008b. The Water Framework Directive: setting the phosphorus loading target for a deep lake in Denmark using the 1D lake ecosystem model Dyresm–Caedym. Ecol Model. 219:138–152.
- Webb WL, Newton M, Starr D. 1974. Carbon dioxide exchange of Alnus rubra. Oecologia. 17:281–291.
- Westernhagen VN. 2011. Measurements and modelling of eutrophication process in Lake Rotoiti, New Zealand.[D]. Waikato (New Zealand): University of Waikato.
- Wu J, Chen Z. 2006. Study on eutrophication in Shahe Reservoir. Pollut Control Technol. 19:23–25.
- Yeates PS, Imberger J. 2004. Pseudo two-dimensional simulations of internal and boundary fluxes in stratified lakes and reservoirs. Int J River Basin Res. 1:1–23.
- Zhang Y, Chen W, Yang D, Huang W, Jiang J. 2005. Main physical and chemical factors in Tianmuhu Lake, with evaluation of eutrophicaiton from 2001 to 2002. Res Environ Yangtze Basin. 14:99–103.
- Zhu G, Chen W, Li H, Ren L, Gu Z. 2013. Response of water quality to the catchment development and protection in Tianmuhu Reservoir, China. Lake Sci. 25:809–817.
- Zhu G, Cui Y, Han X, Li H, Zhu M, Deng J, Li H, Chen W. 2015. Response of phytoplankton to nutrient reduction in Shahe Reservoir, Taihu Catchment, China. J Freshwater Ecol. 30:41–58.