Abstract
The most recent update of the U.S. Environmental Protection Agency (EPA) health assessment document for asbestos (Citation, referred to as “the EPA 1986 update”) is now 20 years old. That document contains estimates of “potency factors” for asbestos in causing lung cancer (KL's) and mesothelioma (KM's) derived by fitting mathematical models to data from studies of occupational cohorts. The present paper provides a parallel analysis that incorporates data from studies published since the EPA 1986 update.
The EPA lung cancer model assumes that the relative risk varies linearly with cumulative exposure lagged 10 years. This implies that the relative risk remains constant after 10 years from last exposure. The EPA mesothelioma model predicts that the mortality rate from mesothelioma increases linearly with the intensity of exposure and, for a given intensity, increases indefinitely after exposure ceases, approximately as the square of time since first exposure lagged 10 years. These assumptions were evaluated using raw data from cohorts where exposures were principally to chrysotile (South Carolina textile workers, Citation; mesothelioma only data from Quebec miners and millers, Citation) and crocidolite (Wittenoom Gorge, Australia miners and millers, Citation) and using published data from a cohort exposed to amosite (Paterson, NJ, insulation manufacturers, Citation).
Although the linear EPA model generally provided a good description of exposure response for lung cancer, in some cases it did so only by estimating a large background risk relative to the comparison population. Some of these relative risks seem too large to be due to differences in smoking rates and are probably due at least in part to errors in exposure estimates. There was some equivocal evidence that the relative risk decreased with increasing time since last exposure in the Wittenoom cohort, but none either in the South Carolina cohort up to 50 years from last exposure or in the New Jersey cohort up to 35 years from last exposure.
The mesothelioma model provided good descriptions of the observed patterns of mortality after exposure ends, with no evidence that risk increases with long times since last exposure at rates that vary from that predicted by the model (i.e., with the square of time). In particular, the model adequately described the mortality rate in Quebec chrysotile miners and millers up through >50 years from last exposure. There was statistically significant evidence in both the Wittenoom and Quebec cohorts that the exposure intensity-response is supralinear1 rather than linear. The best-fitting models predicted that the mortality rate varies as [intensity]0.47 for Wittenoom and as [intensity]0.19 for Quebec and, in both cases, the exponent was significantly less than 1 (p< .0001).
Using the EPA models, KL's and KM's were estimated from the three sets of raw data and also from published data covering a broader range of environments than those originally addressed in the EPA 1986 update. Uncertainty in these estimates was quantified using “uncertainty bounds” that reflect both statistical and nonstatistical uncertainties. Lung cancer potency factors (KL's) were developed from 20 studies from 18 locations, compared to 13 locations covered in the EPA 1986 update. Mesothelioma potency factors (KM's) were developed for 12 locations compared to four locations in the EPA 1986 update. Although the 4 locations used to calculate KM in the EPA 1986 update include one location with exposures to amosite and three with exposures to mixed fiber types, the 14 KM's derived in the present analysis also include 6 locations in which exposures were predominantly to chrysotile and 1 where exposures were only to crocidolite.
The KM's showed evidence of a trend, with lowest KM's obtained from cohorts exposed predominantly to chrysotile and highest KM's from cohorts exposed only to amphibole asbestos, with KM's from cohorts exposed to mixed fiber types being intermediate between the KM's obtained from chrysotile and amphibole environments. Despite the considerable uncertainty in the KM estimates, the KM from the Quebec mines and mills was clearly smaller than those from several cohorts exposed to amphibole asbestos or a mixture of amphibole asbestos and chrysotile.
For lung cancer, although there is some evidence of larger KL's from amphibole asbestos exposure, there is a good deal of dispersion in the data, and one of the largest KL's is from the South Carolina textile mill where exposures were almost exclusively to chrysotile. This KL is clearly inconsistent with the KL obtained from the cohort of Quebec chrysotile miners and millers.
The KL's and KM's derived herein are defined in terms of concentrations of airborne fibers measured by phase-contrast microscopy (PCM), which only counts all structures longer than 5 μm, thicker than about 0.25 μ m, and with an aspect ratio ≥3:1. Moreover, PCM does not distinguish between asbestos and nonasbestos particles. One possible reason for the discrepancies between the KL's and KM's from different studies is that the category of structures included in PCM counts does not correspond closely to biological activity. In the accompanying article (Citation) the KL's and KM's and related uncertainty bounds obtained in this article are paired with fiber size distributions from the literature obtained using transmission electron microscopy (TEM). The resulting database is used to define KL's and KM's that depend on both the size (e.g., length and width) and mineralogical type (e.g., chrysotile or crocidolite) of an asbestos structure. An analysis is conducted to determine how well different KL and KM definitions are able to reconcile the discrepancies observed herein among values obtained from different environments.
INTRODUCTION
The health hazards associated with asbestos remain a major concern: (1) Substantial quantities of commercial asbestos products remain in commerce and in public buildings (HEI-AR, Citation1991); (2) due to the long latency between exposure and disease, new cases of asbestos-related diseases are likely to continue to occur; and (3) rocks that contain naturally occurring asbestos are found in some areas of the United States where population growth is large (see, for example, NYAS, Citation1991).
Given the above factors, a critical need exists for reliable risk management policies and practices that can effectively mitigate asbestos-related health threats. However, such policies and practices need to be based on sound science that adequately distinguishes hazardous situations from those that are not. Otherwise, the policies and practices that are intended to limit asbestos disease have the potential to cause unwarranted economic dislocation.
Reliable methods for assessing asbestos risks in different environments are needed to underpin sound policies and practices for mitigating such risks. Since the 1980s the U.S. Environmental Protection Agency (EPA) has employed an approach for assessing asbestos-related cancer risk based primarily on the findings of their latest Asbestos Health Effects Assessment Update, which was published in 1986 (Nicholson, Citation1986, henceforth called the “EPA 1986 update”).
Much relevant research has been published since the EPA 1986 update was completed, including several epidemiological studies of asbestos in heretofore unstudied environments, as well as additional years of follow-up for many of the epidemiological studies evaluated in the EPA 1986 update. In this article and a companion article, important aspects of the approach for assessing asbestos-related cancer risks described in the EPA 1986 update are reexamined by taking advantage of the broader literature that is currently available. These articles build upon analyses described in Berman and Crump (Citation2003), which was supported by the EPA as part of a larger effort to revise their 1986 update.
In the present article, certain features of the mathematical models used in the EPA 1986 update for predicting the asbestos-induced mortality from lung cancer and mesothelioma are examined. The lung cancer model predicts that mortality rate varies linearly with cumulative exposure and the mesothelioma model predicts that mortality rate varies linearly with exposure intensity. Both models predict that asbestos-induced risk remains indefinitely after exposure ceases. The relative risk of lung cancer is predicted to remain constant after 10 years from the end of exposure. The absolute risk of mesothelioma is predicted to increase indefinitely after a given increment of exposure approximately as the square of time since that exposure lagged 10 years. The present article examines these assumptions using raw data from studies of workers at chrysotile mines in Quebec (Liddell et al., Citation1997), a textile factory in South Carolina that handled primarily chrysotile from Quebec (Hein et al., Citation2007), a crocidolite mine in Wittenoom Gorge, Australia (Berry et al., Citation2004), and published summary data on workers at an insulation products factory in New Jersey that used amosite (Seidman et al., Citation1986).
Following the evaluation of the lung cancer and mesothelioma models, these models are applied to data from published studies representing a wide range of exposure settings to obtain potency estimates for asbestos in causing lung cancer and mesothelioma. Since an ultimate goal of this work is to understand the reason for the wide differences in potency estimates obtained from different occupational environments and to reconcile these differences, we attempted to include as many different environments in this analysis as reasonably possible. Consequently, a fairly low standard was set for inclusion of a study and the relative strengths of the individual studies were explicitly considered in the analysis.
The EPA models assume that there is no difference in the potencies of different types2 of asbestos (chrysotile or different varieties of amphibole asbestos) in causing lung cancer or mesothelioma and that risk can be predicted from exposures quantified using phase-contrast microscopy (PCM), which provides counts only of fibers3 longer than 5 μm and thicker than approximately 0.25 μm (NIOSH, Citation1994a, Citation1994b). However, there is increasing evidence that neither of these assumptions is valid, which could account for the disparate estimates of potency obtained in different environments (Berman and Crump, Citation2003). In a follow-up paper (Berman and Crump, Citation2008) the EPA models are modified by allowing different types of asbestos to have different potencies and risk is predicted from exposures quantified using size fractions of asbestos different from the one determined by PCM. The ability of these different assumptions to reconcile potency estimates derived from different environments is evaluated. That work builds on the potency estimates obtained from different environments that are reported in the present article.
BACKGROUND
The Nature of Asbestos
Rather than representing a single chemical species, the term “asbestos” refers to a particular fibrous form (asbestiform crystalline habit) of a set of minerals from the serpentine and amphibole mineral groups (IARC, Citation1977; Veblen and Wylie, Citation1993). About 95% of the asbestos that has been mined commercially is chrysotile, which is a member of the serpentine mineral group (Schreier, Citation1989). Five amphibole asbestos types make up the remaining set of minerals traditionally recognized as asbestos, including asbestiform reibeckite (crocidolite), asbestiform grunerite (amosite), anthophyllite asbestos, tremolite asbestos, and actinolite asbestos (IARC, Citation1977; Klein, Citation1993). Although other amphibole varieties were not included in the traditional definition of asbestos, the asbestiform habits of some of these amphiboles (such as winchite–richterite asbestos) occur as contaminants in deposits of a variety of other mined minerals, such as vermiculite from Libby, MT, and have raised health concerns (Meeker et al., Citation2003).
While unique names have been assigned to the asbestiform habit of serpentine (chrysotile) and two commercially mined amphibole minerals (crocidolite and amosite) to distinguish them from their more common, massive forms, such nomenclature has not been developed for other mineral types of asbestos. Hence the term “asbestos” is added to these mineral names to denote the asbestiform habit.
Measures of Exposure
Due to a complex history, a range of analytical methods have been employed to measure airborne asbestos concentrations in the various studies conducted over time (see, for example, Walton, Citation1982). Exposure estimates in the epidemiological studies considered here were derived from a variety of methods and were converted to approximate what would be obtained using PCM. Traditionally, PCM counts fibers longer than 5 μm with an aspect (length to width) ratio ⩾3:1 and largely parallel sides, and which are visible when viewed by PCM at a magnification of 400× (see, for example, NIOSH, Citation1994a). Due to the limited resolving power of a phase-contrast microscope, PCM counts only fibers thicker than approximately 0.25 μm (NIOSH, Citation1994b).
Health Effects Linked to Asbestos Exposure
Exposure to asbestos has been linked to several adverse health effects, including primarily lung cancer, mesothelioma, and asbestosis—a chronic degenerative lung disease (see the EPA 1986 update). The present analysis focuses on lung cancer and mesothelioma.
Lung cancer is one of the more common forms of cancer and it occurs much more frequently in smokers than nonsmokers. A synergistic relationship has been found between smoking and asbestos in causing lung cancer that originally was reported to be multiplicative (Hammond et al., Citation1979). However, more recent investigations have found the relationship to be intermediate between multiplicative and additive (Liddell, Citation2001; Liddell and Armstrong, Citation2002; Berry and Liddell, Citation2004).
Mesothelioma is a rare cancer of the connective tissue that lines the pleural cavity (containing the heart and lungs) and the peritoneal cavity (i.e., the gut). Although there is some evidence of a low background incidence of spontaneous mesotheliomas, this cancer has been primarily associated with exposure to asbestos and certain other fibrous substances (HEI, Citation1991). There is no evidence that smoking has an effect upon mesothelioma.
Exposure-Response Models
The risks of lung cancer and mesothelioma from exposure to asbestos are quantified in the EPA 1986 update using mathematical models for each of these diseases that relate exposure to risk. These models are calibrated by fitting them to data from epidemiological studies of occupational populations exposed to asbestos.
Lung Cancer Model
The lung cancer model (Selikoff et al., 1968) described in the EPA 1986 update was adopted based on the reportedly broad acceptance of a relative risk model to describe the time and age dependence of lung cancer and to fits of this model to available data sets. The model assumes that the relative risk, RR, of mortality from lung cancer at any given age is a linear function of cumulative asbestos exposure as measured by PCM (f-yr/ml), not including any exposure in the most recent 10 years prior to the given age. This exposure variable is denoted by CE10. The 10-year lag embodies the assumption that exposures during the most recent 10 years do not affect current lung cancer mortality risk. The mathematical expression for this model iswhere the linear slope, KL, is the “lung cancer potency factor.” To make allowance for the possibility that the background lung cancer risk in the exposed population differs from that of the comparison population, the model is expanded to the form
With this form of the model the relative risk at zero exposure is α rather than 1. Both KL and α are estimated by fitting the model to data.
This model predicts that the relative risk varies linearly with cumulative exposure lagged 10 years. A corollary to this prediction is the prediction that the relative risk remains constant past 10 years from the end of exposure (since cumulative exposure remains constant after that time). These predictions are evaluated herein by fitting the model to data from three cohorts.
Mesothelioma Model
The absolute risk model adopted for mesothelioma in the EPA 1986 update is a particular adaptation of the multistage model for carcinogenesis, which predicts that incidence is independent of age at first exposure and increases as a power of time since first exposure (Armitage and Doll, 1961; Pike, 1966; Cook et al., 1969; Peto et al., 1982). The model adopted in the EPA update was based on evidence of the time course of the mortality rate in several cohorts. This model can be derived by assuming that the mortality rate at time t after the beginning of exposure is the sum of the contributions from exposure at each increment of time, du, in the past. The contribution to the mortality rate at time t from exposure to E(u) fibers (f)/ml (as measured by PCM) at time u is assumed to be proportional to the product of the exposure rate, E(u), and (t – u –10)2, the square of the elapsed time minus a lag of 10 years. Thus, as with the lung cancer model [Eq. (2)], the mesothelioma model assumes a 10-year lag before exposure has any effect upon risk. With the additional assumption that the background rate of mesothelioma is zero, the mesothelioma mortality rate at time t after the beginning of exposure is given bywhere t and u are in years, and IM(t) is the mortality rate per year at year tafter the beginning of exposure. (The factor of “3” is needed to retain the same meaning of the mesothelioma potency factor, KM, as defined in the EPA 1986 update.)
If exposure is at a constant intensity, E, for a fixed duration, D, beginning at t= 0, this model can be written in the form presented in the EPA 1986 update:This model predicts that the mesothelioma mortality rate varies linearly with exposure intensity E (for fixed duration, D, and time since first exposure, t). Upon rewriting the model in the equivalent form,
for t⩾D+ 10, it is clear that the model also predicts that mesothelioma mortality rates increase indefinitely, even after exposure ends, and approximately as the square of time since exposure began lagged 10 years for times that are large in comparison to the duration of exposure. Both of these predictions are examined herein by fitting the model to data from three cohorts.
In the application of this model in the EPA 1986 update, no distinction was made between pleural and peritoneal mesothelioma. That practice is followed here as well. Since some studies do not distinguish between the two types, requiring such a distinction would leave fewer studies for analysis. This approach does not imply that risk from the two types of mesothelioma are equal or even that their individual mortality rates have the same general mathematical form; other studies have suggested that they do not (for example, Burdorf et al., 2007). Rather, our approach suggests only that the combined rate can be approximated by Eq. (3).
DATA USED TO EVALUATE RISK MODELS
For this study, raw data with follow-up through 2001 for the cohort of textile workers at the Charleston, SC, plant (Hein et al., Citation2007) were provided by the National Institute for Occupational Health and Safety (NIOSH) through the efforts of Everett (Chip) Lehman and others. Nicholas de Klerk graciously provided raw data for the cohort of crocidolite miners in Wittenoom, Australia (Armstrong et al., Citation1988; de Klerk et al., Citation1994; Berry et al., Citation2004). Raw data from the cohort of chrysotile miners and millers in Quebec, Canada, were graciously provided by Douglass Liddell (deceased) and Corbett McDonald (Liddell et al., Citation1997). However, the only specific information on cause of death provided for this cohort was whether a subject died of mesothelioma.
The South Carolina Cohort
There have been a number of investigations of a cohort of workers exposed primarily to chrysotile at a textile plant in South Carolina (Dement et al., Citation1982, Citation1983a, Citation1983b, Citation1994, Citation2007; Stayner et al., Citation1997, Citation2007; Dement and Brown, Citation1998, Hein et al., Citation2007). The present analysis is based upon follow-up though 2001 (Hein et al., Citation2007) of 3072 workers (1256 white men, 1.5% lost to follow-up; 1265 mostly white females, 17% lost to follow-up; and 551 nonwhite males, 7% lost to follow-up) who worked for at least one month between 1940 and 1965. In total, 1961 deaths were identified and cause of death was determined for all but 120 of these. There were in total 198 deaths from lung cancer, with 101.7 expected based on U.S. mortality rates. Only three deaths from mesothelioma were identified.
Based on data from nearly 6000 air samples taken at the plant between 1930 and 1975 and analyzed using PCM, linear statistical models were used to reconstruct exposure levels (Dement et al., Citation1983a), while taking into account textile processes, dust control methods, and job assignments. A department-, operation-, and calendar year-specific job/exposure matrix (JEM) was used to link air measurements with the detailed work histories to calculate individual worker exposures. Duration of employment was short, with a median of only about 1 year. Median cumulative exposure was about 4 f-yr/ml for white males and females and 14 f-yr/ml for black males.
Because a large percentage of females and nonwhite males were lost to follow-up, our investigation of the adequacy of the lung cancer and mesothelioma models [Eqs. (2) and (3)] is confined to the data for white males. In this group, there were 116 deaths from lung cancer for an overall SMR of 2.34 based on U.S. referent rates (Hein et al., Citation2007). Although all three deaths from mesothelioma occurred among white males, this small number severely limits our ability to investigate the adequacy of the mesothelioma model.
The Wittenoom, Australia, Cohort
Several studies have been conducted of a cohort employed at a crocidolite mine and mill in Wittenoom, Australia (Armstrong et al., Citation1988; de Klerk et al., Citation1989, Citation1994; Musk et al., Citation2002). The data provided for the current analysis includes information on 6910 men and women with follow-up through December 31, 2000 (Berry et al., Citation2004). The data consist of a record number, sex, date of birth, date of beginning employment, total days of employment, average exposure level (f/cc), date of last contact, ICD code for cause of death, indicator variables for lung cancer and mesothelioma deaths, and date of death, if applicable.
The cohort includes only 415 women (6%) and consequently this analysis is limited to men. In total, 135 men were removed because their records were missing some critical piece of information, along with 2 subjects of unknown sex. The remaining cohort consists of 6,358 men who were employed at Wittenoom Gorge at some time between 1943 and 1966. Of these, 2,421 were known to be deceased by the last date of follow-up (December 31, 2007), 222 from pleural or peritoneal mesothelioma and 302 from lung cancer. However, 27% were lost to view prior to the end of follow-up. In the analyses presented herein, follow-up of a subject stops when he is lost to view. (This approach was preferred by Armstrong et al. [Citation1988].) For consistency, this approach is also applied to the data for Quebec and South Carolina.
The concentrations of fibers greater than 5 μm in length, as determined by PCM, were measured at various work sites in a survey conducted in 1966. Job category data were obtained from employment records and supplemented by records from the Perth Chest Clinic and the Western Australian Mineworkers Relief Fund. The concentration measurements and job category information were used to estimate the exposure level for each subject in the cohort (de Klerk et al., Citation1989). The exposure levels were high, with a median of 17.8 fibers/ml. The durations of employment were low, with a median of 128 days.
The Quebec Cohort
Liddell et al. (Citation1997) reported on a cohort of about 11,000 workers at chrysotile asbestos mines and related mills in each of two areas of Quebec (Asbestos and Thetford Mines) that had been studied earlier by McDonald et al. (Citation1980b) (follow-up through 1975) and McDonald et al. (Citation1993) (follow-up through 1988). The cohort also includes workers at a small asbestos products manufacturing factory associated with the mine/mill in Asbestos, Quebec. Production at the mines began before 1900. The cohort consists of workers who worked at least 1 month and who were born between the years of 1891 and 1920. Liddell et al. (Citation1997) traced 9780 men through June 1, 1992, of which 1138 (10%) were lost to view, most of whom worked prior to 1935 and for only a few months. Of those traced, 8009 (82%) were deceased as of 1992. By that time, 38 deaths from mesothelioma had been identified in this cohort (Liddell et al., Citation1997).
Estimates of dust levels in specific jobs were made from some 4,000 midget impinger measurements collected systematically starting in 1948 and periodically in the factory beginning in 1944. Estimates for the period prior to 1949 utilized interviews with long-term employees and comparison with more recent conditions. These dust-level estimates were matched to individual job histories to produce estimates of average exposure (in millions of particles per cubic foot, or mppcf, as measured by midget impinger) for each worker by year, beginning with the first year of employment. On the basis of over 600 side-by-side midget impinger and PCM measurements, it was estimated that 1 mppcf was, on the average, equivalent to 3.14 PCM fibers/ml (f/ml) (McDonald et al., Citation1980b). This factor was used in the present analysis to convert the exposures in mppcf to f/ml.
The data provided for this cohort contain the following information on each worker: date of birth, last date of follow-up, whether follow-up ended as a result of death from mesothelioma, location of first employment, whether a worker had been employed at more than one location, and estimates by year of asbestos exposure in mppcf.
In the present analysis, based on a recommendation by Douglass Liddell, 1417 subjects whose exposures were uncertain (412 who worked at one of five small mines and 1005 who worked at more than one location) were omitted. This left 9244 subjects for the analysis, 6703 (73%) of whom were known to be deceased by the end of follow-up, including 35 with mesothelioma listed as the cause of death. In total, 1090 (12%) were lost to view prior to the end of follow-up. In addition to analyses involving the entire group of 9244 subjects, separate analyses were also conducted for the 4195 workers in the mines and mills at Asbestos (8 mesotheliomas), the 4291 workers in the mines and mills around Thetford Mines (22 mesotheliomas), and 758 workers in the small asbestos products factory at Asbestos (five mesotheliomas). These separate analyses were recommended by Professor Liddell because crocidolite was used at the Asbestos factory and the degree of tremolite asbestos contamination is thought to be higher in the ore at Thetford Mines than at Asbestos. Evidence for a higher tremolite content of ore at Thetford Mines comes from a combination of air measurements collected in the vicinity of mines in each area (Sebastien et al., 1986) and several studies of the fiber content of lung tissue obtained from deceased cohort members (Case et al., 1997; McDonald et al., 1997; Sebastien et al., 1989).
The Cohort of New Jersey Insulation Manufacturers
Seidman et al. (Citation1986) studied a cohort of 820 men (mostly white) who worked at an insulation manufacturing factory that operated in Paterson, NJ, from 1941 through 1954. The men began work between 1941 and 1945 and follow-up was through 1982. The follow-up of a worker began 5 years following the beginning of employment. Workers who had prior asbestos exposure were not included in the cohort and follow-up was stopped when a worker was known to have begun asbestos work elsewhere (six men). Exposures were generally brief, as 76% were exposed for ⩽2 years, although a few were exposed for as long as 10 years.
No asbestos exposure measurements were available for this plant. Estimates of exposures in particular jobs were based on air measurements made between 1967 and 1970 at plants in Tyler, TX, and Port Allegheny, PA, that were operated by the same company and made the same products using some of the same machinery as the Patterson facility. The estimated median exposure level was 50 f/ml. Amosite was the only type of asbestos used at the plant.
Although only the summary data in Seidman et al. (Citation1986) are available for this analysis, these data are well suited for evaluating the time course of disease following short exposures because the majority of cohort members in this study experienced very brief exposures (average of 1.5 years) relative to decades of follow-up, and observed and expected lung cancer and mesothelioma deaths are reported as a function of time since first exposure. Thus, the interval between last exposure and disease can be reasonably estimated. By restricting the analysis only to those exposed for less than 2 years and to times since first exposure longer than 10 years, results from such an analysis closely parallel those using time since last exposure for the Wittenoom and South Carolina cohorts. Moreover, although Seidman et al. did not include a lag in their exposure estimates, using only data for times since first exposure >10 years also implies that lagged and unlagged exposures are similar.
METHODS FOR EVALUATING MODELS
Fitting Models to Published Data
The published data most appropriate for fitting the lung cancer model [Eq. (2)] generally consist of observed and expected numbers of lung cancer deaths (based on an external control group, such as U.S. males) categorized by cumulative exposure. A likelihood is calculated from such data, assuming that the numbers of lung cancer deaths in different cumulative exposure categories are independent and Poisson distributed, with the expected numbers given by the expected numbers from the external control group times the relative risks calculated from Eq. (2). Estimates of KL are restricted to be nonnegative, so the possibility that exposure to asbestos is associated with decreased lung cancer risk is not allowed.
The published data most appropriate for fitting the mesothelioma model [Eq. (4)] generally consist of the number of mesothelioma deaths and person-years of observation categorized by time since first exposure. A likelihood is calculated by assuming that the numbers of mesothelioma deaths in different categories are independent and Poisson distributed, with the expected numbers given by the numbers of person-years of observation in a category times the per-year mortality rates predicted by Eq. (4), assuming average values for E, D, and t in Eq. (4) as appropriate for each category.
The published report on the New Jersey cohort (Seidman et al., Citation1986) contains data on mesothelioma deaths categorized by time since first exposure. Estimates of exposure intensity are made for each category of time since first exposure and the average exposure duration of 1.5 years is assumed for each cell. The mesothelioma model [Eq. (4)] is fitted to these data, assuming that the numbers of mesothelioma deaths in different cells are independent and Poisson distributed.
Fitting Models to Raw Data
To fit the lung cancer model [Eq. (2)] to the raw data from the South Carolina and Wittenoom cohorts, person-years of follow-up are cross-categorized by cumulative exposure lagged 10 years and time since last exposure. Observed and expected numbers of lung cancer deaths are computed for each cell formed by the categorization. Expected numbers are based on sex-race-age- and calendar-year-specific background rates (U.S. rates for South Carolina, and Australian rates for Wittenoom). Person-year-weighted averages are used to represent cumulative exposure lagged 10 years and time since last exposure for a cell. A likelihood is determined by assuming that the observed number in each cell is Poisson distributed with the expected number given by the expected number from the background rates times the relative risk predicted by the lung cancer model [Eq. (2)]. After fitting the lung cancer model to the bivariate data, the data are collapsed by combining over time since last exposure or cumulative exposure lagged 10 years and the resulting collapsed tables are used to construct graphs showing how observed relative risks and relative risks predicted by the lung cancer model vary with cumulative exposure lagged 10 years or time since last exposure.
The mesothelioma model [Eq. (3)] is fit to the raw data from Wittenoom and Quebec using a likelihood function in which each member of the cohort makes an independent contribution. The contribution to the likelihood of a person whose follow-up terminated at time t is exp[−H(t)], if follow-up did not terminate in death from mesothelioma, and IM(t)×exp[−H(t)], if the person died of mesothelioma, whereis the cumulative mortality hazard and IM(u) is the instantaneous rate [Eq. (3)]. The complete likelihood is the product of the contributions from individual subjects. To evaluate the assumption that the mortality rate is proportional to exposure intensity, the intensity, E, in Eq. (3) is replaced by EK,
and the nonlinearity parameter K is estimated and tested for departures from K= 1. Similarly, to evaluate the power of the time dependence, the exponent, 2, in Eq. (3) is replaced by z,
and the value for the exponent z is tested for significant variation from z= 2.
Statistical Methods
Model parameters are estimated by maximizing the log-likelihoods (Cox and Hinkley, Citation1974). Confidence bounds for model parameters (all are 95% one-sided bounds in order to be comparable to the traditional 5% error rate for one-sided hypothesis tests) are computed by the profile likelihood method and hypothesis tests are conducted by the likelihood ratio method (Venzon and Moolgavkar, Citation1988; Crump, Citation2002). Confidence intervals for observed counts and rates in graphs are exact 95% intervals assuming that the underlying counts are Poisson distributed.
RESULTS OF MODEL EVALUATION
Evaluation of Lung Cancer Model
Results obtained by fitting the lung cancer model [Eq. (2)] to data categorized by cumulative exposure (after collapsing the bivariate tables over time since last exposure) are shown in (Wittenoom), (South Carolina), and (Paterson, NJ). These graphs all show a similar pattern. In each case the fit with α= 1 is poor and the hypothesis that α= 1 is rejected. The fits with α= 1 (dotted lines in –) underpredict the observed relative risk (gray circles) in the lower cumulative exposure categories and overpredict in the highest exposure category. However, with α estimated (Wittenoom: α= 2.81; South Carolina: α= 1.65; New Jersey: α= 3.32) the fit of the lung cancer model is adequate in each case. The relative risks predicted with α estimated (solid lines in –3) all fall within the 95% confidence intervals for the observed relative risks. However, the values of α seem too large to be due to differences in background rates of lung cancer between the cohort and the reference population. This problem is addressed in more detail later. The estimated potencies with α estimated (Wittenoom: KL= 0.0042 (f-yr/ml)−1; South Carolina: KL=0.018 (f-yr/ml)−1; New Jersey: KL=0.011 (f-yr/ml)−1) are 3 to 10 times smaller than the corresponding estimates with α= 1 (Wittenoom: KL= 0.043 (f-yr/ml)−1; South Carolina: KL= 0.030 (f-yr/ml)−1; New Jersey: KL= 0.062 (f-yr/ml)−1)\.
FIG. 2 Observed and predicted lung cancer relative risk vs. cumulative exposure (South Carolina Cohort).
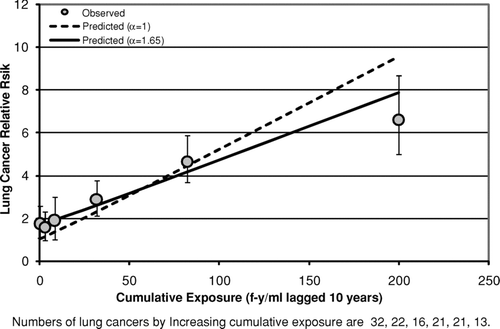
FIG. 3 Observed and predicted lung cancer relative risk vs. cumulative exposure (Patterson, New Jersey Cohort).
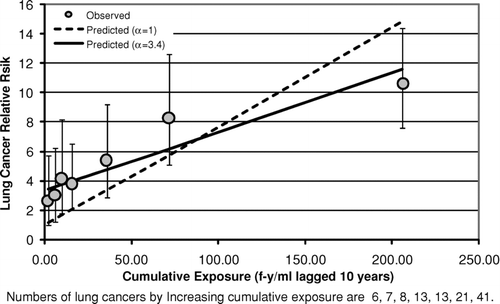
– show the fits of the lung cancer model [Eq. (2]) to data categorized by time since last exposure (time since first exposure in ) after collapsing the bivariate tables over cumulative exposure. In all three cases the predicted relative risks decrease with time due simply to the fact that cumulative exposures are lower at longer times since last exposure, even though the model predicts that, for fixed exposure, the relative risk will remain constant following 10 years past the end of exposure. For South Carolina () and New Jersey (), there is no evidence that the observed values tend to fall progressively below the predictions of the lung cancer model (which would occur if the relative risk decreased with time since last exposure) and the fits of both the models with α= 1 and α estimated are reasonable (p⩾ .1 in each case).
FIG. 4 Observed and predicted lung cancer relative risk vs. years after exposure ends (Wittenoom Cohort).
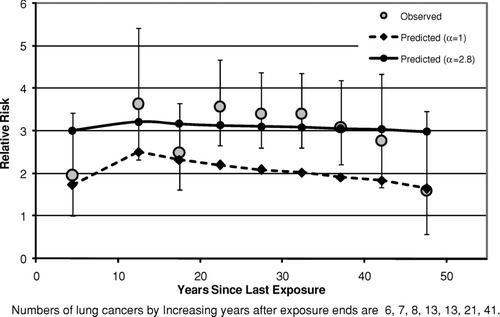
FIG. 5 Observed and predicted lung cancer relative risk vs. years after exposure ends (South Carolina Cohort).
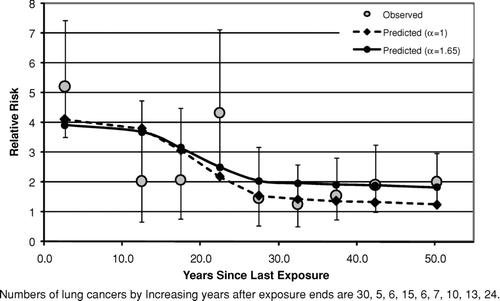
FIG. 6 Observed and predicted lung cancer relative risk vs. years after beginning of exposure (New Jersey Cohort).
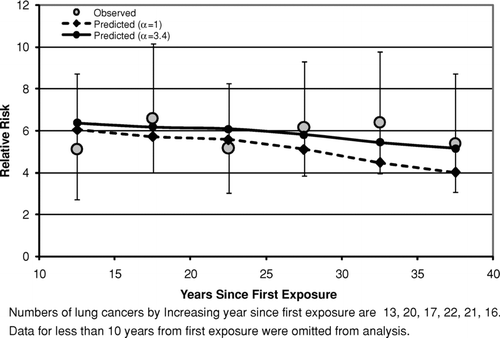
The situation is somewhat different with Wittenoom (), as these data show some evidence of a downward trend in relative risk with increasing time since last exposure, which cannot be explained by a decreasing cumulative exposure with increasing time. In this case, the fit with α= 1 is poor (p< .00001), although the fit with α estimated is adequate (p= .25). The downward trend with increasing time since last exposure is even stronger when cohort members lost to view are assumed to be alive at least until age 85 or the end of follow-up (results not shown), as suggested by Armstrong et al. (Citation1988) to account for the large percentage (27%) of the cohort that was lost to view before the end of follow-up. In this latter case, both of the fits with α= 1 and α estimated provide poor fits to these data.
Evaluation of Mesothelioma Model
contains results for Wittenoom, South Carolina, and three locations in Quebec of tests of the hypothesis that the mesothelioma incidence rate is proportional to the intensity of exposure [as predicted by the mesothelioma model, Eq. (4)] versus the alternative that the rate is a supralinear function of exposure intensity [as predicted by Eq. (7) with K< 1]. In each of the five sets of data, K was estimated to be <1, and significantly so for Wittenoom, and for the mines and mills of both Thetford Mines and Asbestos, Quebec. shows graphically the nonlinearity in the mesothelioma incidence rate for Wittenoom. The linear mesothelioma model [Eq. (3)] significantly underpredicts the observed mesothelioma incidence at the lowest exposure and significantly overpredicts at the highest exposure. By comparison, the nonlinear model [Eq. (7) with K= 0.47] describes the data adequately and the improvement in fit is highly significant (p< .0001).
TABLE 1 Results of tests of hypotheses that mesothelioma mortality varies linearly with exposure intensity (test of K = 1 in Eq. 7), or with the square of time since exposure lagged 10 Years (test of z = 2 in Eq. 8)
FIG. 7 Observed and predicted mesothelioma deaths per 100,000 person-years vs. exposure intensity (Wittenoom Cohort).
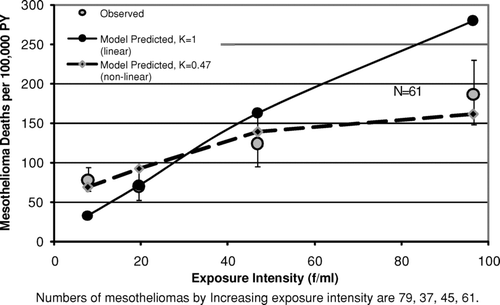
– show the mesothelioma mortality rates per 100,000 person-years categorized by time since last exposure (time since first exposure in ). For the Wittenoom cohort () the observed mesothelioma rate per 100,000 person-years is very close to the predicted rate at all times since last exposure. For the Quebec cohort (), although the number of mesotheliomas (35) is much smaller than in Wittenoom (222), the mesothelioma rates predicted by the mesothelioma model [Eq. (3)] are in good agreement with the observed rates for all categories of time since last exposure. In particular, there is no evidence that the model overpredicts the rate at long times after last exposure. In the longest time since last exposure category (>50 years) 4 mesotheliomas were observed with 2.9 predicted. For the New Jersey cohort (), the predicted mesothelioma rates are well within the 95% confidence intervals for the observed rates for every category of time since first exposure although there is some scatter at the two longest time intervals. However, the confidence intervals are wide, as only 17 mesotheliomas were observed in this study.
FIG. 8 Observed and predicted mesothelioma deaths per 100,000 person-years vs. years since last exposure (Wittenoom Cohort).
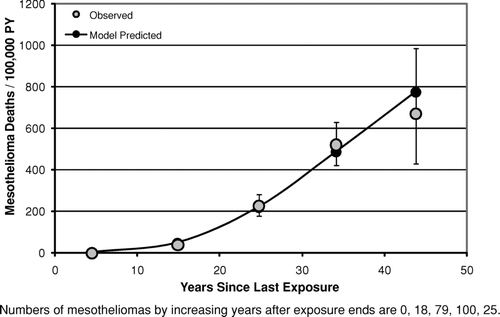
FIG. 9 Observed and predicted mesothelioma deaths per 100,000 person-years vs. years since last exposure (Quebec Cohort).
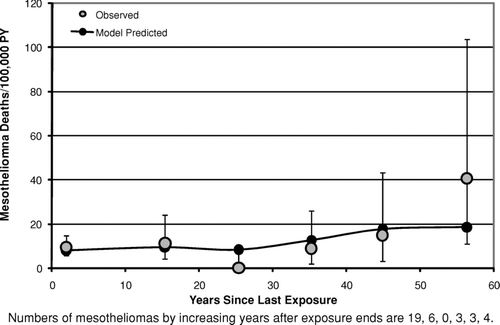
FIG. 10 Observed and predicted mesothelioma deaths per 100,000 person-years vs. years since first exposure (New Jersey Cohort).
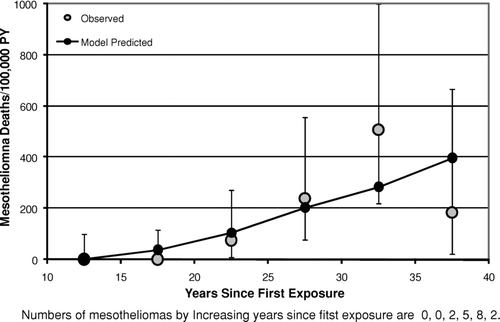
Impressions from – that the time dependence for the mesothelioma rate is adequately described by the mesothelioma model [Eq. (3)] are reinforced by the findings presented in indicating that maximum likelihood estimates for the time exponent [z in Eq. (8)] are not significantly different from 2 when fit to the data from Wittenoom, Quebec, or South Carolina.
Conclusions Concerning the Adequacy of the EPA Models
With respect to the predictions of the models for time after exposure ends, this analysis produced no convincing evidence against the assumption of the lung cancer model [Eq. (2)] that the relative risk remains constant after 10 years from last exposure. Although the Wittenoom data (crocidolite exposure) show some evidence of a decrease in relative risk following time since last exposure (), even after accounting for a small decrease in cumulative exposure with increasing time, there is no evidence of this either in the South Carolina data (, primarily chrysotile exposure) or in the New Jersey data (, amosite exposure). Likewise, the assumption of the mesothelioma model that the mesothelioma risk continues to rise as the square of time since first exposure [Eq. (5)] at long times after exposure ends appears to hold at least up to 50 years after exposure ends ( for Wittenoom and 9 for Quebec). The observed decrease seen in the New Jersey cohort () between 35 and 40 years since first exposure is statistically compatible with the model prediction.
With regard to the assumption in the lung cancer model that the relative risk varies linearly with cumulative exposure lagged 10 years, although the lung cancer model fits the exposure-response data for Wittenoom, South Carolina, and New Jersey adequately (–), it does so only by estimating large values for α (2.81, 1.65, and 3.32, respectively). These large values of α could theoretically reflect a higher background risk of lung cancer in a cohort than in the comparison population, due, for example, to higher rates of smoking in the cohort. However, it is questionable whether differences in smoking habits alone could account for values of α as large as these. Bang and Kim (2001) reported that smoking prevalence in different industry groups ranged up to 42% (95% CI: 32–52%) compared to a rate of 32% (95% CI: 30–34%) among men in the general U.S. population. Thus it seems that smoking in a particular cohort would be unlikely to be more than double the rate of the general population. This suggests that, even assuming a full multiplicative interaction, smoking would be unlikely to account for an α as large as 2. Moreover, as previously discussed, recent evidence indicates that the interaction between smoking and asbestos exposure is less than multiplicative (Liddell, Citation2001; Liddell and Armstrong, Citation2002).
If the true exposure response for lung cancer is supralinear, that could also result in α> 1 when fitting a linear model to responses to widely dispersed exposures bounded away from zero dose. However, this explanation doesn't seem likely here, given that the responses in – appear linear throughout a range of cumulative exposures down to relatively low values of cumulative exposure. A more likely cause of the elevated values of α is errors in exposure estimation. Exposure estimates are quite uncertain in all of these cohorts and this uncertainty is not addressed in the analysis. For example, suppose the most highly exposed members of a cohort employed respiratory protection more frequently than those less exposed, but respirator use was not accounted for in assessing personal exposures. This would cause exposures among the most highly exposed to be overestimated and would tend to result in an elevated estimate of α and a concomitant attenuation of KL. In addition, random errors in the explanatory variable (here represented by cumulative exposure), even if unbiased, can attenuate the regression slope, KL, while inflating α (see, e.g., Carroll et al., Citation2006).
Turning now to the assumption in the mesothelioma model that risk varies linearly with exposure intensity, in the analyses of both the Wittenoom and Quebec data, the linear model was strongly rejected in favor of a supralinear model ( and ). As with lung cancer, errors in exposure could cause a true linear response to appear nonlinear. If, for example, true exposure intensity at the highest intensity graphed in (∼95 f/ml) were in reality only half as large (due, say, to respirator use that was unaccounted for in the exposure estimates), then the exposure response would be approximately linear. It is also possible for errors in exposure, even if unbiased, to cause a linear exposure-response to appear nonlinear (Carroll et al., Citation2006; Crump, Citation2005). However, if the true exposure response for mesothelioma is supralinear, this would have important implications for efforts to estimate risk from low exposures.
Even if mesothelioma risk is a supralinear function of exposure intensity, that would not invalidate the use of the linear model in the present study, the goal of which is to reconcile potencies obtained from data at high doses in different environments. Furthermore, that few published studies report worker-specific exposure response data for mesothelioma precludes the application of a nonlinear model to these studies. Consequently, we have provisionally used the linear EPA model for mesothelioma [Eq. (4)] in our efforts to reconcile the data from different studies, while recognizing that this is an area where further research is needed.
APPLICATION OF MODELS TO DATA FROM PUBLISHED STUDIES
Methods for Selecting Studies and Quantifying Uncertainty
We applied the EPA lung cancer and mesothelioma models to as many studies as feasible using data available from the published literature. A fairly low standard was set for a study to be included in this work, although the relative strengths of the individual studies are accounted for in the analysis. Essentially, the minimum requirements for inclusion were that a study have both an adequate measure of response (for lung cancer: an observed and expected number of cancers; and for mesothelioma: an observed number of mesotheliomas and an associated number of person-years) and an adequate characterization of exposure. Since the present work utilizes somewhat different methods from the EPA 1986 update, for consistency, all KL's and KM's were recalculated, even those from studies for which no new data are available. In cases in which a cohort has been reported on multiple times using different follow-up dates, only the study with the most recent follow-up is used in this analysis.
Any study with suitable health and exposure data was eligible for the analysis, regardless of the number of lung cancers or mesotheliomas reported. The statistical uncertainty attributable to small numbers was accounted for through the use of statistical confidence bounds. Likewise, a statistically significant increase in lung cancers was not required to calculate a KL, but only that the data needed for the calculation be available.
For each study from which a KL or KM is estimated, a “best estimate” is obtained. For mesothelioma, the best estimate of KM is generally the maximum likelihood estimate. Statistical uncertainty in a KM estimate is quantified using 95% upper and lower statistical confidence bounds. For lung cancer, the best estimate of KL is generally the maximum likelihood estimate with α estimated. However, as noted earlier, very large estimates of α sometimes result, which are unlikely to be due solely to differences in background lung cancer rates between the studied cohort and the comparison population (e.g., differences in smoking habits), but are probably due at least in part, and perhaps mainly, to errors in exposure estimates.
Although differences in background risk would not tend to bias a KL estimate, exposure errors would tend to bias an estimate toward the null. Two steps are taken in this work to address this problem. First, extremely large values of α, which are highly unlikely to represent differences in background rates of lung cancer, are avoided by restricting α to be no larger than 2. Second, in quantifying the statistical uncertainty in KL the larger of two 95% statistical upper bounds are used for the upper bound—one resulting from the analysis with α= 1 and the other from the analysis with α estimated (or set at 2 when the estimated α is larger than 2)—and the smaller of these two statistical bounds is used for the lower bound. As indicated in the descriptions of individual studies, in a few cases, these general rules had to be adapted to fit the particular form of the data available from a specific study.
To illustrate the effect of the special provisions for applying the lung cancer model, for the case of the New Jersey cohort (Seidman et al., Citation1986) with α estimated (= 3.32), the KL estimate is 1.1 (f−y/ml)−1, 90% CI: (0.58, 1.9); with α= 2, the KL estimate is 2.4 (f−y/ml)−1; 90% CI: (1.8, 3.1); and with α= 1, the KL estimate is 6.2 (f−y/ml)−1, 90% CI: (5.0, 7.6). Consequently, rather than using the KL estimate and statistical confidence bounds associated with α= 3.32, the best estimate of KL was taken as 2.4 (f−y/ml)−1, with lower and upper statistical bounds of 1.8 (f−y/ml)−1 and 7.6 (f−y/ml)−1, respectively. As this illustrates, this approach results in an increase in both the KL estimate and in the statistical bounds (particularly the upper bound) over the values obtained with α unrestricted. Thus this approach accounts in some respects not only for statistical uncertainty, but also for uncertainty associated with the potential effect of exposure error.
Nonstatistical sources of uncertainty are at least as important as statistical uncertainty. Although these uncertainties are difficult to quantify, presentation of statistical uncertainty alone may provide a misleading picture of the reliability of the estimates. Consequently, an approach for addressing nonstatistical uncertainty was also adopted in this analysis. In this approach three factors were specified for each study to address three specific sources of uncertainty in exposure estimates: F1, uncertainty in air concentrations to which workers were exposed; F2, uncertainty resulting from the need to convert exposure measurements made using midget impinger and other previously used sampling methods to values equivalent to those generated when sampling on membrane filters and analyzing by PCM; and F3, uncertainty in workers' job histories. A fourth factor (F4L for lung cancer and F4M for mesothelioma) addressed special sources of uncertainty in the health response. These four factors were combined into a single factor, F (see Appendix A, Eq. A2), and “uncertainty ranges” for KL and KM were calculated by multiplying the statistical upper bound for a KL or KM by F and dividing the statistical lower bound by F. Guidelines used for specifying the individual uncertainty factors are described in Appendix A. The application of these guidelines to individual studies is described in Appendix B. The individual factors so obtained are summarized in .
TABLE 2 Uncertainty Factors Used to Develop Uncertainty Intervals for Exposure-Response Coefficients (KL's and KM's)
Results of Applying Models
Descriptions of the epidemiological studies and the calculation of KL's and KM's from these studies are presented in Appendix B. and summarize the study-specific potency factors (KL's and KM's) and related uncertainty intervals obtained in this work. The values are organized by fiber type, type of operation within fiber type, and specific location within type of operation. For comparison, the best estimate of KL or KM from the U.S. EPA (1986) update for the same location is also provided.
TABLE 3 Lung cancer dose-response coefficients (KL) derived from various epidemiological studies.
TABLE 4 Mesothelioma dose-response coefficients (KM) derived from various epidemiological studies.
Lung Cancer Potency Factors
The KL's and the associated uncertainty intervals shown in are plotted in , grouped by primary fiber type (chrysotile, mixed, crocidolite, amosite, or winchite–richterite) and industry (mining, friction products, asbestos-cement pipe, textiles, insulation manufacturing, or insulation application). For studies conducted at the same facility (generally among highly overlapping cohorts), a single study was selected for presentation in . Thus, for South Carolina, the Hein et al. (Citation2007) study was selected because this study includes the most recent follow-up and because of access to the raw data for this study. Similarly, the Sullivan (Citation2007) study was selected to represent the Libby vermiculite site over the other studies at this facility (McDonald et al., Citation1986; Amandus and Wheeler, Citation1987).
FIG. 11 Plot of estimated KL values and associated uncertainty intervals by study environment (from ).
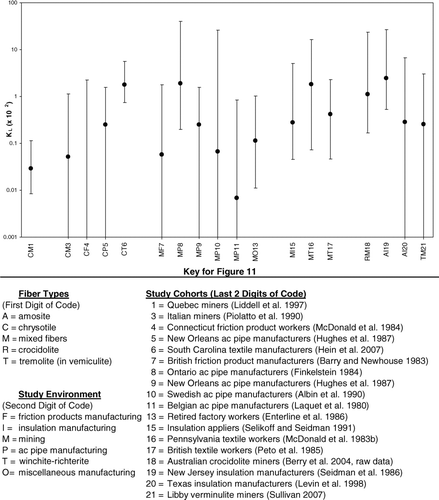
Within primarily chrysotile studies (and excluding the negative friction products study), lowest and highest KL's vary by approximately a factor of 70. Moreover, the uncertainty intervals for the lowest nonzero value (Quebec miners) and the highest value (South Carolina textile workers) have no overlap (). Both of these studies were deemed to be of high quality, which is reflected in the relatively narrow uncertainty intervals for the KL's from these two studies. With the exception of the South Carolina textile worker study, the uncertainty intervals of the remaining chrysotile studies all overlap, although, except for the study of Quebec miners, these uncertainty intervals are quite wide.
This difference in lung cancer potency observed among Quebec miners versus that among South Carolina textile workers has been the subject of much discussion and evaluation. The inability to reconcile this difference appears to be among the biggest obstacles to reconciling potency factors for lung cancer. The leading hypothesis for this difference is a difference in the relative distribution of fiber sizes in these industries (see, for example, Berman and Crump, Citation2003, Appendix D). Evidence from several sources suggests that textile workers were exposed to dusts containing substantially greater fractions of longer fibers than dusts to which miners were exposed.
There is much better agreement among the KL's obtained for amphibole asbestos studies than for chrysotile. Among “pure” amphibole asbestos studies, the lowest and highest of the KL's vary by a factor of approximately 9 and the 2 extremes both derive from within the same industry (amosite insulation). The two studies in question are of amosite insulation manufacturing plants (one in Paterson, NJ, and one in Tyler, TX) that utilized the same equipment (literally) and apparently the same source of asbestos (South Africa). Despite the ninefold difference in KL's, the uncertainty intervals for these two estimates have substantial overlap. If the arithmetic mean of the values for the two amosite insulation studies is used, KL's estimated, respectively, for crocidolite mining, amosite insulation manufacture, and mining of vermiculite contaminated with winchite–richterite vary by less than a factor of 3. However, this is possibly fortuitous, given the magnitude of the associated uncertainty intervals ().
Within industries (especially for exposure to a single fiber type), the data are limited. The KL's from studies from the two chrysotile mines (Quebec and Italy) show remarkably close agreement, varying by less than a factor of 2. Those from the three studies involving mining of amphibole asbestos or material containing amphibole asbestos (Wittenoom and the two studies of Libby) also vary only by about a factor of 3. However, the value for amphibole asbestos mining (Wittenoom) is approximately 40 times that for chrysotile mining (Quebec). Moreover, based on their respective uncertainty intervals, the KL's for these two mining operations are incompatible ().
Two of the largest KL's both come from textile mills, one involving primarily chrysotile (South Carolina) and one involving mixed exposures (Pennsylvania). Across all asbestos types (including mixed), the asbestos-cement pipe industry appears to show the greatest variation, although the uncertainty intervals associated with these studies are wide. The two KL's from manufacture of friction products are among the smallest (including one zero value), although the associated uncertainty intervals are wide. The two mixed-fiber textile plants show KL's that vary by no more than a factor of 5 from each other or from the KL for the South Carolina textile plant (where exposure was primarily to chrysotile).
Mesothelioma Potency Factors
The KM's () and the associated uncertainty bounds () are plotted in using the same format as in . As in , data from the Hein et al. (Citation2007) study are used to represent the South Carolina textile cohort. Also, the estimate of KM for the Quebec miners is based on the combined data from the Asbestos and Thetford Mines.
FIG. 12 Plot of estimated KM values and associated uncertainty intervals by study environment (from ).
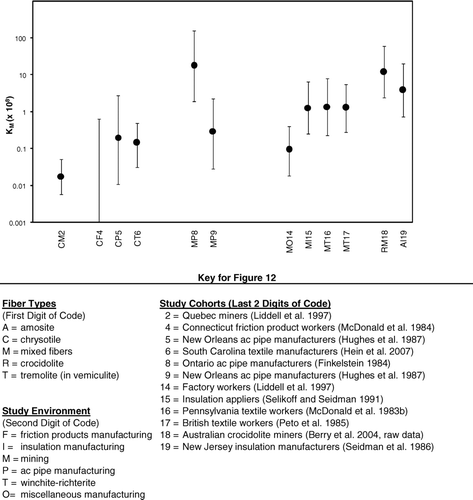
As indicates, the lowest and highest of the KM's differ by a factor of approximately 1,500 (excluding the one negative study of Connecticut friction product manufacturers, which would make the range infinite), and many of the pairwise sets of uncertainty intervals do not overlap. For example, none of the uncertainty intervals for the KM's derived for any of the environments involving predominantly exposure to chrysotile overlap the uncertainty intervals associated with the KM's derived for either crocidolite mining or asbestos-cement manufacture using mixed fibers at the Ontario plant (Finkelstein Citation1984). Furthermore, the uncertainty interval for the KM derived for chrysotile mines in Quebec does not overlap the uncertainty intervals for any of the amphibole asbestos environments or any of the mixed environments, except for the Quebec factory that is associated with the mine in Asbestos, Quebec (Liddell et al., Citation1997).
The KM's for the two “pure” amphibole asbestos studies (crocidolite mining and amosite manufacturing) agree to within a factor of 3 and, with the exception of the value from the Ontario asbestos-cement plant, are the largest values of KM. Also, with the exception of the Ontario plant and the factory associated with the Asbestos, Quebec mines, the KM's from mixed exposure environments lie between the KM's for the “pure” amphibole asbestos exposures and the KM's for the predominantly chrysotile environments. Although the KM for the Ontario asbestos-cement plant appears high, its corresponding uncertainty interval overlaps those of the other “pure” amphibole asbestos studies as well as those of a number of the other studies involving mixed exposure. The KM reported for the factory associated with the Asbestos, Quebec, mine is the lowest of those reported for mixed exposures, but its uncertainty interval overlaps those for several of the other mixed exposures, as well as all of those involving predominantly chrysotile exposures. Except for the Quebec mines and mills, the smallest KM comes from the manufacture of friction products at the Connecticut plant which used predominantly chrysotile.
Within predominantly chrysotile studies alone, lowest and highest KM's vary by approximately a factor of 16 (excluding the negative friction products study), which is a relatively small amount of variation compared to the spread across all of the studies in . Also, the uncertainty intervals for the chrysotile studies all overlap.
As with KL's, the asbestos-cement pipe industry shows the greatest variation in KM's across all asbestos types. The KM from the Ontario plant (Finkelstein, Citation1984) being about 90 times larger than those from the New Orleans plants (Hughes et al., Citation1986), and the uncertainty intervals do not overlap. Within the textile industry, the KM for the South Carolina plant (primarily chrysotile exposure) is about nine times smaller than the values for the two textile plants that processed mixed fibers, although there is considerable overlap in their uncertainty intervals.
DISCUSSION
Lung Cancer and Mesothelioma Potency Factors
In this article, lung cancer potency factors (KL's) were developed from 20 studies covering 18 locations, compared to 13 locations covered in the EPA 1986 update. Mesothelioma potency factors (KM's) were developed for 12 locations compared to four locations in the EPA 1986 update. In addition, nine of the KL's and all four of the KM's developed in the EPA 1986 update were revised using results from more recent follow-up. Although the 4 locations used to calculate KM's in the EPA 1986 update include 1 location with exposure primarily to amosite (Paterson, NJ, factory) and 3 with exposures to mixed fiber types, the 14 KM's derived in the present analysis include 6 locations in which exposures were primarily to chrysotile and one (Wittenoom) where exposures were only to crocidolite. Thus, this work provides substantial new information on the relative potency of different types of asbestos in causing lung cancer and mesothelioma.
Although generally similar, our estimates of KL and KM differ from those in the EPA 1986 update, even when based on the same data ( and ). Reasons for the differences include different treatments of background lung cancer relative risk and use in the EPA 1986 update of weighted linear regression to fit the models, whereas maximum likelihood based on a Poisson assumption was employed in the present analysis.
Published data are usually limited, and often inadequate, for calculating a mesothelioma factor, KM. The published data for mesothelioma generally consist, at best, of tables of observed numbers of mesothelioma deaths and person-years of observation categorized by time since first exposure. To use such data in the mesothelioma model [Eq. (4)], both an average duration of exposure and an average exposure level have to be assumed. In the present analysis, the availability of raw data from three cohorts obviated the need for such assumptions and allowed maximum flexibility in fitting the mesothelioma model and testing related hypotheses. This illustrates that access to raw data can be very useful for exploring issues not fully addressed in the published reports and such access should be encouraged, particularly for studies supported by public funds.
Limitations in the published mesothelioma data not only limit the number of studies available for modeling, but also cause bias because studies that find fewer mesotheliomas are less likely to report the needed data. We have attempted to avoid this problem in the present analysis by going to extra lengths to calculate KM's from some studies with limited data (e.g., see Appendix B, Tables B4, B8, and B22). Even so, for seven of the locations for which KL's were calculated, the available data would not support calculation of a KM.
The uncertainty intervals we report for KL and KM are intended to reflect, in addition to statistical variation, other forms of uncertainty that are difficult to quantify, such as model uncertainty and uncertainty in exposure estimates. These uncertainty intervals are interpreted informally as providing an overall uncertainty range for each KL or KM that seems reasonable, based on the data available for a given study. The degree of overlap of the uncertainty intervals provides an indication of the extent to which KL's or KM's from different studies are in agreement.
The KM's developed in the present analysis show evidence of a trend, with lowest KM's obtained from cohorts exposed predominantly to chrysotile, highest KM's from cohorts exposed to amphibole asbestos, and with KM's from cohorts exposed to mixed fiber types being intermediate between the KM's obtained from chrysotile and amphibole asbestos environments ().
For lung cancer, the conclusions are less clear (). Although there is some evidence of larger KL's from amphibole asbestos exposure, there is a good deal of dispersion in the data and one of the largest KL's is from the South Carolina textile mill, where exposures were almost exclusively to chrysotile.
In fact, there appears to be an association between KL's and industry type that may be as strong as (or stronger than) that with fiber type. For example, KL's from the three textile operations (one involving predominantly chrysotile exposure and two involving mixed exposures) are compatible with each other (despite the narrow uncertainty interval for the South Carolina study) and are all among the highest of the KL's observed. In contrast, two of the smallest KL's are from the two sites involved with manufacture of friction products (one involving predominantly chrysotile exposure and the other involving mixed fiber exposure). Although one of these is in fact a negative study (with a KL of zero), they too are nevertheless compatible due to the broad uncertainty intervals derived for these studies.
Differences in KL's from different industries could be a reflection of different fiber sizes in the raw product processed in the different industries. For example, textiles require a higher commercial grade of fibers (containing visibly longer fibers) than friction products. Consequently, workers in textile mills were likely exposed to longer fibers (even among the microscopic fibers that are respirable) than those in plants that produced friction products.
One exception to the general compatibility of KL's within industry types occurs in the mining industry where the KL reported for chrysotile mining is substantially smaller than the KL's either for mining of crocidolite (with which it is statistically incompatible) or mining of vermiculite (containing winchite-richterite asbestos). Thus both size and fiber type may be important determinants of asbestos lung cancer potency.
Among the largest and clearest discrepancies noted, the KL for chrysotile mining in Quebec is considerably smaller than, and incompatible with, the KL for the South Carolina textile mill that processes predominately chrysotile obtained from the Quebec mines. The most promising hypothesis to account for this incongruent finding is differences in fiber dimensions in the two environments (see, for example, Berman and Crump, Citation2003, Appendix D, and Stayner et al., Citation2007). Moreover, in addition to the higher concentration of long fibers in dusts from textile operations, dusts in mining environments likely contain many particles that are included in PCM counts, but may be either too thick to be respirable, or too short or thick to be biologically active. Thus, PCM-derived exposure estimates in mining may be somewhat inflated relative to those from other industries.
The exposures upon which the KL's and KM's in and are based are determined by PCM, which assumes that all asbestos structures longer than 5 μm and with an aspect ratio ⩾3:1 are equally potent and structures in all other size ranges are nonpotent. It also assumes that potency is independent of type of asbestos. The incompatibility of KL's or KM's estimated from different environments may be due to the fact that this index of exposure does not correlate well with biological activity. There is evidence from studies in both animals (Stanton et al., Citation1981; Davis et al., Citation1988; Berman et al., Citation1995) and humans (Stayner et al., Citation2007) that potency increases with increasing fiber length. And there is epidemiological evidence, including that obtained herein, of differences between the potency of chrysotile and amphibole asbestos, particularly with respect to mesothelioma ().
Questions concerning the affect of fiber size and type are investigated more systematically in the accompanying article (Berman and Crump, Citation2008). In that article, KL's and KM's determined by fitting data across multiple studies are allowed to depend both on the mineralogical types and on the sizes (e.g., lengths and widths) of asbestos fibers. This is accomplished by utilizing fiber size distributions from the literature to enable KL's and KM's to be defined in terms of fibers sizes other than those determined by PCM. An ultimate goal of this work is to identify indices of exposure that correlate better with biological activity and consequently bring the KL's and KM's into closer agreement. If exposure indices can be found that would reconcile KL's and KM's from all studied environments, that would increase our confidence that these same indices and the corresponding KL's and KM's would also apply to unstudied environments.
The Adequacy of the Lung Cancer and Mesothelioma Models
In each of the studies for which a KL was calculated, the lung cancer model [Eq. (2)], which assumes relative risk is linearly related to cumulative exposure lagged 10 years, provided an adequate fit to the lung cancer data categorized by cumulative exposure. However, of the 17 studies for which α was estimated (see tables in Appendix B pertaining to lung cancer) α was estimated to be >1 in 14 studies and significantly so in 8 studies, compared to only one study in which α was found to be significantly <1. The estimates of α do not appear to be related by mineral type, by industry, or by the size of the study.
These results are not inconsistent with those reported by Lash et al. (Citation1997) from their evaluation of 21 studies, including many of the same studies that are evaluated herein. Lash et al. report values for α derived from the vast majority of these studies to be slightly greater than 1 and indicate that under a random effects model the maximum likelihood estimate for α across all of the studies is 1.36, which is significantly greater than 1.
The two most likely causes for α's larger than 1 are (1) higher smoking rates in the studied cohort than in the comparison population and (2) errors in exposure estimation. Although certain worker populations smoke more than the general population (see, e.g., Bang and Kim 2001), it seems unlikely that difference in smoking rates alone would cause an α larger than about 1.5. Importantly, an elevated α due to differences in smoking rates is not expected to cause KL to be biased, so long as smoking rates are not correlated with exposure to asbestos. On the other hand, elevated α's that are attributable to errors in exposure would tend to cause the corresponding KL's to be biased downward (Carroll et al., Citation2006). In the present work, estimates of KL made with α simultaneously estimated were smaller than estimates of KL made with α fixed at 1 by as much as an order of magnitude. Thus, this is an important issue for quantifying lung cancer risk and further study is needed to disentangle the relative effects of different causes of elevated α's. This problem was addressed informally in the present analysis both by not allowing extremely large value of α (restricting α to be ⩽2) and by using the most extreme lower and upper statistical confidence bounds obtained from either estimating α or fixing α= 1 to quantify statistical uncertainty.
Only a few studies have information on smoking and that which is available is often limited to a subset of the cohort and, even for that subset, provides only limited information such as knowledge of whether or not a subject was a smoker at a particular point in time. None of the calculations of KL reported herein took into account smoking rates in the cohort. Since the EPA lung cancer model [Eq. (2)] assumes that the effect of asbestos is to multiply the background rate (including that part of the background contributed by smoking), this model effectively assumes a multiplicative relationship between smoking and asbestos. However, recent studies (Liddell, Citation2001; Liddell and Armstrong, Citation2002; Berry and Liddell, Citation2004) have concluded that the relationship is intermediate between additive and multiplicative. The most serious effect of this model misspecification (i.e., assuming a multiplicative relationship when the true relationship is intermediate between additive and multiplicative) would probably be to overestimate the asbestos-induced risk somewhat in smokers and underestimate the risk in nonsmokers. Although we have not investigated this issue herein, we believe that it is unlikely to cause serious errors in relative values of KL's, which is the focus of the present work.
The EPA model for mesothelioma assumes that the mesothelioma mortality rate varies linearly with the intensity of exposure. Published studies rarely present the information needed to study this assumption and we are not aware of a previous analysis of this issue that is comparable to the one presented herein ( and ). Access to raw data from the cohort exposed to crocidolite in the mines and mills at Wittenoom, Australia (Berry et al., Citation2004), three subcohorts exposed to chrysotile in the mines and mills in Quebec, Canada (Liddell et al., Citation1997), and the cohort exposed primarily to chrysotile at a textile plant in Charleston, SC (Hein et al., Citation2007), allowed us to formally test the linearity assumption in these cohorts. A supralinear exposure response was found in all five cohorts (), and the departure from linearity is statistically significant for Wittenoom (p< .0001) Asbestos, Quebec (p= .02), and Thetford Mines, Quebec (p< .0001).
Hodgson and Darnton (2000) also found evidence that the exposure response for plural mesothelioma is supralinear, but the responses for both lung cancer and peritoneal mesothelioma appeared to be sublinear (e.g., risk increasing with exposure raised to a power >1). However, unlike the present work, their conclusions were based on a meta-analysis that looked across studies, rather than analyzing the exposure responses within studies. A more complete comparison of our results with those of Hodgson and Darnton (2000) is presented in the companion to the present article (Berman and Crump, Citation2008).
Further study to more rigorously elucidate the nature of the mathematical relationship between asbestos exposure and mesothelioma appears warranted. Among other things, it will be important to evaluate the degree that exposure error may be contributing to the appearance of supralinearity. At the same time, if the true relationship for mesothelioma is supralinear, this would mean that risks at low exposures are larger than would be predicted by the linear model. Such a finding could have important implications upon efforts to quantify risk in environmental settings where exposures are much lower than in the occupational settings used to develop the exposure-risk models.
As noted earlier, there is no convincing evidence from this analysis of any serious error in the risk versus time predictions of either the lung cancer model or the mesothelioma model after exposure ends (– and –). It is particularly noteworthy that for chrysotile exposure there is no evidence that the elevated lung cancer relative risk does not remain constant following the end of exposure [as predicted by the lung cancer model, Eq. (2)] at least up through 50 years following the end of exposure (). This contradicts suggestions by Walker (Citation1984) that the relative risk of lung cancer eventually begins to decrease after a sufficiently long period of time past the end of exposure. Likewise, there is no evidence to suggest that mesothelioma risk for chrysotile exposure does not continue to rise after exposure ends as approximately the square of time since first exposure lagged 10 years [as predicted by the mesothelioma model, Eq. (5)], at least up through 55 years following the end of exposure (). These results are particularly noteworthy given the substantial evidence of a much shorter biological half-life for chrysotile than for amphibole asbestos (for example, Berman and Crump, Citation2003, Section 6.2). They suggest that, to the extent that biological persistence does affect the potency of asbestos (and other fibers), it must be through a mechanism that does not affect the time dependence of the response.
Peto et al. (1982) proposed a model for mesothelioma in which the mortality rate is proportional to t3.2, where t is unlagged time since first exposure, as opposed to the rate being proportional to (t – 10)2, as predicted by the EPA model [Eq. (3)]. However, these two functions, when fit to data, are virtually indistinguishable for 0 ⩽t⩽ 50 years.
Regarding the time development of mesothelioma following exposure to amphibole asbestos, McDonald et al. (Citation2006) found 45 deaths from mesothelioma among a group of 1,061 persons (mainly women) who, between 1940 and 1944, worked in a factory that assembled gas masks with filter pads containing 20% crocidolite and were followed through 2003. After about 20 years from first exposure the mesothelioma mortality rate increased for the next 10 years but then remained fairly steady for the next 30 years. No mesotheliomas were observed in the last 8 years of follow-up between 1996 and 2003. If the rate has remained the same as in 1991–1995, 5 more would have been expected (p= .0065). Thus, this study suggests that the mesothelioma rate does not increase indefinitely as predicted by the mesothelioma model [Eq. (4)] but remains constant between about 25 and 55 years following the end of exposure and decreases thereafter. These results are at variance with results obtained herein for Wittenoom miners exposed to crocidolite for whom the mortality rate for mesothelioma was found to increase steadily with increasing years from last exposure up through 45 years after last exposure (). Unlike our analysis, that of McDonald et al. did not adjust for potential differences in exposure in different time ranges. This could possibly explain some of the discrepancy, although it seems unlikely that it would fully explain it.
APPENDIX A: GUIDELINES FOR QUANTIFYING EFFECT OF NONSTATISTICAL SOURCES OF UNCERTAINTY UPON KL AND KM
To quantify the effect of nonstatistical sources of uncertainty upon KL and KM, distinct sources of uncertainty were identified and guidelines were developed for assigning “uncertainty factors” to address quantitatively each source of uncertainty. Three factors (F1, F2, and F3) address sources of uncertainty associated with exposure. An additional factor (F4L for lung cancer and F4M for mesothelioma) accounts for uncertainty due to special limitations in the data available for estimating disease-specific potency factors in particular studies. The basis for assigning these factors and how they are combined into an overall uncertainty factor is described in this appendix. The assignment of values to factors in individual epidemiological studies is described in Appendix B. These values are summarized in .
To define the factors used to address uncertainty associated with exposure, it was first considered that, ideally, cumulative exposure would be estimated in an epidemiological study by:
Continuously monitoring the concentrations to which the worker is exposed over his or her entire working life.
Measuring such concentrations using personal monitors (samplers worn by workers with sampling ports placed within a few inches of the breathing zone of the worker).
Analyzing samples in a manner appropriate for determining the concentration of the specific range of fibers of interest.
In practice, however, measurements were commonly collected only periodically at fixed locations considered representative of worker exposures for jobs performed at that location (local operations). Moreover, measurements were frequently derived using analytical methods that report results in units different from those of interest, so that some type of conversion was required. Then, typically, cumulative exposures were estimated for individual workers as the sum over discrete intervals of employment of the product of the mean exposure concentration during that interval and the duration of the interval, with the different intervals being defined either by specific jobs or differences in exposures assigned to a particular job. Under these conditions,where:
CEPCM is the (age-specific) cumulative exposure to fibers measured by PCM (f-yr/ml);
Q is a factor that converts concentration measurements in a particular study to PCM fiber concentrations whenever the measurements in the study were collected using a different method (usually dust concentrations determined by midget impinger in units of million particles per cubic foot, mppcf, in which case the units of Q are f/ml/mppcf).
CLOj is the concentration estimated for a particular time interval for a particular “local operation”, typically derived by a combination of measurement and extrapolation.
Dj is the duration of time interval “j.”
Based on Eq. (A1), a factor, F1, is defined to account for uncertainty introduced in the manner that the CLO's were determined in specific epidemiological studies; a factor, F2, is used to address uncertainty associated with the determination of the conversion factors, Q, for specific studies; and F3 is defined to represent uncertainty in the manner that job matrices were developed in specific studies to assign workers to specific local operations over specific durations. The guidelines used to assign values to each uncertainty factor are described next.
The Factor F1
This factor represents the uncertainty in concentrations to which workers were exposed (in whatever units of exposure that were reported in a particular study). In addition to analytical uncertainty, considerations addressed when assigning values for F1 for specific epidemiological studies include:
To what extent exposure concentrations were directly determined from measurements collected at the locations and times that worker exposures actually occurred.
Whether measurements were derived from personal monitoring or from area monitoring.
Regarding the latter consideration, exposure concentrations estimated in the published epidemiological studies were almost universally determined by area, rather than personal monitoring. As reported in several of these studies (see, for example, McDonald et al., Citation1983b), area monitoring can miss short-term, high-level exposures contributed by the personal actions being performed by a worker. Moreover, certain periodic activities potentially associated with extremely high exposures (typically involving cleanup) were not performed during time periods when work areas were routinely monitored. On the other hand, monitoring was not always carried out systematically and appears in some cases to have been performed in areas were exposures were thought to be high.
Regarding the first consideration just listed, published epidemiological studies differ in the frequency and time period over which sampling was conducted. With few exceptions, little or no sampling was conducted prior to the 1950s when exposure concentrations are thought generally to be higher than those monitored more recently, due to lack of use of dust control equipment and dust suppression procedures that were introduced only later. For many studies, therefore, early exposures had to be estimated by extrapolation from later measurements and the care with which such extrapolations were performed also varies from study to study.
Studies vary in the degree to which the local operations associated with a particular facility were individually sampled. Exposures in jobs not sampled directly would then be extrapolated from measurements collected for other local operations assumed to be associated with “comparable exposures.” As with extrapolations in time, the care with which such spatial extrapolations were performed varies from study to study.
Values assigned for F1 in individual studies () vary between 1.5 and 4 (all to the nearest 0.5). The most typical value assigned was 2 for studies in which additional uncertainty was introduced due to use of area samplers rather than personal samplers, lack of measurements representative of episodic but high-exposure jobs (usually associated with cleanup), and lack of direct measurements from the earliest periods of exposure (when dust control equipment and procedures were less effective). For a study to be assigned a value of 2, however, the authors must have had access to substantial numbers of samples representative of the majority of the local operations of interest, must have described a systematic procedure for extrapolating exposure estimates to less well studied local operations, and must have described a systematic procedure for extrapolating exposure estimates to earlier times when measurements were lacking.
The Factor F2
This factor is used to characterize the uncertainty introduced in deriving conversion factors to convert from the exposure indices measured in a particular study to the exposure index typically reported using the membrane filter method (as determined by PCM). In about half of the studies, concentrations were estimated in millions of dust particles per cubic foot (mppcf) as determined by midget impinger. The uncertainty introduced by such conversions varies from study to study because:
For a small number of studies, the majority of measurements were performed by the membrane filter method so that conversion was unnecessary.
For some studies, conversion factors were derived from a statistical analysis of a set of side-by-side measurements determined, respectively, using the membrane filter method and the other method from which measurements need to be converted (typically the midget impinger method).
For some studies, lack of side-by-side measurements required expert judgment for comparing across samples collected at different times and locations.
For some studies, conversion factors were adapted from other studies of similar processes.
Moreover, as has been demonstrated in several studies, the factors used to convert other measurements (primarily midget impinger) to the exposure index determined by PCM vary as a function of study environment, local operation, and time. For example, the ratio of PCM to midget impinger measurements derived from side-by-side measurements in a single study reportedly vary between 0.3 and 30 (McDonald et al., Citation1980a).
Given these issues, the factors used to convert measured concentrations to exposure concentrations in units of interest [Q in Eq. (A1)] ideally should be determined individually for each local operation. However, with the exception of the South Carolina study (Hein et al., Citation2007), only study-wide conversion factors were estimated in any particular study.
Values for F2 assigned to particular studies () vary between 1 and 3. Studies in which conversions were not required (due to routine use of PCM) or studies in which conversion factors were determined for specific operations are assigned an F2 value of 1. Studies in which a study-wide conversion factor was determined from paired measurements are assigned a value of 1.5. Studies in which conversion factors were adapted from other studies or for which authors did not define a conversion factor are assigned larger values for F2.
The Factor F3
This factor is used to represent the uncertainty attributable to the manner in which job-exposure matrices were constructed in the various published epidemiological studies. Authors for some studies had detailed work histories that could be used to identify the complete set of specific jobs that each worker performed over their working life and the duration of time spent on each job. Authors from other studies did not have access to individual work histories so that crude estimates of average duration were applied to all members of the cohort. The factor F3 is used to account for conditions in which less than optimal job histories were used to identify the set of jobs performed by each worker and the duration that each worker spent performing each such job.
The Factor F4L for Lung Cancer and F4M for Mesothelioma
An additional factor is included (F4L for lung cancer and F4M for mesothelioma) to account for uncertainties in mortality data (e.g., when diagnosis was uncertain for a substantial fraction of potential mesothelioma cases) or when approximations or assumptions were required because the data are not presented in the form needed for fitting the exposure-response models. Two assigned F4L values are greater than 1 (1.5 and 2), and six F4M values are greater than 1 (2 to 5.0) ().
Combining Uncertainty Factors into an Overall “Uncertainty Range''
An overall uncertainty factor, F, is calculated as:
This formula is used rather than multiplying the uncertainty factors because it is unlikely that all of the uncertainty sources would cause errors in the same direction in the same study; the individual types of errors considered here can either cause estimates to increase or decrease. The overall “uncertainty range” for each KL () or KM () is calculated by dividing the statistical 95% lower bound by F and multiplying the 95% upper bound by F.
APPENDIX B: CALCULATION OF KL AND KM FROM INDIVIDUAL EPIDEMIOLOGICAL STUDIES AND ASSIGNMENT OF RELATED UNCERTAINTY FACTORS
This appendix provides a brief description of each epidemiological study used to calculate a KL or KM and how these values are calculated. It also describes the specification of uncertainty factors based on the guidelines described in Appendix A. All uncertainty factors not specifically mentioned were assigned a value of 1. The resulting KL and KM estimates and related uncertainty intervals are summarized in and , respectively. The uncertainty factors used in determining the uncertainty intervals are summarized in .
Predominately Chrysotile Exposure
Quebec Mines and Mills
Liddell et al. (Citation1997) extended the follow-up into 1992 of a cohort of about 11,000 workers at chrysotile asbestos mines and related mills in each of 2 areas in Quebec. Details regarding follow-up and exposure estimates for this cohort are presented in the main article. Liddell et al. (Citation1997) categorized cancer deaths after age 55 from lung, trachea, and bronchus by cumulative asbestos exposure to that age (Table 8 in Liddell et al., Citation1997). Standardized mortality ratios (SMRs) were calculated based on Quebec rates from 1950 onward, and Canadian, or a combination of Canadian and Quebec rates, for earlier years. shows the fit of the lung cancer model [Eq. (2)] to these data. Although the models both with α= 1 and α variable provide reasonably adequate fits to the data, the hypothesis α= 1 can be rejected (p= .014). The model with α estimated (α= 1.15) yields a best estimate of KL of 0.00029 (f-yr/ml)−1, 90% CI: (0.00019, 0.00041). With α= 1, the estimate is KL= 0.00041 (f-yr/ml)−1, 90% CI: (0.00032, 0.00051).
TABLE B1 Cancer of lung, trachea, or bronchus by cumulative exposure level among workers in quebec chrysotile mines and mills Liddell et al. (1997)
Our reanalysis of the raw mesothelioma data for this cohort (provided by Dr. Corbett McDonald and Professor Douglass Liddell) is described in the main article. Since workers at the factory located in Asbestos were exposed to substantial amounts of commercial amphibole asbestos, separate estimates of KM were made for the factory workers and for the remaining cohort of miners and millers at Asbestos and at Thetford Mines. For factory workers, the estimate of KM is 0.095 × 10−8, 90% CI: (0.041 × 10−8, 0.18 × 10−8), and for the remainder of the cohort the estimate is 0.018 × 10−8, 90% CI: (0.013 × 10−8, 0.024 × 10−8).
For this study F1 was set equal to 2. This study is the paradigm used to define the typical case in which increased uncertainty can be attributed to use of area rather than personal samplers, lack of measurements early in the study, and lack of direct measurements from certain episodic but high-exposure operations. At the same time, the authors of this study appear to have used the available data in a systematic and objective manner to address the issues raised by the lack of sampling. The uncertainty factor F2 was set equal to 1.5 to reflect use of a conversion factor that was derived from paired samples, but that was based on a project-wide average, rather than addressing variation for specific, local operations.
Italian Mine and Mill
Piolatto et al. (Citation1990) conducted additional follow-up of workers at a chrysotile mine and mill in Italy that was earlier studied by Rubino et al. (Citation1979). The cohort consists of 1058 workers with at least 1 year of employment between 1946 and 1987. Follow-up extended from 1946 through 1987, which is 12 more years of follow-up than in Rubino et al. (Citation1979). Lung cancer mortality was compared to that of Italian men.
As described in Rubino et al. (Citation1979), fiber levels were measured by PCM in 1969. To estimate earlier exposures, information on daily production, equipment changes, number of hours worked per day, et cetera were used to recreate conditions at the plant during earlier years. PCM samples were obtained under these simulated conditions and combined with work histories to create individual exposure histories.
Piolatto et al. (Citation1990) observed 22 lung cancers compared to 11 in the earlier study (Rubino et al., Citation1979). Lung cancer was neither significantly in excess nor was it significantly related to cumulative asbestos exposure. Piolatto et al. (Citation1990, ) presented observed and expected lung cancers (based on age- and calendar-year-specific rates for Italian men) categorized by cumulative exposure in f-yr/ml. The lung cancer model [Eq. (2)] with α= 1 provides a good fit to these data (, p = 0.75) and allowing α to vary did not significantly improve the fit. The KL estimate with α= 1 is 0.00035 (f-yr/ml)−1, with 90% CI: (0, 0.0015). With α allowed to vary (α= 0.937) the estimate is KL= 0.00051 (f-yr/ml)−1 with 90% CI: (0, 0.0057).
TABLE B2 Lung cancer mortality among chrysotile asbestos miners in balangero, Northern Italy Piolatto et al. (1990)
Two mesotheliomas were observed by Piolatto et al. (Citation1990), compared to one found by Rubino et al. (Citation1979). However, data were not presented in a form that allowed KM to be estimated.
Regarding uncertainty factors, F1 was assigned a value of 2 for this study for reasons similar to those described for Quebec. F2 was assigned a value of 1 because measurements were conducted using PCM so that conversion was unnecessary for this study.
Connecticut Friction Product Plant
McDonald et al. (Citation1984) evaluated the mortality of workers employed in a Connecticut plant that manufactured asbestos friction products. The plant began operation in 1913 and used only chrysotile until 1957, when a little anthophyllite asbestos was used. Also, a small amount of crocidolite (about 400 lb) was handled experimentally between 1964 and 1972. Brake linings and clutch facings were made beginning in the 1930s, and production of automatic transmission friction materials, friction disks, and bands was begun in the 1940s.
The cohort was defined to include any man who had been employed at the plant for at least 1 month before 1959, omitting all that had worked at a nearby asbestos textile plant that closed in 1939. This cohort consists of 3,515 men, of whom 36% had died by the end of follow-up (December 31, 1977). Follow-up of each worker was only begun past 20 years from first employment.
Information on dust levels from impinger measurements was available for the years 1930, 1935, 1936, and 1939. There was little other exposure information available until the 1970s. An industrial hygienist used these measurements and information on processes and jobs, environmental conditions, and dust controls to estimate exposures by process and by period in units of mppcf. No conversion from mppcf to f/ml is suggested by the authors, although a conversion factor between 1.4 and 10 is suggested by other studies. The most common value seems to be around 3 f/ml per mppcf, which has been observed in diverse environments such as mining and textile manufacture. This value is provisionally applied to this cohort, although this conversion has considerable uncertainty associated with it.
Total deaths and deaths from most individual causes investigated were elevated; these elevations are due primarily to increased deaths in the group working for <1 year. This pattern holds for lung cancer in particular; the SMR for lung cancer is highest (180) for persons exposed for <1 year. A similar pattern holds when the analysis is carried out by cumulative exposure (); the SMR in the lowest exposure category is higher than in any other category. The linear relative risk lung cancer model [Eq. (2)] provides a poor fit (p= .01) to these data when the Connecticut rates are assumed to be appropriate for this cohort (fixing the parameter α= 1); use of U.S. rates gives similar results. However, the fit is adequate (p= .28) if the background response is allowed to rise above that of Connecticut men (α= 1.49). Although the reason for this increased response in persons that worked for a short period or had low exposures is not clear, the analysis in which the background response is allowed to vary appears to be the most appropriate. This analysis yields an estimate of KL= 0 (f-yr/ml)−1, 90% CI: (0, 0.0017). The analysis with α= 1 yields KL= 0.0019 (f-yr/ml)−1, 90% CI: (0, 0.0061).
TABLE B3 Lung cancer mortality among workers in a chrysotile asbestos friction products plant in connecticut McDonald et al. (1984)
McDonald et al. did not find any mesotheliomas in this cohort. It is useful to determine the range of mesothelioma risk that is consistent with this negative finding. Although McDonald et al. do not furnish data in the form needed for this calculation, these data can be approximated from of McDonald et al. (Citation1984). In this table they list 511 deaths occurring after age 65. Assuming that the overall SMR of 108.5 holds for persons over 65 years of age, the expected number of deaths is 511/1.085 = 471. The death rate in U.S. white males between 65 and 75 years of age is approximately 0.050 per year (from 1971 vital statistics). Therefore the number of person years observed in persons post 65 years of age is estimated as 471/0.050 = 9,420.
A lower bound on the person-years of follow-up between ages 45 and 65 can be estimated by assuming that follow-up was complete for this age group. First, the number of persons that would have had to have been in the cohort to experience the observed deaths was estimated. Assuming that x persons in the cohort were alive at age 45, the following estimates of the number entering each successive 5-year age interval and the corresponding number of deaths (based on death rates in 1971 white males) are obtained.
Since there were 616 deaths in men between the ages of 45 and 65, the expected number of deaths is estimated as 616/1.085 = 567.7 between ages of 45 and 60, and the number of persons entering this age interval is estimated as x= 567.7/0.27 = 2,100. The person-years are then estimated as (2,100) (18.0) = 38,000.
Using the average age of beginning work to be 30.95 years ( in McDonald et al., 1984) yields the data in . Moreover, the average duration of exposure in this cohort is 8.04 years and the average exposure level is 1.84 mppcf ( in McDonald et al., 1984), which is equivalent to 1.84 × 3 = 5.52 f/ml. These data yield an estimate of KM= 0 with 90% CI: (0, 1.2 × 10−9).
TABLE B4 Mesothelioma mortality among connecticut friction product plant workers McDonald et al. (1984)
The best estimate of KM was assumed to be zero. For uncertainty factors, F1 was assigned a value of 2 for reasons similar to those described for Quebec. F2 was assigned a value of 3 for this study because no conversion factor is reported by the authors and an average value of 3 for the range of conversion factors observed among the available studies (U.S. EPA, 1986) was selected for this study. To derive an exposure-response factor for mesothelioma from this study, an upper bound had to be estimated by reconstructing the data because the authors do not provide the data in a form suitable for performing the required calculation. Therefore, F4M was assigned a value of 3 for this study.
While reviewing mesothelioma cases reported to the Connecticut Tumor Registry from 1955 through 1977, Teta et al. (Citation1983) identified three cases of mesothelioma among persons who had been employed by the company that operated the friction products plant. One of these was a man who had worked at a textile plant owned by the same company but had never worked at the friction products plant. The other two cases had worked at the friction products plant. One was a woman with probable pleural mesothelioma who had worked at the plant for 5 years and the other was a woman with a confirmed peritoneal mesothelioma who had worked as a clerk for 30 years (Teta et al., Citation1986). The failure of McDonald et al. (Citation1984) to identify these two cases is apparently due to their reliance upon death certificates for cause of death, whereas Teta et al. identified mesothelioma cases using the Connecticut Tumor Registry and confirmed most of them, including the two in question, from review of pathologic material. The information needed to include these two cases in the current analysis is not available because the McDonald et al. (Citation1984) published data was limited to men. However, it should be noted that the 95% statistical upper bound on KM for this cohort (1.2 × 10−9) predicts about two mesotheliomas among male workers. Also, the upper bound of the uncertainty range for KM for this cohort (6.5 × 10−9), which includes the factor F4M = 3 for uncertainty in diagnosis of mesothelioma, predicts about 10 mesotheliomas among male workers. Thus, it seems unlikely that an analysis that includes the additional two mesotheliomas found in women would have a large effect upon the overall conclusions of this analysis.
New Orleans Asbestos-Cement Plants
Hughes et al. (Citation1987) report on follow-up through 1981 of a cohort of Louisiana workers from two asbestos cement plants studied previously by Weill et al. (Citation1979). Although chrysotile, amosite, and crocidolite were used at these plants, a group of workers at one of the plants was exposed only to chrysotile. The cohort contains 6,931 workers, of whom 95% were traced, compared to a 75% success in tracing by Weill et al. (Citation1979). This improved trace is the result both of greater access to Social Security Administration records and greater availability of computerized secondary information sources (Dr. Hughes, personal communication).
Both of the plants have operated since the 1920s. Chrysotile was used predominantly in both plants. Some amosite was used in Plant 1 from the early 1940s until the late 1960s, constituting about 1% of some products, and crocidolite was used occasionally for approximately 10 years beginning in 1962. Plant 2 utilized only chrysotile, except that pipe production, which began in 1946 and was housed in a separate building, produced a final product that contained about 3% crocidolite. Since the total percentage of asbestos fiber in most asbestos cement products ranges from 15% to 28%, it is estimated that crocidolite constituted between 10% and 20% of the asbestos used to make cement pipe (Dupré; Ontario Royal Commission, Citation1984). Workers from Plant 2 that did not work in pipe production were exposed only to chrysotile.
Estimates of airborne dust levels were made for each job by month and year from midget impinger measurements initiated in the early 1950s. Levels estimated from initial samples in the 1950s were also assumed to hold for all earlier periods because no major dust control measures had been introduced prior to that time. New exposure data from Plant 2 became available after the earlier study (Weill et al., Citation1979) was completed and these, along with a complete review of all the exposure data, were used to revise the previous estimates of exposure. In Plant 1 the earlier and revised estimates are reasonably similar, but in Plant 2, the revised estimates tend to be about one-third of the previous estimates through the 1940s and about one-half the previous estimates thereafter. Based on 102 side-by-side measurements by midget impinger and PCM in various areas of one of the plants, Hammad et al. (Citation1979) estimated an overall conversion factor of 1.4 f/ml per mppcf. There are substantial variations in this factor among different areas of the plant.
The principal cohort studied consists of all workers who, according to company records, were employed for at least 1 month prior to 1970, had a valid Social Security number, and were first employed in 1942 or later (Plant 1), or in 1937 or later (Plant 2). Mortality experience was compared with that expected based on Louisiana rates.
Hughes et al. found no significant difference between the exposure responses for lung cancer in Plant 2 among workers exposed to chrysotile only and those who were also exposed to crocidolite in pipe production. A single lung cancer exposure response model adequately describes the lung cancer data from Plants 1 and 2 combined (p= .42, ). The fit of this model is good when Louisiana men are assumed to be an appropriate control group (fixing the parameter α= 1). This fit provides an estimate of KL= 0.0040 (f-yr/ml)−1, 90% CI: (0.0015, 0.0070). With α allowed to vary (α= 1.14), the estimate is 0.0025 (f-yr/ml)−1, 90% CI: (0, 0.0066).
TABLE B5 Lung cancer mortality among workers employed in two asbestos cement manufacturing plants in New Orleans, Louisiana Hughes et al. (1987)
Six mesotheliomas were identified in the primary cohort studied by Hughes et al., 2 in Plant 1 and 4 in Plant 2. Four other mesotheliomas are known to have occurred, one among those initially employed in Plant 2 before 1937 and 3 among Plant 2 workers shortly after follow-up ended in 1981. A case control analysis conducted among Plant 2 workers found a relationship between mesothelioma risk and length of employment and proportion of time spent in the pipe area after controlling for length of exposure, which is consistent with a greater risk of mesothelioma from crocidolite exposure.
Data are not presented in the paper in the form required for estimating KM. However, Hughes and Weill (Citation1986) present estimates of mesothelioma potency from several data sets, including the cohort studied in Hughes et al. and containing six mesotheliomas, but using a model slightly different from the one used herein [Eq. (4)]. Estimating KM by multiplying the potency estimated by the Hughes and Weill (Citation1986) model by the ratio of the potency values estimated for another study using Eq. (4) and the Hughes–Weill (Citation1986) model yields the following estimates of KM for the Hughes et al. (Citation1987) data: 0.25 × 10−8 (Selikoff et al., Citation1979); 0.21 × 10−8 (Dement et al., Citation1983b); 0.27 × 10−8 (Seidman et al., Citation1979); and 0.43 × 10−8 (Finkelstein Citation1983). Based on these calculations, KM= 0.30 × 10−8 seems to be a reasonable estimate for the Hughes et al. cohort.
It would be worthwhile to estimate mesothelioma risk using additional follow-up that includes the three cases that occurred shortly after follow-up ended. However, such an estimate should be no larger than about KM= 0.45 × 10−8. This is because, since there are 6 mesotheliomas in the cohort studied by Hughes et al., even if the additional person years of follow-up post-1981 are not taken into account, the 3 additional mesotheliomas would increase the estimate of KM by only about 50%.
The finding by Hughes et al. (Citation1987) of an association with crocidolite exposure implies that a smaller KM would correspond to the chrysotile-only exposed group in Plant 2. Although the Hughes et al. report doesn't furnish the data needed for precise estimation of KM from this cohort, it was possible to make some reasonable approximations to this KM. Since none of the 6 mesotheliomas occurred among workers exposed only to chrysotile, KM= 0 would be the point estimate derived from the data used by Hughes et al.
However, one mesothelioma was discovered in a person whose employment began in 1927 and thus was not eligible for inclusion in the cohort. This person was employed continuously for 43 years in the shingle production area, where only chrysotile was used. In an attempt to compute an alternate KM using this one case, it was noted that the duration of observation of the Hughes et al. cohort is roughly equivalent to that of the Dement et al. (Citation1983b) cohort. If the person-years from this cohort, categorized by years since first exposure, are adjusted by the ratio of the sizes of the Dement et al. cohort and the Hughes et al. non-crocidolite-exposed cohort from Plant 2, one mesothelioma is assumed to occur (in 30+ years from first exposure category) and the average duration of exposure (2.5 years) and fiber level (11.2 f/ml) appropriate for the Hughes et al. cohort are applied to these data, a value of KM= 0.2 × 10−8 is obtained.
The best estimate of KM is assumed to be 0.2 × 10−8 for workers exposed only to chrysotile and 0.3 × 10−8 for workers exposed to both chrysotile and amphibole asbestos. The number of mesotheliomas observed here is small (one for the predominantly chrysotile plant and three or six for the mixed plant) and similar to the number of mesotheliomas observed among South Carolina textile workers (three cases). Therefore, it was assumed that a statistical confidence interval similar to that observed among the South Carolina cohort would also apply to the two subcohorts from this study. The lower bounds were constructed for the two subcohorts in this study by dividing their point estimates by the ratio of the South Carolina point estimate to its lower bound. Similarly, upper bounds were constructed for each subcohort by multiplying their point estimates by the ratio of the upper bound to point estimate from the South Carolina study.
For uncertainty factors, F1 was assigned a value of 2 for reasons similar to those described for Quebec. F2 was assigned a value of 1.5 because most early measurements were collected by midget impinger and the authors report using a conversion factor of 1.4 derived from paired measurements. Due to the lack of adequate data for estimating both the overall mesothelioma rate and a confidence interval for such rates and the consequent need to reconstruct the data (incorporating numerous assumptions) to be able to obtain the needed estimates, a value of 5 was assigned to the factor F4M for chrysotile exposures and 2.5 for mixed exposures.
South Carolina Textile Factory
The latest update of this cohort (Hein et al., Citation2007) is described in the main article. Although the investigation of how well the lung cancer model described these data was based only on data for white males, for the purpose of estimating KL and KM all the data were used. shows observed and expected deaths for lung cancer among white males, black males and females, categorized by cumulative exposure lagged 10 years (Hein et al., Citation2007, ). The mean values for cumulated exposure are the person-year-weighted values calculated from the raw data in the present analysis.
TABLE B6 Lung cancer mortality among chrysotile asbestos textile workers in Charleston, South Carolina Hein et al. (2007)
The fit of the model with α= 1 is marginal (p= .09), and the hypothesis that α= 1 is rejected (p= .008). The estimate of KL with α= 1 is 0.030 (f-yr/ml)−1, 90% CI: (0.023, 0.037), and the estimate with α variable (α= 1.35) is KL= 0.018 (f-yr/ml)−1, 90% CI: (0.011, 0.027).
Hein et al. (Citation2007) reported three deaths from mesothelioma, and Hein graciously identified these for us in the data files. Applying the method described in the main article for calculating KM from raw data, the following estimate was obtained: KM= 0.15 × 10−8, 90% CI: (0.047 × 10−8, 0.33 × 10−8).
Regarding uncertainty factors, F1 was assigned a value of 1.5 for this study to give credit for the reasonably complete sampling coverage of exposures by a combination of midget impinger and extensive PCM measurements and the formal statistical evaluation conducted to derive job-specific exposure estimates. However, the exposure estimates were still based on analyses of area rather than personal samples. Because multiple factors were used to convert midget impinger measurements to PCM measurements based on side-by-side samples collected from specific areas (associated with specific operations) within the plant, a value of 1 was assigned for F2 for this study. The treatment of statistical confidence limits described above was considered adequate to account for the uncertainty in the number of mesotheliomas, and a value of F4M = 1 was assigned.
McDonald et al. (Citation1983a) conducted a cohort mortality study in the same South Carolina textile plant. Their cohort consists of all men employed for at least 1 month before 1959 and for whom a valid Social Security record existed. This cohort consists of 2,410 men, of whom 36% had died by the end of follow-up (December 31, 1977). Follow-up of each worker was begun past 20 years from first employment.
McDonald et al. (Citation1983a) had available the same exposure measurements as Dement et al. (Citation1983b) and used these to estimate cumulative exposures for each man in mppcf-yr. In their review of the environmental measurements in which both dust and fiber concentrations were assessed, they found a particle to fiber conversion range of from 1.3 to 10.0 with an average of about 6 f/ml per mppcf. This value, which is intermediate between the values of 3 and 8 found by Dement et al. (Citation1983b) for different areas of the same plant, was used in the calculations involving the McDonald et al. (Citation1983a) study.
McDonald et al. describe two practices at the plant that entailed very high exposures and that were not reflected in either their estimates or those of Dement and coworkers: cleaning of burlap bags used in the air filtration system by beating them with buggy whips during the years 1937–1953, and the mixing of fibers, which was carried out between 1945 and 1964 by men with pitchforks and no dust suppression equipment.
A strong exposure response for lung cancer was observed (), which parallels the results of Dement et al. (Citation1994). Unlike Dement et al., McDonald et al. used South Carolina men as the control group rather than U.S. men. Use of this control group provides an adequate description of the data. Lung cancer potency values estimated both with α= 1 and allowing α to vary provides excellent descriptions of the data (p= .88) and the hypothesis α= 1 could not be rejected (p= .80). Assuming α= 1 results in KL=0.012 (f-yr/ml)−1, 90% CI: (0.0075, 0.016), and when α was allowed to vary, KL=0.010 (f-yr/ml)−1, 90% CI: (0.0044, 0.025). These results are reasonably consistent with the potency estimated from Dement et al. (Citation1994), and the differences can be largely accounted for by the different assumptions regarding the fiber/particle ratio.
TABLE B7 Lung cancer mortality among workers in a chrysotile asbestos textiles plant in South Carolina McDonald et al. (1983a)
McDonald et al. (Citation1983a) found one case of mesothelioma in this cohort—apparently the same one discovered by Dement et al. (Citation1983b): a man born in 1904 who died in 1967 and worked at the plant for over 30 years. Since this study was conducted exactly as McDonald et al. (Citation1984), the same method used there to reconstruct person-years by years from first exposure was applied to this cohort as well. The reconstructed data are listed in . The estimated potency MLE is KM= 0.088 × 10−8, with a 90% CI: (0.0093 × 10−8, 0.32 × 10−8).
TABLE B8 Mesothelioma mortality among South Carolina textile plant workers McDonald et al. (1983a)
For uncertainty factors, F1 was assigned a value of 2 for reasons similar to those described for Quebec. F2 was assigned a value of 1 because McDonald et al. essentially used the same data that Dement and coworkers used to estimate conversion factors (see above), although they favored a slightly higher mean value. The values favored by Dement were used in the current analysis when evaluating this study.
Unlike the study by Dement and coworkers (for which the raw data were acquired so that the exposure-response factor and the attendant confidence interval for mesothelioma could be calculated directly), the mesothelioma data published in the McDonald study of this facility were not suitable to estimating confidence bounds. Thus the data had to be reconstructed, which required incorporation of numerous assumptions. To account for the uncertainty associated with the reconstruction, F4M was assigned a value of 3 for this study.
Predominately Amphibole Asbestos Exposure
Wittenoom, Australia, Mine and Mill
There have been several follow-ups of a cohort of 6,904 men and women employed at a crocidolite mine and mill in Wittenoom, Australia (Armstrong et al., Citation1988; de Klerk et al., Citation1994; Berry et al., Citation2004). The data from the latest follow-up through the year 2000, which were provided for this analysis through the courtesy of Nick de Klerk, are described in the main article.
More recent discussion of the exposure characterization associated with this cohort indicates that it may not have been as quantitative as originally envisioned (Rogers and Major, 2002). Based on this citation, many hundreds of total dust measurements (by konimeter) were collected at the Wittenoom mines in the 1950s up through 1966. It was noted, however, that many of these may have been overloaded rendering their utility controversial. It was also noted that these were converted to fiber-equivalents based on a very limited data set of 38 paired samples, which were determined by thermal precipitators (rather than by the membrane filter method itself). These measurements were then linked to a generalized worker/exposure matrix developed based on observations and interviews to rank each category by relative dustiness.
The lung cancer model was fit, both with α= 1 and α estimated, to the data cross-categorized by cumulative exposure lagged 10 years and time since last exposure, and the results were then summed over categories of time since last exposure. The result is shown in . The fit, which is illustrated in , is poor with α= 1 (p< .0001) but good with α estimated (α= 2.81, p= .31) and the hypothesis that α= 1 can be rejected (p< .001). With α= 1, KL= 0.043 (f−y/ml)−1, 90% CI: (0.034, 0.053). Since the estimated α is > 2, the model was refit with α= 2, which yielded KL= 0.011, 90% CI: (0.0074, 0.015).
TABLE B9 Lung cancer mortality among asbestos workers in Wittenoom, Australia, Berry et al. (2004), based on raw data from de Klerk
There were in total 222 deaths from mesothelioma in this cohort. The application of the methodology described in the main paper for fitting the mesothelioma model to raw data yielded KM= 12.2 × 10−8, 90% CI: (10.9 × 10−8, 13.6 × 10−8).
Regarding uncertainty factors, F1 was assigned a value 2.5, due to the apparent limited quality of the dust measurements available to assess exposure. F2 was assigned a value of 3 due to the sparseness of the paired measurements available to determine the conversion factor and the fact that they were not directly based on PCM measurements. Finally, F3 was assigned a value of 1.5 due to the more qualitative nature of the job/exposure matrix developed for this cohort.
Paterson, NJ, Insulation Factory
This study is described in the main article. Seidman et al. cross-categorized lung cancer deaths by eight cumulative exposure categories (f-yr/ml) and seven categories of length of time worked (Table XXXIV in Seidman et al., Citation1986). Although this table apparently was created by categorizing workers by their final cumulative exposure (rather than categorizing person-years of follow-up by the cumulative exposure to that point in time, which is more appropriate for calculating KL), because exposures were brief, this likely makes little difference. Expected numbers of lung cancer deaths were based on age- and year-specific rates for New Jersey white males.
shows the results of applying the lung cancer model [Eq. (2)] to these data, after collapsing the table by summing over lengths of time worked. Results were highly dependent upon whether the background lung cancer mortality rate was assumed to be equal to that predicted by the comparison population of New Jersey white males (equivalent to α= 1). The test for departure from the null hypothesis, α= 1, was highly significant, and the maximum likelihood estimate was α= 3.32. Similarly, the model gave a poor overall fit to the data with α= 1 (p< .001), but the fit was quite good when α was allowed to vary (p= .90). The estimated potency parameter, KL, also is highly dependent upon the assumption regarding the parameter, α. The estimate of KL is 0.062 (f-yr/ml)−1, 90% CI: (0.050, 0.076), when α was fixed at α= 1, and KL is 0.011 (f-yr/ml)−1, 90% CI: (0.0058, 0.019), when α was allowed to vary, a 6-fold difference. Since the estimated α was >2, the model was refit with α= 2. The result was KL= 0.024 (f−y/ml)−1, 90% CI: (0.018, 0.031),
TABLE B10 Lung Cancer Mortality by Cumulative Exposure among Amosite Asbestos Factory Workers in Paterson, New Jersey Seidman et al. (1986)
The lung cancer model [Eq. (2)] was also fitted to the data cross-classified by both cumulative exposure and length of time worked, allowing α to assume a different value in each category of time worked. Although the estimated values of α tend to increase with increasing duration of exposure, allowing different values of α did not significantly improve the fit (p= .64). The reason for this behavior is not clear. There is no indication that workers with shorter durations experienced disproportionately higher mortality, since, as noted earlier, α tended to increase with increasing duration of exposure. Although it is possible that cumulative exposure is not the appropriate exposure metric, it is difficult to envision what metric would predict this response, as long as a linear model is assumed. It is also possible that a linear model for relative risk is not correct and a supralinear model is more appropriate, or that the increased risk is not proportional to the background risk, as assumed by this simple relative risk model. Finally, it is possible that the background rate in this population is significantly greater than that in the comparison population, although it seems unlikely that it could be three times greater as suggested by the model.
Seidman et al. (Citation1986) recorded 17 deaths from mesothelioma in this population. Table III of Seidman et al. categorized mesothelioma deaths and person-years of observation by years since onset of work. To apply the mesothelioma model [Eq. (4)], it is necessary to have estimates of the duration of exposure and level of exposure for each category. Using the categorization of the members of the cohort by duration of work in Table XXIII of Seidman et al., it was estimated that the mean duration of work was 1.5 years. Using data from Table XIV in Seidman et al., an average cumulative exposure was estimated for each category of time from onset of exposure by weighting exposures according to the expected total number of deaths. These averages were divided by 1.5 years to obtain the average fiber concentrations in . The estimated exposure levels decrease with time since onset, which is consistent with higher mortality among more heavily exposed workers.
TABLE B11 Mesothelioma Mortality among Amosite Insulation Workers in New Jersey Seidman et al. (1986)
The mesothelioma model [Eq. (4)] provided an adequate fit to these data (p= .35), although it over-predicted somewhat the number of cases in the highest latency category (>35 years). The estimate of KM was 3.9 × 10−8, 90% CI: (2.6 × 10−8, 5.7 × 10−8).
Regarding uncertainty factors, F1 was assigned a value of 3.5 for this study because exposure concentrations were not measured at this facility at all. Rather exposures were estimated (as described in Lemon et al., Citation1980) based on measurements collected at another facility in Tyler, TX (see later description), that manufactured the same products from the same source of raw materials using some of the same equipment, which was moved from the Paterson plant to the plant in Tyler. Because the measurements collected in Tyler were analyzed by PCM, no conversion factor was required. Thus, F2 was assigned a value of 1 for this study.
Tyler, TX, Insulation Factory
Levin et al. (Citation1998) studied the mortality experience of 1,121 men who formerly worked at a plant in Tyler, TX, that manufactured asbestos pipe insulation. The plant operated from 1954 through February 1972. The plant used the same raw materials and some of the same equipment that were used in the Paterson, NJ, plant that was studied by Seidman et al. (Citation1986). The asbestos used was amosite from the Transvaal region of South Africa. The insulation was manufactured from a mixture that contained 90% amosite asbestos.
Environmental surveys were conducted at the plant in 1967, 1970, and 1971, with average fiber concentrations ranging from 15.9 to 91.4 f/ml. An average exposure of 45 f/ml was assumed for this plant, which is near the middle of the range obtained in the 3 surveys. It is also consistent with average levels assumed for the Paterson, NJ, plant, which operated under very similar conditions.
The cohort consists of 744 white, 305 nonwhite (mostly black), and 72 with missing race and who were assumed to be white, based on hiring practices at that time. For the entire cohort, the median age of first employment was 25 years, and the mean duration of employment was 12.7 months (range of 1 day to 17.3 years). Follow-up was through 1993. Death certificates were obtained for 304 of the 315 men known to be dead. In the mortality analysis only white men were evaluated and follow-up started 10 years after first employment. After additional exclusions of men with missing birth dates or missing employment information, the cohort analyzed in the mortality analysis consisted of 753 former workers, among whom 222 deaths were recorded. These deaths were compared with those expected based on age-, race-, and sex-specific U.S. rates.
There was an excess of deaths from respiratory cancer (SMR = 277, based on 36 deaths, not including 4 deaths from mesothelioma). contains observed and expected numbers of deaths from respiratory cancer, categorized by duration of exposure. Cumulative exposure in f-yr/ml is estimated by multiplying the duration of exposure times the assumed average fiber level of 45 f/ml. There was an excess of lung cancer deaths in the lowest exposure group (23 observed, 8.9 expected), and the model with α= 1 does not fit these data (p< .001). Thus the hypothesis α= 1 is rejected (p< .01). With α= 1, KL= 0.013 (f-yr/ml)−1, 90% CI: (0.0055, 0.022). The KL with α variable (α= 2.48) is KL= 0.0013 (f−y/ml)−1, 90% CI: (0, 0.0060). Since the estimated α is >2, the model was refit with α= 2, with the result, KL= 0.0028 (f−y/ml)−1, 90% CI: (0, 0.0069).
TABLE B12 Lung cancer deaths among asbestos workers in Tyler, Texas Levin et al. (1998)
Four mesotheliomas were reported in this study. However, the data are not presented in a form that would permit application of the mesothelioma model.
Regarding uncertainty factors, F1 was assigned a value of 3 because, although exposure concentrations were measured at this facility, the data are sparse so that only an overall average concentration for the entire plant can be derived. Because the measurements collected were analyzed by PCM, no conversion factor was required. Thus, F2 was assigned a value of 1 for this study.
Libby, MT, Vermiculite Mine
Sullivan (Citation2007) studied the mortality of 1,672 white males who had worked as vermiculite miners, millers, and processors in the vicinity of Libby, MT. As reported by Sullivan, Libby vermiculite is contaminated by asbestiform amphiboles whose respirable fraction consists of 84% winchite, 11% richterite, and 6% tremolite.
Follow-up of the cohort was through 2001 and was for between a minimum of 20 years and >65 years following first exposure. Vital status was determined for 97.8% of the cohort at the end of follow-up. By that time 767 were deceased, 99 of whom died from lung cancer.
A total of 376 impinger samples were available that had been collected during 1950–1969, although only 40 of these were collected prior to 1965. In addition 4,118 PCM samples were available from the period 1967–1982. Exposure estimates for years later than 1968 were based on historical measures of fiber concentrations (f/ml) and those for earlier years were based on concentrations measured by midget impinger (mppcf) and converted to f/ml assuming a conversion ratio of 4 f/ml per mppcf. This conversion factor was derived from 336 impinger samples collected during 1965–1969 and 81 filter samples collected during 1967–1971. Individual cumulative fiber exposure estimates (f-yr/ml) were computed from job-specific exposure estimates and work histories (Amandus et al., Citation1987).
Sullivan (Citation2007) categorized person-years of follow-up into four groups according to cumulative exposure and compared the observed number of deaths from lung cancer in each group with the number expected based on age- and calendar-year-specific rates for U.S. white males. These data are reproduced in . The lung cancer model [Eq. (2)] with α= 1 provides a marginal fit to these data (p= .11) and the hypothesis that α= 1 is rejected (p = 0.02). The fit with α estimated (α= 1.5) is good (p= .97) and the corresponding estimate of KL is 0.0026 (f−y/ml)−1, 90% CI: (0, 0.0076). With α= 1, the estimate is KL= 0.0088 (f−y/ml)−1, 90% CI: (0.0048, 0.013).
TABLE B13 Lung cancer mortality by cumulative exposure among vermiculite miners near Libby, Montana Sullivan (2007)
Sullivan (Citation2007) reported 15 deaths from mesothelioma. Unfortunately these data were not summarized in a manner that allowed applying the mesothelioma model [Eq. (4)].
For uncertainty factors, F1 was assigned a value of 2 for reasons similar to those described for Quebec. F2 was assigned a value of 1.5 because most early measurements were collected by midget impinger and the authors report using a conversion factor of 4 derived from temporally overlapping (but not paired) measurements.
McDonald et al. (Citation1986, Citation2004) also conducted a cohort study of workers at the Libby, MT, vermiculite mine and mill. Their cohort was composed of 406 workers employed for at least 1 year prior to 1963. The latest follow-up (McDonald et al., Citation2004) was through 1998. Vital status was determined for all cohort members and cause of death was determined for all 285 deaths. Cumulative exposures (f-yr/ml) were estimated for each worker using work histories based on 42 job categories, and 1,363 environmental measurements, including samples analyzed by PCM (f/ml) and by midget impinger (mppcf) (McDonald et al. (Citation2004).
A total of 44 deaths from lung cancer were observed (SMR = 240, based on Montana rates). McDonald et al. (Citation2004) used Poisson regression to develop a linear exposure response for lung cancer. They obtained an exposure response slope (equivalent to KL) of 0.0036 (f−y/ml)−1, 95% CI: (0.0003, 0.012). Since this analysis apparently used internally standardized mortality rates, these estimates are equivalent to an application of the U.S. EPA lung cancer model with α estimated. An application of the lung cancer model to their earlier follow-up (McDonald et al., Citation1986) resulted in an estimate of α= 1.91 with a ratio of KL's estimated with α= 1 to α estimated of 2.8 (Berman and Crump, Citation2003). Also, an analysis of the recent follow-up of a different cohort from Libby (Sullivan, Citation2007) estimated α= 1.5 with a ratio of KL's of 4.6 (). Consequently, we multiply the KL values obtained by McDonald et al. for this cohort by 3 to crudely estimate what would be obtained in an analysis with α fixed. The result is 0.011 (f-yr/ml)−1, 95% CI: (0.0009, 0.036).
McDonald et al. (Citation2004) identified 12 deaths from mesothelioma, compared to only 2 identified in their earlier follow-up. These data were analyzed for exposure response using intensity of exposure, cumulative and a type of “residence weighted” exposure. Unfortunately, there is no clear way to use the results of these analyses to fit to the mesothelioma model.
Because this study and the Sullivan (Citation2007) study used virtually the same data and very similar approaches to analysis, the same values were assigned to uncertainty factors for this study that were assigned for the Sullivan (Citation2007) study.
Exposure to Mixed Fiber Types
British Friction Products Factory
Berry and Newhouse (Citation1983) conducted a mortality study of 13,460 workers in a factory in Britain that manufactured brake blocks, brake and clutch linings, and other friction materials. Only chrysotile was used at the plant except for two relatively short periods before 1945 when crocidolite was used in the production of railway blocks.
The cohort consists of all men or women employed at the plant between 1941 and 1977. Follow-up was to the end of 1979 and the mortality experience was examined after 10 years from first exposure. Airborne dust measurements were only available from 1967 onward and these were made using PCM. Fiber concentrations in earlier years were estimated by reproducing earlier working conditions using knowledge of when processes were changed and exhaust ventilation introduced.
Deaths from all causes were less than expected both prior to 10 years from first employment (185 observed versus 195.7 expected) and afterward (432 observed versus 450.8 expected). There is no indication of an effect of employment at the plant upon lung cancer; there are 51 lung cancers >10 years from first employment compared to 47.4 expected. A significant deficit of gastrointestinal cancers was observed after 10 years from first employment (25 observed versus 35.8 expected, p= .04).
A linear exposure-response model relating cumulative exposure and lung cancer was fitted to case-control data presented by Berry and Newhouse. The resulting KL was 0.00058 (f-yr/ml)−1 and the 95% upper limit was 0.0080 (f-yr/ml) −1. This estimate was used as the best estimate of KL, and the lower confidence bound was assumed to be zero.
A case control study on mesothelioma deaths showed that 8 of the 11 cases had been exposed to crocidolite and another possibly had intermittent exposure to crocidolite. The other two cases had been employed mostly outside the factory and possibly had other occupational exposures to asbestos. The case control analysis showed that the distribution of cases and controls with respect to exposure to crocidolite was quite unlikely assuming no association with crocidolite. This indicates that some, and possibly all, of the eight mesotheliomas with crocidolite exposure were related to that exposure. The data were not presented in a form that permitted a quantitative estimate of mesothelioma risk.
Regarding uncertainty factors, F1 was assigned a value of 2 because, although the manner in which unmeasured exposure was estimated in this study was different than for that reported for the majority of other studies (see, for example, Quebec), it was unlikely to introduce greater uncertainty. Rather than extrapolating measured estimates to earlier times based on expert judgments, judgments were used to simulate earlier conditions at the plant and exposures were measured directly. Because the measurements collected were analyzed by PCM, no conversion factor was required. Thus, F2 was assigned a value of 1 for this study. An uncertainty factor F4L = 1.5 was included to account for the fact that, due to the form of the data available, it was not possible to estimate α.
Ontario Asbestos-Cement Plant
Finkelstein (Citation1984) studied mortality among a group of 535 exposed and 205 unexposed employees of an Ontario asbestos-cement factory who had been hired before 1960 and who had been employed for at least 1 year. This cohort contains the cohort studied by Finkelstein (Citation1983), which required at least 9 years of employment for inclusion. Follow-up continued until 1977 or 1981.
The plant produced asbestos cement pipe from 1948 and asbestos cement board from 1955–1970. Manufacture of asbestos insulation materials was added in 1960. Both chrysotile and crocidolite were used in each batch processed in the pipe process, but only chrysotile was used in the cement board operation. Crocidolite constituted approximately 20% of the asbestos used in the pipe process (Dupré; Ontario Royal Commission Citation1984).
Fiber concentrations in various work areas and for various epochs were estimated from membrane filter samples taken after 1969, impinger measurements taken during 1949, 1954, 1956, 1957, and semiannually during the 1960s, and information on changes in dust control methods. Finkelstein judged that the resulting exposure estimates were “probably accurate to within a factor of 3 or 5.” Exposures of maintenance workers were not estimated and the exposure response analysis consequently involved only the unexposed workers (n= 205) and the production workers (n= 428).
Only 21 deaths from lung cancer were observed among production workers. Based on these deaths, Finkelstein compared age-standardized lung cancer mortality rates in production workers after a 20-year latency, categorized into 5 groups according to their cumulative exposure through 18 years from date of first employment (Finkelstein, Citation1984, Table 7). Mortality rates were standardized with respect to age and latency using the person-years distribution in the cohort as a whole as the standard. Using similarly standardized mortality rates in Ontario males as the comparison population, lung cancer rates were elevated in all 5 categories, and Finkelstein found a significant exposure-response trend. However, the trend was not monotonic, as rates increased up to the middle exposure category and decreased thereafter ().
TABLE B14 Lung cancer mortality by cumulative exposure among ontario asbestos cement plant workers Finkelstein (1984)
These data may be put into a form roughly equivalent to the more conventional age-adjusted comparison of observed and expected lung cancer deaths by dividing the rates in the exposed group by that of Ontario men. (The rate for unexposed workers was not used because it was based on only three deaths.) The results are shown in , which also shows the results of fitting the lung cancer model both assuming the Ontario rates were appropriate for this cohort (fixing the parameter α= 1) and not making this restriction (resulting in α= 4.26). Neither approach provides an adequate fit to these data (p⩽ .05) and the test of α= 1 is marginally significant (p= .07). The KL estimate with α= 1 is 0.050 (f-yr/ml)−1, 90% CI: (0.028, 0.074). With α allowed to vary the estimate is KL= 0.0029 (f-yr/ml)−1, 90% CI: (0, 0.0068). The fact that the lower limit is zero indicates that the exposure-response trend was not significant when the background was allowed to vary. Since in this case the estimated α is >2, the model was refit with α= 2, with the result: KL= 0.019, 90% CI: (0.012, 0.028).
Based on a “best evidence” classification of causes of death, Finkelstein identified 17 deaths from mesothelioma among production workers. of Finkelstein (Citation1984) gives these mesotheliomas categorized by years since first exposure. This table also provides the mortality rate, from which can be calculated the person-years of observation. Finkelstein states that the average cumulative exposure for production workers was about 60 f-yr/ml, but does not provide information for determining duration and level of exposure separately. CHAP (Citation1983) used an average exposure of 9 f/ml for a subcohort of production workers, although it provided no support for this assumption. If this value is assumed to be appropriate for the expanded cohort, the average duration is estimated as about 60/9 = 6.7 years. However these values are uncertain. presents the result of applying the mesothelioma model to the Finkelstein (Citation1984) data based on these assumptions. This model describes these data adequately (p= .26) and provides an estimate of KM= 18 × 10−8, 90% CI: (13 × 10−8, 24 × 10−8).
TABLE B15 Mesothelioma mortality among employees of an Ontario Asbestos cement factory Finkelstein (1984)
Regarding uncertainty, F1 was assigned a value of 4 because Finkelstein indicated that exposure estimates derived for this study were probably good to within a factor of 3 or 5. Finkelstein also noted that many of the assumptions employed to extrapolate exposures were only weakly supported by limited, earlier impinger measurements. A value of 3 was assigned to F2 because the source of the conversion factor employed to link impinger measurements and PCM measurements in this study is unclear. Because data for evaluating mesothelioma incidence was not provided in a format suitable for deriving confidence intervals (so that some reconstruction is required), a value of 2 was assigned for F4M.
Swedish Asbestos-Cement Plant
Albin et al. (Citation1990) studied workers at a Swedish plant that operated from 1907 to 1978 and produced various asbestos cement products, including sheets, shingles, and ventilation pipes. The asbestos handled was mainly chrysotile (>95%). Crocidolite was used before 1966, but never exceeded 3–4% of the total asbestos. Amosite was used for a few years in the 1950s but never exceeded 18% of the total asbestos used. The plant used commercial fiber grades 3–7 and all asbestos was milled prior to incorporation into products.
Impinger and gravimetric dust measurements were available for 1956–1969 and PCM measurements after 1969. These data, along with information on production and dust control, were used to estimate exposures for different jobs and periods of time.
The cohort, which contained 2,898 men, was defined as all male employees who worked for at least 3 months between 1907 and 1977. A reference cohort was composed of 1,233 men who worked in other industries in the region and who were not known to have worked with asbestos. Vital status of both groups was determined through 1986. Follow-up of both began after 20 years from first employment.
Excluding mesothelioma, other respiratory cancers were not significantly increased. Albin et al. presented relative risks of these respiratory cancers and corresponding 95% CI's for 3 categories of cumulative exposure (), based on Poisson regression with control for age and calendar year. To obtain crude estimates of the range of KL's that are consistent with these data, the lung cancer model was fit, assuming that the Ln(RR) are normally distributed with fixed variances computed from the reported confidence intervals for the RR. Although elevated, the RR does not exhibit an exposure response and the hypothesis α= 1 is not rejected (p= .13). In this analysis KL is not significantly different from zero, regardless of whether α is fixed at 1 or estimated. With α= 1 the estimate of KL is 0.019 (f-yr/ml)−1, 90% CI: (0, 0.065), and KL=0.00067 (f-yr/ml)−1, 90% CI: (0, 0.036) with α estimated (α= 1.82).
TABLE B16 Lung cancer mortality among Asbestos cement workers in Sweden Albin et al. (1990)
Thirteen mesotheliomas were identified among exposed workers and one in the referent population and a significant exposure response was observed with increasing cumulative exposure. Unfortunately, the mesothelioma data were not presented in a format that would permit application of the mesothelioma model [Eq. (4)].
Regarding uncertainty factors, F1 was assigned a value of 4 due to the sparseness of data and the need to extrapolate. Several assumptions were incorporated into the extrapolations that were based, among other things, on the scarcity of raw-material asbestos during World War II.
Belgian Asbestos-Cement Plant
Lacquet et al. (Citation1980) conducted a roentgenologic, asbestosis, and mortality study in a Belgian asbestos cement factory employing about 2,400 employees that annually processed about 39,000 tons of asbestos, of which 90% was chrysotile, 8% crocidolite, and 2% amosite. The mortality study considered male workers who worked in the factory for at least 12 months during the 15-year period 1963–1977. Apparently no minimal latency was required before follow-up began.
Fiber counts were available for the years 1970–1976. Fiber levels were estimated for as far back as 1928, but these estimates were considered to be “only good guesses at best.” Individual exposures were estimated in f/ml-yr from work histories and estimated yearly air concentrations in four work areas.
The incidence of respiratory cancer is very close to that which was expected in a Belgian population of matched age and sex (). The models with α= 1 (p= .51) and α variable (p= .39) gave similar results and the hypothesis α= 1 was not rejected (p= .77). With α= 1, the estimate of KL was 0 (f-yr/ml)−1, 90% CI: (0, 0.0010). With α estimated (α= 0.924), KL= 6.8 × 10−5 (f-yr/ml)−1, 90% CI: (0, 0.0021).
TABLE B17 Lung cancer mortality among belgian Asbestos-cement factory workers Laquet et al. (1980)
One death was due to pleural mesothelioma. Unfortunately, the data were not presented in a way that allowed the estimation of KM.
Regarding uncertainty, F1 was assigned a value of 4 due to the sparseness of data and the need to extrapolate. Much of the data appears to be based on PCM measurements, so conversion from other exposure units was not necessary.
Retirees From U.S. Asbestos Products Company
Enterline et al. (Citation1986) extended follow-up through 1980 for a cohort of U.S. retirees from a large asbestos products company that had been the subject of an earlier report (Henderson and Enterline, Citation1979). Products manufactured by the company include textiles, cement shingles, sheets, insulation, and cement pipe. Exposure was predominately to chrysotile in most operations, although amosite predominated in insulation production and crocidolite in manufacture of cement pipe. Each worker's exposure was estimated from dust measurements in mppcf obtained from environmental surveys that started in the mid-1950s and were extrapolated back in time by the company industrial hygienist. No data were provided for conversion from mppcf to PCM measurements. Given the wide range of products manufactured, this conversion likely varied according to operation. Conversions calculated in different environments have ranged from 1.4 to 10, the most common value being around 3 f/ml per mppcf, which has been observed in diverse environments such as mining and textile manufacture. This value was applied to this cohort.
The cohort consisted of 1,074 white males who retired from the company during 1941–1967 and who were exposed to asbestos in production or maintenance jobs. The average duration of employment was 25 years. Follow-up started at age 65 or at retirement if work continued past age 65. By the end of follow-up in 1980, 88% were deceased.
Overall, respiratory cancer was significantly increased (SMR = 258 in comparison to U.S. rates, based on 79 observed deaths). Enterline et al. (Citation1986) categorized lung cancer deaths by cumulative exposure ( in Enterline et al., Citation1986). Results of applying the lung cancer model to these data are shown in . Both the model with α= 1 and α variable fit the data adequately (p= .75) and, correspondingly, the test of α= 1 was not rejected (p= .24). With α= 1 the estimate of KL was 0.0021 (f−y/ml)−1, 90% CI: (0.0015, 0.0027). With α variable (α= 1.43), KL= 0.0011 (f-yr/ml)−1, 90% CI: (0.00041, 0.0028).
TABLE B18 Lung cancer mortality among retirees from a US Asbestos Company Enterline et al. (1986)
From the death certificates Enterline et al. identified eight deaths from mesothelioma. These data were not presented in a form that permitted application of the mesothelioma model [Eq. (4)].
Regarding uncertainty, F1 was assigned a value of 2 for this study for reasons similar to those described for Quebec. Because the manner employed for deriving the conversion factor used to convert impinger counts to fiber concentrations was not documented, a value of 3 was assigned to F2 for this study.
U.S. Insulation Applicators
Selikoff and Seidman (Citation1991) reported on follow-up through 1986 of a cohort of 17,800 asbestos insulation applicators that had been followed through 1976 by Selikoff et al. (Citation1979). The cohort consists of men enrolled as members of the insulator's union in the United States and Canada. Deaths were classified both based on the information on the death certificate, and using “best evidence,” in which death certificate information was augmented by clinical data, histopathological material, and x-rays.
Based on the composition of insulation material, it seems likely that these workers were exposed to substantial amounts of both chrysotile and amosite. Data on exposures of insulators were reviewed by Nicholson (Citation1976), who concluded that average exposures of insulation workers in past years could have ranged between 10 and 15 f/ml and could have been 15–20 f/ml in marine construction. The EPA 1986 update assumed a value of 15 f/ml as an overall average, with an associated threefold uncertainty. This estimate of 15 f/ml is used provisionally here as well.
The form of the data provided in Selikoff and Seidman (Citation1991) is not optimal for calculating KL. of Selikoff and Seidman (Citation1991) contains observed and expected deaths from lung cancer (determined from either death certificates or “best evidence”) categorized by years from first exposure (<15, 15–19, 20–24, …, 50+). Death certificate information was utilized herein to facilitate comparisons with expected deaths (based on the mortality experience of U.S. white males), which were also based on death certificates. Lung cancer was significantly increased over expected, except for the category of <15 years from onset of exposure. Selikoff and Seidman did not provide information on the duration of exposure. The EPA 1986 update (p. 90) assumed an average exposure duration of 25 years. By assuming that all workers worked an average of 25 years and were exposed to 15 f/ml, the data in of Selikoff and Seidman were used to categorize lung cancer deaths by cumulative exposure lagged 10 years. The result is shown in . The lung cancer model provided a reasonable fit to these data with α variable (p= .12), but not with α= 1 (p= .002). Also, the hypothesis that α= 1 was rejected (p< .001). The estimate of KL with α variable (α= 2.39) was 0.0018 (f-yr/ml)−1, 90% CI: (0.00065, 0.0038). With α= 1, KL= 0.0087 (f-yr/ml)−1, 90% CI: (0.0081, 0.0093). With α fixed at 2, KL = 0.0028 (f-yr/ml)−1, 90% CI: (0.0025, 0.0031).
TABLE B19 Lung cancer deaths among insulation workers in the United States and Canada Selikoff and Seidman (1991)
Based on “best evidence,” Selikoff and Seidman (Citation1991) found 458 mesotheliomas in this cohort. shows these deaths categorized by years from onset (based on Tables 5 and 6 in Selikoff and Seidman 1991). also shows the results of fitting the mesothelioma model to these data, assuming, as earlier, that workers worked for 25 years and were exposed to 15 f/ml. The mesothelioma model provided a poor fit to these data (p< .01), as it overestimated by more than a factor of 2 the number of mesothelioma deaths after 50+ years from first exposure. The estimate of KM was 1.3 × 10−8, 90% CI: (1.2 × 10−8, 1.4 × 10−8).
TABLE B20 Mesothelioma deaths among Asbestos Insulation workers Selikoff and Seidman (1991)
Regarding uncertainty factors, F1 was assigned a value of 4 because data employed to estimate exposure was not facility-specific, but represented general, industry-wide exposure estimates derived from limited data. F3 was assigned a value of 2 because the study provides no information on worker histories. F4L was assigned a value of 2 for this study because the data presented in the study are not provided in a form suitable for fitting the lung cancer model [Eq. (2)]. Thus, the data had to be partially reconstructed.
Pennsylvania Textile Plant
McDonald et al. (Citation1983b) report on mortality in an asbestos plant located near Lancaster, Pennsylvania that produced mainly textiles, but also some friction materials. About 3,000 to 6,000 tons of chrysotile were processed annually at the plant, which began operation in the early 1900s. Crocidolite and amosite were used from 1924 onward. About 3–5 tons of raw crocidolite were processed annually, and the use of amosite reached a peak of 600 tons during World War II.
The cohort consists of all men employed for at least 1 month prior to 1959 and who had a valid record with the Social Security Administration. This group consists of 4,022 men, of whom 35% had died by the end of follow-up (December 31, 1977). Follow-up of each worker was begun 20 years after first employment.
To estimate exposures, McDonald et al. had available reports of surveys conducted by the Metropolitan Life Insurance Company during the period 1930–1939, Public Health Service surveys conducted during 1967 and 1970, and company measurements made routinely from 1956 onward. These data were used to estimate exposures by department and year in units of mppcf.
The lung cancer mortality in this cohort exhibited a significant exposure response trend (), which was partially due to a deficit of cancers in the group exposed to <10 mppcf-yr (21 with 31.4 expected). A survey of those employed in the plant in 1978 revealed a larger percentage of nonsmokers (25%) than were found in the other plants studied by these researchers (McDonald et al., Citation1983a, Citation1984), although this finding was based on a sample of only 36 workers. Regardless of the reason for this shortfall in the number of lung cancers, it appears that the most appropriate analysis is that in which the background is allowed to vary; this analysis fit the data well (p> 0.7), whereas the analysis which assumed the Pennsylvania rates were appropriate (i.e., using α= 1) provided a marginal fit (p= .08). The hypothesis α= 1 was rejected (p= .01). Consequently, the analysis in which α is allows to vary was judged to be the most appropriate. McDonald et al. (Citation1983b) did not provide a factor for converting from mppcf to f/ml. Assuming that 3 f/ml was equivalent to 1 mppcf, the resulting estimate of lung cancer potency with α variable (α= 0.519) was 0.018 (f-yr/ml)−1, 90% CI: (0.0075, 0.045). With α= 1, KL= 0.0057 (f-yr/ml)−1, 90% CI: (0.0027, 0.0094).
TABLE B21 Lung Cancer Mortality among Workers in a Pennsylvania Textile Factory McDonald et al. (1983b)
A diagnosis of mesothelioma was specified on 14 death certificates (10 pleural and 4 peritoneal). Thirty other deaths 15 or more years after first employment were given the ICD code 199 (malignant neoplasms of other and unspecified sites) and the diagnosis given in many of these cases was said to be consistent with an unrecognized mesothelioma. However, 17 of these cases occurred before 1965, the year after which most of the confirmed mesotheliomas occurred. in McDonald et al. (Citation1983b) listed the average age at beginning of employment as 28.92 and the average duration of employment as 9.18 years. in McDonald et al. (Citation1983b) listed 191, 667, and 534 deaths as occurring before age 45, between 45 and 65, and after 65 years of age, respectively. Assuming that 50% of the additional 30 deaths given the ICD code 199 might have been due to mesotheliomas, the total number of mesotheliomas in this cohort was, for definiteness, provisionally estimated to be 29, although the uncertainty in this estimate is recognized. Proceeding as in the mesothelioma analysis carried out for the McDonald et al. (Citation1984) data, the data in were generated. Note that, due to the fact that KM occurs simply as a multiplier in the mesothelioma model [Eq. (4)], the maximum likelihood estimate of KM does not change when mesotheliomas are moved from one age since first exposure category to another (although the goodness of fit will change). The resulting estimate of KM was 1.38 × 10−8, 90% CI: (1.0 × 10−8, 1.84 × 10−8). These estimates are uncertain due to the uncertainty regarding the number of mesotheliomas in the cohort.
TABLE B22 Mesothelioma mortality among Pennsylvania textile plant workers McDonald et al. (1983b)
Regarding uncertainty, F1 was assigned a value of 2 for reasons similar to those described for Quebec. Because the manner employed for deriving the conversion factor used to convert impinger counts to fiber concentrations was not documented, a value of 3 was assigned to F2.
Rochdale, England Textile Factory
Peto et al. (Citation1985) studied a textile factory in Rochdale, England, that has been the subject of a number of earlier reports (Peto et al., Citation1977; Peto, Citation1980a, Citation1980b). Peto et al. (Citation1985) was based on the most complete follow-up (through 1983) and emphasized assessment of risk. The factory, which began working with asbestos in 1879, used principally chrysotile, but approximately 5% crocidolite was used between 1932 and 1968.
Quantitative estimates of risk were based on a subgroup of the Peto et al. (Citation1985) “principal cohort,” which consists of all men first employed in 1933 or later who had worked in scheduled areas or on maintenance and had completed 5 years of service by the end of 1974. In the analyses of interest relating to lung cancer, follow-up only began 20 years after the beginning of employment and exposure during the last 5 years of follow-up was not counted.
Routine sampling using a thermal precipitator began at 23 fixed sampling points in 1951. Comparisons of particle counts and fiber counts taken in 1960 and 1961 were used to convert between particles/ml and f/ml. Dust levels prior to 1951 were assumed to be the same as those observed during 1951–1955 for departments for which no major changes had been made. In departments in which conditions had improved, higher levels were assigned. These levels and work histories were used to assign individual exposure estimates. A conversion factor of 34 particles/ml per f/ml was determined by comparing average results obtained by the Casella thermal precipitator (particles/ml) with Otway long running thermal precipitator (f/ml) at the same sampling point during 1960 and 1961. However, a conversion factor of 35.3 was used by Peto et al. (Citation1985) for the sake of consistency with earlier work and this factor was used here as well.
After 20 years from first employment, there were 93 lung cancer deaths with only 64.6 expected. Using a lung cancer model essentially the same as in the EPA 1986 update [Eq. (2)], Peto et al. estimated KL= 0.0054 (f-yr/ml)−1 for the entire cohort, and KL= 0.015 (f-yr/ml)−1 when the analysis was restricted to men first employed in 1951 or later. Peto et al. felt that the most plausible explanation for this difference was that it was largely due to chance and also to the possibility that exposure to the most carcinogenic fibers was not reduced as much as changes in particle counts from 1951 to 1960 would suggest.
displays the exposure response data based on men first employed in 1933 or later for lung cancer. This table shows that the excess occurs mainly in workers whose cumulative exposure exceeds 400 f-yr/ml (10 observed, 1.7 expected). The lung cancer model fit these data adequately (p= .63) both with α= 1 and α variable (α=1.10) and the hypothesis α= 1 was not rejected (p= .57). With α= 1, KL was estimated as 0.0052 (f-yr/ml)−1, 90% CI: (0.0028, 0.0079), and with α variable, KL= 0.0041 (f-yr/ml)−1, 90% CI: (0.0012, 0.0087).
TABLE B23 Lung Cancer Mortality among Rochdale Asbestos Textile Factory Peto et al. (1985)
Ten mesotheliomas were observed in the cohort used by Peto et al. for quantitative analysis. (An 11th case that was exposed for 4 months and died 4 years later was omitted because the short latency made it unlikely that this case was related to exposure at the factory.) Observed mesotheliomas and corresponding person-years of observation by duration of service and years since first employment (Table 8 in Peto et al., Citation1985) are shown in . An overall average exposure was estimated by applying the Peto mesothelioma model to the data in with a common exposure estimate and selecting the value that gave the smallest least-squares fit of this model to the mesothelioma data. The fitting was carried out both unweighted and by weighting by person-years, with resulting estimates of 360 and 322 particles/ml, respectively; the latter value was the one selected. Using the conversion factor of 35.3 particles/ml per f/ml, the estimated average exposure was 322/35.2 = 9.1 f/ml. The mesothelioma model [Eq. (4)] fit these data well and the resulting estimate of mesothelioma potency () was KM= 1.3 × 10−8, 90% CI: (0.74 × 10−8, 2.1 × 10−8).
TABLE B24 Mesothelioma mortality among rochdale asbestos textile factory Peto et al. (1985)
Regarding uncertainty factors, F1 was assigned a value of 2 for this study for reasons similar to those described for Quebec. Because a conversion factor was derived for measurements collected using Otway long-running thermal precipitators and PCM measurements based on measurements of each collected under similar conditions (but not side by side), a value of 2 was assigned to F2.
ACKNOWLEDGMENTS
We thank Corbett McDonald and acknowledge the help of Douglass Liddell (deceased) for making available the raw data on mesothelioma mortality for the cohort of Quebec chrysotile miners and millers. We thank Nicholas de Klerk for making available the raw data for the cohort of Wittenoom, Australia, crocidolite miners. We thank NIOSH, and Everett (Chip) Lehman in particular, for making available the raw data for the cohort of workers from the textile plant in Charleston, SC. We thank Misty Hein for her valuable and very timely assistance in providing data on background cancer rates and additional information on the South Carolina cohort. We gratefully acknowledge the guidance and support provided by Aparna Koppikar, Richard Troast, Chris Weis, and Paul Peronard. We are indebted to John Addison, John Dement, Agnes Kane, Bruce Case, Michel Camus, and Vanessa Vu for their input and assistance. We thank Phillip Benge for his technical assistance and careful reading of the final manuscript. Finally, we gratefully acknowledge the financial support for the earlier work provided by the U.S. EPA and the grant provided by the National Stone Sand and Gravel Association to prepare this manuscript. This project was supported in part by an appointment to the Research Participation Program at the National Center for Environmental Assessment, U.S. EPA, administered by the Oak Ridge Institute for Science and Education through an interagency agreement between the U.S. Department of Energy and the EPA. The financial support provided by any group should not be construed as endorsement of the results of this work.
Notes
1. “Supralinear” refers to an exposure response whose slope decreases as exposure increases (e.g., [exposure]K with K< 1). A linear model fit to data that exhibit supralinearity will generally underestimate the response at low exposures and overestimate at high exposures.
2. Although the term “fiber type” typically includes consideration of both mineralogy and crystalline habit, we have focused primarily on mineralogy here due to an almost complete lack of data informative of crystalline habit among published epidemiological studies.
3. As used here, the term, “fiber” is intended to include not only single fibers (fibrils), but the bundles, clusters, and matrices that make up the set of fibrous particles in an asbestos dust (ISO, 1995).
REFERENCES
- Albin M., Jakobsson K., Attewell R., Johansson L., Welinder H. Mortality and cancer morbidity in cohorts of asbestos cement workers and referents. Br. J. Ind. Med. 1990; 79(9)602–610
- Amandus H. E., Wheeler R. The morbidity and mortality of vermiculite miners and millers exposed to tremolite–actinolite: Part II. Mortality. Am. J. Ind. Med. 1987; 11: 15–26
- Amandus H. E., Wheeler R., Jankovic J., Tucker J. The morbidity and mortality of vermiculite miners and millers exposed to tremolite–actinolite: Part I. Exposure estimates. Am. J. Ind. Med. 1987; 11: 1–14
- Armstrong B. K., de Klerk N.H., Musk A. W., Hobbs M. S.T. Mortality in miners and millers of crocidolite in Western Australia. Br. J. Ind. Med. 1988; 45: 5–13
- Berman D. W., Chatfield E. J. Interim Superfund method for the determination of asbestos in ambient air. Part 2: Technical background document. U.S. Environmental Protection Agency, Washington, DC 1990, Office of Solid Waste Remedial Response; EPA/540/2–90/005b. May.
- Berman D. W., Crump K. S. A meta-analysis of asbestos–related cancer risk that address fiber size and mineral type. Crit. Rev. Toxicol. 2008; 38(S1)49–73
- Berman D. W., Crump K. S. Final draft: technical support document for a protocol to assess asbestos–related risk. U.S. Environmental Protection Agency, Washington, DC 2003, EPA Report 9345.4–06. Office of Solid Waste Emergency Response
- Berman D. W., Crump K. S., Chatfield E. J., Davis J. M., Jones A. D. The sizes, shapes, and mineralogy of asbestos structures that induce lung tumors or mesothelioma in AF/HAN rats following inhalation. Risk Anal. 1995; 15(2)181–195, [Erratum in: Risk Anal. 1995;15(4):541.]
- Berry G., de Klerk N.H., Reid A., Ambrosini G. L., Fritichi L., Olsen N. J., Merler E, Musk A. W. Malignant pleural and peritoneal mesotheliomas in former miners and millers of crocidolite at Wittenoom, Western Australia. Occup. Environ. Med. 2004; 61: 1–3
- Berry G., Liddell F. D.K. The interaction of asbestos and smoking in lung cancer: A modified measure of effect. Ann. Occup. Hyg. 2004; 48(5)459–462
- Berry G., Newhouse M. L. Mortality of workers manufacturing friction materials using asbestos. Br. J. Ind. Med. 1983; 40: 1–7
- Carroll R. J., Ruppert D., Stefanski L. A., Crainiceanu C. M. Measurement error in nonlinear models. A Modern Perspective, 2nd. Chapman & Hall/CRC, Boca Raton, FL 2006
- CHAP (Chronic Hazard Advisory Panel on Asbestos). 1983, Report to the U.S. Consumer Product Safety Commission. July.
- Cox D. R., Hinkley D. V. Theoretical Statistics. Chapman and Hall, London 1974
- Crump K. S. The effect of random error in exposure measurement upon the shape of the exposure response. Dose Response 2005; 3(4)456–464
- Crump K. S. Benchmark analysis. Encyclopedia of Environmetrics. John Wiley & Sons, West SussexUK 2002; 163–170
- Davis J. M., Jones A. D. Comparisons of the pathogenicity of long and short fibres of chrysotile asbestos in rats. Br. J. Exp. Pathol 1988; 69(5)717–737
- de Klerk N.H., Armstrong B. K., Musk A. W., Hobbs M. S.T. Cancer mortality in relation to measures of occupational exposure to Crocidolite at Wittenoom Gorge in Western Australia. Br. J. Ind. Med. 1989; 46: 529–536
- de Klerk N.H., Musk A. W., Armstrong B. K., Hobbs M. S.T. Diseases in miners and millers of crocidolite from Wittenoom, Western Australia: A further follow–up to December 1986. Ann. Occup. Hyg. 1994; 38(Suppl 1)647–655
- Dement J. M., Brown D. P. Cohort mortality and case–control studies of white male chrysotile asbestos textile workers. J. Clean Technol. Environ. Toxicol. Occup. Med. 1998; 7: 1052–1062
- Dement J. M., Harris R. L., Symons M. J., Shy C. M. Estimates of dose-response for respiratory cancer among chrysotile asbestos textile workers. Ann. Occup. Hyg. 1982; 26(1–4)869–887
- Dement J. M., Harris R. L., Symons M. J., Shy C.M. Exposures and mortality among chrysotile workers. Part I: Exposure estimates. Am. J. Ind. Med. 1983a; 4: 399–419
- Dement J. M., Harris R. L., Symons M. J., Shy C. M. Exposures and mortality among chrysotile workers. Part II: Mortality. Am. J. Ind. Med. 1983b; 4: 421–433
- Dement J. M., Brown D. P., Okun A. Follow–up study of chrysotile asbestos textile workers: Cohort mortality and case-control analysis. Am. J. Ind. Med. 1994; 26: 431–447
- Dement J. M., Kuempel E., Zumwalde R., Smith R., Stayner L. T. Development of a fiber size-specific job-exposure matrix for airborne asbestos fibers. Occup. Environ. Med. Online 2007, doi:10.1136/oem.2007.033712.
- Dupré J., Mustard J. F., Uffen R. J., Ontario Royal Commission. Report of the Royal Commission on Matters of Health and Safety Arising from the Use of Asbestos in Ontario. 1984, Volume 1–3. Toronto: Ministry of the Attorney General, Province of Ontario; 1984.
- Enterline P. E., Harley J., Henderson V. Asbestos and cancer—A cohort followed to death. Graduate School of Public Health, University of Pittsburgh. 1986
- Finkelstein M. M. Mortality among employees of an Ontario asbestos–cement factory. Am. Rev. Respir. Dis 1984; 129: 754–761
- Finkelstein M. M. Mortality among long-term employees of an Ontario asbestos-cement factory. Br. J. Ind. Med. 1983; 40: 138–144
- Hammad Y. Y., Diem J., Weill H. Evaluation of dust exposure in asbestos cement manufacturing operations. Am. Ind. Hyg. Assoc. J 1979; 40(6)490–495
- Hammond E. C., Selikoff I. J., Seidman H. Asbestos exposure, cigarette smoking and death rates. Ann. NY Acad. Sci. 1979; 330: 473–490
- Health Effects Institute–Asbestos Research (HEI–AR). Asbestos in Public and Commercial Buildings: A Literature Review and Synthesis of Current Knowledge. HEI–AR, Cambridge, MA 1991
- Hein M. J., Stayner L. T., Leyman E., Dement J. M. Follow-up study of chrysotile textile workers: Cohort mortality and exposure-response. Occup. Environ. Med. 2007; 64: 616–625
- Henderson V. L., Enterline P. E. Asbestos exposure: Factors associated with excess cancer and respiratory disease mortality. Ann. NY Acad. Sci. 1979; 330: 117–126
- Hughes J. M., Weill H. Asbestos exposure: Quantitative assessment of risk. Am. Rev. Respir. Dis. 1986; 133: 5–13
- Hughes J. M., Weill H., Hammad Y. Y. Mortality of workers employed at two asbestos cement plants. Br. J. Ind. Med. 1987; 44: 161–174
- International Agency for Research on Cancer (IARC). Monographs on the Evaluation of Carcinogenic Risks to Man. IARC Scientific Publications, LyonFrance 1977; 14
- Klein C. Rocks, minerals and a dusty world. Health Effects of Mineral Dusts, G.D. Guthrie, B.T. Mossman. Reviews in Mineralogy and Geochemistry. 1993; 7–59, 1993; vol. 28
- Lacquet L. M., VanderLinden L., Lepoutre J. Roentgenographic lung changes, asbestosis and mortality in a Belgian asbestos-cement factory. Biological Effects of Mineral Fibres, J. C. Wagner. IARC Scientific Publications, Lyon 1980; 783–793
- Lash T. L., Crouch E. A.C., Green L. C. A meta-analysis of the relation between cumulative exposure to asbestos and relative risk of lung cancer. Occup. Environ. Med. 1997; 54: 254–263
- Lemon R. A., Dement J. M., Wagoner J. K. Epidemiology of asbestos-related diseases. Environ. Health Perspect. 1980; 34: 1–11
- Levin J. L., McLarty J.W., Hurst G. A., Smith A. N., Frank A. L. Tyler asbestos workers: Mortality experience in a cohort exposed to amosite. Occup. Environ. Med. 1998; 55: 155–160
- Liddell F. D.K. The interaction of asbestos and smoking in lung cancer. Ann. Occup. Hyg. 2004; 45(5)341–356
- Liddell F. D.K. 2001, Unpublished raw mesothelioma data provided to Dr. Wayne Berman by Dr. F. D. K. Liddell from multiple studies of the 1891–1920 Birth Cohort of Quebec Chrysotile Miners and Millers most recently described in Liddell, 1997.
- Liddell F. D.K., Armstrong B. G. The combination of effects on lung cancer of cigarette smoking and exposure in Quebec chrysotile miners and millers. Ann. Occup. Hyg. 2002; 46(1)5–13
- Liddell F. D.K., McDonald A.D., McDonald J.C. The 1891–1920 birth cohort of Quebec chrysotile miners and millers: Development from 1904 and mortality to 1992. Ann. Occup. Hyg. 1997; 41: 13–36
- McDonald A. D., Fry J. S., Woolley A. J., McDonald J.C. Dust exposure and mortality in an American chrysotile asbestos friction products plant. Br. J. Ind. Med. 1984; 41: 151–157
- McDonald A. D., Fry J. S., Wooley A. J., McDonald J.C. Dust exposure and mortality in an American chrysotile textile plant. Br. J. Ind. Med. 1983a; 39: 361–367
- McDonald A. D., Fry J. S., Woolley A. J., McDonald J.C. Dust exposure and mortality in an American factory using chrysotile, amosite, and crocidolite in mainly textile manufacture. Br. J. Ind. Med. 1983b; 40: 368–374
- McDonald J. C., Gibbs G. W., Liddell F. D.K. Chrysotile fibre concentration and lung cancer mortality: A preliminary report. Biological Effects of Mineral Fibres, J. C. Wagner. IARC Scientific Publications, Lyon 1980b; 811–817
- McDonald J. C., Harris J., Armstrong B. Mortality in a cohort of vermiculite miners exposed to fibrous amphibole in Libby, Montana. Occup. Environ. Med. 2004; 61(4)363–366
- McDonald J. C., Harris J. M., Berry G. Sixty years on: The price of assembling military gas masks in 1940. Occup. Environ. Med. 2006; 63: 852–855
- McDonald J. C., Liddell F. D.K., Dufresne A., McDonald A.D. The 1891–1920 birth cohort of Quebec chrysotile miners and millers: Mortality 1976–1988. Br. J. Ind. Med. 1993; 50: 1073–1081
- McDonald J. C., Liddell F. D.K., Gibbs G. W., Eyssen G. E., McDonald A.D. Dust exposure and mortality in chrysotile mining, 1910–1975. Br. J. Ind. Med. 1980a; 37: 11–24
- McDonald J. C., McDonald A.D., Armstrong B., Sebastien P. Cohort study of mortality of vermiculite miners exposed to tremolite. Br. J. Ind. Med. 1986; 43: 436–444
- Meeker G. P., Bern A. M., Brownfield I. K., Lowers H. A., Sutley S. J., Hoefen T. M., Vance J. S. The composition and morphology of amphiboles from the Rainy Creek Complex, near Libby, Montana. Am. Mineral. 2003; 88: 1955–1969
- Musk A. W., de Klerk N.H., Olsen N. J., Hansen J., Ambrosini G. L., Fritschi L., Merler E., Hobbs M. S.T. Mortality in miners and millers of crocidolite in Western Australia: Follow-up to 1999. Ann. Occup. Hyg. 2002; 46(suppl. 1)90–92
- National Institute for Occupational Safety and Health (NIOSH). Method for Determination of Asbestos in Air Using Positive Phase Contrast Microscopy. NIOSH Method 7400. NIOSH, Cincinnati, OH 1994a, 1985. Current revision.
- National Institute for Occupational Safety and Health (NIOSH). Method for Determination of Asbestos in Air Using Transmission Electron Microscopy. NIOSH Method 7402. NIOSH, Cincinnati, OH 1994b, 1986. Current revision.
- New York Academy of Sciences (NYAS). The Third Wave of Asbestos Disease: Exposure to Asbestos in Place—Public Health Control, P. J. Landrigan, H. Kazemi. New York Academy of Sciences, New York 1991; 643
- Nicholson W. J. Airborne asbestos health assessment update. U.S. Environmental Protection Agency, Washington, DC 1986, Report 600/884003F
- Nicholson W. J. Part III. Recent approaches to the control of carcinogenic exposures. Case study 1: Asbestos—The TLV approach. Ann. NY Acad. Sci. 1976; 271: 152–169
- Peto J. Lung cancer mortality in relation to measured dust levels in an asbestos textile factory. Biological Effects of Mineral Fibres, J. C. Wagner. IARC Scientific Publications, Lyon 1980a; 829–836
- Peto J. The incidence of pleural mesothelioma in chrysotile asbestos textile workers. Biological Effects of Mineral Fibres, J. C. Wagner. IARC Scientific Publications, Lyon 1980b; 703–711
- Peto J., Doll R., Hermon C., Binns W., Clayton R., Goffe T. Relationship of mortality to measures of environmental asbestos pollution in an asbestos textile factory. Ann. Occup. Hyg 1985; 29(3)305–355
- Peto J., Doll R., Howard S. V., Kinlen L. J., Lewinsohn H. C. A mortality study among workers in an English asbestos factory. Br. J. Ind. Med. 1977; 34: 169–173
- Piolatto G., Negri E., LaVecchia C., Pira E., Decarli A., Peto J. An update of cancer mortality among chrysotile asbestos miners in Balangero, Northern Italy. Br. J. Ind. Med. 1990; 47: 810–814
- Rubino G. F., Piolatto G. W., Newhouse M. L., Scansetti G., Aresini G. A., Murray R. Mortality of chrysotile asbestos workers at the Balangero mine, Northern Italy. Br. J. Ind. Med. 1979; 36: 187–194
- Schreier H. Asbestos in the Natural Environment. Elsevier, New York 1989; 159
- Seidman H., Selikoff I. J., Gelb S. K. Mortality experience of amosite asbestos factory workers: Dose-response relationships 5 to 40 years after onset of short-term work exposure. Am. J. Ind. Med. 1986; 10(5/6)479–514
- Seidman H., Selikoff I. J., Hammond E. C. Short-term asbestos work exposure and long-term observation. Ann. NY Acad. Sci. 1979; 330: 61–89
- Selikoff I. J., Hammond E. C., Seidman H. Mortality experience of insulation workers in the United States and Canada 1943–1976. Ann. NY Acad. Sci. 1979; 330: 91–116
- Selikoff I. J., Seidman H. Asbestos-associated deaths among insulation workers in the United States and Canada, 1967–1987. Ann. NY Acad. Sci 1991; 643: 1–14
- Stanton M. F., Layard M., Tegeris E., Miller E., May M., Morgan E., Smith A. Relation of particle dimension to carcinogenicity in amphibole asbestoses and other fibrous minerals. JNCI 1981; 67: 965
- Stayner L. T., Kuempel E., Gilbert S., Hein M., Dement J. An epidemiologic study of the role of chrysotile asbestos fiber dimensions in determining respiratory disease risk in exposed workers. Occup. Environ. Med 2007, doi:10.1136/oem.2007.035584
- Stayner L., Smith R., Bailer J., Gilbert S., Steenland K., Dement J., Brown D., Lemen R. Exposure-response analysis of risk of respiratory disease associated with occupational exposure to chrysotile asbestos. Occup. Environ. Med. 1997; 54: 646–652
- Sullivan P. Vermiculite, respiratory disease, and asbestos exposure in Libby, Montana: Update of a cohort mortality study. Environ. Health Perspect. 2007; 115(4)579–585
- Teta M. J., Lewinsoln H. C., Meigs J. W., Vidone R. A., Mowad L. Z., Flannery J. T. Mesothelioma in Connecticut: The authors reply. J. Occup. Med. 1986; 28: 808–809
- Teta M. J., Lewinsoln H. C., Meigs J. W., Vidone R. A., Mowad L. Z., Flannery J. T. Mesothelioma in Connecticut, 1955–1977: Occupational and geographic associations. J. Occup. Med. 1983; 25: 749–755
- Veblen D. R., Wylie A. G. Mineralogy of amphiboles and 1:1 layer silicates. Health Effects of Mineral Dusts, G. D. Guthrie, B.T. Mossman. Reviews in Mineralogy and Geochemistry 1993. 1993; 28: 61–137
- Venzon D., Moolgavkar S. A method for computing profile–likelihood–based confidence intervals. Appl. Stat 1988; 37: 87–94
- Walker A. M. Declining relative risks for lung cancer after cessation of asbestos exposure. J. Occup. Med 1984; 26(2)422–426
- Walton W. H. The nature, hazards, and assessment of occupational exposure to airborne asbestos dust: A review. Ann. Occup. Hyg. 1982; 25: 117–247
- Weill H., Hughes J., Waggenspack C. Influence of dose and fibre type on respiratory malignancy risk in asbestos cement manufacturing. Am. Rev. Respir. Dis. 1979; 120: 345–354