Abstract
To accurately characterize human health hazards, human, animal, and mechanistic data must be integrated and the relevance to the research question of all three lines of evidence must be considered. Mechanistic data are often critical to the full integration of animal and human data and to characterizing relevance and uncertainty. This novel evidence integration framework (EIF) provides a method for synthesizing data from comprehensive, systematic, quality-based assessments of the epidemiological and toxicological literature, including in vivo and in vitro mechanistic studies. It organizes data according to both the observed human health effects and the mechanism of action of the chemical, providing a method to support evidence synthesis. The disease-based component uses the evidence of human health outcomes studied in the best quality epidemiological literature to organize the toxicological data according to authors’ stated purpose, with the pathophysiology of the disease determining the potential relevance of the toxicological data. The mechanism-based component organizes the data based on the proposed mechanisms of effect and data supporting events leading to each endpoint, with the epidemiological data potentially providing corroborating information. The EIF includes a method to cross-classify and describe the concordance of the data, and to characterize its uncertainty. At times, the two methods of organizing the data may lead to different conclusions. This facilitates identification of knowledge gaps and shows the impact of uncertainties on the strength of causal inference.
Introduction
Methods for carrying out literature reviews have been evolving, and the purposes for carrying out systematic reviews have expanded to support a variety of regulatory and scientific objectives. One application of literature review methods in toxicology is for hazard identification, the first step in the risk assessment process for chemicals. Risk assessment is a process which also includes dose–response assessment, exposure assessment, and risk characterization. Traditionally, hazards were identified based exclusively on the existence of studies that provide evidence of an effect following exposure to a chemical. Hazards identified through such “strength of evidence” assessments omit information from studies that do not suggest an effect of the exposure (United States Environmental Protection Agency Citation1986; National Toxicology Program Citation2019a).
Driven by advances in regulatory science, at least in part, chemical risk assessments have evolved toward a more comprehensive, weight of evidence approach. Weight of evidence assessments consider all available data – whether or not the exposure appears to exert an effect – and they incorporate evaluations of study quality, risk of bias, uncertainty, and the precision of estimates, as well as the consistency of findings within a body of literature (EFSA Citation2017a, Citation2017b; OECD Citation2019; National Toxicology Program Citation2019b; LaKind et al. Citation2020; Burns and LaKind Citation2021). The Organization for Economic Co-operation and Development (OECD) describes weight of evidence assessments as considering each of the assembled lines of evidence to come to an overall decision or conclusion (OECD Citation2019). The more comprehensive weight of evidence approaches expand risk assessments to include causal inferences necessary for some types of regulatory decisions.
The Grading of Recommendations Assessment, Development and Evaluation (GRADE) guidelines represent one of the first attempts to establish a system for applying weight of evidence concepts within one stream of evidence, specifically human data from clinical trials (Guyatt et al. Citation2011). More recently, GRADE-like approaches have been applied to integrate data between streams of evidence (Vincent et al. Citation2017; Applegate et al. Citation2019), including information from in vitro cell cultures and in vivo animal studies. The focus on evidence integration has been adopted both for risk assessment and for science-based decision-making, and it has been codified in recommendations from the National Academy of Sciences (National Research Center [NRC] Citation2014). These methods have been adopted, and, in some cases, refined by agencies including the US Office of Health Assessment and Translation (OHAT) (National Toxicology Program Citation2019b), the European Commission (SCHEER) (Citation2018), the EFSA (Citation2017a, Citation2017b), the Organization for Economic Co-operation and Development (OECD Citation2018, Citation2019), and the USEPA (Citation2011, Citation2016, Citation2018), and by scientists attempting to advance risk assessment methods (Vandenberg et al. Citation2016; Suter et al. Citation2020; Krewski et al. Citation2022).
Most of the available regulatory and other guidance documents that focus on weight of evidence assessments include these general steps: (1) problem formulation, (2) systematic literature review to identify and select sources of evidence relevant to the problem formulation, (3) assessment of study quality, relevance, and risk of bias within lines of evidence (i.e. human, animal and mechanistic), (4) integration of all lines of evidence, (5) assessment of uncertainty, and (6) overall conclusion based on all of the integrated data (USEPA Citation2011, Citation2016, Citation2018; Vandenberg et al. Citation2016; EFSA Citation2017b; SCHEER Citation2018; OECD Citation2019). EFSA (Citation2017a) focuses specifically on issues and criteria related to food that should be considered when determining biological relevance (i.e. whether an observation is an adverse or possible beneficial human health effect) and offers a general framework for establishing biological relevance.
OECD (Citation2018) and others focus on the organization of the data related to mechanistic events leading to an adverse outcome and provide guidance for establishing adverse outcome pathways (AOP). AOPs are sequences of events that begin with initial interaction(s) of a stressor with a biomolecule within an organism progressing through a dependent series of intermediate key events (KE) to culminate in an adverse outcome (AO) considered relevant to risk assessment or regulatory decision-making (OECD Citation2018; Villeneuve et al. Citation2014a, Citation2014b). AOP analyses are distinct from the types of evidence integration described in this article, because they can include information from multiple chemicals to support a pathway to outcome, as they are not considered chemical-specific. In contrast, the methods described here focus on the evidence for potential effects of exposure to a single chemical.
What available guidelines and frameworks for evaluating the weight of evidence for a single chemical lack is a clear statement of best practices for organizing the available body of literature to facilitate its integration, and specific guidance for cross-classifying the evidence from different evidence streams to reach overall conclusions. Such guidelines are needed because of the complexity inherent in integrating information from an inclusive body of evidence. This complexity was highlighted in a 2022 National Academies of Science, Engineering and Medicine (NASEM) workshop titled “Triangulation in Environmental Epidemiology for USEPA Human Health Assessments.” During the workshop, panelists discussed the current state of the art with respect to identifying and incorporating data within the disciplines of epidemiology and toxicology for use in risk assessments and regulatory decision-making, and they also identified the need for a framework for integrating information across disciplines.
This article addresses the specific need for an evidence integration framework (EIF). It includes both recommendations for assessing study quality and for integrating evidence from human studies, animal toxicity studies, and in vivo or in vitro mechanistic studies. While other frameworks discuss the importance of considering disease etiology and chemical modes of action to assess the relevance of toxicological data to human disease (Schwarzman et al. Citation2015; Villeneuve et al. Citation2018; Smith et al. Citation2020; Whaley et al. Citation2020; Wikoff et al. Citation2020), the EIF presented here provides two complementary methods of integrating information about disease etiology and chemical mechanistic data and uses that information to integrate epidemiological with toxicological evidence from two perspectives: (1) based on observations in the relevant human studies (disease-based component) and (2) based on the proposed mechanisms by which exposure to the chemical of interest might affect development of the outcome or disease of interest (mechanism-based component). The EIF also provides guidance on how to complete the critical step of synthesizing information between and across evidence streams and provides an approach to summarizing the quantity and quality of available evidence. Finally, the EIF supports identification of data gaps that may be critical in drawing conclusions. Simple and easily described adjustments to the EIF allow it to be used for purposes ranging from hazard identification to causal inference.
The EIF method: overview
The EIF process consists of the following steps, presented in a flow diagram () and described in more detail in the sections below:
Problem formulation and protocol development
Systematic and comprehensive identification and review of the relevant human, animal, and mechanistic literature (i.e. three evidence streams)
Bridging between evidence streams based on disease etiology and pathophysiology and based on mode of action of the chemical of interest
Critical evaluation and synthesis of the evidence within streams
Integration of the evidence between streams, using separate, disease- and mechanism-based organizational schemes, accounting for:
○ Quality
○ Relevance
○ Certainty or uncertainty
○ Gap analysis
Formulate the problem and develop a protocol
The problem formulation step first identifies the overall goal of applying the EIF. The problem formulation step must identify the chemical of interest; stipulate whether acute, repeated, or all exposures are of interest; and specify the health outcome or outcomes that will be evaluated. Depending on the goal, the focus of the EIF can be adjusted from very nonrestrictive (to identify signals of potential hazards) to very restrictive (to identify possible causal associations), and the goal should be clearly stated in the problem formulation.
Once the problem has been formulated, developing a specific protocol for addressing the problem will support more efficient and effective literature searches and a more consistent application of inclusion, exclusion, and quality assessment criteria. The protocol should also include (1) guidelines for documentation; (2) templates for abstracting and summarizing relevant data; (3) methods of rating the quality of individual studies; (4) methods for synthesizing evidence within disciplines; and (5) methods of integrating evidence between disciplines. Problem formulation and protocol development are expected to be familiar to readers and are not discussed further.
Systematic and comprehensive identification of relevant literature
Searches should be designed to systematically and comprehensively identify literature that contains all relevant data and should be conducted in a manner consistent with the guidelines discussed above (EFSA Citation2017a, Citation2017b; OECD Citation2019; National Toxicology Program Citation2019b). All processes and results must be tracked for clear reporting; we suggest using the Preferred Reporting Items for Systematic Reviews and Meta-Analyses (PRISMA) guidance (Mohler et al. Citation2009).
Searches of the human (epidemiological) literature should be designed to capture studies of exposures to the chemical or chemicals of interest, along with any outcomes of a priori interest and any relevant clinical indicators of effect. Including search terms for nonspecific outcomes such as symptoms or clinical indicators of multiple disease states will increase the sensitivity of the search and possibly decrease the specificity of the conclusions but may be warranted if the goal of the project is general hazard identification. Searches may also include surrogate exposures (e.g. to a mixture containing the chemical of interest), although this also tends to introduce uncertainty, potentially reducing the weight of the available human evidence and limiting its relevance specifically to the chemical of interest.
In contrast to the preference for more specific searches of the human literature, searches of the toxicological literature should be designed to identify studies investigating general toxicological measurements, as well as studies of the relationship between the specified exposure (e.g. acute or repeated exposure to a specific chemical) and animal endpoints analogous or relevant to the development of the outcome of interest in humans. This is to identify data pertaining to all events relevant to cellular, systemic and target organ potentially related to an adverse effect of exposure. Including literature related to endpoints or assay results that elucidate the mode or mechanism of action of the chemical to assist in the integration of human and animal data is one major advance in this EIF over other previously introduced evidence integration methods.
Bridge between disease etiology/pathophysiology and chemical mode of action/mechanism of effect
After cataloging and selecting the human endpoints of interest from the epidemiological literature, reviewing medical textbooks and scholarly articles helps to identify relevant clinical markers or precursor conditions for the endpoint or disease of interest. Understanding these markers or precursor conditions provides information to determine those events that have been identified, through research, to be associated with the initiation and progression of the outcome of interest. This information is also important to differentiate studies that have been conducted to test hypotheses about the chemical of interest, rather than conducted to measure events that are known to be associated with the outcome. Unlike an AOP, this step bridges between disease etiology and mode of action data for the chemical of interest; it is not another weight of evidence integration step, but rather an explicit identification of what is known or widely accepted regarding the pathophysiology of the disease or outcome. If an AOP is available for the outcome of interest, this should be considered. This bridging activity will assist in evaluating the available toxicological mechanistic literature and determining whether there is certainty or uncertainty related to the connection between the event being evaluated and the development of the outcome or disease of interest.
Understanding the pathophysiology of the outcome or disease of interest supports the integration of epidemiological observations and toxicological measures by helping to identify events in the development of the health outcome of interest that could be affected by exposure to the chemical of interest. In addition, difficulties identifying relevant information for mechanisms of effect and modes of action for the chemical, or for the general pathophysiology of the endpoint of interest, will indicate gaps in current knowledge and inform decisions about the certainty or uncertainty of the ultimate conclusions regarding the relationships between the chemical exposure and the health outcomes evaluated. It is helpful to represent this material visually and to include it in the protocol. In this paper, we selected a cancer outcome for illustrative purposes; the EIF can be adapted for any type of health outcome, however (see ).
Figure 2. Example – proposed pathophysiology of gastric cancer showing key events in cancer development and events that may be meaningfully affected by exposure. a – Jensen et al. (Citation2012). b – Wu and Cho (Citation2004).
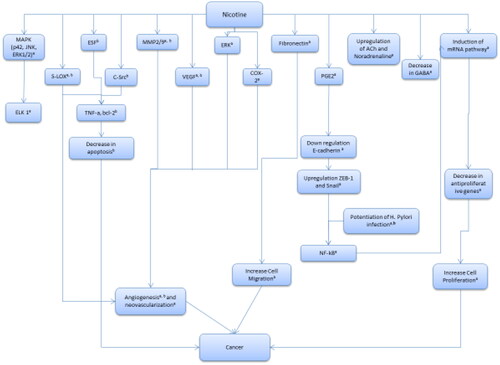
Critical assessment of the literature
A key element of the EIF is its consideration of study quality. While all quality assessments require expert judgment, the EIF maximizes the reproducibility of the quality assessment by applying pre-determined criteria, such as those applied in Institute of Medicine’s (IOM) report, Gulf War and Health (2010). The protocol should document the selected quality criteria and specify a reasonable percentage of papers for dual review and re-assessment by one or more senior scientists for quality assessment purposes.
Epidemiology study quality
Rather than using numerical quality scores that imply a precision that does not exist, the epidemiology studies are rated as “adequate,” “fair,” or “inadequate” quality based on expert assessment of the study design, execution, reporting, and potential for bias – the key elements of epidemiology study quality () (Sulsky et al. Citation2002; Sanderson et al. Citation2007; Lang and Kleijnen Citation2010; LaKind et al. Citation2020; Burns and LaKind Citation2021). Studies rated as “adequate” quality are expected to provide reasonably valid and reliable evidence that either suggests or fails to suggest an association between the exposure and the disease or outcome of interest. “Fair” studies may include certain design flaws or other limitations but still provide supporting evidence regarding the presence or absence of an association. “Inadequate” studies do not contribute to the analysis because of their limitations. More stringent criteria can be applied depending on the reason for applying the EIF, as specified in the problem formulation statement.
Table 1. Epidemiology study attributes and their contributions to assessment of quality (Sulsky et al. Citation2002).
Toxicology study quality
The quality of toxicological experiments can be rated according to a reasonably reproducible scale, though professional judgment is still required. For example, the Klimisch et al. (Citation1997) criteria were developed for evaluating studies for use in quantitative risk assessments (QRA) that provide numerical estimates of changes in risks associated with quantified changes in exposure. QRA require high-quality data. When the EIF is intended to be used for hazard identification (i.e. judging whether a hazard is present or absent), data from lower quality studies can provide supportive evidence regarding the potential association between exposure to the chemical of interest and the outcome. More stringent criteria for inclusion based on study quality can be implemented if appropriate. Modifications to the Klimisch et al. (Citation1997) criteria are shown in . Modifications include the addition of a study quality category, refinements to the requirements for studies receiving a study quality score of 3, and criteria for studies that would be categorized as not useful.
Table 2. Criteria for evaluating the quality of toxicological studies.Table Footnote*
Synthesizing the evidence within disciplines
Epidemiology
If the EIF is being used to identify evidence of any potential signal indicating a possible hazard, then both adequate and fair quality epidemiology studies should be included. If the EIF is being used for regulatory decision-making or to support causal inference, then the inclusion criteria may be more stringent, to include only higher quality studies. Evidence from poor quality studies is excluded from the assessment due to lack of reliability.
Evaluating the epidemiological evidence for the EIF begins with the most specific human health outcomes studied in the included epidemiological literature and moves toward categories of related outcomes as the data allow and the problem formulation dictates. If the goal of applying the EIF is hazard identification or to identify any potential signal of a health effect, then it may be appropriate to consider lower quality evidence that could provide a more sensitive tool for signal detection, though this decision increases the uncertainty of the conclusions. If the goal is causal inference, then only higher quality evidence should be included.
Grouping specific outcomes into categories can be complicated by the varied definitions adopted by different authors. Maintaining the different definitions can be useful for gap analysis, particularly if different conclusions are supported by different outcome definitions; this situation could point to areas needing further investigation. If groupings are not possible or advisable, the epidemiological evidence may be considered for each individual outcome reported in the literature.
Any one epidemiology study may include data for several outcomes, and the quality of evidence within the study may depend on the specific outcome. For example, a study reporting results for multiple outcomes may have had sufficient power and precision to detect changes in risk for more common or easily diagnosed diseases (e.g. certain types of cancer), but not for outcomes that are less specific (e.g. combined diseases that may have different etiologies) or that are less common (e.g. resulting in insufficient numbers of cases for adequately powered statistical power analyses). Thus, while a study may be ranked as being of adequate overall quality, the quality of the evidence for each individual outcome of interest must also be assessed. After evaluating the available evidence for each outcome, the epidemiological evidence is synthesized and categorized as shown in .
Table 3. Synthesis of epidemiological evidence for hazard identification.Table Footnote*
Toxicology
Disease-based organization of toxicological data
After cataloging all of the human health outcomes (i.e. diseases) that were assessed in one or more epidemiological studies with acceptable quality, the animal and mechanistic studies, which include animal toxicity studies, in vivo (e.g. animal or human) and in vitro (e.g. cellular or bacterial) studies, are grouped according to measurements or endpoints that correspond to the outcomes from the epidemiological literature. This grouping is based on the purpose of the toxicological study as described by the author(s) and any measured apical endpoints that are relevant to the human disease of interest. The reasons for grouping according to the authors’ purpose are (1) to classify the evidence as major or minor within domains (defined below) and (2) to identify sources of uncertainty in the linkage with the human disease of interest during the evidence integration step. For example, the author(s) of an exploratory study may indicate that the study addressed a specific endpoint (e.g. inflammation) in the development of a specific disease (e.g. cardiovascular disease). However, the bridging step may indicate a lack of evidence to support the proposed connection. Including results from exploratory studies – those studies where authors pursued a hypothesis in absence of other evidence – would increase the amount of uncertainty in the evidence provided by applying the EIF. In contrast, if a given toxicological study was conducted to evaluate a specific relationship with a strong basis in prior research, including its results would reduce the amount of uncertainty in the evidence provided by applying the EIF. As noted above, methods for searching the literature and conducting discipline-specific critical reviews and syntheses of the literature are likely familiar to readers and are not presented here.
Mechanism-based organization of toxicological data: major and minor domains of toxicological data
Toxicological studies may provide evidence of an association between exposure to a chemical and the occurrence of endpoints or events in the development of the disease, for example, based on the proposed mechanism or mode of action. The occurrence of such precursor events that might, or might not, lead to the disease or outcome of interest cannot always be directly tied to human health outcomes. To account for this inherent uncertainty regarding the relevance of the toxicological data to human health, they are classified into domains and major and minor subcategories. For the cancer example provided here, we used the classifications proposed by Dekant and Bridges (Citation2016). Major evidence includes endpoints clearly related to or associated with the development of the human disease, such as the apical endpoint; gross physiological or histopathological changes that are sustained and clearly analogous to health effects observed in humans with relevant exposures; and endpoints that have been identified as events in the development of specific human health effects. The evidence in each domain is subdivided into information that is considered to be of likely relevance or of uncertain relevance to the human health outcome; this division is based on the bridging activity that links the observed endpoints following exposure to the chemical to the events in the development or progression of the human disease. Evidence in a major domain may be of uncertain relevance to human health if the endpoint in question is not reported in high-quality epidemiological studies or occurs by a mode of action or involves events that occur in animals but not in humans. Uncertain major evidence may be derived from a toxicological study designed to test a hypothesis about the relationship between an exposure and an endpoint when the mode of action or the association with events in the etiology of the disease or outcome is not clear. Minor evidence may be considered of likely relevance to the human health effect, or it may be of uncertain relevance. The latter includes endpoints with unknown reversibility or sustainability, that are less directly analogous or are less clearly related to the human disease in question. Definitions of the types of data included in each domain and subcategory are provided in , with examples of data that would be included if the outcome of interest was cancer. The definitions in can be adapted for non-cancer outcomes; contact the authors for assistance or information.
Table 4. Categories of toxicological evidence, with examples from domains relevant to cancer.Table Footnote*
Synthesizing the toxicological data and drawing conclusions about the presence or absence of a signal (hazard identification)
Within the major and minor categories of each domain (i.e. biochemical, functional, gross, pathological, gene expression, and – for cancer outcomes – genotoxic), the available toxicological data are synthesized to identify those that suggest a signal is present and those that suggest a signal is absent. provides a framework to reach an overall conclusion that a signal is present or absent, or that the overall toxicological evidence for that domain is insufficient or equivocal. The sensitivity of the EIF must be tailored to its purpose, such that more data of more variable quality and relevance will be considered for hazard identification (i.e. very sensitive for signal detection) and data will be restricted to higher quality evidence that is of more certain relevance to human health for causal inference. Note that higher levels of sensitivity will increase both the amount of information to be evaluated and the degree of uncertainty in the evidence.
Table 5. Framework for synthesizing the toxicological evidence for each outcome or disease, integrating evidence in the major and minor categories of each domain.Table Footnote*
The overall toxicological conclusions within the major and minor categories of each domain are determined by the proportions of endpoints that provide evidence of a signal (signal present) versus no evidence of a signal (signal absent). For hazard identification that requires a higher level of sensitivity, we recommend setting the threshold to determine the toxicological evidence for a signal between 40% and 60%. If fewer than 40% of the results within a major or minor domain are statistically significant, we propose that the toxicological weight of evidence in that domain does not suggest a signal is present. If the proportion of the measurements that are statistically significantly associated with the exposure is between 40% and 60%, we propose that the evidence for a signal is equivocal. If more than 60% of the results within a domain are statistically significant, we propose that the weight of evidence suggests a signal is present.
The EIF process can be adjusted at this stage to increase or decrease its sensitivity, depending on the purpose for using it. For example, if the EIF were implemented to make causal inferences, rather than for hazard identification, the threshold proportion should be less sensitive; proportions of statistically significant results considered to indicate the evidence suggests a signal is present could be increased to 45%, 50%, or higher. We suggest it is acceptable to look at trends across studies or to rely on statistical significance for this application because only studies of acceptable methodological quality have been included at this stage, and alternative explanations for the observations have already been accounted for in the critical review of each individual study.
Synthesizing the evidence across disciplines
Evidence integration: disease-based and mechanism-based components
shows how to integrate the overall toxicological and epidemiological conclusions (i.e. discipline-specific syntheses) to arrive at a summary conclusion about the weight of evidence supporting the presence or absence of a signal pertinent to each disease of interest. For the disease-based component of the EIF, this integration is driven by, and generally favors, the epidemiological evidence, with the chemical-specific toxicological data providing supporting evidence. This is the case even though the exposures analyzed epidemiologically are likely to be to mixtures and not to the chemical of interest, per se. In the case of mixed exposures, the conclusion that the evidence does not suggest the presence of a signal for the mixture would also apply to the individual chemicals in the mixture. The conclusion of “no signal” based on the epidemiology data is stronger than (a) concluding that the evidence suggests a signal is present for the mixture, because the signal could be due to a different component of the mixture; or (b) concluding that the data are insufficient to reach a conclusion for the mixture, because the lack of detectible signal could be due to the dilution or contradictory of effects of different components of the mixture.
Table 6. Summary conclusions integrating the available toxicological and epidemiological evidence.
To integrate the toxicological and epidemiological evidence, the tissue or cell type for which evidence is provided in the toxicological studies is considered in combination with the specific endpoints reported in the epidemiological literature. Those results with concordance are considered across species for each individual domain and cancer hallmark or enabling characteristic. The epidemiological data may or may not be concordant and therefore may or may not corroborate conclusions supported by the toxicological data.
The shell provided as shows how the data can be summarized to facilitate integrating the epidemiological and toxicological evidence. Each cell should be filled in with the number of endpoints in each domain that provide evidence of an effect and the total number of endpoints reported within that domain. The proportions are then compared with the threshold proportions that were determined in advance to indicate that a signal may be present, is equivocal, or may be absent. The threshold proportions are adjustable to address different potential purposes of applying the EIF.
Table 7. Presence/absence of signals from the epidemiological and toxicological evidence.
Mechanism-based component of the EIF
The mechanism-based component of the EIF organizes the data based on the proposed mechanisms of effect and mechanistic data supporting events leading to each human health endpoint. For this methods paper, we describe organizing data around the hallmarks and enabling characteristics leading to cancer (Hanahan and Weinberg Citation2011; Senga and Grose Citation2021). A different, non-cancer outcome would require evidence to be organized around different mechanisms or events.
The hallmarks of cancer include two enabling characteristics: (1) genome instability, which is the potential to generate genetic changes that orchestrate cancer development, and (2) inflammation, which provides a favorable environment for cancer development. There are eight hallmark characteristics associated with the creation of a tumor microenvironment and include characteristics that allow cancer cells to survive, proliferate, and metastasize. The eight hallmarks are cell proliferation, angiogenesis, evasion of growth suppressors, resistance of cell death, evasion of immune destruction, invasion and metastasis, enabling of replicative immortality, and reprogramming of energy metabolism (Hanahan and Weinberg Citation2011).
To apply the mechanism component of the EIF to cancer, in general, each of the toxicological endpoints and measurements is evaluated to determine if it either is a direct measurement of a cancer hallmark (e.g. cell proliferation or inflammation) or is directly relevant to a cancer hallmark (e.g. increases in reactive oxygen species leading to oxidative stress). Endpoints that are specific to a hallmark or enabling characteristic provide evidence “likely” relevant to cancer, while nonspecific endpoints that could be related to multiple cancer hallmarks, or those relevant to multiple diseases (e.g. migration of endothelial cells is associated with angiogenesis but could also be relevant to cardiovascular disease) are of “uncertain” relevance. For non-cancer outcomes, an analogous listing of mechanisms pertinent to the specific disease of interest must be created.
To integrate the toxicological and epidemiological evidence regarding the association between chemical exposure and cancer, the types of tumors reported in the epidemiological literature are used to assess tissue concordance (i.e. to identify which tumor types had been investigated in both toxicological and epidemiological studies) for each individual domain and cancer hallmark or enabling characteristic. The mechanism-based approach allows for all potentially relevant endpoints or measurements from any human, animal, or mechanistic (human in vivo, animal in vivo, or in vitro) study to be included in the analysis without using the authors’ stated objective for the study to classify the endpoint of interest. It is data-derived and it excludes theoretical mechanisms of action. Furthermore, this approach to organizing the available information allows integration of more of the toxicological data than does the disease-based approach.
To integrate this information with epidemiological data, a review of the types of tumors reported in humans exposed to the chemical of interest is conducted to assess site or tissue concordance, that is, tumor types that were investigated in both toxicological and epidemiological studies. If observations from the epidemiological data provide corroborating evidence due to tissue or site concordance, evidence of a signal is stronger than if there is no corroboration.
Like , shows a method to summarize the evidence available from the mechanism-based component of the EIF to integrate the evidence according to the rules described in . The proportion of endpoints suggesting an effect in each major and minor domain are to be compared with the threshold proportions determined a priori to indicate that the evidence suggests a signal is present or absent, or that the evidence is equivocal. Adjustments to the threshold proportions will adjust the sensitivity of the EIF for its different potential purposes.
Table 8. Mechanistic evidence: Exposure related to hallmarks of cancer development.
Overall conclusions based on mechanism-based component to evidence integration
shows an example of how to summarize the integrated toxicological and epidemiological evidence provided in , in the mechanism-based component of the EIF.
Table 9. Summary and integration of evidence from the mechanism-based approach regarding the presence or absence of a signal.
Gap analysis
Completing a gap analysis within the EIF entails examining the summary data in . Domains for which evidence is either absent or insufficient to reach a conclusion indicate specific research questions to be followed up. Domains that provide equivocal evidence in the disease-based and/or the mechanism-based components of the EIF also indicate areas to be investigated more thoroughly in the future, particularly if there are toxicological endpoints that are of likely relevance to human health but epidemiology or clinical studies are lacking. If toxicological endpoints suggest an effect and relevant epidemiology studies do not indicate an effect of exposure to the chemical, this indicates uncertainty in the toxicological evidence that might be clarified in subsequent, targeted studies. Any conclusion that differs depending on the organization of the data or the definition of the endpoint also indicates a gap to be filled by targeted research studies in the future. The gap analysis should also account for any difficulties encountered while attempting to bridge between the epidemiology and toxicology data. Important gaps in knowledge would be identified if there was limited information about the mechanism of effect or mode of action of the chemical or the pathophysiology of the endpoints of interest, or if there was limited information about how exposure to the chemical might influence the pathophysiology of the endpoints.
Discussion
The value of this, and any other evidence integration activity, is determined by the quantity and quality of the data available. If the epidemiology database is insufficient, then the EIF will be limited in application to in silico, in vitro, and animal in vivo evidence and the relevance of the data to human health will depend on that state of knowledge regarding the pathophysiology of the disease(s) in question. Similarly, if there are very few toxicological data available for a given endpoint or domain, following the EIF may lead to overly definitive conclusions about the presence or absence of signal if those data happen to be consistent. The integrative tables show how much evidence is available that supports the presence or absence of a signal and how relevant that evidence is to the human disease of interest.
The EIF is most easily applied when there is a high degree of specificity in both the outcome and exposure measures, facilitating linkages between the epidemiological and toxicological evidence. It is inevitable that establishing links between the human, animal, and mechanistic data will be challenging because of inherent differences in epidemiologic data compared with that from controlled experiments. Exposures in epidemiological studies are always mixed, and evidence can rarely be tied to exposure to a single chemical. In experiments, many potential variables can be controlled by study design, and effects can be attributed to exposure to the chemical per se. Nevertheless, the epidemiological data provide information on humans, who always experience complex and mixed exposures. Likewise, the toxicological data provide information specific to the effects of individual chemicals that is not typically available from epidemiological studies.
Defining outcomes also presents challenges. Within the epidemiology database, different authors may define or group diseases differently, adding variability to the system. For example, studies of cardiac outcomes might define the outcome of interest as heart disease or cardiovascular disease. Different authors might include different subsets of the specific diseases that fit this category, such as stroke, myocardial infarction, and heart failure. Similarly, toxicological studies may be based on a hypothesis regarding relationships between exposures and outcomes without a solid evidence base. These factors complicate the synthesis of the data and can make it difficult to identify concordance between human health outcomes and the types of endpoints studied toxicologically. The value of the EIF is its inclusion of all available information, and its explicit discussion of sources of uncertainty and the effect of uncertainty on the conclusions that can be drawn.
The disease-based component of the EIF is driven by the diseases observed and evaluated in humans and is enhanced by an understanding of the pathophysiology of those specific diseases. It allows for consideration of endpoints that mark the progression of disease, if they are measurable in humans or animals, but it does not address potential initiating events or include assay results at the cellular level that are not linked to identifiable human pathology. In addition, endpoints that share common biological mechanisms, but not similar pathophysiological manifestations, are often considered relevant to different disease groupings. Therefore, applying the disease-based categorization system requires an additional integrative step to identify common modes of action across specific diseases within a group.
In contrast, the mechanism-based component of the EIF accounts for general mechanisms of disease causation and can incorporate assay results at the cellular level that have been used as potential indicators of disease risk (e.g. Ames assay). Poor or incomplete understanding of the mechanisms leading to diseases proposed to be associated with exposure will increase the level of uncertainty of the conclusions. Recognizing and delineating these uncertainties in basic medical and scientific knowledge may direct future research decisions.
As much as the EIF aims to be objective and to make explicit the processes for rating and weighting the available evidence, some qualitative, subjective elements remain. Professional judgment is required to rate the quality of the underlying studies and to determine which human and toxicological endpoints are related sufficiently to be grouped together. The disease-based approach to organizing the toxicological data relies on the original authors’ definitions of outcomes and their study purposes, which influence the conclusions drawn from the evidence integration. The mechanism-based approach is limited by the current state of knowledge regarding the mechanisms of disease, and uncertainties about those mechanisms increase the uncertainties inherent in linking the toxicological and epidemiological evidence.
Both the disease-based and mechanism-based components incorporate in vitro and gene or protein expression research, which is being more commonly performed as whole animal testing is limited or phased out. While much progress is being made in validating these types of assays, questions remain about the likelihood that the endpoints they measure will progress to an apical endpoint and about their ability to predict future diseases. These types of measurements provide a large quantity of data that is of uncertain relevance to human disease and may or may not contribute to signal detection.
The EIF represents an advance in the methods available for weight of evidence-type analyses. It accounts for the quality and quantity of the underlying data and explicitly addresses uncertainties in the data. It uses information about specific disease etiology to link the epidemiological and toxicological evidence. Categorizing the data into major and minor domains captures the relationship between the toxicological measures and the apical endpoint, as well as the human disease. Data included in minor domains are relevant to the analysis and explicitly provide more supportive than direct evidence. The EIF can be adjusted by modifying the quality threshold for including studies. If only the best quality subset of studies is included, a signal is less likely to be detected but confidence in the conclusion is higher than if lower quality studies were included. If studies with more limitations are included, a signal is more likely to be detected.
The EIF can additionally be adjusted by modifying the definition of equipoise to classify evidence as equivocal. Increasing the likelihood of detecting a signal may be appropriate when there are great concerns about the potential risk expected to result from the exposure or when the state of knowledge is not far advanced or if the goal is hazard identification. A more restrictive system that is less likely to detect a signal may be appropriate when the cost of committing to a decision is high, when the state of knowledge is mature, and/or when the goal is to make causal inferences.
Clear advantages can be gained by incorporating multiple approaches into the EIF. The disease-based component of the framework allows toxicological data that are relevant to human health endpoints to be directly identified, by way of the pathophysiological processes that lead to the disease. The mechanism-based approach directly assesses the evidence indicating that exposure affects indicators of the development and progression of a disease state. Categorizing information according to the biological mechanism of the chemical’s effect, such as the hallmarks and enablers of cancer, allows more complete use of available toxicological data by incorporating information about measurable endpoints that are associated with each mechanism. These endpoints may impart insights into the potential for human health effects in the absence of epidemiology data.
The EIF is flexible and can incorporate new information at any level (epidemiological, in vivo, and in vitro), as it becomes available. The EIF supports scientifically defensible conclusions regarding the connection between exposure and outcome based on the plausibility of the proposed mechanisms of effect. Applying the EIF also allows for data gaps to be identified and for that information to be used in targeting future research to address key questions.
Authors CRediT statement
Sandra Sulsky: Conceptualization, methodology (supporting), supervision, writing – original draft (lead), data visualization, validation, writing – review and editing (supporting). Tracy Greene: Methodology (supporting), project administration (lead), writing – original draft, writing – review and editing (supporting). P. Robinan Gentry: Conceptualization, methodology (lead), supervision, writing – original draft (supporting), data visualization (supporting), validation (lead), writing – review and editing (lead).
Abbreviations | ||
AOP | = | adverse outcome pathways |
DNA | = | deoxyribonucleic acid |
DPA | = | diphenylamine |
EFSA | = | European Food Safety Agency |
EIF | = | evidence integration framework |
GRADE | = | grading of recommendations assessment, development and evaluation |
IOM | = | Institute of Medicine |
KE | = | Key Event |
mRNA | = | messenger-ribonucleaic acid |
NASEM | = | National Academies of Science, Engineering and Medicine |
NRC | = | National Research Center |
NTP | = | National Toxicology Program |
OECD | = | Organization for Economic Co-operation and Development |
OHAT | = | Office of Health Assessment and Translation |
PGI2 | = | prostacyclin |
PRISMA | = | preferred reporting items for systematic reviews and meta-analyses |
QRA | = | quantitative risk assessment |
SCHEER | = | Scientific Committee on Health, Environmental and Emerging Risks |
SOD | = | superoxide dismutase |
USEPA | = | United States Environmental Protection Agency |
Acknowledgments
The authors acknowledge and appreciate the comments of the Editor and the external reviewers (selected by the Editor) who were anonymous to the authors. Reviewers’ constructive comments helped strengthen the manuscript.
Declaration of interest
The authors’ employment affiliations are shown in the title block above. Ramboll is a private consulting firm providing services to private and public organizations on toxicology and risk assessment issues.
This project was a concept presented by Ramboll to Altria Client Services, Inc. (Altria). This work was supported by Altria; however, no one from Altria was involved in the preparation of the manuscript. Altria’s Dr. Donna Smith, Associate Fellow of Pre-Clinical within Regulatory Sciences for Altria, was given the opportunity to review the draft manuscript. The purpose of this review was for the authors to receive input on the clarity of the science presented but not on the interpretation of research results. The scientific methods and guidance presented were not subject to the sponsor’s control; the contents of this manuscript reflect solely the views of the authors. The manuscript provides a method for organizing data available in the scientific literature, but no specific examples or data are included. We therefore did not seek ethics approval or patient consent. No outside materials are included or reproduced in this manuscript.
None of the authors received direct compensation from Altria for this project. The project was funded through contracts between Altria and Ramboll. All the scientists of Ramboll (SS, RG, and TG) involved in the development of the current manuscript were provided salary compensation as part of their employment as consultants. There are no conflicts of interest for any of the authors to disclose related to the submission of this manuscript.
The authors would like to thank Dr. Donna Smith, who served as the sponsor’s technical contact, for her helpful support and patience during this effort. We also acknowledge and appreciate the efforts of many past and current scientists at Ramboll who contributed to early applications of the EIF that allowed us to refine the methods described in this manuscript.
References
- Applegate CC, Rowles JK, 3rd, Erdman JW. Jr. 2019. Can lycopene impact the androgen axis in prostate cancer?: a systematic review of cell culture and animal studies. Nutrients. 11(3):633. doi: 10.3390/nu11030633.
- Burns CJ, LaKind JS. 2021. Using the matrix to bridge the epidemiology/risk assessment gap: a case study of 2,4-D. Crit Rev Toxicol. 51(7):591–599. doi: 10.1080/10408444.2021.1997911.
- Dekant W, Bridges J. 2016. A quantitative weight of evidence methodology for the assessment of reproductive and developmental toxicity and its application for classification and labelling of chemicals. Regul Toxicol Pharmacol. 82:173–185. doi: 10.1016/j.yrtph.2016.09.009.
- EFSA (European Food Safety Authority). 2017a. Guidance on the assessment of the biological relevance of data in scientific assessments. EFSA J. 15(8):4970.
- EFSA (European Safety Authority). 2017b. Guidance on the use of the weight of evidence approach in scientific assessments. EFSA J. 15(8):4971.
- European Commission (Scientific Committee on Health, Environmental and Emerging Risks (SCHEER)). 2018. Memorandum on weight of evidence and uncertainties Revision 2018. https://health.ec.europa.eu/system/files/2019-02/scheer_o_014_0.pdf.
- Guyatt G, Oxman AD, Akl EA, Kunz R, Vist G, Brozek J, Norris S, Falck-Ytter Y, Glasziou P, Debeer H, et al. 2011. GRADE guidelines: 1. Introduction – GRADE evidence profiles and summary of findings tables. J Clin Epidemiol. 64(4):383–394. doi: 10.1016/j.jclinepi.2010.04.026.
- Hanahan D, Weinberg RA. 2011. Hallmarks of cancer: the next generation. Cell. 144(5):646–674. doi: 10.1016/j.cell.2011.02.013.
- Institute of Medicine. 2010. Gulf war and health. Volume 8: update of health effects of serving in the Gulf war. Committee on Gulf water and health: health effects of serving in the Gulf war, update 2009. Washington, DC: Board on the Health of Select Populations, Institute of Medicine of the National Academies, The National Academies Press.
- Jensen K, Afroze S, Munshi MK, Guerrier M, Glaser SS. 2012. Mechanisms for nicotine in the development and progression of gastrointestinal cancers. Transl Gastrointest Cancer. 1:81–87.
- Klimisch HJ, Andreae M, Tillmann U. 1997. A systematic approach for evaluating the quality of experimental toxicological and ecotoxicological data. Regul Toxicol Pharmacol. 25(1):1–5. doi: 10.1006/rtph.1996.1076.
- Krewski D, Saunders-Hastings P, Baan RA, Barton-Maclaren TS, Browne P, Chiu WA, Gwinn M, Hartung T, Kraft AD, Lam J, et al. 2022. Development of an evidence-based risk assessment framework. ALTEX. 39(4):667–693. doi: 10.14573/altex.2004041.
- LaKind JS, Naiman J, Burns CJ. 2020. Translation of exposure and epidemiology for risk assessment: a shifting paradigm. Int J Environ Res Public Health. 17(12):4220. doi: 10.3390/ijerph17124220.
- Lang S, Kleijnen J. 2010. Quality assessment tools for observational studies: lack of consensus. Int J Evid Based Healthc. 8(4):247. doi: 10.1111/j.1744-1609.2010.00195.x.
- Mohler D, Liberati A, Tetzlaff J, Altman DG, The Prisma Group. 2009. Preferred reporting items for systematic reviews and meta-analyses: the PRISMA statement. Ann Intern Med. 151(4):264–269.
- National Research Council. 2014. Review of EPA’s integrated risk information system (IRIS) process. Washington, DC: Committee to Review the IRIS Process, Board on Environmental Studies and Toxicology, Division on Earth and Life Studies, National Research Council, The National Academies Press.
- National Toxicology Program. 2019a. Report on carcinogen process and listing criteria. National Toxicology Program, United States Department of Health and Human Services. https://ntp.niehs.nih.gov/pubhealth/roc/process/index.html.
- National Toxicology Program. 2019b. Handbook for conducting a literature-based health assessment using OHAT approach for systematic review and evidence integration. Office of Health Assessment and Translation, Division, National Toxicology Program, National Institute of Environmental Health Sciences. March 4, 2019 [online]. https://ntp.niehs.nih.gov/ntp/ohat/pubs/handbookmarch2019_508.pdf.
- OECD. 2018. Users’ handbook supplement to the guidance document for developing and accessing adverse outcome pathways, Series on Testing and Assessment No. 233. Series on Adverse Outcome Pathways No. 1.
- OECD. 2019. Guiding principles and key elements for establishing a weight of evidence for chemical assessment, Series on Testing and Assessment No. 311, Environment, Health and Safety Division, Environment Directorate. https://www.oecd.org/chemicalsafety/risk-assessment/guiding-principles-and-key-elements-for-establishing-a-weight-of-evidence-for-chemical-assessment.pdf.
- Sanderson S, Tatt IT, Higgins PT. 2007. Tools for assessing quality and susceptibility to bias in observational studies in epidemiology: a systematic review and annotated bibliography. Int J Epidemiol. 36(3):666–676. doi: 10.1093/ije/dym018.
- Schwarzman MR, Ackerman JM, Dairkee SH, Fenton SE, Johnson D, Navarro KM, Osborne G, Rudel RA, Solomon GM, Zeise L, et al. 2015. Screening for chemical contributions to breast cancer risk: a case study for chemical safety evaluation. Environ Health Perspect. 123(12):1255–1264. doi: 10.1289/ehp.1408337.
- Senga SS, Grose RP. 2021. Hallmarks of cancer-the new testament. Open Biol. 11(1):200358.
- Smith MT, Guyton KZ, Kleinstreuer N, Borrel A, Cardenas A, Chiu WA, Felsher DW, Gibbons CF, Goodson WH, 3rd, Houck KA, et al. 2020. The key characteristics of carcinogens: relationship to the hallmarks of cancer, relevant biomarkers, and assays to measure them. Cancer Epidemiol Biomarkers Prev. 29(10):1887–1903. doi: 10.1158/1055-9965.EPI-19-1346.
- Sulsky SI, Hooven FH, Burch MT, Mundt KA. 2002. Critical review of the epidemiological literature on the potential cardiovascular effects of occupational carbon disulfide exposure. Int Arch Occup Environ Health. 75(6):365–380. doi: 10.1007/s00420-001-0309-x.
- Suter G, Nichols J, Lavoie E, Cormier S. 2020. Systematic review and weight of evidence are integral to ecological and human health assessments: they need an integrated framework. Integr Environ Assess Manag. 16(5):718–728. doi: 10.1002/ieam.4271.
- USEPA (Untied States Environmental Protection Agency). 1986. Guidelines for Carcinogen Risk Assessment. Washington, DC: United States Environmental Protection Agency (USEPA), Risk Assessment Forum. Published on September 24, 1986, EPA/630/R-00/004. Fed Register 51(185), 33992–34003.
- USEPA (United States Environmental Protection Agency). 2011. Endocrine Disruptor Screening Programme, 2011. Weight-of-evidence guidance document: https://www.epa.gov/endocrinedisruption/endocrine-disruptor-screening-program-documents. Direct link to guidance document: https://www.regulations.gov/document?D=EPA-HQ-OPPT-2010-0877-0021.
- USEPA (United States Environmental Protection Agency). 2016. Weight of evidence in ecological assessment. EPA/100/R-16/001. Washington, DC, December.
- USEPA (United States Environmental Protection Agency). 2018. Application of systematic review in TSCA evaluations. Office of Chemical Safety and Pollution Prevention. EPA Document# 740-P1-8001.
- Vandenberg LN, Ågerstrand M, Beronius A, Beausoleil C, Bergman Å, Bero LA, Bornehag CG, Boyer CS, Cooper GS, Cotgreave I, et al. 2016. A proposed framework for the systematic review and integrated assessment (SYRINA) of endocrine disrupting chemicals. Environ Health. 15(1):74. doi: 10.1186/s12940-016-0156-6.
- Villeneuve DL, Angrish MM, Fortin MC, Katsiadaki I, Leonard M, Margiotta-Casaluci L, Munn S, O'Brien JM, Pollesch NL, Smith LC, et al. 2018. Adverse outcome pathway networks II: network analytics. Environ Toxicol Chem. 37(6):1734–1748. doi: 10.1002/etc.4124.
- Villeneuve DL, Crump D, Garcia-Reyero N, Hecker M, Hutchinson TH, LaLone CA, Landesmann B, Lettieri T, Munn S, Nepelska M, et al. 2014a. Adverse outcome pathway (AOP) development I: strategies and principles. Toxicol Sci. 142(2):312–320.
- Villeneuve DL, Crump D, Garcia-Reyero N, Hecker M, Hutchinson TH, LaLone CA, Landesmann B, Lettieri T, Munn S, Nepelska M, et al. 2014b. Adverse outcome pathway development II: best practices. Toxicol Sci. 142(2):321–330.
- Vincent MJ, Parker A, Maier A. 2017. Cleaning and asthma: a systematic review and approach for effective safety assessment. Regul Toxicol Pharmacol. 90:231–243. doi: 10.1016/j.yrtph.2017.09.013.
- Whaley P, Edwards SW, Kraft A, Nyhan K, Shapiro A, Watford S, Wattam S, Wolffe T, Angrish M. 2020. Knowledge organization systems for systematic chemical assessments. Environ Health Perspect. 128(12):125001. doi: 10.1289/EHP6994.
- Wikoff D, Lewis RJ, Erraguntla N, Franzen A, Foreman J. 2020. Facilitation of risk assessment with evidence-based methods – a framework for use of systematic mapping and systematic reviews in determining hazard, developing toxicity values, and characterizing uncertainty. Regul Toxicol Pharmacol. 118:104790. doi: 10.1016/j.yrtph.2020.104790.
- Wu W, Cho C. 2004. The pharmacological actions of nicotine on the gastrointestinal tract. J Pharmacol Sci. 94:348–358.