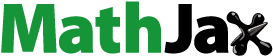
ABSTRACT
This study analyzes the general public’s framing of ‘mental health’ and critically assesses the implications of these findings. A mismatch between how people think about mental health and what messages are used in mental health campaigns may hinder attempts to improve mental health awareness and reduce stigma. We have conducted frame analysis by using a combination of topic modeling and sentiment analysis, examining 10 years of mental health-related tweets (n = 695,414). The results reveal seven distinctive mental health frames: ‘Awareness’, ‘Feelings and Problematization’, ‘Classification’, ‘Accessibility and Funding’, ‘Stigma’, ‘Service’, and ‘Youth’ (arranged by salience). In analyzing these frames, we have learned that (1) the general awareness about mental health relates to mental illness, while health and well-being framing, although present, is prone to low quality of information, (2) mental health discourse is often used to problematize social issues and externalize personal anxieties, which tends toward trivialization and, possibly, treatment delays, (3) mental health discourse often revolves around popularized mental illness (e.g., depression, anxiety, but not neurocognitive diseases), (4) the mental health ‘Stigma’ frame is not overly pronounced; it revolves around violence, fear, and madness, (5) mental health is frequently politicized, especially concerning gun laws in the US and service accessibility and funding in the UK. Additionally, some narrower frames discovered may warrant further examination. For instance, PTSD is mostly framed around veterans and suicide, ADHD around youth, and substance abuse in relation to women, teens, and impoverished.
Introduction
Mental illness is the leading cause of disability worldwide (Whiteford et al., Citation2015). Even though some mental health concerns are highly manageable, people with symptoms of mental illness tend to avoid help-seeking, as mental health discourse is associated with stigma and lack of awareness (Chisholm et al., Citation2016). Mental health advocates, public health authorities, researchers, and educators are striving to bring positive awareness to the subject of mental health. However, the evidence about the effectiveness of these programs is inconclusive (Henderson et al., Citation2013).
In this paper, we focus on two main reasons to clarify the difficulties in raising awareness and de-stigmatizing mental health. First, many scholars, as well as the World Health Organization, argue that “health is a state of complete physical, mental and social well-being and not merely the absence of disease or infirmity” (Kühn & Rieger, Citation2017, p. 887). At the same time, even in the presence of a clear health definition, the research community continues contestations of mental health definitions and trying to understand how it is different from being merely an absence of mental illness (Galderisi et al., Citation2015; Manwell et al., Citation2015; Westerhof & Keyes, Citation2010). Hence, this study aims to provide an empirical contribution by assessing how members of the general public understand what mental health is (not) and what specifically about mental health that is important.
Second, we posit that mental health advocacy efforts may be lost due to cultural entropy – where the intended message vanishes in a myriad of conflicting information, resulting in unintended interpretations or, oftentimes, a complete failure to deliver the message to the audience (McDonnell, Citation2016). Previous studies have mostly focused on the media production of mental health frames, demonstrating that media portrayals of mental health are frequently overrun with stigma and sensationalism (Stuart, Citation2006; Whitley & Berry, Citation2013). However, and especially for topics characterized by adverse personal and social outcomes (i.e. mental health), solutions to the challenges associated with such issues will be contingent on the individuals’ appraisal of the causes thereof or, in other words, their individual framing (Benford & Snow, Citation2000).
To learn how the general public frames mental health, we consulted the online social media platform Twitter. Online social media networks increasingly became the platform where discussion of (mental) health occurs (Naslund et al., Citation2016). In addition to becoming the major sources of information (Shearer & Gottfried, Citation2017), social media networks are also said to be more informationally-inclusive as they allow marginalized members of society to voice their discontent, express frustration and create awareness about issues from a lived-experience perspective (Papacharissi, Citation2015). In fact, the framing of social issues on social media has proven to build new frames and shift the dominant narratives. The #BlackLivesMatter social movement could be considered a great example where the voice of the people usually excluded from the discourse bypassed the mainstream media, creating salience for the frames that are currently motivating political and social change (Jackson & Foucault Welles, Citation2016).
Recent studies have successfully used Twitter to assess the attitudes of the general public regarding mental illness. On the one hand, the findings showed that mental illness related discourse on Twitter was more supportive and neutral than that of the traditional media, focused on the increased awareness, sharing research, and other consumer resources. On the other hand, Twitter discourse was not immune from more stigmatizing frames, even though these were somewhat different – more focused on trivialization of mental health problems (e.g., “snapping out of it”) or sharing of inaccurate beliefs (e.g., confusion of schizophrenia with the bipolar disorder) (Budenz et al., Citation2019; Joseph et al., Citation2015; Reavley & Pilkington, Citation2014; Robinson et al., Citation2019). While these studies can give an idea about the framing of mental health discourse, the research objectives have either been focused on specific mental health disorders or assessed levels of stigma. This study hopes to provide an empirical contribution by casting a broader net and assessing the general public understanding of “mental health” in general.
Combining the quantitative machine learning technique of topic modeling with qualitative interpretation, the research questions put forth in this study are as follows:
What individual frames do Twitter users assign to mental health?
Which mental health frames carry the most salience?
What attributes do mental health frames have with regard to stigma and emotions?
Theoretical framework
Individual frames
Individual frames are cognitive clusters of concepts that guide individuals’ information processing (Entman, Citation1993) that entail a person encountering a phenomenon, perceiving the phenomenon by selecting the aspects which are subjectively more salient, and communicating about the phenomenon “as to promote a particular problem definition, causal interpretation, moral evaluation, and/or treatment recommendation” (Entman, Citation1993, p. 53). Individual frames do not necessitate research on an individual subject level and can be analyzed from a group perspective. Hence, the term individual frame sometimes is used interchangeably with audience frame. However, in the age of social media, the individual frame is a better term to use than the audience frame as it also implies the production of meanings.
Furthermore, individual frames can be differentiated into unique and consistent frames (Borah, Citation2011). Unique frames are frames that help to analyze the understanding of particular topics or issues. Consistent frames, on the other hand, are frames that are consistent across different topics. The focus of this study is to uncover the unique frames of mental health discourse in the broad sense. However, these unique frames might be consistent frames across discourses on different mental health disorders. For example, research has shown that stigma-related frames are common in discussing certain mental health disorders (e.g., schizophrenia, bipolar disorder) (Budenz et al., Citation2019; Robinson et al., Citation2019).
From a different perspective, consistent frames have an advantage over unique frames in understanding framing effects, e.g., gain and loss goal, risky choice, attribute frames (Levin et al., Citation2002). In the context of social movements, consistent frames – such as diagnostic, prognostic, and motivational frames – are useful to research the evolution of collective action process (Benford & Snow, Citation2000). Considering the advantages of using consistent frames, however, a thorough (descriptive) analysis of unique frames is a necessary step prior to analyzing consistent frames. This is particularly the case in areas where little is known about public conception of a phenomenon (Borah, Citation2011).
Frame attributes
Frames manifest themselves through framing devices – various text attributes such as keywords, stereotypical portraits, stock phrases, or other thematically reinforced clusters of facts or judgments (Entman, Citation1993). Reasoning devices (e.g., causes and consequences), metaphors and historical examples are other possible components. Yet, the number of framing devices is not instrumental for recognizing a frame; even the presence of a single framing device – say, a catch-phrase – can often be sufficient (Gamson & Modigliani, Citation1994).
From the analysis of traditional media, we know that discussions of mental health that use descriptors of criminality, danger and violence, use popular derogatory terms (‘crazy’, ‘psycho’), negative emotional states (‘disturbed’, ‘confused’), disability (‘disabled’, ‘demented’), alienation and victimization vocabulary (‘hopeless victim’, ‘lonely’, ‘strange’), and certain psychiatric diagnoses (‘schizophrenic’) of mental illness, point to stigmatized framing of mental health (Rose et al., Citation2007; Thornicroft et al., Citation2013). On the other hand, discourse about the causes of mental illness (e.g., socio-economic, genetic, psychosocial), treatment solutions, sympathetic portrayals (e.g., positive role-models and public figures suffering from mental illness) and mental health awareness (e.g., anti-stigma advocacy, prevalence discussion, problematizing injustice and lack of funding/services) points to de-stigmatizing mental health frames (Whitley & Berry, Citation2013).
Beyond the stigma and anti-stigma mental health frames, there are other attributes that are important. For instance, Kenez et al. (Citation2015) found that newspapers’ coverage of mental health has so far concentrated on illness rather than wellbeing. Paterson (Citation2007) described some more granular subframes that can influence day-to-day lives, public opinions, and policy in a more nuanced way. For instance, the portrayals of violence which feature mental health, were often framed as a community care tragedy, where there was insufficient support and care on account of the perpetrator. When previously such acts of violence have been considered accidental, the community care tragedy framing has created opportunities for change in social policy and might have played a role in the reduction of stigma.
Additionally, we know that frames can elicit emotions and that emotions can help to understand frames (Gross, Citation2008). Not only could we derive whether certain frames have positive or negative connotations, but the intensity of the emotions could also indicate the likelihood of civic engagement (Major, Citation2018; Van Zomeren et al., Citation2004). More intense emotions, whether those are negative (e.g., anger) or positive (e.g., pride), are known to be more effective for triggering an action than less intense emotions (e.g., worry, content). With interest in promoting a positive change, knowing the sentiment of mental health frames will help identify what frames are more problematic and what frames are more hopeful.
Frame salience
When an individual encounters a ‘mental health’ phrase, they will perceive and describe it in different ways in accordance with their own mental categorizations. Sometimes, certain subjective categorizations are similar for many people. Especially during times of the traditional and social media convergence, when there are no longer clear distinctions in frame setting agency (Moon & Hadley, Citation2014), individual discourse plays a greater role in identifying the problems of concerns (i.e. diagnostic framing), articulating solutions (i.e. prognostic framing), and motivating the public to action or the change in behavior (i.e. motivational framing) (Benford & Snow, Citation2000). The more available, consistent, and used by the broader audiences are these common ways of thinking – individual frames – the more salient they can be considered. Once such salient frames are established, these will have an impact on how the messages from other sources (e.g., mental health advocates, media) are employed by the broader audiences (Scheufele & Tewksbury, Citation2007) – reinforcing the positive effect if the frames align, and countering the change when the messages differ (Shen & Edwards, Citation2005).
To exemplify, Mavandadi et al. (Citation2018) found that framing mental health care in terms of benefits, instead of risks, positively affected the participants’ healthcare engagement. Contrary to other health-related engagements (e.g., cancer screening), the authors suggest that the reasons for such outcomes might stem from the fact that mental health assessments are not considered “risky” or important, seen as a more preventative or recuperative task. Alternatively, Major (Citation2018) found that, contrary to the belief that focusing on an individual in a news story would increase compassion, focusing on an individual with depression resulted in blame, showing that depression might be trivialized and is subject to the fundamental attribution error. In considering these outcomes, the importance of understanding how the general public frames mental health becomes apparent as to provide more targeted care, facilitate positive change, and do no harm.
Data and methods
Data collection
Twitter data was used to discover individual mental health frames. First, Twitter is one of the biggest social media platforms that allows people to engage in open conversations with others. Second, unlike Facebook, Twitter allows researchers to download tweets produced by virtually any user (Bail, Citation2012). Although Twitter’s data is publicly available and no ethical approval was necessary to conduct this study, to protect the users from any unwanted attention, we anonymized all the tweets and private Twitter accounts mentioned in this research.
Using search terms “mental health” and #mentalhealth, we downloaded 10 years of publicly available English language tweets (2007–2017) through the Jefferson-Henrique Python script – widely used in social media research (Birnbaum et al., Citation2017; Su & Borah, Citation2019). We took a sample of time-series data with tweets gathered on one single day (24 hours) in the middle of the month/middle of the week for each month, collecting 695,414 posts by 339,493 unique users. Besides tweets’ text, the data also included date stamp, username, number of replies and favorites. To make sure that frames do indeed represent the general public discourse rather than an elite competition (Druckman, Citation2004), we also collected a 1-year sample (2015, N = 4815) of tweets’ users’ number of followers, denoted as user social capital. The text data were cleaned in Python by stemming and lowercasing, and removing stopwords (e.g., and, or, that), corpus specific words included in > 80% of the text (e.g., mental health), and rare words that appeared in less than 200 documents.
We also calculated echo-chamber to control if certain frames are not restricted to a certain closed network (Kretschmer et al., Citation2002). Echo chamber here is operationalized via inverse Krackhardt’s E/I Ratio:
where EL represents the number of edges that are external to a given topic per period (quarter) and IL is the number of edges internal to or between vertexes within that topic. This measure varies on a scale from −1 to 1 with −1 representing a perfectly open community and +1 representing an absolute echo chamber (Krackhardt & Stern, Citation1988). Internal Edges are calculated as a total number of users minus a unique number of users per topic per period while the External Edges are calculated by a difference of a total number and a unique number of users of all topics per quarter minus Internal Edges of the topic.
Textual sentiment
Sentiment characteristics of tweets were derived using LIWC – Linguistic Inquiry and Word Count software (Pennebaker et al., Citation2015). LIWC is a dictionary-based, automated text analysis program which counts proportions of language cues associated with texts’ sentiment characteristics. We focused specifically on the emotionality of tweets such as display of positive or negative emotions, sentiment (positive minus negative emotions), and emotional intensity calculated as the square root of the sum of squares of positive and negative emotions proportions, see Lee and Nerghes (Citation2017).
We also used LIWC to create custom dictionaries to quantify stigma-related vocabularies that were defined by an average of LIWC in-house dictionaries of Risk (“danger”, “beware”, “fear”), Power (related to victimization, submission or domination such as “criminal”, “pitiful”, “hopeless”, “victim”) and Swear (i.e. such as “moron”, “idiot”, “psycho”) words, and a custom LIWC dictionary created from inappropriate words or phrases used to describe mental illness as identified by Rose et al. (Citation2007) (i.e. “crazy”, “dumb”, “freak”). To avoid bias, we have excluded words which can also be understood in a non-stigmatizing way depending on the context (i.e. “ill”, “schizophrenia”, “mental illness”) or implied polysemy (i.e. the word “challenged” can be used in a stigmatizing way but also in a context of an opinion being challenged) (see Supplementary Info for Operationalization and tweet examples).
Topic modeling
We used topic modeling – an inductive quantitative machine learning technique – to discover discursive frames. Entman (Citation1993) argued that words that tend to occur together in texts are clusters of meanings that point at frames. Although framing analysis has usually been done qualitatively on smaller datasets, there are examples of research which used quantitative methods (e.g., Crawley (Citation2007) relied on the keyword frequencies and factor analysis, Matthes and Kohring (Citation2008) – hierarchical cluster analysis, Murphy and Maynard (Citation2000) – a semantic network analysis combined with cluster analysis). The Latent Dirichlet Allocation (LDA) topic modeling technique mimics these analyses by exploring latent structures in texts by clustering words that “occur in documents together more frequently than one would expect by chance” (DiMaggio et al., Citation2013, p. 578).
Additionally, LDA is easily applied to big data and helps identify dominant and subservient frames and see how these develop over time. Namely, we look at the prominence of the frames within the discourse by counting the number of documents in which a specific frame occurs. A document (here: tweet) is attributed to a specific topic (here: frame) if topic representation exceeds a cut off value of 20% as a proportion of the document (see Supplementary Info for reasons behind 20% cutoff point).
To run LDA analyses, the number of topics was set to 30, based on 20 top words (see Supplementary Info for the optimal number of topics algorithm). Considering that a tweet has a maximum length of 140 characters (changed to 280 since 2017) two assumptions can be made: (1) the author should be concise; therefore, many topic-relevant words would be expected to appear in a short text of a tweet, (2) removing the stopwords and the like will shorten the number of words within tweet.
Since a 30 topics solution, although methodologically optimal, is very extensive, we clustered the topics into greater themes for better contextual comprehension. We did so by performing an LDA analysis in stages with a decreasing number of topics: 30, 10, 7 and 5-topics solutions (see Supplementary Info for all solutions). Whereas the 5-topics solution proved too general – clustering themes that appear to be distinct, the 10-topics solution created less meaningful categories. We settled at the 7-topics solution which provided the most logical solution. By qualitative matching of keywords from the 30-topics solution (subframes) to 7-topics solution (frames), we were better able to understand the general discourse and contextual meanings of the frames.
Statistical analyses
We also assessed trend patterns of discourse characteristics and frames by using Mann-Kendall trend test (McLeod, Citation2011). Secondly, we looked at the between-group differences in frames sentiment as compared to the overall mental health discourse by comparing means with One Sample t-test (x, mu = overall discourse means) by using R t.test function. For statistical analyses all variables are mean-aggregated per topic per quarter (N = 1320, t = 44) which takes care of high variability due to outliers.
Results
The following 7 frames based on 30 subframes have emerged via topic modeling analysis: Awareness (14.7%), Feelings and Problematization (10.8%), Classification (10.4%), Accessibility and Funding (5.6%), Stigma (3.2%), Services (2.0%), Youth (1.9%). These frames represent 48.7% of the mental health discourse on Twitter. The remaining 51.3% of discourse consists of topics which are more fragmented (representing <0.5% of discourse); hence, they could not be meaningfully identified by an LDA model. The topics were labeled according to their keywords and qualitative assessment looking at the tweets they represented (for tweet examples see Supplemental Info).
We can infer that the general public on Twitter understands mental health in terms of ‘Awareness’ (14.7% – ). To Twitter users, the ‘Awareness’ frame does not only mean social media, blogging and posting, but also creating community by organizing events and other group activities (2.97%), thinking about education, research and providing support within the educational system (2.93%), and addressing stigma by initiating conversations, providing support and campaigning (2.51%). Interestingly, #BellLetsTalk – a Canadian campaign to fight mental health stigma and support youth mental health and wellness services featured prominently in the ‘Awareness’ frame, with a great uptake from the public reusing the hashtag. Charity work and fundraising to support mental health initiatives are also featured in the ‘Awareness’ subframes (1.11% and 0.75% respectively). A very distinct mental health awareness subframe that deserves attention is ‘Mental health at work’, focusing on work conditions, benefits, along with digital technology (i.e. apps, wristbands) to aid mental health awareness (0.8%). The ‘Awareness’ frame is motivational, encouraging Twitter users to support relevant causes, donate, or help with spreading awareness about mental health.
Table 1. LDA-derived MH frames (N = 695,414, T = 7, t = 30)
The ‘Awareness’ frame carries significantly lower levels of stigmatizing vocabulary (p < .001); it has significantly lower levels of negative emotions (p < .01) and emotional intensity (p < .01) than general mental health discourse. ‘Awareness’ engages diverse publics as can be derived from a significantly lower echo-chamber (p < .05). ‘Awareness’ is stable (M-K p > .05), dominant (average topics per tweet = 1.28 vs. 1.57 for the overall discourse), and shows a higher level of engagement in terms of replies (p < .05) ().
Table 2. Frames’ one sample t-test and Mann-Kendall trend test (N = 1320)
The second most prominent frame is ‘Feelings and Problematization’, representing 10.8% of the discourse (). People often use the phrase ‘mental health’ when they want to problematize mental health and their own illness, and express their feelings and anxieties, especially in the context of everyday life (“Thinking about a mental health day. My brain is oatmeal lately”). It is mostly about oneself, externalizing one’s personal problems. In line with (Robinson et al., Citation2019), mental health here appears to be trivialized (“Life sucks now. I have got to do something fun. Anybody want to donate a weekend trip to Vegas for my mental health?”). Additionally, pop-culture influences may contribute to such trivialization. For example, a tweet “See you messed up my mental health.I was quite unwell … . #LilyAllenFeelings” takes its inspiration from a popular song. In general, however, this frame also helps to identify some factors associated with psychosocial symptoms such as busy work, school, relationship problems, and even suggests some solutions (e.g., taking a break from work or study, encouraging exercise).
The ‘Feelings and Problematization’ frame uses significantly more stigmatizing vocabulary (p < .01), it has significantly higher positive and negative emotions (p < .001), and is more emotionally intense (p < .001). Yet, negative emotions override positive emotions – the sentiment of this frame is significantly lower (p < .001). The frame engages the public broadly – significantly lower echo-chamber (p < .001) – and is initiated by the users with significantly lower average social capital (p < .05). This frame has seen a significant upward trend (p < .001) and is very dominant (average topics per tweet = 1.04 vs. 1.57 for the overall discourse) ().
The ‘Classification’ frame (10.4% – ) is labeled ‘Classification’ because it speaks about distinctive disorders, similar to DSM-5 mental illness categories, such as Anxiety, Depression, Bipolar Disorder (BD), Post Traumatic Stress Disorder (PTSD) as well as physical symptoms (stress, sleep disorders) and mental health related presentations such as substance abuse or suicidality. Additionally, the ‘Classification’ frame frames certain disorders. For instance, PTSD is often referred to in the context of gender (male) and occupation (military and veterans) and is linked to substance abuse and suicide. ADHD and autism are mainly spoken about in relation to children/young adults. Substance abuse in relation to mental health is often talked about with regards to drugs more so than alcohol or tobacco, and more often refers to women, teenagers and people who are impoverished.
With regards to diagnostic categories, mental health mainly revolves around ‘culture-bound syndromes’ (i.e. depression, often followed by a hashtag #depression, #stress) and popularized mental health disorders (i.e. anxiety, depression, ADHD, Autism, bipolar) (Dowrick, Citation2013). These disorders often receive the most critique in respect to the difficulty of classification and diagnosis within the DSM-5 (American Psychiatric Association, Citation2013) and “no sound evidence for a discrete pathophysiological basis” (Cohen, Citation2016; Dowrick, Citation2013, p. 229). Furthermore, physiological disorders such as eating disorders, sleeping disorders or learning disabilities are frequently used within the discourse, reflecting Rose et al. (Citation2007) stigma-inducing association of physical illness with mental disability. Mental health within the ‘Classification’ frame also seems to shy away from overly stigmatized or severe mental illness (i.e. schizophrenia), neurocognitive diseases (i.e. Alzheimer’s and Parkinson’s disease) and neurological disorders (i.e. epilepsy). Hence, we observe that these disorders are rarely popularly contextualized in relation to mental health. To a great extent the ‘Classification’ frame also features a positively oriented healthy lifestyle, self-care, mindfulness and well-being. Although the positive framing is encouraging, along with the more evidence-based observations (e.g., Interesting article about exercise and anxiety linked from the Mental Health Awareness report ‘Living with Anxiety’<link>”) such framing also features contestable health claims (e.g., “Coconut oil can maintain mental health”).
The emotionality of the ‘Classification’ frame does not significantly differ from the emotionality of the overall mental health discourse and the frame does not show significant trends ().
The frame ‘Accessibility and Funding’ (5.6% – ) problematizes the accessibility of mental health services – particularly the treatment coverage and insurance, especially for such populations as veterans, or people suffering from addictions or substance abuse. Government funding cuts are criticized, and the state of mental health services is often accompanied by the word ‘crisis’. People call for budget reform and more government support. Especially prominent is this frame in England, in relation to NHS.
Related services (police, prisons) are discussed in terms of the mental health approach, where the necessity of training and the problem of underfunding are brought to attention. We have also noticed that the ‘Accessibility and Funding’ frame uses mental health (services) in political discourse as exemplified by the following tweets: #HillaryClinton on issues: more mental health & drug treatment, no more mass incarceration & private prisons.” <link> or 1,876 #Mentalhealth Beds have been axed since 2010 in English #NHS whereas numbers patients being detained have increased 6% #CameronMustGo.
‘Accessibility and Funding’ uses significantly less stigmatizing vocabulary (p < .01) than the overall discourse and displays significantly lower negative emotions (p < .001), which drives the sentiment up (p < .01) and the intensity down (p < .001). The ‘Accessibility’ frame has seen a significant 5- (p < .001) and 10-year (p < .01) decline and engages fewer people in terms of replies (p < .01) ().
As expected, mental health discourse is often framed in association with ‘Stigma’ (). The ‘Stigma’ frame is comparable to its representation in traditional media discourse (Thornicroft et al., Citation2013; Whitley & Wang, Citation2016) and revolves around risk, danger and violence (topics of ‘Guns and Shooting’ and ‘Death and Murder’) and the connection of mental illness to madness and insanity as portrayed by its contextual use within the ‘Donald Trump’ subframe. Luckily, the ‘Stigma’ framing of mental health enjoys lower prominence than more positive frames, representing just 3.2% of the discourse. Yet, we can also see that the frame is more often used in conjunction with other topics (average topics per tweet = 3.26) and uses significantly lower levels of stigmatizing vocabulary (p < .05), meaning that the frame has high latency and is difficult to catch. In other words, stigma does not necessarily use more pejoratives, low power words or risk words than average mental health discourse, but rather frames mental health by associating it with an undesirable context such as mass shootings, murders or public figures’ incompetence, often inside other topics. The ‘Stigma’ frame also politicizes mental health, using mental health to advance party politics, for example: He was mentally ill w/a gun. Let’s look @ mental health care & gun regulations #virginiashooting or “Congress to hold a hearing on a mental health bill” Obama’s Narcissistic Personality Disorder?
We note that the ‘Stigma’ frame is significantly more emotionally intense (p < .01). Interestingly, it has significantly higher positive (p < .001) and significantly lower negative (p < .001) emotions, hence higher sentiment (p < .001). We can see an increase in the use of the frame in the last 3 (p < .01) to 5 years (p < .05), though it is significantly less engaged ().
Considering high positive emotionality and emotional intensity, looking at the ‘Stigma’ frame tweets in-depth, many examples emerged which indicate that the positivity of stigma-related mental health discourse could emerge from the use of sarcasm. For example, “Murderer of […] teenager says escape from mental health unit was ‘best three month of his life’” Additionally, the frame is prone to sensationalism, especially from the traditional media outlets using Twitter, for example, [Mental health resource/blog] #Nepal: mentally ill man freed after 20 years of chains. #humanrights #mentalhealth #recovery or [CBS News] A violent psychopath who escaped from a Hawaii mental health hospital is behind bars after 72 hours on the run. Officials say the “psychopathic predator” used wads of cash, plane rides and an alias to evade police. <link>
The frame ‘Services’ (2.0%) is usually used by mental health workers to find jobs and for mental health services to post jobs (). It also problematizes mental health employment and structural issues experienced by mental health workers (psychiatric nurses, psychologists) such as underfunding and understaffing, and challenges mental health workers and psychiatric professions. A small but not less important part of the ‘Services’ frame is the criticism of mental health workers by service users.
This frame shows a high echo-chamber (p < .001), which means it is discussed in narrow circles. Additionally, the frame is engaged by users with significantly lower social capital (p < .01). The frame uses significantly more stigmatizing language (p < .01) and has a low positive (p < .001) and high negative (p < .001) emotions which result in a very negative sentiment (p < .001). This frame is often used in conjunction with other topics (average topics per tweet = 2.29), but it has been in decline in the last 3 (p < .01) to 5 (p < .001) years ().
The majority of the stigma comes from the high level of usage of words ‘risk’ (i.e. youth risk coordinator, risk management etc.) and ‘victim’ (i.e. ‘suicide victim’, ‘domestic abuse victim’ etc.); there are also many instances of mental health service users criticizing mental health workers: “THE MENTAL HEALTH SYSTEM AT […] HOSPITAL FUCKING SUCKS. Honestly who hires these people” or “Can’t bloody trust anyone not even your mental health nurse?! Xx”.
‘Youth’ is another less prominent but still salient mental health frame (1.9%) and revolved around mental issues related to life-stages (parenting, child development, adolescents) (). It features discourse about children with learning support needs, the parenting process, challenges related to both child and parents’ mental health (especially women/mothers), and discourses about youth at risk and child abuse.
The frame has significantly lower than average sentiment (p < .001) – lower positive (p < .001) and higher negative emotions (p < .001). The frame is used in conjunction with other topics (average topics per tweet = 2.37). In the course of 10 years the youth frame showed a positive trend (p < .01) ().
Discussion
Empirical findings
The main goal of this study was to infer how mental health is understood and used by the general public on Twitter. Our findings suggest that, as opposed to a more problematic framing of mental health by the traditional media channels, the online environment is tilted toward mental health awareness, while mental health stigma is mostly related to trivialization and self-stigma, rather than danger and violence similar to findings for the specific mental health disorders – see Budenz et al. (Citation2019), Reavley and Pilkington (Citation2014). We identified that ‘Awareness’ framing is engaged by a broader public than ‘Stigma’, and that when ‘Stigma’ framing does relate to violence and danger, it tends to do so in a politicized discourse (gun laws, justifications for funding, etc.).
As compared to traditional media, frames related to problematization, service, and accessibility and funding are similar to ‘feature’ and ‘social policy’ stories described by Paterson (Citation2007). Conversely, the online discourse did not feature stories related to foreigners, depicted less legal cases of violence and homicide, and did not identify salient frames related to medication, trauma, or tragedy. It also featured fewer stories depicting real people in 3rd person, with most of the content discussed being either related to self, or to mental health or illness in general.
There were also some novel frames and frame attributes that appeared online. These are the ‘Youth’ frame, the work attribute within the ‘Problematization’ frame, ‘Classification’ subframes such as the PTSD/Veterans subframe, ADHD and Youth subframe, and Substance Abuse subframe which featured teens, women and people in poverty. The aforementioned frames warrant further research as these might be helpful to understand the sociology of certain issues (e.g., antecedents, implicit prejudice).
Where some frames showed detail, other frames were characterized by vagueness. For instance, the ‘Awareness’ frame often showed awareness for the awareness sake, and lacked more depth. To elaborate, in addition to raising awareness about mental health or mental health disorders, it could be helpful to raise awareness that long working hours negatively affect mental health, or that going to the doctor and engaging in psychotherapy can help to manage anxiety. As per Benford and Snow (Citation2000), clearly identifying the range of causes and potential solutions can help motivate an action where the feasibility of positive outcomes would be more plausible.
This, of course, is not to say that there were no positive examples. We found that some advocacy efforts were very successful. Canadian #Bellletstalk campaign, for example, could warrant a case study to gain a better understanding of what makes a good mental health awareness campaign. From our own perspective, we noted that the ‘Awareness’ frame in general was very low in emotions. Our note to public health campaigners would be to check whether their communication could benefit from a more emotional language. A recent paper by Pavlova and Berkers (Citation2020) notes that online communications characterized by higher emotional energy (i.e. high level of engagement with confidence and solidarity) tend to drive the more desirable mental health discourse.
From a more cautionary perspective, we found that the phrase ‘mental health’ is often used to express one’s feelings and state, externalizing one’s problems. While this way of thinking and communicating about mental health might be a helpful tool to deal with personal anxieties, we need more research to identify if this type of discourse causes more harm than good by (1) problematizing mental health further, (2) trivializing mental health, (3) worsening mental health symptoms by delaying help-seeking. Considering that the ‘Feelings and Problematization’ frame is broadly used by ordinary users (low social capital), uses a lot of stigmatizing vocabulary, and is highly emotional – it warrants further investigation. Especially because in recent years this way of talking about mental health became more prominent. It is also worth noting that some everyday life concerns are used in a diagnostic manner, implying that mental health is often affected by being overworked, high educational demands, and relationship problems that warrant taking time off and more focus on self-care. More research is needed to analyze what socio-demographic and psychosocial factors cause people’s distress in order to tackle them.
From the ‘Classification’ frame we have learned that Twitter discourse is still mostly comprised of broader, often popularized mental health categories (i.e. depression and anxiety) which only make up a limited proportion of mental health disorders as compared to possible DSM-V diagnoses. For instance, neurocognitive diseases such as Alzheimer’s or Parkinson’s are rarely mentioned in discussion of mental illness. Additionally, mental health is often framed from the perspective of physical condition, which may cause stigma (Rose et al., Citation2007). Although mental health is still mostly framed from the perspective of mental illness, a positive observation comes from mental health also being framed from the perspective of health and wellbeing. However, future research is needed to assess to what extent the information in the wellbeing-related ‘Classification’ frame is well-informed and whether it features good advice or is prone to inaccurate information and the perpetuation of myths, as can be seen in other health-related topics prone to misinformation (e.g., vaccination).
We also note that in a Twitter discourse of mental wellbeing, caring for one’s mental health tend to relay all the responsibility to individuals (e.g., exercising, buying supplements, doing mindfulness) rather than providing and motivating solutions which imply external professional help (e.g., going for a mental health assessment, engaging in CBT). A positive observation that counteracts such individual responsibility came from such frames as ‘Accessibility and Funding’ and ‘Youth’ where very particular topics are being addressed and issues related to the environment rather than to individuals are highlighted (e.g., lack of funding, need for improved education). These frames are said to have a de-stigmatizing influence on mental health discourse (Stuart, Citation2006; Whitley & Berry, Citation2013), whereas the frame ‘Services’ might explain the reasons behind the privatization of mental health (e.g., understaffing, lack of service continuity).
While frames like ‘Services’ might diagnose some broader societal problems that cause the deterioration of public mental health, we also note that such frames – along with frames ‘Stigma’ and ‘Accessibility and Funding’ – are often used in a political context which may imply that ‘mental health’ is a master frame often used to justify or explain other societal problems (e.g., violence, mass shooting) and motivate other, often political, actions (e.g., maintain legislation, engage in party politics).
Remarkably, ‘Stigma’ is framed in a highly emotional way, using a lot of positive emotionality which contributes to Whitley and Wang (Citation2016) results indicating that mental health related articles with a positive tone have gained more attention in recent years. However, on social media as opposed to traditional media which [largely] adheres to professional standards, this positivity hides sarcasm. To second that, the ‘Stigma’ frame has a significantly lower stigmatizing vocabulary which highlights the need for the combination of qualitative and quantitative research methods as the stigma construct is characterized by high latency and is often contextual and implicit. The ‘Stigma’ frame is dangerous as it spreads across the discourse and is often used in conjunction with other frames. For instance, the subset of the ‘Service’ frame has identified highly stigmatizing vocabulary and emotional negativity. Upon a closer look we found that these usually stem from the service users trying to express their frustrations about the service providers, constituting an echo-chamber. With this finding, we note that frame ‘Service’ could be useful for future research of service users’ perceptions and analyzing the needs of the service uses that have not been satisfied.
Implications for framing theory
This research of the unique frames used in public discourse of mental health also marks several contributions to framing theory. First, we posit that unique frames applied to mental health in general may be consistent frames across different categories of mental health. We touched upon stigma as a likely consistent frame. Yet, future comparative research should examine which of the other unique frames we found could be consistent over more narrow mental health categories. For example, we do not know whether ‘Awareness’ frame will manifest similarly for wellbeing as compared to mental illness, and whether there are differences in ‘Awareness’ framing for different mental health disorders. Additionally, there is a possibility to consistently use the unique frames discovered in this research by applying trend analyses, as we have done in this paper, or using dynamic topic modeling to see how certain frames develop over time (i.e. will ‘Service’ frame have the same meaning in 10 years from now? Will ‘Classification’ frame still consist the same diagnostic categories?).
Second, we have noticed that for social issues that are not clearly defined, it might be difficult to create social change because the prognostic and motivational features are not specific enough to be actioned (e.g., raise mental health awareness, fight stigma, increase funding). Perhaps more speculatively, we noticed that mental health became a salient attribute in other frames (i.e. a master frame), which over time might have resulted in a static definition that is difficult to change (Lawrence & Birkland, Citation2004), and which may impede diagnostic processes and cause prognostic and motivational aspects to be vague and difficult to action (Benford & Snow, Citation2000). Ultimately, of course, the question of whether mental health being an attribute in other frames may hinder the diagnostic frames and positive mental discourse development is an empirical one.
Third, we have observed that framing differs per different medium. Because discursive power can be exercised by anyone who can successfully create and manage the flow of information that agrees with their goals (Papacharissi, Citation2015), for future research it is important to understand the changes in power positions and how this influences overall discourse. When traditional media embedded some norms and practices that reflected in the discourse (e.g., journalism code of ethics, using sensational stories to increase readership), social media discourse might reflect other dominant norms and values; some of those could be of benefit (e.g., inclusivity, different voices), while others bring out attributes causing more negative framing effects (e.g., commercial interests, unverified information sources). Moreover, to find out if the frames identified within this research would be sustainable and successful in creating positive impact it is imperative to understand whether these will align with the beliefs of the people who would benefit from the improvements in the area of mental health communications the most (Benford & Snow, Citation2000). There are, therefore, some important avenues for future research in identifying the main framing agents in this highly networked environment and studying whether these agents represent the targets well.
Other limitations and avenues for future research
As the main limitation of this research, we see the reliance on the single platform of Twitter, with the focus on English tweets. Although Twitter is considered to be a good representation of public opinion, Twitter demographics represent a younger, more educated and wealthier audience (Wojcik & Hughes, Citation2019, April, p. 24). Moreover, not everyone has access to Twitter or chooses to use Twitter, which questions good representation. Future research may look at other social media platforms or forums as well as at the framing of mental health offline (i.e. conversation analysis). Topic modeling of traditional media mental health discourse (online and offline) could provide a useful comparison. Lastly, looking at the framing of mental health it is important to consider how it compares to the framing of mental illness. Further research is needed to address this gap.
Supplemental Material
Download MS Word (1.3 MB)Acknowledgments
We thank the two anonymous reviewers whose comments/suggestions helped improve and clarify this manuscript.
Supplementary material
Supplemental data for this article can be accessed on the publisher’s website.
References
- American Psychiatric Association. (2013). Diagnostic and statistical manual of mental disorders (5th ed.). American Psychiatric Publishing.
- Bail, C. A. (2012). The fringe effect: Civil society organizations and the evolution of media discourse about Islam since the September 11th attacks. American Sociological Review, 77(6), 855–879. https://doi.org/https://doi.org/10.1177/0003122412465743
- Benford, R. D., & Snow, D. A. (2000). Framing processes and social movements: An overview and assessment. Annual Review of Sociology, 26(1), 611–639. https://doi.org/https://doi.org/10.1146/annurev.soc.26.1.611
- Birnbaum, M. L., Ernala, S. K., Rizvi, A. F., De Choudhury, M., & Kane, J. M. (2017). A collaborative approach to identifying social media markers of schizophrenia by employing machine learning and clinical appraisals. Journal of Medical Internet Research, 19(8), e289. https://doi.org/https://doi.org/10.2196/jmir.7956
- Borah, P. (2011). Conceptual issues in framing theory: A systematic examination of a decade’s literature. Journal of Communication, 61(2), 246–263. https://doi.org/https://doi.org/10.1111/j.1460-2466.2011.01539.x
- Budenz, A., Klassen, A., Purtle, J., Yom Tov, E., Yudell, M., & Massey, P. (2019). Mental illness and bipolar disorder on Twitter: Implications for stigma and social support. Journal of Mental Health, 29(2), 1–9. https://doi.org/https://doi.org/10.1080/09638237.2019.1677878
- Chisholm, D., Sweeny, K., Sheehan, P., Rasmussen, B., Smit, F., Cuijpers, P., & Saxena, S. (2016). Scaling-up treatment of depression and anxiety: A global return on investment analysis. The Lancet Psychiatry, 3(5), 415–424. https://doi.org/https://doi.org/10.1016/S2215-0366(16)30024-4
- Cohen, B. M. (2016). Psychiatric hegemony: Mental illness in neoliberal society. Springer.
- Crawley, C. E. (2007). Localized debates of agricultural biotechnology in community newspapers: A quantitative content analysis of media frames and sources. Science Communication, 28(3), 314–346. https://doi.org/https://doi.org/10.1177/1075547006298253
- DiMaggio, P., Nag, M., & Blei, D. (2013). Exploiting affinities between topic modeling and the sociological perspective on culture: Application to newspaper coverage of U.S. government arts funding. Poetics, 41(6), 570–606. https://doi.org/https://doi.org/10.1016/j.poetic.2013.08.004
- Dowrick, C. (2013). Depression as a culture-bound syndrome: Implications for primary care. British Journal of General Practice, 63(610), 229–230. https://doi.org/https://doi.org/10.3399/bjgp13X665189
- Druckman, J. N. (2004). Political preference formation: Competition, deliberation, and the (ir)relevance of faming effects. American Political Science Review, 98(4), 671–686. https://doi.org/https://doi.org/10.1017/S0003055404041413
- Entman, R. M. (1993). Framing: Toward clarification of a fractured paradigm. Journal of Communication, 43(4), 51–58. https://doi.org/https://doi.org/10.1111/j.1460-2466.1993.tb01304.x
- Galderisi, S., Heinz, A., Kastrup, M., Beezhold, J., & Sartorius, N. (2015). Toward a new definition of mental health. World Psychiatry, 14(2), 231–233. https://doi.org/https://doi.org/10.1002/wps.20231
- Gamson, W. A., & Modigliani, A. (1994). The changing culture of affirmative action. In P. Burstein (Ed.), Equal employment opportunity: Labor market discrimination and public policy (Vol. 3, pp. 373–394). Aldine de Gruyter.
- Gross, K. (2008). Framing persuasive appeals: Episodic and thematic framing, emotional response, and policy opinion. Political Psychology, 29(2), 169–192. https://doi.org/https://doi.org/10.1111/j.1467-9221.2008.00622.x
- Henderson, C., Evans-Lacko, S., & Thornicroft, G. (2013). Mental illness stigma, help seeking, and public health programs. American Journal of Public Health, 103(5), 777–780. https://doi.org/https://doi.org/10.2105/AJPH.2012.301056
- Jackson, S. J., & Foucault Welles, B. (2016). #Ferguson is everywhere: Initiators in emerging counterpublic networks. Information, Communication & Society, 19(3), 397–418. https://doi.org/https://doi.org/10.1080/1369118X.2015.1106571
- Joseph, A. J., Tandon, N., Yang, L. H., Duckworth, K., Torous, J., Seidman, L. J., & Keshavan, M. S. (2015). #Schizophrenia: Use and misuse on Twitter. Schizophrenia Research, 165(2), 111–115. https://doi.org/https://doi.org/10.1016/j.schres.2015.04.009
- Kenez, S., O’Halloran, P., & Liamputtong, P. (2015). The portrayal of mental health in Australian daily newspapers. Australian and New Zealand Journal of Public Health, 39(6), 513–517. https://doi.org/https://doi.org/10.1111/1753-6405.12441
- Krackhardt, D., & Stern, R. N. (1988). Informal networks and organizational crises: An experimental simulation. Social Psychology Quarterly, 51(2), 123–140. https://doi.org/https://doi.org/10.2307/2786835
- Kretschmer, M., Klimis, G., & Choi, C. (2002). Increasing returns and social contagion in cultural industries. British Journal of Management, 10, 61–72. https://doi.org/https://doi.org/10.1111/1467-8551.10.s1.6
- Kühn, S., & Rieger, U. M. (2017). Health is a state of complete physical, mental and social well-being and not merely absence of disease or infirmity. Surgery for Obesity and Related Diseases, 13(5), 887. https://doi.org/https://doi.org/10.1016/j.soard.2017.01.046
- Lawrence, R. G., & Birkland, T. A. (2004). Guns, hollywood, and school safety: Defining the school‐shooting problem across public arenas. Social Science Quarterly, 85(5), 1193–1207. https://doi.org/https://doi.org/10.1111/j.0038-4941.2004.00271.x
- Lee, J.-S., & Nerghes, A. (2017, July 28–30). Labels and sentiment in social media: On the role of perceived agency in online discussions of the refugee crisis [Research article]. Proceedings of the 8th International Conference on Social Media & Society, Toronto, ON, Canada.
- Levin, I. P., Gaeth, G. J., Schreiber, J., & Lauriola, M. (2002). A new look at framing effects: Distribution of effect sizes, individual differences, and independence of types of effects. Organizational Behavior and Human Decision Processes, 88(1), 411–429. https://doi.org/https://doi.org/10.1006/obhd.2001.2983
- Major, L. H. (2018). Mental health news: How frames influence support for policy and civic engagement intentions. Journal of Health Communication, 23(1), 52–60. https://doi.org/https://doi.org/10.1080/10810730.2017.1411994
- Manwell, L. A., Barbic, S. P., Roberts, K., Durisko, Z., Lee, C., Ware, E., & McKenzie, K. (2015). What is mental health? Evidence towards a new definition from a mixed methods multidisciplinary international survey. BMJ Open, 5(6), e007079. https://doi.org/https://doi.org/10.1136/bmjopen-2014-007079
- Matthes, J., & Kohring, M. (2008). The content analysis of media frames: Toward improving reliability and validity. Journal of Communication, 58(2), 258–279. https://doi.org/https://doi.org/10.1111/j.1460-2466.2008.00384.x
- Mavandadi, S., Wright, E., Klaus, J., & Oslin, D. (2018). Message framing and engagement in specialty mental health care. Psychiatric Services, 69(3), 308–314. https://doi.org/https://doi.org/10.1176/appi.ps.201700251
- McDonnell, T. E. (2016). Best laid plans: Cultural entropy and the unraveling of AIDS media campaigns. University of Chicago Press.
- McLeod, A. I. (2011). Kendall: Kendall rank correlation and Mann-Kendall trend test. R package version 2.2. https://CRAN.R-project.org/package=Kendall
- Moon, S. J., & Hadley, P. (2014). Routinizing a new technology in the newsroom: Twitter as a news source in mainstream media. Journal of Broadcasting & Electronic Media, 58(2), 289–305. https://doi.org/https://doi.org/10.1080/08838151.2014.906435
- Murphy, P., & Maynard, M. (2000). Framing the genetic testing issue: Discourse and cultural clashes among policy communities. Science Communication, 22(2), 133–153. https://doi.org/https://doi.org/10.1177/1075547000022002002
- Naslund, J. A., Aschbrenner, K. A., Marsch, L. A., & Bartels, S. J. (2016). The future of mental health care: Peer-to-peer support and social media. Epidemiology and Psychiatric Sciences, 25(2), 113–122. https://doi.org/https://doi.org/10.1017/S2045796015001067
- Papacharissi, Z. (2015). Affective publics: Sentiment, technology, and politics. Oxford University Press.
- Paterson, B. (2007). A discourse analysis of the construction of mental illness in two UK newspapers from 1985-2000. Issues in Mental Health Nursing, 28(10), 1087–1103. https://doi.org/https://doi.org/10.1080/01612840701581206
- Pavlova, A., & Berkers, P. (2020). Mental health discourse and social media: Which mechanisms of cultural power drive discourse on Twitter. Social Science & Medicine, 263(2020), 113250. https://doi.org/https://doi.org/10.1016/j.socscimed.2020.113250
- Pennebaker, J. W., Boyd, R. L., Jordan, K., & Blackburn, K. (2015). The development and psychometric properties of LIWC2015. The University of Texas at Austin.
- Reavley, N., & Pilkington, P. (2014). Use of Twitter to monitor attitudes toward depression and schizophrenia: An exploratory study. PeerJ, 2, e647. https://doi.org/https://doi.org/10.7717/peerj.647
- Robinson, P., Turk, D., Jilka, S., & Cella, M. (2019). Measuring attitudes towards mental health using social media: Investigating stigma and trivialisation. Social Psychiatry and Psychiatric Epidemiology, 54(1), 51–58. https://doi.org/https://doi.org/10.1007/s00127-018-1571-5
- Rose, D., Thornicroft, G., Pinfold, V., & Kassam, A. (2007). 250 labels used to stigmatise people with mental illness. BMC Health Services Research, 7(1), 97. https://doi.org/https://doi.org/10.1186/1472-6963-7-97
- Scheufele, D. A., & Tewksbury, D. (2007). Framing, agenda setting, and priming: The evolution of three media effects models. Journal of Communication, 57(1), 9–20. https://doi.org/https://doi.org/10.1111/j.0021-9916.2007.00326.x
- Shearer, E., & Gottfried, J. (2017, September 7). News use across social media platforms 2017. Pew Research Center. https://www.journalism.org/2017/09/07/news-use-across-social-media-platforms–2017/
- Shen, F., & Edwards, H. H. (2005). Economic individualism, humanitarianism, and welfare reform: A value‐based account of framing effects. Journal of Communication, 55(4), 795–809. https://doi.org/https://doi.org/10.1111/j.1460-2466.2005.tb03023.x
- Stuart, H. (2006). Media portrayal of mental illness and its treatments. CNS Drugs, 20(2), 99–106. https://doi.org/https://doi.org/10.2165/00023210-200620020-00002
- Su, Y., & Borah, P. (2019). Who is the agenda setter? Examining the intermedia agenda-setting effect between Twitter and newspapers. Journal of Information Technology & Politics, 16(3), 236–249. https://doi.org/https://doi.org/10.1080/19331681.2019.1641451
- Thornicroft, A., Goulden, R., Shefer, G., Rhydderch, D., Rose, D., Williams, P., Thornicroft, G., & Henderson, C. (2013). Newspaper coverage of mental illness in England 2008-2011. British Journal of Psychiatry, 202(s55), s64–s69. https://doi.org/https://doi.org/10.1192/bjp.bp.112.112920
- Van Zomeren, M., Spears, R., Fischer, A. H., & Leach, C. W. (2004). Put your money where your mouth is! Explaining collective action tendencies through group-based anger and group efficacy. Journal of Personality and Social Psychology, 87(5), 649–664. https://doi.org/https://doi.org/10.1037/0022-3514.87.5.649
- Westerhof, G. J., & Keyes, C. L. M. (2010). Mental illness and mental health: The two continua model across the lifespan. Journal of Adult Development, 17(2), 110–119. https://doi.org/https://doi.org/10.1007/s10804-009-9082-y
- Whiteford, H. A., Ferrari, A. J., Degenhardt, L., Feigin, V., & Vos, T. (2015). The global burden of mental, neurological and substance use disorders: An analysis from the global burden of disease study 2010. PLoS ONE, 10(2), e0116820. https://doi.org/https://doi.org/10.1371/journal.pone.0116820
- Whitley, R., & Berry, S. (2013). Trends in newspaper coverage of mental illness in Canada: 2005–2010. The Canadian Journal of Psychiatry, 58(2), 107–112. https://doi.org/https://doi.org/10.1177/070674371305800208
- Whitley, R., & Wang, J. (2016). Good news? A longitudinal analysis of newspaper portrayals of mental illness in Canada 2005 to 2015. The Canadian Journal of Psychiatry, 62(4), 278–285. https://doi.org/https://doi.org/10.1177/0706743716675856
- Wojcik, S., & Hughes, A. (2019, April 24). Sizing up Twitter users. Pew Research Center. https://www.pewresearch.org/internet/2019/04/24/sizing-up-twitter-users/