Abstract
Conversational agents (CAs) are often included as virtual coaches in eHealth applications. Tailoring conversations with these coaches to the individual user can increase the effectiveness of the coaching. An improvement for this tailoring process could be to (automatically) tailor the conversation at the topic level. In this article, we describe the design and evaluation of a blueprint topic model for use in the implementation of such topic selection.
First, we constructed a topic model by extracting actions from the literature that a CA as coach could perform. We divided these actions in groups and labeled them with topics. We included literature from the behavioral psychology, relational agents and persuasive technology domains. Second, we evaluated this topic model through an online closed card sort study with health coaching experts.
The constructed topic model contains 30 topics and 115 actions. Overall, the sorting of actions into topics was validated by the 11 experts participating in the card sort. Cards with actions that were sorted incorrectly mostly missed an immediacy indicator in their description (e.g., the difference between “you could plan regular walks” as opposed to “let’s plan a walk”) and/or were based on behavior change techniques that were difficult to translate to a conversation.
The blueprint topic model presented in this article is an important step towards more intelligent virtual coaches. Future research should focus on the implementation of automatic topic selection. Furthermore, tailoring of coaching dialogues with CAs in multiple steps could be further investigated, for example, from the technical or user interaction perspective.
1. Introduction
In recent years, research and development in the area of health behavior change applications has increased (Brinkman, Citation2016). Where initially, there has been a focus on providing health information via websites or telemedicine services, in later years the focus shifted to building interventions that could also actively send notifications and provide the user with insight into their personal situation (e.g., through the use of sensors and tailored feedback). A major challenge for these health behavior change applications is user’s adherence (Nijland, Citation2011; Wangberg et al., Citation2008). Users tend to be engaged with an application at first, but then stop using it or stop following its suggestions after the novelty effect wears off. Potential causes for this lack of adherence are actively being researched. It appears that contributing factors are a lack of direct involvement of a health care professional (no social incentive) and content that does not always fit the user’s personal situation (relevance of content) (Andersson et al., Citation2009; Buimer et al., Citation2017). Two possible solutions investigated to tackle these issues are the use of conversational agents (CAs) and tailoring the application to the user (Op den Akker et al., Citation2014).
As Starr (Citation2008) states: “The coaching process can be considered as a series of conversations between two individuals — the coach and the coachee — for the benefit of the coachee in a way that relates to the coachee’s learning process”. In health behavior change applications, conversational agents (CAs) can take on the role of a coach and can have coaching conversations with the user (the coachee) (Kramer et al., Citation2020). These CAs are “computer systems that imitate natural conversation with human users through images and written or spoken language” (Laranjo et al., Citation2018). Non-agent approaches (such as traditional websites or apps) tend to use a one-way method of communication. The possibility for two-way communication—i.e. interactive dialogues—with conversational agents provides a number of advantages. For example, an agent can ask the user questions about their interests, or elaborate on a topic per user request (Bickmore & Giorgino, Citation2006). While some of these functionalities might also be fulfilled by static text, an agent adds a social element and makes the process more dynamic and interactive. Furthermore, while human coaches may be the preferred way of coaching, CAs are always available, never grow tired of answering (the same) questions and can provide continuous support. These aspects allow for CAs to support users in their daily life by providing support after a diagnosis, but also coaching in preventative care.
1.1. Tailoring coaching conversations with CAs
CAs communicate with users through dialogues, where often the dialogue content is carefully designed by domain experts based on existing interventions (e.g., Callejas et al. (Citation2014)). This process requires translation of intervention content to the dialogue domain. In addition, CAs also need to motivate the user to complete the objective of their interaction (Bickmore et al., Citation2010). From counseling literature, we know that the quality of a working alliance between a counselor and client is a factor in the therapeutic change and adherence (Castonguay et al., Citation2006) and that it has three key aspects, namely goal agreement, task agreement and development of a personal bond. Ideally, CAs would build up such a working alliance with their users. However, this does require dialogues between CAs and users to not only contain coaching content, but social elements as well (Bickmore et al., Citation2005; Schulman & Bickmore, Citation2009). Furthermore, there should be variation between dialogues in terms of content and structure to keep participants engaged (Bickmore et al., Citation2013). Finally, a key aspect that sets dialogues with health coaching CAs apart from, for example, chatbots for customer support is that their conversations continue over multiple interactions (Bickmore et al., Citation2018).
Tailoring has been shown to be effective in digital health applications (Krebs et al., Citation2010; Ryan et al., Citation2019; Wangberg et al., Citation2008) and has been researched in CA applications for things like automatic goal selection, switching topics on the sentence level (Glas & Pelachaud, Citation2018; Smith et al., Citation2011), and—in the case of agents with an embodiment—non-verbal behavior adjustments (Krämer et al., Citation2010). Tailoring can be seen as the adjustment of a communication’s timing, intention, content and representation to the user (Op den Akker et al., Citation2014). Brinkman (Citation2016) poses that such tailoring is a capability that an advanced eHealth system should be able to perform, that is, it should be able “to select the most effective and acceptable treatment for the individual and tailor the treatment protocol to optimize potential conflicting values a person holds, e.g., autonomy versus safety” (Brinkman, Citation2016). When it comes to tailoring of conversations with conversational agents, tailoring can be performed for what is discussed and for how it is discussed (Richards & Caldwell, Citation2017). While both are equally important, in this article we focus on tailoring of what is discussed.
Literature on conversational systems typically tends to distinguish various levels in a conversation, namely: domains, topics (of conversation as a whole and on an utterance level), dialogue acts and utterances (e.g., McTear et al., Citation2016, pp. 161–162). In addition, there are different actions that a coach could take to discuss a topic. For example, an agent that wants to inform a user on why healthy behavior is important could simply provide facts and allow the user to ask questions, but they could also apply a quiz-like format if a user is already quite knowledgeable. While these different actions do not change the main topic of the conversation, they can require a different combination of dialogue acts to be performed. We propose that tailoring a coaching conversation can happen at five levels, namely domain, topic (of conversation), action, dialogue act, and utterance (see ).
Once a domain (e.g., physical activity coaching) has been selected, a discussion topic can be personally decided upon, by using the available information (e.g., the user’s profile, their interaction history, available sensor data, and domain knowledge). Tailoring of topics allows for the CA to take the initiative by suggesting a topic to discuss and can provide users with conversations that are relevant and suitable for their specific situation. Selection of topics can also be influenced by the goals and strategies that are set, either by the system or by the user, or shared. If the CA follows a health education strategy, topics that fit that strategy could be emphasized, such as providing information about healthy behavior (Zhang & Bickmore, Citation2018). For an implementation intentions strategy, discussing concrete actions to achieve the goal might be more relevant. Once a conversational topic has been chosen, the actions that are selected to execute the topic can be adapted to the user as well. Finally, once tailored actions are selected, the execution of these actions through (multiple) dialogue acts and utterance selection can, in turn, be tailored too. The combination of all approaches can potentially increase adherence and engagement, by providing users with relevant tools and information only.
However, CAs across application domains tend to apply a one-size-fits-all approach when it comes to their responses (Følstad & Brandtzaeg, Citation2017). When looking at the literature on health coaching, there are a number of examples where tailoring in coaching conversations with CAs is performed at the lower levels—with participants all being provided with a predefined order of topics that are discussed (e.g., Zhang and Bickmore (Citation2018)), but there are no examples that tailor the higher level content in the manner that we described above. There are some cases in which tailoring of the higher levels is performed by a health care professional who assigns personalized approaches to participants in the system’s backend (e.g., Abdullah et al., Citation2018; Benítez-Guijarro et al., Citation2018; Fadhil et al. (Citation2019)). While this method of personalization has positive results, the involvement of a health care professional makes it labor-intensive. Bickmore et al. (Citation2011) describe an ontology and task model that are developed to make task execution of health coaching dialogues more modular, dynamic and reusable, but they do not provide such automation for the higher level topics.
1.2. Objectives
To automatically tailor health coaching conversations with CAs on a topic level, we need a blueprint topic model that can be used as the basis for a topic model in a practical implementation. That is, researchers can use it to select and structure topics that they would like to include in their agent applications and can extend it with new topics if that is desired for specific domains or coaching approaches. Since it is essential in the health context that the resulting coaching advice can be thoroughly verified and is predictable from a developer’s point of view (e.g., to ensure safety and to enable certification), an important prerequisite for the model is that it is suitable for use in rule-based dialogue systems.
In this article we focus on the development of that blueprint topic model. We structure the topics in our model as a hierarchical tree, so that in a future implementation a topic selection algorithm can work from the tree’s root and can chose the most relevant subtopic at each split, until a topic has been reached that has no further subtopics. This topic can then be returned as the topic that should be discussed in the conversation that will be started. We therefore address the following research question:
RQ: Which topics should be included in a blueprint topic model for health coaching conversations with CAs, and how should they be structured?
In order to answer this question, we first present a background and review of related research to illustrate the context of this work. We will then report on the two steps we took towards the resulting topic model and its practical implementation in CA systems. First, we investigated which topics are relevant to include in the blueprint topic model based on literature. Second, we evaluated the model that resulted from the first stage by performing a card sort study with experts. We conclude this paper with a discussion of the process, our findings, potential implementation and suggestions for future research.
2. Background and related research
Health behavior change applications with CAs have been researched in the past fifteen years as a method to assist people in obtaining a healthy lifestyle. These agents offer assistance on one or more domains, such as physical activity, nutrition or well-being. In some cases, agents are just a component of a broader eHealth application (e.g., Ter Stal et al., Citation2020; van Velsen et al., Citation2020), while in other cases they are the intervention’s main component (e.g., Op den Akker et al., Citation2018; Sebastian and Richards, Citation2017). In all cases, agents are designed to communicate with the user and in this manner hopefully increase application usage and potential effect.
Coaching sessions with CAs in health behavior change systems generally follow a certain structure. While there are, to our knowledge, no topic models for coaching, there are papers that discuss the structure of a coaching session with a virtual coach as a series of phases. For example, de Kok et al. (Citation2014) present a structure for coaching with a virtual agent during squat exercise sessions. Such a session consists of an introduction, initial assessment, coaching cycle and closing. The coaching cycle itself is a series of explanation, demonstration, instruction, performance assessment and provision of feedback. For insomnia therapy, Beun et al. (Citation2014) describe three phases for the long-term, which are an opening phase, an intervention phase, and a closure phase. The opening phase involves an introduction between coach and coachee, introduction to the therapy, inclusion/exclusion advice, and planning and committing. The intervention phase involves four exercise steps, which consist of an introduction, plan and commit, task execution and evaluation. The closing phase involves closing the therapy.
In addition to interventions having a certain general structure, a number of researchers also motivate the combined use of both a CA and assistive tools. Beun et al. (Citation2017) motivate the combined use of both an agent and tools such as diaries and graphs for self-monitoring in a mobile application. Another example is the agent designed by Bickmore et al. (Citation2013) who augmented the dialogues that could be held with their agent with various images (e.g., characters demonstrating exercises and proper pedometer use) and ‘dynamically generated self-monitoring chart showing the participant’s step counts relative to goals over time’.
While we have found no instances of automatic topic selection as is the subject of this article, there are some examples of tailoring and automatic dialogue or content generation in health behavior change applications with CAs that are relevant to discuss (see for an overview of the literature discussed in the following two paragraphs). For example, the application developed by Fitrianie et al. (Citation2015), which follows the session structure described by Beun et al. (Citation2014), automatically generates the dialogue for the subtopics. In the paper by Beun et al. (Citation2017) a more general approach to instantiating the dialogues using persuasive features is reported. The manner in which Fitrianie et al. (Citation2015) structure the dialogue using interaction recipes, that refer to scripted dialogue actions, allows them to do this in a mobile phone CA application, without the natural language generation process becoming too computationally heavy for the phone. This approach is similar to the use of the task model described by Bickmore et al. (Citation2011), but where Beun et al. (Citation2016) focus on incorporating persuasive features, Bickmore et al. (Citation2011) focus on behavior change models and techniques. Another virtual coach discusses diet one day and physical activity the other day (Bickmore et al., Citation2013), which can be seen as an example of a holistic coaching approach that addresses multiple domains. Both the approaches by Fitrianie et al. (Citation2015) and Bickmore et al. (Citation2011) seem to focus on automatically generating the content to instantiate scripted sets of actions. Montenegro et al. (Citation2019) on the other hand, define a dialogue act taxonomy for a virtual coach, which they use to classify the dialogue acts in coaching dialogues. They do define a hierarchical model for topics, but this is a classification that contains topics such as “Sport and Leisure” with subtopics “Demotivation” (subtopics “Free time,” “Loneliness,” “Fear”), “Hobbies” and “Sport,” which have no coaching aspect to them. That is, they do not include intents to, for example, inform or give feedback. Similarly, their “Intent” model covers relevant intents, but these are aimed at the level of dialogue actions.
Table 1. An overview of the referenced literature on tailoring and automatic dialogue or content generation.
Examples of tailoring communication to users on a more specific level include the generation of brief interventions for excessive alcohol consumption using Markov decision processes and reinforcement learning (Yasavur et al., Citation2013). Smith et al. (Citation2008) include a recommender system that is used in conversations with an agent that suggests and discusses the activities for the day with its user. Richards and Caldwell (Citation2018) describe an agent that discusses a suggested treatment option with patients following a website-based intake. Gupta et al. (Citation2018) worked on the extraction of health goals and topic boundary detection as research towards building a coach that can have SMS conversations. Beinema et al. (Citation2021) investigated the adjustment of coaching strategies to users’ motivation to live healthy in a CA application, and Gross et al. (Citation2021) investigated personalization of interaction styles (e.g., deliberative or paternalistic) for CAs in chronic disease management. Furthermore, even though this is a one-way manner of communication mostly aimed at an utterance level, the process of tailoring messages that are sent to the user has been researched in relationship with users’ stage of change (de Vries et al., Citation2016; Uribe et al., Citation2011), stage of change and personality and gender (de Vries et al., Citation2017), activity and self-efficacy (Achterkamp et al., Citation2013), score on the susceptibility to persuasion scale (Kaptein et al., Citation2012), and the state of the COMBI model (Klein et al., Citation2013). Different tailoring techniques can be used for this purpose, as described in Op den Akker et al. (Citation2014). While not all of these approaches were developed for dialogue-based interactions specifically, we do feel that these tailoring principles can be applied when tailoring the dialogue generation and execution phase that comes after the topic selection.
3. Methods: Topic model construction
The intervention structures discussed in the background section already provide some insight into which topics are prevalent in health behavior change applications. In this section, we will elaborate on our methods for constructing a topic model for use in a coaching conversation with a conversational coach.
We started the process of constructing a blueprint topic model by clearly defining the concepts for the higher levels of tailoring coaching conversations. What do we understand to be a topic? What do we mean with actions? How are they related to the concept of tailoring (as, for example, defined for motivational messages)?
Following the definition phase, we extracted a set of actions from literature. Each of these actions was given a name and description. We took a multidisciplinary approach, looking at literature from the behavioral psychology, relational agents and persuasive technology fields.
Once we had extracted the set of actions from literature, we began by splitting this set into groups of actions that contributed to the same general topic of conversation (following the concept of a hierarchical tree of topics). We then defined a topic name to each of these groups. This resulted in a topic model, with actions being grouped as contributing to the discussion of a shared topic.
4. Results: Topic model construction
4.1. Defining concepts
The first step in building the topic model was to define what we understood a topic and action to be and how this related to the existing literature on tailoring. In this article, we use the term topic, following the definition for a “discourse topic” by Riou (Citation2015) (which is on a higher level than the topic of a sentence). The term topic is used frequently in literature, but as Riou (Citation2015) states, it is rarely defined beyond an intuitive understanding and interpretations vary. She states that a topic is what a portion of the interaction is about and that it must be the center of shared attention. Furthermore, a topic is participant- and interaction-specific; it is jointly determined during an interaction by its participants.
An action is something a coach can do or say during the coaching process. For example, during a conversation on how a user could be more active, a coach could suggest to add a specific activity to their daily schedule (e.g., go on lunch walks), but they could also suggest to replace one activity with a healthier option (e.g., take the stairs instead of the elevator). While these are different actions, they both contribute to a conversation on how someone could be more active. Inclusion of actions in a coaching conversation can require multiple dialogue moves to be executed, but a dialogue that discusses a specific topic might include a combination of actions.
4.2. Extracting actions from literature
The next step was to extract a set of actions from the literature. As stated in the method, the literature we included in our process came from the following three research fields:
The behavioral psychology field. The behavior change technique taxonomy (BCT Taxonomy) by Michie et al. (Citation2013) provides an overview of “techniques a coach may apply,” when it comes to human-human coaching. We therefore included all 93 techniques as actions in our set of actions.
The relational agents field. Research on relational agents teaches us that social actions are important to include if we want a relationship to develop between a coach (the CA) and a user. We therefore defined actions that contribute to this social aspect and included them in our set of actions.
The persuasive technology field. We defined additional actions by reasoning about the actions that are involved when executing persuasive strategies. Examples include the persuasive principles listed by Fogg (Citation2002), which are included in the Persuasive Systems Design model (Oinas-Kukkonen & Harjumaa, Citation2009). We also include a listing of often used persuasive features in the article by van Velsen et al. (Citation2019), which has a specific focus on health coaching.
All three types of literature provided actions that we feel can be important to include when building a health coaching agent. First, the BCT Taxonomy focuses on the core techniques for coaching, but its origin in human-human interaction means that social processes are not addressed. The literature on relational agents allowed us to include important actions for the discussion of social topics. Furthermore, the inclusion of actions based on persuasive technology literature allowed us to include actions that that are relevant to build a persuasive agent application in an eHealth context. Finally, we also defined some basic actions that are relevant for human-computer interaction, such as “explain how to interact with the system” and “assist with sensor connection”.
The resulting set of actions included 115 items. The full list of all 93 behavior change techniques can be found in the article by Michie et al. (Citation2013). The list of 22 additional actions that we defined can be found in Appendix A.
4.3. The topic model
As described in Section 3, we constructed a topic model from the set of actions we extracted from literature by splitting the set of actions into groups that seemed to contribute to the same dialogue and defining a topic to cover each subset. An overview of the resulting topic model can be found in . An overview of topic descriptions and contributing actions can be found in Appendix B.
Figure 2. The final version of the full topic model. The setup of the card sort pilot was based on the initial version of the model, which was adjusted following that pilot’s results as indicated by the asterisks. *The actions covered by the assist with sensor connection subtopic were initially a part of the discuss sensors topic, and the discuss coaching approach topic was initially a subtopic of coaching.
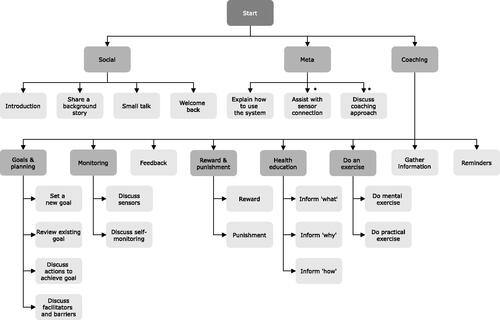
In the following subsections we will discuss the topics in the topic model step by step. Note that we discuss the model as it resulted from the first study and that some topics were moved based on the pilot in the evaluation study.
4.3.1. Split one: the social, meta, and coaching topics
The first distinction that we make is a split between social and coaching topics (see ). We know that if we want to build a working alliance, goal agreement, task agreement and development of a personal bond are important (Castonguay et al., Citation2006; Horvath & Greenberg, Citation1989). From literature on relational and social agents, we also know that it is important to include social behaviors in dialogues (Bickmore et al., Citation2005).
We also include a meta topic at this level, which contains actions in which the agent explains the application (their application) and how to interact with it (and the agent), as such topics can neither be considered social, nor coaching.
4.3.2. Split two: social subtopics
Social communication between an agent and a user is suggested to contribute to the trust and working alliance between an agent and the user (Bickmore, Citation2010). Oinas-Kukkonen and Harjumaa (Citation2009) also list principles such as “similarity,” “liking” and “social role” as important for dialogue support. In their papers on establishing the computer-human working alliance, Bickmore et al. (Citation2005) provide a number of specific examples of verbal relation behaviors. These verbal relation behaviors are: Expressing empathy for a user, social dialogue, reciprocal self-disclosure, humor, meta-relational communication (talk about the relationship), expressing happiness to see the user, talking about the past and future together, continuity behaviors, and reference to mutual knowledge. Similar behaviors are named by Kowatsch et al. (Citation2018).
Bickmore and Picard (Citation2005) explain that they focus on behaviors for relational agents that can be employed by a computer and provide literature that motivates their effects in human-human interaction. Schulman and Bickmore (Citation2009) state that social dialogue may only be relevant in long-term interventions, emphasizing to carefully consider the difference between short-term compliance and long-term adherence. The behaviors listed in these papers are typically intertwined in dialogues that are primarily about another topic (e.g., the use of humor), but some of them might in addition also serve as inspiration for the topic of a full conversation. The getting acquainted talk behavior, for example, inspires a dedicated dialog in which the user and the coach introduce themselves, but this does not prohibit such getting acquainted statements from being used in conversations that are mainly about another topic.
We split the four actions that we extracted from this literature, and we define four subtopics for social dialogues that cover these four actions contributing to “social” conversations: introduction, share a background story, small talk, and welcome back (see ).
4.3.2.1. Introduction
A topic that covers the “getting acquainted talk” (Bickmore & Picard, Citation2005) action. A typical example would be an introduction between user and agent when they meet for the first time. The introduction topic would be most relevant at the start of the relationship between agent and user. Furthermore, an introduction is also a starting point for the information that the coach collects about the user, which can then be used for tailoring the topic selection or lower level personalization (e.g., using the user’s name in a sentence).
4.3.2.2. Share a background story
Discussing the share a background story topic could result in dialogues in which the agent shares a background story about themselves with the user. This type of dialogue is intended to help the process of reciprocal self-disclosure (Bickmore et al., Citation2005) and it can provide a short break in the discussion of coaching content. Furthermore, these types of dialogues could contribute to a user’s engagement by, for example, sharing increasingly more personal parts of the coach’s story, thus keeping the user curious to learn more.
4.3.2.3. Small talk
A topic that covers the “social dialogue” action. Typical dialogues could involve the discussion of general small talk topics, such as the weather. While not of obvious relevance to the coaching process, answers given by the user in these conversations might provide input for tailoring the system’s content to the user, and these dialogue can provide a welcome break in otherwise serious coaching conversations. Furthermore, sometimes interacting with an application is enough to build a social bond (following the computers as social actors (CASA) paradigm (Nass et al., Citation1994)) even when not performing a task (Bickmore & Picard, Citation2005).
4.3.2.4. Welcome back
This topic covers an often short but important dialogue between coach and user in which the user is welcomed back to the application (Bickmore & Picard, Citation2005). As discussed by Bickmore and Picard (Citation2005) for the “good morning” sentence, the sentence itself has lost much of its semantic meaning, but whether you say it and how you say can influence the development of a relationship.
4.3.3. Split three: a meta subtopic
The meta topic is a topic that covers actions that lead to conversations about the application. We defined one subtopic for the meta topic, namely explain how to use the system (see ). Note that following the pilot card sort study, we also added the assist with sensor connection and discuss coaching approach subtopics, which were originally a part of discuss sensors and a separate topic.
4.3.3.1. Explain how to use the system
A topic that covers the “explain interaction paradigm” and “explain user interface” actions. Where in human-human interaction it is rarely necessary for someone to explain that you can talk to them to communicate, in an agent application a user might need some explanation for the use of reply-buttons or where to find certain features of the application (profile page, etc.).
4.3.4. Split four: coaching subtopics
The next split we made is a split in subtopics for coaching (see ).
Figure 6. The subtopics for the coaching topic. *Please note that following the pilot study discuss coaching approach was moved to be a subtopic for the Meta topic.
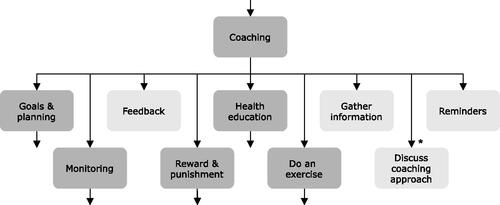
Michie et al. (Citation2013) define 93 behavior change techniques grouped in 16 categories in their taxonomy. These techniques and their categories provided us with a basis for defining the topics for dialogues that a coach might have when it comes to coaching. We also took frequently defined persuasive features in eHealth applications (van Velsen et al., Citation2019) into account when defining these topics (e.g., health education).
4.3.4.1. Goals & planning
The first subtopic is defined for the discussion of goals and planning. In turn, this topic has four subtopics in itself: set a new goal, review existing goal, discuss actions to achieve goal and discuss facilitators and barriers (see ).
One of the important elements in changing behavior is having a goal to work towards. In their Goal-Setting Theory, Locke and Latham (Citation2002) describe that the difficulty and specificity of a goal are the two core factors that influence performance. They describe that four mechanisms influence performance towards a goal: people will be focused on the goal and direct effort towards it, goals have an energizing function, persistence is higher for more difficult goals, and people will act towards it and use task-relevant knowledge and strategies.
Goals and planning is also the first category that Michie et al. (Citation2013) define for their taxonomy. The category features behavior change techniques for setting goals in terms of behavior or outcomes of behavior. It also features techniques regarding reviewing those goals. Within the persuasive technology field, automatic goal setting and self-goal setting are persuasive features that are often used, as well as implementation intentions, for which planning is a key step (van Velsen et al., Citation2019).
4.3.4.1.1. Set a new goal
A topic that covers actions for setting a new goal. There are two typical dialogues for setting a new goal. A long-term goal can be set as a dot on the horizon, while setting short-term goals can help with working towards the long-term goal in smaller and more achievable steps (the reduction principle (Oinas-Kukkonen & Harjumaa, Citation2009)). These dialogues can include a shared decision-making process or a negotiation (Snaith et al., Citation2018). Even if the goal is set automatically by the system, the system can still explicitly ask the user if they accept it (Op den Akker et al., Citation2016).
4.3.4.1.2. Review existing goal
A topic that covers actions for reviewing an existing goal. In a typical dialogue the agent would discuss with the user if the existing goal (long-term or short-term) should be adjusted, and they would adjust the goal if so (e.g., as mentioned by King et al. (Citation2017)). Alternatively, the user can indicate they would like to adjust their goal.
4.3.4.1.3. Discuss actions to achieve goal
A topic covering actions that help the user to take action. In a typical dialogue the coach helps the user to plan actions for achieving their goal, for example by discussing today’s plan (Smith et al., Citation2008), co-creating of a weekly activity plan (King et al., Citation2017), or agreeing to plan a new appointment and that the user will work towards the goal in the meantime (King et al., Citation2017).
4.3.4.1.4. Discuss facilitators and barriers
A topic that covers actions that can help a user overcome barriers or leverage facilitators. In a typical dialogue, a coach could discuss potential problems or opportunities with the user, and they could argue against any self-doubts the user might have (e.g., Bickmore et al. (Citation2005); Bickmore et al. (Citation2013); Gardiner et al. (Citation2017); King et al. (Citation2017); Watson et al. (Citation2012)).
4.3.4.2. Monitoring
Monitoring is an important aspect for tailored coaching and it is strongly connected with another important aspect, namely feedback. For monitoring, we distinguish two subtopics: discuss sensors and discuss self-monitoring (see ).
Michie et al. (Citation2013) defined “feedback and monitoring” as the second category in their taxonomy and distinguish between self-monitoring and monitoring by others. They also distinguish among monitoring of behavior and behavior outcomes, and monitoring with and without feedback. Monitoring is also a key element in persuasive technology, since systems need the information about a user’s behavior to implement persuasive features such as showing progress, social competition, automatic goal setting and rewards (either through compliments or monetary).
4.3.4.2.1. Discuss sensors
A topic that covers actions to do with monitoring through sensors. It also covers the “explain use for sensors” action. In a typical dialogue, the user will get an explanation about the sensor and will then start using the sensor (e.g., for a pedometer (King et al., Citation2017)). Users can also receive help with connecting the sensor to the system if needed (the “connect sensor,” “provide assistance with sensor problems,” and “confirm measured values” actions). Note that the actions covered by the assist with sensor connection subtopic were moved to meta after the pilot of the card sort study.
4.3.4.2.2. Discuss self-monitoring
A topic that covers actions that relate to the user monitoring themselves, e.g., by using a (digital) diary. It also covers the “explain use for self-monitoring” action.
4.3.4.3. Feedback
Being able to provide feedback to the user is an important skill for a coach. Feedback can be given verbally, but the coach could also show a graph of the measured behavior.
As mentioned under monitoring, “feedback and monitoring” is the second category that Michie et al. (Citation2013) define in their taxonomy. Furthermore, persuasive features such as showing progress and rewards are forms of feedback, and features such as social competition can also be seen as a form of feedback.
4.3.4.4. Reward & punishment
The reward & punishment topic covers actions that have to do with consequences for the user’s behavior. We define two subtopics for the reward & punishment topic, namely: reward and punishment (see ).
Reward and punishment are key elements of operant conditioning (Miltenberger, Citation2008), and are essential in human behavior change as external factors that have an influence on people’s motivation. For example, the Health Belief Model states that the perceived benefits of an action and perceived threat influence a person’s likelihood of taking action (Janz & Becker, Citation1984, p. 4) and Protection Motivation Theory (Norman et al., Citation2005) and the Health Action Process Approach (Schwarzer et al., Citation2011) model threat appraisal and risk perception to have a similar effect. Michie et al. (Citation2013) define a “reward and threat” and a “scheduled consequences” category in their taxonomy, which contain BCTs related to incentives, rewards and punishment; and the “reward” persuasive principle (Oinas-Kukkonen & Harjumaa, Citation2009) is reflected in often used persuasive features such as compliments or monetary rewards (van Velsen et al., Citation2019) and also in gamification features such as tokens, points, badges or elements that can be unlocked for customization (e.g., agent outfits or backgrounds) (Edwards et al., Citation2016). A specific example included in the agent literature is shaping (Bickmore et al., Citation2005) (Watson et al., Citation2012).
4.3.4.4.1. Reward
A topic that covers actions that have to do with rewarding the user and discussing the terms for rewards.
4.3.4.4.2. Punishment
A topic that covers actions that have a punishing effect on the user and discussing the terms for punishment.
4.3.4.5. Health education
This topic covers actions through which the coach educates the user about healthy behavior in the coach’s domain. We define three subtopics for health education, namely: inform “what,” inform “why” and inform “how” (see ).
Health education is an educative feature often applied in eHealth applications (van Velsen et al., Citation2019). It is also a topic that is covered in the “shaping knowledge” category of the BCT taxonomy by Michie et al. (Citation2013) and is relevant for some techniques in the “natural consequences,” “comparison of behavior,” and “antecedents” categories. Health education is important for ensuring that the user is informed about their health problem and the options available to them (King et al., Citation2017; Zhang & Bickmore, Citation2018).
4.3.4.5.1. Inform “what”
A topic that covers actions that inform on what healthy behavior is. A typical dialogue provides information based on domain standards and guidelines, but could also involve a short quiz to be more interactive and test the user’s knowledge.
4.3.4.5.2. Inform “why”
Another important aspect of health education is informing why healthy behavior in the domain is important. This can involve fear appeals or listing benefits. The design of inform “why” dialogues could benefit from the work of Chalaguine et al. (Citation2019) who list six types of arguments for health coaching with chatbots and investigate their effects.
4.3.4.5.3. Inform “how”
Actions that inform on how to perform or facilitate healthy behavior are covered by this topic. A typical dialogue would involve suggestions or clear instructions, but other options are to give a demonstration (Jofré et al., Citation2018) or a tip of the day (Bickmore et al., Citation2013; Gardiner et al., Citation2017).
4.3.4.6. Do an exercise
A coach could let the user perform exercises to, for example, practice behavior or gather new insights. In a typical dialogue, the coach would explain an exercise to the user and lets the user do that exercise. Depending on the system, the coach could potentially provide suggestions or feedback during execution. We distinguish do mental exercise or do practical exercise (see ).
4.3.4.6.1. Do mental exercise
A topic that covers actions related to the coach instructing the user to perform mental exercise. Examples could be to have dialogues for exercises aimed at enhancing motivation and using a change ruler (Olafsson et al., Citation2019)) or making a list of pros and cons for changing behavior in the coach’s domain.
4.3.4.6.2. Do practical exercise
Actions related to the coach instructing the user to perform practical exercises are covered by this topic. An example of a typical dialogue could be to introduce and explain a physical activity exercise (Ruttkay & van Welbergen, Citation2008).
4.3.4.7. Gather information
Gathering additional information about the user that can be used for tailoring the provided coaching can be an important topic for a virtual coach.
A typical dialogue could involve questions on previous experience with an exercise (de Kok et al., Citation2014), questionnaires turned into dialogues (e.g., to determine stage of change, motivation to live healthy (van Velsen et al., Citation2019), frailty parameters (Ter Stal et al., Citation2020), or readiness to change (Olafsson et al., Citation2019)), questions about the user’s living environment that might be relevant for activity suggestions (e.g., “Do you live near a park or forest?”). Furthermore, this dialogue can be used to check for important health changes (King et al., Citation2017) or to perform well-being checks (Bickmore et al., Citation2013). When discussed regularly between coach and user, it can also be used for monitoring user’s general physical and mental state (Ruttkay & van Welbergen, Citation2008); essentially using the coach as a sensor.
4.3.4.8. Discuss coaching approach
Another topic that can be important for tailoring coaching conversations is this topic that covers actions aimed at explaining and discussing how the user will be coached. Note that this topic was moved to meta following the results of the pilot for the card sort study.
A typical dialogue could be a dialogue in which a coach would explain their general coaching approach and manners in which it could be tailored to the user. For example, they could explain the purpose and frequency of meetings, and that they can coach the user with a strict or understanding tone of voice. Another example is the suggestion of possible coaching strategies as investigated by Beinema et al. (Citation2021). Depending on the complexity of the system, the user could then indicate what their preference is (e.g., meeting more often). As with the gather information topic, this topic is another example of explicit personalization as defined by Fan and Poole (Citation2006).
4.3.4.9. Reminders
A topic that covers actions related to reminding the user to perform behavior, take measurements, or to use the system.
The potential 24/7 availability of agents as coaches makes that they can also support their user through reminders and following its status as one of the persuasive principles (Oinas-Kukkonen & Harjumaa, Citation2009) “reminders” is an often-included persuasive feature in mobile apps. Reminders could be used for compliance with guidelines/goals (Benítez-Guijarro et al., Citation2018), taking a break (Bickmore et al., Citation2008), or starting a coaching session (Fitrianie et al., Citation2015).
5. Methods: Card sort study
To evaluate the topic model that was constructed in the first stage, as a second step, a closed card sort study with experts was conducted to verify the classification of actions as relevant for the discussion of the topics that were defined.
5.1. Design
In the closed card sort study, experts were asked to sort cards into predefined categories using an online card sorting tool (Proven By Users LLC, Citation2021). Each category in the card sort represented a topic. Each card included the name and description of an action (as defined and extracted from literature in the first study). Since our topic model has a hierarchy, we let the experts perform multiple card sorts. Every round of the card sort represented a split (or “layer”) in the topic model (see ). The first round of the card sort started at the top of the topic model with the split into “social,” “meta” and “coaching”. Participants were therefore asked to sort all cards (with actions) into those three categories. The following rounds each covered further splits into subtopics. For each round, cards were included with the actions that contributed to the discussion of the topics that were included (or their subtopics). In this manner, eight rounds were defined. An overview of round numbers, number of cards (actions), topics (categories), and category labels (topic names) can be found in .
Table 2. Number of cards and categories per round for the initial set-up of the card sort study as tested in the pilot.
5.2. Participants and recruitment
The target group for the study were experts on coaching, conversational agents and eHealth. Potential participants with the relevant expertise were identified through personal connections and recommendations, and sent a recruitment email. This email contained short descriptions of the general research aim, the card sort principle and an estimate for the time it would take to participate. The experts that were asked to participate all had practical experience with the development of eHealth applications in multidisciplinary research settings. While they all worked on eHealth technologies for behavior change, their backgrounds included biomedical engineering, psychology, communication science, computer science, human movement science, nursing, physical therapy, nutrition and health, and health sciences.
5.3. Ethics
The performed online study does not require formal medical ethical approval according to Dutch law. As will be described in the procedure section below, participants were provided with information about the experiment, and the privacy statements of the research facility and the online tool before accessing the online tool. Digital informed consent was obtained from each participant before starting the card sort.
5.4. Procedure and collected data
If participants agreed to participate, they received an email with the details for participating in the study. This email started with an introductory text with a task description, and two links to the privacy statements of the online tool and our research facility. Furthermore, it explained that they should sort the cards as they thought was right and that cards were not necessarily equally divided over groups. Then, the email asked them to fill in a participant code for each of the rounds (with a short note explaining that this would allow us to anonymously connect rounds to the same participant) and provided links to the different rounds of the card sort.
When entering the first card sort round, participants were asked to complete an informed consent form that explained that they could stop their participation whenever they wanted without providing a reason, and that the anonymized data would be used for scientific publications. This was followed by three statements to measure their self-perceived expertise on eHealth, coaching, and conversational agents (“I am an expert on […]”) with a five-point Likert scale ranging from “Completely disagree” to “Completely agree”.
After the intake step, participants were shown the first round of the card sort with a short instruction (to drag cards from the list on the left to the categories on the right). Upon completing each card sort round, they were shown a “thank you”-message with a button that would refer them to the next round. The next round then started again with entering the participant code, a short welcome message, and a short instruction. During all rounds of the card sort, participants had the possibility to save and continue at a later moment. For each round of the card sort, the completion time and final sorting of the cards were stored.
5.5. Data analysis
In each round of the card sort, each participant in the card sort study performed a multi-class classification task (assigning one label to each card). In order to analyze this, we first created confusion matrices for all participants individually. Second, using our original classification of actions as the ground truth, we computed the overall percentage of correctly sorted cards per participant (which in this case is equal to the micro F1-score and accuracy). Third, we computed agreement between participants using Krippendorff’s Alpha. Fourth, we computed the class-wise F1-score for all categories in a round (per participant). Finally, taking the agreement and class-wise F1-scores into account, we looked at the confusion matrices and the raw classification data in order to investigate which cards were classified differently from our labeling and what could be the underlying reasons for those classifications.
6. Results: Card sort study
6.1. Pilot
Prior to the card sort study, a pilot sort was conducted with two experts in June of 2020 to test the study’s procedure and setup (e.g., for clarity of instructions, and to test the cognitive load). The experts were asked to perform the sorts for the first two rounds of the card sort.
The procedure in general was clear, but the cognitive load for completing the two card sort rounds was too high. Furthermore, it was suggested to add a description to the topics. The first round took them 47 min on average and the second round 45 min. For the first round, the experts classified 95.22% (on average) of the cards into the “correct” group. They agreed on the classification of 103 cards, and did not agree on 12. The average precision and recall values can be found in . As can be seen, the recall is high for all categories, while the precision of the “meta” category is quite low. This is because the experts sorted a relatively high number of cards from the “coaching” category (4 for Expert 1 and 13 for Expert 2) into the “meta” category as opposed to the 2 cards that were supposed to be labeled as “meta”.
Table 3. The precision and recall values for both experts for the three categories in the first round of the pilot study.
6.2. Adjustments to study setup and model
Since the number of correct classifications and the agreement was high for the first round in the pilot, it was decided to remove this round from the card sort to lower the cognitive load and time to complete. Cards on which the experts did not agree or that they classified incorrectly were discussed between the first author and the experts. This lead to a reclassification of the “discuss coaching approach” topic as a subtopic for “meta”. Actions that discussed procedural aspects from the “discuss sensors” topic were also reclassified as a second new “meta” subtopic: “Assist with sensor connection”. We also decided to remove the third round (on social subtopics), since participants were asked in that round to sort four cards into four categories with very similar names. These changes led to the updated topic structure as indicated by the asterisks in and a new setup of the card sort rounds as presented in . Furthermore, descriptions were added to clarify the topics (these are included as Appendix B).
Table 4. Number of cards and categories per round for the final set-up of the card sort study.
6.3. Card sort results
The card sort study was conducted in July and August of 2020 in the Netherlands. A total of 11 experts participated in the first round of the card sort study, and 10 of them completed all rounds. The participants rated their expertise on coaching relatively high (Mdn = 4, IQR = 3–4). They also indicated a high expertise on eHealth (Mdn = 5, IQR = 4–5). They were less convinced about their expertise on conversational agents (Mdn = 2, IQR = 1–3.5). On average a participant spent about one hour to complete all rounds of the card sort.
shows the percentage of correctly sorted cards and the agreement between participants for each round of the card sort. The mean class-wise F1-scores for all categories and rounds of the card sort can be found in .
Figure 12. An overview of the mean class-wise F1-scores for the six card sort rounds. N indicates the number of cards.
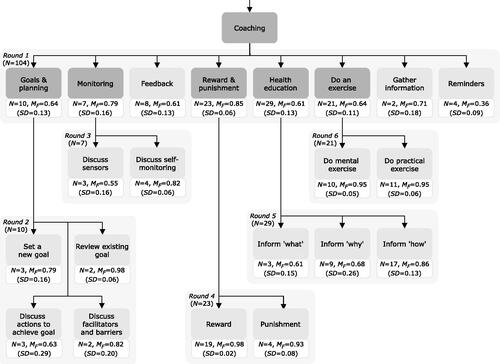
Table 5. Distribution of the percentage of correctly sorted cards per round according to the intended labeling, and the inter-sorter agreement (Krippendorff’s alpha).
The first round, which had the most cards and categories, had the lowest percentage of correctly sorted cards, with participants not fully agreeing on where cards had to go. A frequently occurring switch was sorting “health education” cards as “do an exercise,” and vice versa. Two participants also showed a clear preference for a class (“goals & planning” and “reward & punishment”). For the four cards in the “reminders” category, most participants assigned the cards related to cues into other categories.
A frequent occurrence in the second round was that cards from the “discuss actions to achieve goal” category were assigned to the “set a new goal” category. For the third round there was a clear interpretation of “self-monitoring” to also include monitoring through sensors, while it was intended to be “as opposed to sensors”. Therefore, the two monitoring cards that were labeled as “discuss sensors” were consequently sorted into the “discuss self-monitoring” category by all but one participants.
The fourth round had a high percentage of correctly sorted cards, but some participants mislabeled the “remove punishment” card and the “remove reward” card. The “reduce reward frequency” card was also found to be challenging. These were cards with actions based on BCTs 14.10, 14.3, and 14.9, respectively. In the fifth round, the ‘inform ‘what” cards were mostly sorted correctly, but some cards from the ‘inform ‘why” and ‘inform ‘how” categories were assigned to either of the three. An example was the sorting of the card ‘demonstration of the behavior’ into the ‘inform ‘what” category instead of into the ‘inform ‘how” category. Participants did not have much trouble sorting the cards for the sixth round.
Overall, the results of the card sort showed that most of the topics we defined were clear with the ‘core’ actions that defined the topics being sorted correctly and actions for which it was more difficult to imagine a specific dialogue being sorted differently than intended.
7. Discussion
In this article, we defined a topic model for health coaching conversations with CAs, and evaluated it with experts. The defined model is a blueprint model, which can be used as a basis for developing tailored coaching content and can be extended to support specific types of coaching. While it might be necessary to update the model in the future, to integrate new insights or technological developments, we consider it fixed for now, and it provides a practical and intuitive starting point for implementing systems to automatically select a conversational topic that is tailored to the user. A valuable direction for future work could be to use the model in mapping existing dialogues of conversational agents for health coaching. This might not just provide an overview of which topics and actions are often used, but comparison of used topics and actions between domains or between applications intended for at-home or more clinical settings could also provide valuable insights for dialogue and agent design.
The resulting topic model provides a set of topics that are relevant to include in CAs for health coaching. These topics include a set of “social” topics, for which the actions were deduced from literature on relational agents (e.g., Bickmore et al., Citation2005; Bickmore & Picard, Citation2005; Kowatsch et al., Citation2018), as social interaction is essential for building up a personal bond and beneficial for interactions spanning a longer period of time (Bickmore, Citation2010; Bickmore et al., Citation2018; Schulman & Bickmore, Citation2009). They also include a set of “meta” topics, since it may be useful to let the CA (as the coach or expert) explain how to use various functionalities of the system. We also included a “discuss coaching approach” topic, which can be used to discuss users’ explicit preferences for coaching, since such control features can be beneficial for engagement (Perski et al., Citation2017) and facilitate the tailoring process. Finally, a set of “coaching” topics is also included, which are deduced from behavior change techniques (Michie et al., Citation2013) and persuasive strategies (Oinas-Kukkonen & Harjumaa, Citation2009; van Velsen et al., Citation2019). The topics that are included in the model can be used to generate dialogues with similar resulting structures as described in previous research (for example, the structure by de Kok et al. (Citation2014)), but the hierarchical setup of topics in the model allows for a dynamic implementation with tailored selection. Furthermore, dialogues matching the topics in this model can be combined with tools that support communication and coaching, such as, the diary and graphs discussed by Beun et al. (Citation2017) or the images used by Bickmore et al. (Citation2013).
In addition to its primary use, namely implementing automatic topic selection, the topic model can be used as a starting point when designing dialogue content for a new coaching agent. The model can be used in discussions with domain experts, for example, to identify which topics are important for a specific domain and application. When requesting domain experts to author dialogues, the actions that are classified as contributing to the topics can help clarify what the content for those dialogues could be. Using the topic model in this manner in the content design process also facilitates clear and concise description of the coaching content in scientific publications. Such need for description of coaching content is an issue reported by Michie et al. (Citation2013) for health coaching, but that is still relevant when it comes to coaching with CAs (Kramer et al., Citation2020).
On a larger scale, tailoring coaching dialogues on different levels (domains, topics, actions, dialogue acts, utterances) in a step-wise process has practical benefits. That is, the task goes from deciding on what to say next (in general) to a series of decisions (Which domain? Which topic? Which action? Which dialogue act? Which utterance?). This means that each decision can be made by an expert module, where each module further specifies within the bounds of the previous decision. For example, once the topic has been specified to goal-setting within the physical activity domain, the dialogue could be modeled as a dialogue game (as in Snaith et al., Citation2018) or a dialogue script for that specific topic. This limits the size and complexity of the models or scripts involved, which in turn not only simplifies their construction, but also helps to evaluate and check the resulting coaching dialogues from a safety perspective.
7.1. Implementation of topic selection
We started the process of developing the topic model with a practical application in mind: improving coaching conversations by tailoring the selection of topics that are discussed with individual users. Implementing this topic selection has two key stepsFootnote1. First, a representation of topics and a selection algorithm would need to be implemented. Second, the calculation of a topic’s relevance by the selection algorithm requires parameters to be added to topics that can be used for that purpose.
When it comes to the representation of topics, we made a deliberate choice to structure our topic model as a hierarchical tree. This means that, intuitively, the selection of topics can be implemented as making a choice at each split (e.g., “Would it be more relevant to have a social, meta or coaching conversation now?”). An implementation of such a tree structure would require the topics to be implemented as nodes. Each of these nodes can then be assigned a set of selection parameters that can be used to calculate a topic’s relevance.
An algorithm that traverses the topic tree and outputs which topic to discuss starts at the top of the structure (“start”) and uses the calculated relevance (e.g., a weighted average of selection parameters) for the nodes in the first split to select a subtopic. It then continues this process until it selects a topic that has no more subtopics. Selection of a topic in each step could follow an exploitation strategy by always selecting the node with the highest relevance, but an exploration element could also be included (e.g., by randomly selecting from a distribution based on the topics’ calculated relevance).
Selection parameters that are added to the topic nodes can represent various important elements for a topic’s relevance. Any information available in the system could be used to define these parameters, for example, information collected during an intake, stored during conversations with the coach, set in a preference menu or deduced from sensor data. Examples that come to mind are: availability (e.g., are there still dialogues available for this topic?), prerequisites (e.g., is there sensor data to give feedback on?), strategy (e.g., the inform topic could be more relevant when following a health education strategy) and time (how long has it been since this topic has been discussed previously?). These can be supplemented with parameters that represent other connections between relevant topics and user characteristics as investigated in previous research on tailoring coaching content.
7.2. Limitations
In this article we focused on a blueprint model that includes a set of commonly included topics for health coaching conversations with CAs. A first aspect of our study which might be seen as a limitation could be the use of a closed-card sort study to verify the constructed model instead of constructing the model itself using an open card-sort. However, the large number of actions (115 items) that we extracted from literature would have made an open card sort a task with an enormous cognitive load—as was also confirmed by the feedback from the two participants in the pilot for the closed-card sort.
A second limitation is that there were some actions based on behavior change techniques for which the description in the card sort was ambiguous. For example, these lacked an immediacy indicator which means that there was no clear distinction between “explain to the user how they might perform healthy behavior” and “give the user a specific exercise to perform now”. We made the decision to stay true to the original BCT descriptions when defining actions (and on the cards for the card-sort), but such ambiguity might have made some of the cards in the card sort more difficult to sort. However, this does indicate that some behavior change techniques might simply be more difficult to translate to the digital domain and conversations with an agent than others—a notion that is also supported by reports of occurrence of BCTs in the literature (DeSmet et al., Citation2019; Kramer et al., Citation2020).
8. Conclusion
The inclusion of automatic topic selection in conversational agent applications for health coaching has the potential to improve user’s interactions with these applications by presenting them with engaging and relevant conversations. The concept of a topic-tree was introduced both as a framework for the automatic topic selection, but also as a human understandable structure of the possible topics of conversation. While there are still steps to be taken, the definition of a blueprint topic model as described in this article is a step in the right direction.
Now that we have a blueprint topic model and initial implementations of the topic selection component, the further development of the topic selection component provides plenty of directions for future research. Future work could include the development of tools that allow domain experts to construct topic models and define selection parameters for the embedded topics. A generic variable-driven topic selection tool would allow domain experts to easily influence the artificial intelligence algorithms that automatically select conversation topics based on human understandable variable input. Furthermore, insights from the existing literature on tailoring coaching content within eHealth applications in general might be transferred to the topic selection step in the tailoring process. Finally, the general development of systems that tailor dialogues with conversational coaches in multiple steps can be further investigated, for example, from the technical and user interaction perspectives.
Disclosure statement
No potential conflict of interest was reported by the author(s).
Additional information
Funding
Notes on contributors
Tessa Beinema
Tessa Beinema is a researcher at the University of Twente’s Personalized eHealth Technology program. She has a background in AI with a specialization in web and language interaction. Her research focuses on personalized intelligent applications for health coaching. This paper represents part of her PhD research conducted at Roessingh Research and Development.
Harm op den Akker
Harm op den Akker is the Head of Virtual Coaching at Innovation Sprint. Harm has a background in computer science and a PhD degree from the University of Twente. Harm has over 10 years of experience working in EU R&D projects, and has coordinated the H2020 Project “Council of Coaches”.
Hermie J. Hermens
Hermie J. Hermens is professor in Telemedicine at the University Twente and director of the Personalized eHealth Technology Program. His work focusing on remote monitoring and smart coaching technology has been cited over 26000 times (H-index 70). He participated in over 25 European projects, and coordinated 4 of them.
Lex van Velsen
Lex van Velsen is the head of the eHealth department at Roessingh Research and Development. His expertise includes human-centered design, requirements engineering eHealth, and usability & UX. He has led numerous (inter)national innovation projects and is associate editor at BMC Medical Informatics and Decision Making.
Notes
1 A proof-of-concept implementation of a topic selection component that uses the process described in this section has been included in the Agents United Platform (Beinema et al., Citation2021), where dialogue execution can be performed using either WOOL scripts (Beinema et al., Citation2022a) or dialogue games (Snaith et al., Citation2020). We also evaluated topic selection in a micro-randomized trial as part of the final evaluation of the Council of Coaches project (preprint version published in Beinema (Citation2021), final version published as Beinema et al. (Citation2022), protocol published as Hurmuz et al. (Citation2020)).
References
- Abdullah, A., Gaehde, S., & Bickmore, T. (2018). A tablet based embodied conversational agent to promote smoking cessation among veterans: A feasibility study. Journal of Epidemiology and Global Health, 8(3–4), 225–230. https://doi.org/10.2991/j.jegh.2018.08.104
- Achterkamp, R., Cabrita, M., Op den Akker, H., Hermens, H., Vollenbroek-Hutten, M. (2013). Promoting a Healthy Lifestyle: Towards an Improved Personalized Feedback Approach. 2013 IEEE 15th International Conference on e-Health Networking, Applications and Services, Healthcom 2013 (Healthcom), 725–727. IEEE.
- Andersson, G., Carlbring, P., Berger, T., Almlöv, J., & Cuijpers, P. (2009). What makes internet therapy work? Cognitive Behaviour Therapy, 38(1), 55–60. https://doi.org/10.1080/16506070902916400
- Beinema, T. (2021). Tailoring coaching conversations with virtual health coaches. [Ph.D. thesis]. University of Twente. https://doi.org/10.3990/1.9789036552608
- Beinema, T., Davison, D., Reidsma, D., Banos, O., Bruijnes, M., & Donval, B. (2021). Agents United: An open platform for multi-agent conversational systems. In IVA ’21: Proceedings of the 21st ACM International Conference on Intelligent Virtual Agents (pp. 17–24). ACM.
- Beinema, T., Op den Akker, H., Hurmuz, M., Jansen-Kosterink, S., & Hermens, H. (2022). Automatic topic selection for long-term interaction with embodied conversational agents in health coaching: A micro-randomized trial. Internet Interventions, 27(3), 100502. https://doi.org/10.1016/j.invent.2022.100502
- Beinema, T., op den Akker, H., Hofs, D., & van Schooten, B. (2022a). The WOOL dialogue platform: Enabling interdisciplinary user-friendly development of dialogue for conversational agents [version 1; peer review: 1 approved, 1 approved with reservations]. Open Research Europe, 2(7), 1–15. https://doi.org/10.12688/openreseurope.14279.1
- Beinema, T., op den Akker, H., Velsen, L., & van Hermens, H. (2021). Tailoring coaching strategies to users’ motivation in a multi-agent health coaching application. Computers in Human Behavior, 121(8), 106787. https://doi.org/10.1016/j.chb.2021.106787
- Benítez-Guijarro, A., Ruiz-Zafra, A., Callejas, Z., Medina-Medina, N., Benghazi, K., & Noguera, M. (2018). General architecture for development of virtual coaches for healthy habits monitoring and encouragement. Sensors, 19(1), 108. https://doi.org/10.3390/s19010108
- Beun, R. J., Brinkman, W.-P., Fitrianie, S., Griffioen-Both, F., Horsch, C., & Lancee, J. (2016). Improving adherence in automated e-coaching. A case from insomnia therapy. In International conference on persuasive technology (Vol. 9638, pp. 276–287). Springer.
- Beun, R. J., Fitrianie, S., Griffioen-Both, F., Spruit, S., Horsch, C., Lancee, J., & Brinkman, W.-P. (2017). Talk and tools: the best of both worlds in mobile user interfaces for e-coaching. Personal and Ubiquitous Computing, 21(4), 661–674. https://doi.org/10.1007/s00779-017-1021-5
- Beun, R. J., Griffioen-Both, F., Ahn, R., Fitrianie, S., & Lancee, J. (2014). Modeling interaction in automated e-coaching: A case from insomnia therapy. In COGNITIVE 2014, The Sixth International Conference on Advanced Cognitive Technologies and Applications (MAY), 1–4. IARIA XPS Press.
- Bickmore, T. (2010). Relational agents for chronic disease self management. In B. M. Hayes & W. Aspray, (Eds.), Health informatics: A patient-centered approach to diabetes (pp. 181–204). MIT Press.
- Bickmore, T., & Giorgino, T. (2006). Health dialog systems for patients and consumers. Journal of Biomedical Informatics, 39(5), 556–571.
- Bickmore, T., & Picard, R. (2005). Establishing and maintaining long-term human-computer relationships. ACM Transactions on Computer-Human Interaction, 12(2), 293–327. https://doi.org/10.1145/1067860.1067867
- Bickmore, T., Gruber, A., & Picard, R. (2005). Establishing the computer - patient working alliance in automated health behavior change interventions. Patient Education and Counseling, 59(1), 21–30. https://doi.org/10.1016/j.pec.2004.09.008
- Bickmore, T., Mauer, D., Crespo, F., & Brown, T. (2008). Negotiating task interruptions with virtual agents for health behavior change. Proceedings of the International Joint Conference on Autonomous Agents and Multiagent Systems, AAMAS, 3, 1217–1220.
- Bickmore, T., Schulman, D., & Sidner, C. (2011). A reusable framework for health counseling dialogue systems based on a behavioral medicine ontology. Journal of Biomedical Informatics, 44(2), 183–197. https://doi.org/10.1016/j.jbi.2010.12.006
- Bickmore, T., Schulman, D., & Sidner, C. (2013). Automated interventions for multiple health behaviors using conversational agents. Patient Education and Counseling, 92(2), 142–148. https://doi.org/10.1016/j.pec.2013.05.011
- Bickmore, T., Schulman, D., & Yin, L. (2010). Maintaining engagement in long-term interventions with relational agents. Applied Artificial Intelligence : AAI, 24(6), 648–666. https://doi.org/10.1080/08839514.2010.492259
- Bickmore, T. W., Silliman, R. A., Nelson, K., Cheng, D. M., Winter, M., Henault, L., & Paasche-Orlow, M. K. (2013). A randomized controlled trial of an automated exercise coach for older adults. Journal of the American Geriatrics Society, 61(10), 1676–1683. https://doi.org/10.1111/jgs.12449
- Bickmore, T., Trinh, H., Asadi, R., & Olafsson, S. (2018). Safety first: Conversational agents for health care. In Studies in conversational UX design (pp. 33–57). Springer.
- Brinkman, W.-P. (2016). Virtual health agents for behavior change: Research perspectives and directions. In Proceedings of the Workshop on Graphical and Robotic Embodied Agents for Therapeutic Systems (GREATS16) Held During the International Conference on Intelligent Virtual Agents (IVA16) (pp. 1–17). http://ii.tudelft.nl/willem-paul/. Online at http://www.macs.hw.ac.uk/∼ruth/greats16-programme
- Buimer, H., Tabak, M., van Velsen, L., van Geest, T., & van der Hermens, H. (2017). Exploring determinants of patient adherence to a portal-supported oncology rehabilitation program: Interview and data log analyses. JMIR Rehabilitation and Assistive Technologies, 4(2), e12. https://doi.org/10.2196/rehab.6294
- Callejas, Z., Griol, D., McTear, M., & López-Cózar, R. (2014). A virtual coach for active ageing based on sentient computing and m-health. In International workshop on ambient assisted living (pp. 59–66). Springer.
- Castonguay, L., Constantino, M., & Holtforth, M. G. (2006). The working alliance: Where are we and where should we go? Psychotherapy: Theory, Research, Practice, Training, 43(3), 271–279. https://doi.org/10.1037/0033-3204.43.3.271
- Chalaguine, L. A., Hunter, A., Potts, H., & Hamilton, F. (2019). Impact of argument type and concerns in argumentation with a chatbot. In Proceedings - International Conference on Tools with Artificial Intelligence, ICTAI (pp. 1557–1562). IEEE.
- de Kok, I., Hough, J., Frank, C., Schlangen, D., & Kopp, S. (2014). Dialogue structure of coaching sessions. In Proceedings of the 18th SemDial Workshop on the Semantics and Pragmatics of Dialogue (DialWatt) (pp. 167–169). SemDial. http://pub.uni-bielefeld.de/download/2685764/2706495
- de Vries, R., Truong, K., Kwint, S., Drossaert, C., & Evers, V. (2016). Crowd-designed motivation: Motivational messages for exercise adherence based on behavior change theory. In Proceedings of the 2016 CHI Conference on Human Factors in Computing Systems (pp. 297–308). ACM.
- de Vries, R., Truong, K., Zaga, C., Li, J., & Evers, V. (2017). A word of advice: How to tailor motivational text messages based on behavior change theory to personality and gender. Personal and Ubiquitous Computing, 21(4), 675–687. https://doi.org/10.1007/s00779-017-1025-1
- DeSmet, A., De Bourdeaudhuij, I., Chastin, S., Crombez, G., Maddison, R., & Cardon, G. (2019). Adults’ preferences for behavior change techniques and engagement features in a mobile app to promote 24-hour movement behaviors: Cross-sectional survey study. Journal of Medical Internet Research, 7(12), e15707. https://doi.org/10.2196/15707
- Edwards, E. A., Lumsden, J., Rivas, C., Steed, L., Edwards, L. A., Thiyagarajan, A., Sohanpal, R., Caton, H., Griffiths, C. J., Munafò, M. R., Taylor, S., & Walton, R. T. (2016). Gamification for health promotion: Systematic review of behaviour change techniques in smartphone apps. BMJ Open, 6(10), e012447. https://doi.org/10.1136/bmjopen-2016-012447
- Fadhil, A., Wang, Y., & Reiterer, H. (2019). Assistive conversational agent for health coaching: A validation study. Methods of Information in Medicine, 58(1), 9–23. https://doi.org/10.1055/s-0039-1688757
- Fan, H., & Poole, M. S. (2006). What is personalization? Perspectives on the design and implementation of personalization in information systems. Journal of Organizational Computing and Electronic Commerce, 16(3), 179–202. https://doi.org/10.1207/s15327744joce1603&4_2
- Fitrianie, S., Griffioen-Both, F., Spruit, S., Lancee, J., & Beun, R. J. (2015). Automated dialogue generation for behavior intervention on mobile devices. Procedia Computer Science, 63(Icth), 236–243. https://doi.org/10.1016/j.procs.2015.08.339
- Fogg, B. (2002). Persuasive technology: Using computers to change what we think and do. Ubiquity, 2002(December), 2. https://doi.org/10.1145/764008.763957
- Følstad, A., & Brandtzaeg, P. B. (2017). Chatbots and the new world of HCI. Interactions, 24(4), 38–42. https://doi.org/10.1145/3085558
- Gardiner, P. M., McCue, K. D., Negash, L. M., Cheng, T., White, L. F., Yinusa-Nyahkoon, L., Jack, B. W., & Bickmore, T. W. (2017). Engaging women with an embodied conversational agent to deliver mindfulness and lifestyle recommendations: A feasibility randomized control trial. Patient Education and Counseling, 100(9), 1720–1729. https://doi.org/10.1016/j.pec.2017.04.015
- Glas, N., & Pelachaud, C. (2018). Topic management for an engaging conversational agent. International Journal of Human-Computer Studies, 120(June), 107–124. https://doi.org/10.1016/j.ijhcs.2018.07.007
- Gross, C., Schachner, T., Hasl, A., Kohlbrenner, D., Clarenbach, C. F., Wangenheim, F. V., & Kowatsch, T. (2021). Personalization of conversational agent-patient interaction styles for chronic disease management: Two consecutive cross-sectional questionnaire studies. Journal of Medical Internet Research, 23(5), e26643. https://doi.org/10.2196/26643
- Gupta, I., Di Eugenio, B., Ziebart, B., Liu, B., Gerber, B., & Sharp, L. (2018). Towards building a virtual assistant health coach. In Proceedings - 2018 IEEE International Conference on Healthcare Informatics, ICHI 2018 (pp. 419–421). IEEE.
- Horvath, A., & Greenberg, L. (1989). Development and validation of the working alliance inventory. Journal of Counseling Psychology, 36(2), 223–233. https://doi.org/10.1037/0022-0167.36.2.223
- Hurmuz, M. Z., Jansen-Kosterink, S. M., Op den Akker, H., & Hermens, H. J. (2020). User experience and potential health effects of a conversational agent-based electronic health intervention: Protocol for an observational cohort study. JMIR Research Protocols, 9(4), e16641. https://doi.org/10.2196/16641
- Janz, N., & Becker, M. (1984). The Health Belief Model: A decade later. Health Education Quarterly, 11(1), 1–47. https://doi.org/10.1177/109019818401100101
- Jofré, N., Rodríguez, G., Alvarado, Y., Fernández, J., & Guerrero, R. (2018). Natural user interfaces: A physical activity trainer. In A. E. De Giusti (Ed.), Computer Science – CACIC 2017 (pp. 122–131). Springer International Publishing.
- Kaptein, M., Ruyter, B., de Markopoulos, P., & Aarts, E. (2012). Adaptive persuasive systems: A study of tailored persuasive text messages to reduce snacking. ACM Transactions on Interactive Intelligent Systems, 2(2), 1–25. https://doi.org/10.1145/2209310.2209313
- King, A. C., Campero, I., Sheats, J. L., Castro Sweet, C. M., Garcia, D., Chazaro, A., Blanco, G., Hauser, M., Fierros, F., Ahn, D. K., Diaz, J., Done, M., Fernandez, J., & Bickmore, T. (2017). Testing the comparative effects of physical activity advice by humans vs. computers in underserved populations: The compass trial design, methods, and baseline characteristics. Contemporary Clinical Trials, 61(July), 115–125. https://doi.org/10.1016/j.cct.2017.07.020
- Klein, M., Mogles, N., & van Wissen, A. (2013). An intelligent coaching system for therapy adherence. IEEE Pervasive Computing, 12(3), 22–30. https://doi.org/10.1109/MPRV.2013.41
- Kowatsch, T., Nißen, M., Rüegger, D., Stieger, M., Flückiger, C., & Allemand, M. (2018). The impact of interpersonal closeness cues in text-based healthcare chatbots on attachment bond and the desire to continue interacting: An experimental design. In Proceedings of the 26th European Conference on Information Systems: Beyond Digitization - Facets of Socio-Technical Change, ECIS 2018. AIS.
- Kramer, L., Ter Stal, S., Mulder, B., de Vet, E., & van Velsen, L. (2020). Developing embodied conversational agents for coaching people in a healthy lifestyle: Scoping review. Journal of Medical Internet Research, 22(2), e14058. https://doi.org/10.2196/14058
- Krämer, N., Hoffmann, L., & Kopp, S. (2010). Know your users! Empirical results for tailoring an agent’s nonverbal behavior to different user groups. In International Conference on Intelligent Virtual Agents (pp. 468–474). Springer.
- Krebs, P., Prochaska, J., & Rossi, J. (2010). A meta-analysis of computer-tailored interventions for health behavior change. Preventive Medicine, 51(3–4), 214–221. https://doi.org/10.1016/j.ypmed.2010.06.004
- Laranjo, L., Dunn, A. G., Tong, H. L., Kocaballi, A. B., Chen, J., Bashir, R., Surian, D., Gallego, B., Magrabi, F., Lau, A. Y. S., & Coiera, E. (2018). Conversational agents in healthcare: A systematic review. Journal of the American Medical Informatics Association : JAMIA, 25(9), 1248–1258. https://doi.org/10.1093/jamia/ocy072
- Locke, E., & Latham, G. (2002). Building a practically useful theory of goal setting and task motivation: A 35-year odyssey. American Psychologist, 57(9), 705–717. https://doi.org/10.1037/0003-066X.57.9.705
- McTear, M., Callejas, Z., & Griol, D. (2016). Evaluating the conversational interface. In The Conversational Interface (pp. 379--402). Springer, Cham.
- Michie, S., Richardson, M., Johnston, M., Abraham, C., Francis, J., Hardeman, W., Eccles, M. P., Cane, J., & Wood, C. E. (2013). The behavior change technique taxonomy (v1) of 93 hierarchically clustered techniques: Building an international consensus for the reporting of behavior change interventions. Annals of Behavioral Medicine : A Publication of the Society of Behavioral Medicine, 46(1), 81–95. https://doi.org/10.1007/s12160-013-9486-6
- Miltenberger, R. (2008). Behaviour modification: Principles and practices. Thompson Wadsworth.
- Montenegro, C., López Zorrilla, A., Mikel Olaso, J., Santana, R., Justo, R., Lozano, J. A., & Torres, M. I. (2019). A dialogue-act taxonomy for a virtual coach designed to improve the life of elderly. Multimodal Technologies and Interaction, 3(3), 52. https://doi.org/10.3390/mti3030052
- Nass, C., Steuer, J., & Tauber, E. (1994). Computer are social actors. In Conference on Human Factors in Computing Systems - Proceedings (pp. 72–78). ACM.
- Nijland, N. (2011). Grounding eHealth - towards a holistic framework for sustainable eHealth technologies (Tech. Rep) [PhD thesis]. University of Twente.
- Norman, P., Boer, H., & Seydel, E. (2005). Protection motivation theory. In Predicting health behaviour: Research and practice with social cognition models (pp. 81–126). Open University Press.
- Oinas-Kukkonen, H., & Harjumaa, M. (2009). Persuasive systems design: Key issues, process model, and system features. Communications of the Association for Information Systems, 24(1), 485–500. https://doi.org/10.17705/1CAIS.02428
- Olafsson, S., O’Leary, T., & Bickmore, T. (2019). Coerced change-talk with conversational agents promotes confidence in behavior change. In ACM International Conference Proceeding Series (pp. 31–40). ACM.
- Op den Akker, H., Jones, V., & Hermens, H. (2014). Tailoring real-time physical activity coaching systems: a literature survey and model. User Modeling and User-Adapted Interaction, 24(5), 351–392. https://doi.org/10.1007/s11257-014-9146-y
- Op den Akker, H., Op den Akker, R., Beinema, T., Banos, O., Heylen, D., & Pease, A. (2018). Council of Coaches: A novel holistic behavior change coaching approach. In Proceedings of the 4th International Conference on Information and Communication Technologies for Ageing Well and e-Health (ICT4AgeingWell 2018) (pp. 978–989). SciTePress. https://doi.org/10.5220/0006787702190226
- Op den Akker, R., Klaassen, R., & Nijholt, A. (2016). Virtual coaches for healthy lifestyle. In Intelligent Systems Reference Library 106 toward Robotic Socially Believable Behaving Systems - Volume II (pp. 121–149). Springer.
- Perski, O., Blandford, A., West, R., & Michie, S. (2017). Conceptualising engagement with digital behaviour change interventions: A systematic review using principles from critical interpretive synthesis. Translational Behavioral Medicine, 7(2), 254–267. https://doi.org/10.1007/s13142-016-0453-1
- Proven By Users LLC. (2021). Proven By Users: Card Sort. Accessed August 2022, at www.provenbyusers.com.
- Richards, D., & Caldwell, P. (2017). An empathic virtual medical specialist: It’s not what you say but how you say it. In VSMM ’17: The 23rd International Conference on Virtual System & Multimedia (pp. 1–8). IEEE.
- Richards, D., & Caldwell, P. (2018). Improving health outcomes sooner rather than later via an interactive website and virtual specialist. IEEE Journal of Biomedical and Health Informatics, 22(5), 1699–1706.
- Riou, M. (2015). A methodology for the identification of topic transitions in interaction. Discours, 16, 3--28. https://doi.org/10.4000/discours.8997
- Ruttkay, Z., & van Welbergen, H. (2008). Elbows higher! Performing, observing and correcting exercises by a virtual trainer. In IVA '08: Proceedings of the 8th international Conference on Intelligent Virtual Agents (pp. 409–416). ACM. https://doi.org/10.1007/978-3-540-85483-8_41
- Ryan, K., Dockray, S., & Linehan, C. (2019). A systematic review of tailored ehealth interventions for weight loss. Digital Health, 5(2), 205520761982668–205520761982629. https://doi.org/10.1177/2055207619826685
- Schulman, D., & Bickmore, T. (2009). Persuading users through counseling dialogue with a conversational agent. In Proceedings of the 4th International Conference on Persuasive Technology (Vol. 350). ACM.
- Schwarzer, R., Lippke, S., & Luszczynska, A. (2011). Mechanisms of health behavior change in persons with chronic illness or disability: The Health Action Process Approach (HAPA). Rehabilitation Psychology, 56(3), 161–170. https://doi.org/10.1037/a0024509
- Sebastian, J., & Richards, D. (2017). Changing stigmatizing attitudes to mental health via education and contact with embodied conversational agents. Computers in Human Behavior, 73(8), 479–488. https://doi.org/10.1016/j.chb.2017.03.071
- Smith, C., Charlton, D., Zhang, L., Cavazza, M., Hakulinen, J., & Turunen, M. (2008). An embodied conversational agent as a lifestyle advisor. In Proceedings of the International Joint Conference on Autonomous Agents and Multiagent Systems, AAMAS, 3(AAMAS) (pp. 1663–1664). ACM.
- Smith, C., Crook, N., Charlton, D., Boye, J., de la Camara, R. S., Turunen, M., Benyon, D., Gambäck, B., Mival, O., Webb, N., & Cavazza, M. (2011). Interaction strategies for an affective conversational agent. Presence: Teleoperators and Virtual Environments, 20(5), 395–411. https://doi.org/10.1162/PRES_a_00063
- Snaith, M., De Franco, D., Beinema, T., Op den Akker, H., & Pease, A. (2018). A dialogue game for multi-party goal-setting in health coaching (Vol. 305). University of Dundee.
- Snaith, M., Lawrence, J., Pease, A., & Reed, C. (2020). A modular platform for argument and dialogue. IOS Press.
- Starr, J. (2008). The coaching manual: The definitive guide to the process, principles, and skills of personal coaching. Pearson Education.
- Ter Stal, S., Broekhuis, M., van Velsen, L., Hermens, H., & Tabak, M. (2020). Embodied conversational agent appearance for health assessment of older adults: Explorative study. JMIR Human Factors, 7(3), e19987. https://doi.org/10.2196/19987
- Uribe, J., Duitama, J., & Gaviria Gómez, N. (2011). Personalized message emission in a mobile application for supporting therapeutic adherence. In 2011 IEEE 13th International Conference on e-Health Networking, Applications and Services, HEALTHCOM 2011 (pp. 15–20). IEEE.
- van Velsen, L., Broekhuis, M., Jansen-Kosterink, S., & Op den Akker, H. (2019). Tailoring persuasive ehealth strategies for older adults on the basis of personal motivation: An online survey. Journal of Medical Internet Research, 21(9), e11759. https://doi.org/10.2196/11759
- van Velsen, L., Cabrita, M., Op den Akker, H., Brandl, L., Isaac, J., Suárez, M., Gouveia, A., Dinis de Sousa, R., Rodrigues, A. M., Canhão, H., Evans, N., Blok, M., Alcobia, C., & Brodbeck, J. (2020). Leaves (optimizing the mental health and resilience of older adults that have lost their spouse via blended, online therapy): Proposal for an online service development and evaluation. JMIR Research Protocols, 9(9), e19344. https://doi.org/10.2196/19344
- Wangberg, S. C., Bergmo, T. S., & Johnsen, J.-A K. (2008). Adherence in internet-based interventions. Patient Preference and Adherence, 2(2), 57–66.
- Watson, A., Bickmore, T., Cange, A., Kulshreshtha, A., & Kvedar, J. (2012). An internet-based virtual coach to promote physical activity adherence in overweight adults: Randomized controlled trial. Journal of Medical Internet Research, 14(1), e1. https://doi.org/10.2196/jmir.1629
- Yasavur, U., Lisetti, C., & Rishe, N. (2013). Modeling brief alcohol intervention dialogue with MDPs for delivery by ECAs. In Lecture Notes in Computer Science (Including Subseries Lecture Notes in Artificial Intelligence and Lecture Notes in Bioinformatics), 8108 LNAI (pp. 92–105). ACM.
- Zhang, Z., & Bickmore, T. (2018). Medical shared decision making with a virtual agent. In Proceedings of the 18th International Conference on Intelligent Virtual Agents, IVA 2018 (pp. 113–118). ACM.
Appendix A.
Overview of actions extracted from literature (excluding BCTs)
Table A.1. An overview of the coaching actions that were defined based on the literature on relational agents and persuasive technology.
Appendix B.
An overview of the descriptions added to the topics for the card sort
Table B.1. An overview of all defined ‘social’ topics, the descriptions added for the card-sort, and actions that contribute to their discussion.
Table B.2. An overview of all defined ‘meta’ and ‘coaching’ topics, the descriptions added for the card-sort, and actions that contribute to their discussion.