Abstract
Widespread adoption of artificial intelligence (AI) technologies is substantially affecting the human condition in ways that are not yet well understood. Negative unintended consequences abound including the perpetuation and exacerbation of societal inequalities and divisions via algorithmic decision making. We present six grand challenges for the scientific community to create AI technologies that are human-centered, that is, ethical, fair, and enhance the human condition. These grand challenges are the result of an international collaboration across academia, industry and government and represent the consensus views of a group of 26 experts in the field of human-centered artificial intelligence (HCAI). In essence, these challenges advocate for a human-centered approach to AI that (1) is centered in human well-being, (2) is designed responsibly, (3) respects privacy, (4) follows human-centered design principles, (5) is subject to appropriate governance and oversight, and (6) interacts with individuals while respecting human’s cognitive capacities. We hope that these challenges and their associated research directions serve as a call for action to conduct research and development in AI that serves as a force multiplier towards more fair, equitable and sustainable societies.
1. Introduction
The time of reckoning for Artificial Intelligence is now. Artificial Intelligence, or AI, started as the quest to not just understand what intelligence is, but to build intelligent entities (Dietrich & Fields, Citation1989). Since the 1950s, AI developed as a field that combined increasing multitasking abilities, computational power, and memory with progressively larger datasets, allowing for computer-based inference and problem-solving (Komal, Citation2014). The subfields of machine learning (ML), deep learning, and reinforcement learning were later developed which leverage AI algorithms – computational units which transform given inputs into desired outputs – to provide predictions and classifications based on available data. Currently the digitalization of most aspects of human activity has produced massive amounts of data for training algorithms. This data coupled with the exponential increase in computational power is propelling AI techniques to become widespread across all industries (Le et al., Citation2020). Although the ultimate goal of building fully intelligent entities remains elusive, the age of AI is already impacting humanity in ways that are substantial yet not well understood. In the recent past, various scientific disciplines including physics and chemistry had to reckon with the societal consequences of their scientific advances when these advancements migrated from conference discussion or a laboratory experiment into wide adoption by industry. In a similar manner, now is the time for the scientific community to grapple with the societal consequences and potential changes to the human condition resulting from the adoption of current AI systems.
AI has permeated many industries and aspects of human life. For example, in healthcare, while AI has improved diagnosis, treatment, and lowered the cost and time to discover and develop drugs, it has also introduced biases in automated decision making. These biases are detrimental to demographic minorities because of the disproportionate over-representation of Caucasian and higher income patients in electronic health record databases (West & Allen, Citation2020, July 28). In criminal justice, the Correctional Offender Management Profiling for Alternative Sanctions (COMPAS) is a decision making AI deployed by the US criminal justice system to assess the likelihood of a criminal defendant’s recidivism. COMPAS has shown significant bias against African American defendants (Chouldechova, Citation2017). Similarly, predictive AI-assisted policing affects minorities disproportionately (Spielkamp, Citation2017). Algorithmic biases also exist in AI systems used in the education sector. For example, bias has been demonstrated in algorithmic decision making for university admissions and in predictive analytics for identifying at-risk students (Williams et al., Citation2018). In the technology sector, studies show that search engine targeted advertising shows high-paying jobs significantly more frequently to males than females (Lambrecht & Tucker, Citation2019). In the financial sector, “color-blind” automated underwriting systems recommend higher denial rates for minority racial/ethnic groups (Bhutta et al., Citation2021). Algorithms that curate social media content for engagement maximization provide personalized content that lack diversity of opinions and information. Studies show that AI curation risks creating silos of opinions and echo chambers that eventually lead to deep divisions in society (Section 2.2.3). In our view, technology companies, admission officers, hiring officers, banking executives and other decision makers could obtain better results by adopting an all-encompassing human-centered approach to AI-driven curation, moderation, and prediction instead of a purely technological one. Indeed, many firms that famously adopted purely technological processes have found it necessary to reintroduce humans.
Throughout previous industrial evolutions, mechanization eclipsed human abilities to perform physical work, while humans maintained cognitive superiority. In the current age, many have warned of AI exceeding human intelligence leading to job loss, dependence, and far-reaching societal effects (Anderson et al., Citation2018). However, human and artificial intelligence are not equivalent. While AI performs well at multitasking, computation, and memory, humans excel in logical reasoning, language processing, creativity and emotion, among other areas (Komal, Citation2014). Although some have envisioned a future in which AI eclipses human intelligence (Grace et al., Citation2018), this group argues for a future in which advances in AI augment rather than replace humans and improve their environment. Ultimately, AI should support the wide-reaching goals of increasing equality, reducing poverty, improving medical outcomes, expanding and individualizing education, ending epidemics, providing more efficient commerce and safer transportation, promoting sustainable communities, and improving the environment (United Nations Department of Economic & Social Affairs, Citation2018, Apr 20).
Given the impact that the human-computer interaction (HCI) field has had on expanding the capabilities and widespread use of computers, HCI is in a unique position to expand the usefulness of AI and ensure that future applications are human-centered. While HCI has previously focused on the human and how technological artifacts can be better designed to meet the user’s needs, in the age of AI, HCI can lead the way in providing a much-needed human-centered approach to AI. While traditional software systems follow a set of rules that guide in the generation of a result, AI systems evolve and adapt over time. For instance, ML has three main design stages: (1) data collection and curation, (2) algorithm design and experimentation and (3) deployment and use (). As a result, the AI design cycle is a continuous process and requires perpetual oversight as part of the design framework to preserve alignment of system goals and objectives with user values and goals. Furthermore, human values, goals and context also change over time. Recognizing these changes and adopting system behavior to ensure value alignment is essential for human-centered design. Moreover, unlike humans and human teams, presently AI systems have no rights, responsibilities and are not accountable for their actions and decisions, though this could change in the future (see 6. Governance and Independent Oversight). People are responsible and accountable for the system design and behavior which requires them to comprehend AI well enough to anticipate potentially problematic system behavior (Hoffman et al., Citation2018). This requires new design methods, practices, verification and validation methods, and frameworks to detect and incorporate ever evolving human goals and values from user research into the AI system’s objectives (Gibbons, Citation1998) and build responsible, transparent, and interpretable AI systems.
In contrast to the problematic AI systems mentioned above that have been developed and deployed to date, human-centered AI (HCAI) seeks to re-position humans at the center of the AI lifecycle (Bond et al., Citation2019; Riedl, Citation2019; Xu, Citation2019) and improve human performance in ways that are reliable, safe, and trustworthy by augmenting rather than replacing human capabilities (Shneiderman, Citation2020a). Such an approach considers individual human differences, demands, values, expectations, and preferences rather than algorithmic capabilities, resulting in systems that are accessible, understandable, and trustworthy (Sarakiotis, Citation2020), allowing for high levels of human control and automation to occur simultaneously (Shneiderman et al., Citation2020b). This approach will encompass establishing frameworks for design, implementation, evaluation, operation, maintenance, decommissioning, and governance of AI systems that will strive to create technologies that are compatible with human values, protect human safety, and assure human agency. HCAI is beginning to make impacts on education (Renz & Vladova, Citation2021) and medicine (Gong et al., Citation2021), but widespread adoption remains forthcoming. Given the current trajectory of AI research and industrial adoption, we can easily envision a future in which AI is even more ingrained in society and its impact more salient. It is in this context that this group proposes six grand challenges in HCAI along with a human-centered research agenda for the responsible study, development, deployment, and operation of AI systems that will guide and promote research that is responsible, sustainable, ethical, and in essence, human-centric. Implementing the HCAI vision is of necessity highly interdisciplinary, requiring the integration of expertise across traditional disciplines such as HCI, ML, and software engineering, but given the increasing reach and worldwide impacts of AI technology, complementary fields such as sociology, ethics, law, bioengineering, and policy will also be required (Bond et al., Citation2019).
This article presents the results of 26 international experts from North America, Europe, and Asia with broad interest in the field of HCAI across academia, industry, and government. Participation was voluntary and recruiting was done through professional networks. Expertise was sought across disciplines in the social and computational sciences as well as industry. Considerations were given to increase participation by members of under-represented groups when announcements and invitations were sent. The group had various educational backgrounds with a majority holding Ph.Ds. Disciplines ranged from Computer Science, Psychology, Engineering, and Medicine, with different specializations within represented disciplines.
The group’s collaboration started in early 2021, with the identification through online questionnaire and discussion of major challenges facing the adoption and application of HCAI principles at present, scientific challenges and opportunities for HCAI in the future, and recommendations for future research directions. Authors of this article collaborated synchronously and asynchronously to concept-map (Falk-Krzesinski et al., Citation2011) the collected opinions, summarize them into major areas, and rank them through an online questionnaire.
Results were presented to the broader research community at the HCI International 2021 on July 26 2021. Major areas included: trustworthy AI for a human-centered future; AI, decision making, and the impact on humans; human-AI collaboration; and exploring a human-centered future for AI (Garibay & Winslow, Citation2021). Announcements about the session were sent to the research community interested in HCAI. Those who accepted were invited to a one-day closed meeting held on July 27 2021, the major challenges were analyzed and discussed, and a condensed set of 6 challenges was produced (), followed by team member development of written descriptions of the challenges, rationale for including them, main research challenges, and emerging requirements. Breakout groups were formed for smaller discussions and online documents, editable by all participants, were used for information collection. The overall session and breakouts were facilitated by members of the author team. Given that this was done in an online and synchronous interaction platform, host facilitation ensured that communication was managed and open among participants.
The six grand challenges of building human-centered artificial intelligence systems and technologies are identified as developing AI that (1) is human well-being oriented, (2) is responsible, (3) respects privacy, (4) incorporates human-centered design and evaluation frameworks, (5) is governance and oversight enabled, and (6) respects human cognitive processes at the human-AI interaction frontier. The first major challenge presented represents the overall purpose of HCAI, human-wellbeing to ensure that AI systems are centered on improving people’s lives and experiences. The second and third challenge areas represent principles that ensure responsible AI system design and development and protect human privacy. The fourth and fifth challenges represents processes to develop and provide a comprehensive HCAI design, evaluation, governance and oversight framework for appropriate guidance and human control over AI life cycle. The final challenge area represents the ultimate product of HCAI, the vision of future human-AI interaction. Since the identified challenges are interrelated, the discussions that follow are interconnected, e.g., a comprehensive HCAI design, implementation, and evaluation framework encompasses aspects of responsibility and privacy. We describe these six challenges and their associated research directions and recommendations (see Sections 8.1 and 8.2). These serve as a call for action to conduct research and development in AI that accelerates the movement towards more fair, equitable and sustainable societies.
2. Human well-being (challenge 1)
2.1. Definitions and rationale
2.1.1. Human well-being
The concept of human well-being is hard to define. Two general perspectives have emerged over the years: the hedonic approach, which focuses on positive emotions such as happiness, positive affect, low negative affect, and satisfaction with life (Diener et al., Citation2009); and the eudemonic approach, which focuses on meaning and self-realization, viewing well-being in terms of the degree to which a person is fully functioning (Ryff & Singer, Citation2008). Today, the common understanding is that well-being is a multi-dimensional construct involving both a hedonic and a eudemonic dimension. For example, Positive Psychology (Seligman, Citation2012) supports the concept of human flourishing, intended as “positive” mental health (Keyes, Citation2002).
Towards these aims there is a need to elaborate and explore what is understood about well-being and to apply them to AI, ultimately translating these higher-level concepts into at least two veins of effort: 1. Discovering implementation opportunities for AI to benefit human well-being and 2. Making specific design considerations to support user well-being when interacting with AI.
2.1.2. Unique features of AI technology that may impact well-being
In its various conceptions, AI or technologies that replicate, exceed, or augment human capabilities have characteristics that may uniquely impact human well-being. By design, AI offers a higher level of automation and self-direction, requiring less human input. However, it lacks a concept of human values, common sense, or ethics (Allen et al., Citation2005; Han et al., Citation2022), and thus may perform its tasks or influence decisions from a basis that is not human-oriented or may cause direct harms. In many cases AI is trained on data derived from human behaviors and thus may adopt inclinations or behaviors that result from implicit bias in that data (Ntoutsi et al., Citation2020). When ML algorithms are trained to optimize a narrowly defined outcome this may be at the expense of other desired (alternative) outcomes representing values that were not included in the model. AI may influence what people believe to be true or important in a decision making task, but do so spuriously with potential negative consequences (Araujo et al., Citation2020). Because underlying methods are generally not transparent, users of AI may overtrust or undertrust the system, leading to errors (Okamura & Yamada, Citation2020). Explainability methods can be complex for end users and have a similar result of overtrust (Ghassemi et al., Citation2021; He et al., Citation2022). Learning systems can be dynamic, such that performance may not be consistent. This may result in an inappropriate shift in responsibility where end users are expected to evaluate a tool’s performance without a desire or realistic capability of doing so (Amershi et al., Citation2014; Groce et al., Citation2014). Finally, AI is an added accelerant for technology development (Wu et al., Citation2020). Any degree of mastery in conceptualizing and creating human-centered AI could have both early, broad, and ultimately persistent impacts.
As the AI becomes increasingly integrated (Poquet & Laat, Citation2021) in work and life, it is essential that we can consider, observe, evaluate, articulate, and act upon the human experience with AI, both individual and collective. A well-being orientation is mindful of these concerns. It approaches AI from a perspective of human impacts, individual and collective, with the aim of generally increasing eudaimonia and flourishing for those who either interact with or are impacted by AI.
In this challenge we consider the unique impacts of AI as a technology and the types of considerations that might be made as human-AI interactions become a common part of the human experience. Social media is discussed as a representative case study.
2.2. Main research issues and state of the art
2.2.1. Foundational and actionable characteristics of well-being-oriented AI
As already mentioned, AI systems are considered as potentially capable of enhancing human well-being, since they offer technical solutions for monitoring and reasoning about human needs and activities, as well as for making decisions about how to intervene in support of humans. In this context, however, it is important to answer the question of which characteristics AI systems must have to achieve the above objectives.
In this respect, the quality of experience becomes the guiding principle in the design and development of new technologies, as well as a primary metric for the evaluation of their applications. “Positive technology” as a general term (Riva et al., Citation2012), and “Positive computing” more specifically (Calvo & Peters, Citation2014), suggest that it is possible to use technology to influence specific features of human experience that serve to promote adaptive behaviors and positive functioning, such as affective quality, engagement, and connectedness.
Targeted well-being factors include positive emotions, self-awareness, mindfulness, empathy, and compassion, and technology has been proposed to support these factors (Calvo & Peters, Citation2014).
In order to enhance human well-being, emerging AI technologies should be inclusive, avoid bias, and be transparent and accountable (Section 3), respect human resources – especially time and data (Section 4), adopt simplicity in design (Section 5), prevent negative side effects (Section 6), and respect, support and expand human cognitive capacities and adapt to humans (Section 7), (Wellbeing AI Research Institute, Citation2022).
As a research foundation, a very robust and potentially useful framework is Self-Determination Theory (SDT) which is well aligned with eudaimonia concepts and offers an evidence-based approach for increasing motivation and well-being (Ryan & Deci, Citation2017). SDT is “deliberate in its embracing of empirical methods, and statistical inferences, as central and meaningful to its epistemological strategy” and has been investigated in thousands of studies across a variety of domains including the workplace, schools and learning, sports and exercise, healthcare, psychotherapy, cultural and religious settings, and even virtual worlds (Ryan & Deci, Citation2017). SDT finds that autonomy, competence, and relatedness are the essential psychological nutrients that are essential for individuals’ motivation, well-being, and growth. As an approach that enhances both well-being and intrinsic motivation, SDT could be an especially useful resource in designing AI tools that support eudaimonia while also creating engagement towards business goals or other imperatives (Peters et al., Citation2018).
2.2.2. Opportunities for AI that support well-being
As it does with other ambitions, AI offers many opportunities towards the pursuit of well-being. For example, a discussion is ongoing among the scientific and the global community regarding how technology can support the UN Sustainable Development Goals (SDGs) (United Nations Department of Economic & Social Affairs, Citation2018, Apr 20) for 2030, which also include good health and well-being for everybody. AI has been identified as a supporting technology in the achievement of 128 targets across all SDGs (Vinuesa et al., Citation2020). The AI for Good initiative (AI for Good, Citation2021) aims to identify practical applications of AI to advance the UN SDGs.
Mental health is a domain where several efforts have leveraged AI towards improving well-being. In this context, AI is often used in the development of prediction, detection, and treatment solutions for mental health care. AI has been incorporated into digital interventions, particularly web and smartphone apps, to enhance user experience and optimize personalized mental health care (Neary & Schueller, Citation2018), and has been used to develop prediction/detection models for mental health conditions (Carr, Citation2020). Smart environments including monitoring infrastructures as well as AI-based reasoning components have been proposed to address common well-being issues relevant for large parts of the population, such as emotion regulation (Fernandez-Caballero et al., Citation2016), stress management (Winslow et al., Citation2016, Citation2022), sleep hygiene (Leonidis et al., Citation2021), and independent living and everyday activities of people with disability and the aging population (Burzagli et al., Citation2022). A common issue for this type of systems is assessing their impact on well-being in a reliable way and using effective instruments.
Another exemplary area effort involves the development of interactive technologies to support universal accessibility (see Section 5.2.3 Ensuring universal accessibility). These tools aim to provide technological solutions that are accessible by all and support the independent living and everyday activities of people with disabilities and advancing age (Stephanidis, Citation2021). In this context, AI has the potential to provide the reasoning means to make decisions about the type of support needed in an individual and context-dependent way (Burzagli et al., Citation2022).
2.2.3. Unintended AI impacts on general well-being
The question of harms is crucial to the design community, especially those harms that are incidental, that arise from choices in the design of AI rather than the specific intent of an AI tool. Certainly, the malevolent use or weaponization of AI is to be safeguarded against (Winfield et al., Citation2019), but a response to those issues may need less advocacy than the issue of less apparent harms from AI that arise from poor design.
Perhaps the most insidious risk with AI is that its applications will have mixed results, with enthusiastic deployments accompanied by harms that are never corrected or evaluated. This might be the case with a tool that increases capability but adds risk, stress, or administrative burdens. A tool might benefit people generally while exhibiting bias or marginalizing subgroups of people. For example, a tool may improve efficiency, such as academic admissions processes, (Vinichenko et al., Citation2020), but result in marginalization or unequal distribution of resources (see Section 1). An application may leverage data in useful ways, but also impact privacy inappropriately (see Section 4). Decision support tools may be generally superior to humans but lack the common sense or values to address outlier cases in a humane way. As discussed in other challenges, AI may exacerbate inequalities, result in job disruption, and cause worker deskilling (Ernst et al., Citation2019). In another example, social media algorithms confer both benefits and harms not only to individual users but also to society at large (Balaji et al., Citation2021). Beyond the individual and groups there are potential impacts on the natural world from AI (Ryan, Citation2022), with which human flourishing is inextricably enmeshed.
The UN targets previously discussed also face the issue of mixed benefits and harms. While AI could improve 128 of the previously mentioned UN SDG’s, it may also inhibit 58 targets. For example, while the SDGs are founded on the values of equity, inclusion, and global solidarity, the advent of AI could further exacerbate existing patterns of health inequities if the benefits of AI primarily support populations in high-income countries, or privilege the wealthiest within countries (Murphy et al., Citation2021).
The underlying aim of AI for well-being is to support “inclusive flourishing” so that as many as possible can experience autonomy, competence, and relatedness, and are enabled to grow and pursue their purpose or goals in life (Calvo et al., Citation2020). While much of this has been discussed in terms of AI as a force for change, it will also become an increasingly common interactional experience for individuals. It is crucial to begin to understand the human-AI interaction itself, which is a poorly explored but potentially impactful experience that will become increasingly persistent in our work and lives.
2.2.4. Understand user impacts of augmented decision making
Decision making is of particular interest to human well-being. With the presence of risk and the possibility of loss, even the most rational decision making process has emotional components (Croskerry et al., Citation2013). A decision making process can result in satisfaction or result in decisional stress, decisional conflict, lack of closure, or regret (Becerra-Perez et al., Citation2016; Joseph-Williams et al., Citation2011). Adding the uncertainty and complexities of AI and AI explanations poses additional uncertain impacts to these decisional stresses.
In domains such as law, healthcare, defense, finance, and admissions, there are longstanding processes to support trustworthy decisions and support the well-being of decision makers by providing an explainable and defensible framework for their outputs. AI can potentially disrupt these processes, offering irresistible capabilities coupled with non-intuitive methods that can fail disastrously and indefensibly. Without effective design strategies, these realities will leave users with the stress of being responsible for AI without having full understanding or control (see also section 3.2.1 Accountability and liability – moral and legal)
The question of responsibility highlights the important issue of task and risk-shifting that can occur with AI in decision making or other tasks. Ultimately, people are responsible for actions performed by AI or decisions made with AI support. However, the reasons that AI delivers a particular result are difficult for users to explain or verify on a variety of levels (Wing, Citation2021). Explainability methods can be useful but are also complicated (T. Miller, Citation2019) and potentially fallible themselves, making them incomplete or inappropriate solutions in some cases (Rudin & Radin, Citation2019). Also, the challenge of verification is ongoing, as outputs can change given the dynamic “learning” of these tools.
In some cases, it may fall to the user to prevent mistakes made by AI, but they may neither desire this nor have the capabilities or time to do so. In one anecdote (Szalavitz et al., Citation2021), a patient was unable to receive adequate pain treatment due to an AI algorithm which suggested that the patient had a high risk of overdose. With little ability to evaluate the AI and a desire to prevent overdose, the physicians generally accepted its recommendation. It was left to the patient to confront the AI company, ultimately discovering that the algorithm had been skewed by prescriptions made for the patient’s dog. In this case, both the risk of AI harm and the work of remediating it was shifted to the patient, paradoxically the person with the least agency to do so. These issues in decision support are possible with loan approvals, school applications, or any similar situation where black-box AI-generated information impacts human benefits.
Automated technologies present similar challenges. From self-driving cars to self-regulating factories, power-grids, financial processes, and robots, there is a need to consider task and risk-shifting such that when it occurs it is intentional and acceptable to the involved stakeholders. Furthermore, tools must provide appropriate feedback and controls so that if users are assigned to bear the risks that AI contributes, they can do so comfortably and capably (Cheatham et al., Citation2019). In short, AI interfaces should result in both peace and power, and it is not simply algorithm design, but ultimately excellence in interface designs and collaborative decisions about risk management that bear this responsibility. proposes 6 principles and questions toward HCAI design for human well-being.
Table 1. Six principles that promote well-being in HCAI
2.2.5. Case study: Ethical use of AI in social media
The ethical use of AI in social media is an important component of promoting human well-being. The SDT concept of relatedness implies social connectivity, or the sense of belonging to a community as a source of happiness. Relatedness can be promoted or distorted by user engagement with social media platforms that relay on automated, algorithmic curation or information resulting on constructive of destructive effects to human well-being.
From life-saving health information to democratic decision making, access to accurate and truthful information is essential for human and societal well-being. With social media, AI has become an invisible but ubiquitous mediator of our social fabric, determining what information reaches who and for what purpose, raising important questions about its effects on the well-being of our society. Currently, most online users consume news through social networks (Shearer & Mitchell, Citation2021) where their news feed is under the control of the social network platforms. Yet, social media platforms acknowledge only a limited role in moderating and curating the information in their systems. This, along with a new ability to publish content easily and cheaply has created an explosion of information coupled with a vacuum of oversight wherein misinformation and disinformation have flourished.
The AI mediation of this information has resulted in a distorted and manipulated information environment with varied impacts ranging from misinformed users to the extreme polarization and radicalization of users. These impacts can result from an admittedly blurry spectrum ranging from misinformation (where false or out-of-context information is presented as fact whether or not the intention to deceive is explicit) to disinformation (with an explicit intent to deceive).
The design objective of social networks themselves centers on the following four activities: View, Like, Comment, and Subscribe/Follow. The success of the platform is determined by the count of these activities, and the AI algorithm, known as the social recommender system (Guy, Citation2015), curates the content provided to the individual user to maximize the activities. The recommender system is essential to the success of the social media website which raises a question regarding the privacy of monitored individuals and other ethical challenges (Milano et al., Citation2020). The appropriate use of AI for mediating or “recommending” news also raises the question of how much power the design of such platforms and their AI algorithms have over the “individual-level visibility of news and political content on social media” (Thorson et al., Citation2021). Perhaps the most notable study is the Facebook emotional contagion study (Kramer et al., Citation2014), in which Facebook would alter its news feed for individual experience to manipulate their emotional state. Using only photographs as input data (Chen et al., Citation2017), social network platforms were able to reliably identify the user’s mental state. Young people are particularly vulnerable to social media effects. For instance, researchers reported increased consumption of alcohol and rising levels of stress (Oliva et al., Citation2018), depression, and loneliness (Park et al., Citation2015) among young people using Facebook.
Perhaps the most serious ethical breaches involve the use of social media for social control (Engelmann et al., Citation2019) and disinformation campaigns (Lazer et al., Citation2018). The most visible example of a social control campaign is the “social credit system” implemented by the Chinese government, in which the “state surveillance infrastructure” (Liang et al., Citation2018) punishes failures to conform to the choices of the regime (Creemers, Citation2018). The system is heavily dependent on AI which monitors public events through installed video cameras around the country using facial recognition systems to identify individuals. While some governments’ champion such systems as a method to fight crime, the use of social control is not without controversy, since it raises serious “legal and ethical challenges relating to privacy” (Završnik, Citation2017). Disinformation campaigns are viewed as sinister state actions since they block independent information and muddle the facts in order to create a narrative interpretation of events that only benefits the disinformation producer. The utilization of AI bots along with trolls to create an alternative internet news keeps the mis/disinformation actor visible. Such strategies known as “flooding the zone” (Ramsay & Robertshaw, Citation2019) or just “flooding” (Roberts, Citation2018) are heavily used by nation states (Pomerantsev, Citation2019). Bots along with trolls are also used by state actors to “start arguments, upset people, and sow confusion among users” (Iyengar & Massey, Citation2019) in countries that the state actors consider their adversaries (Bessi & Ferrara, Citation2016).
In terms of the collective impacts of social media, there is a need to guarantee individuals fair access to knowledge regarding the source of information and the reason they are being exposed to this information. Towards this aim, the concept of Communication Platform Neutrality (CPN) is proposed to promote well-being for users of social media. CPN is a set of principles and a research agenda focusing on how to achieve fair, equitable, and unbiased information creation, communication, and consumption for the benefit of society. Many researchers have argued that social platforms are inherently non-neutral (Berghel, Citation2017; Chander & Krishnamurthy, Citation2018). In proposing CPN, platform neutrality is adopted with a focus on principles that tackle the issue of information consumption by individuals. This work contextualizes principles that guarantee individuals a fair access to knowledge regarding the source of information and the reason they are being exposed to this information. These principles seek to counterbalance the overarching control over the information consumption by individuals that companies like Meta and Twitter currently have by giving control to the user. details the principles of Communication Platform Neutrality along with its foremost research questions.
Table 2. Principles and research questions for communication platform neutrality.
2.3. Summary of emerging requirements
At the heart of this first challenge, expanding into the other 5 challenges, is a question of HCI evolution: How might we apply current knowledge and expand future knowledge such that we can systematically study, design, evaluate, and improve AI-enhanced technologies with primary attention to their human benefits and harms? To achieve HCAI (Shneiderman, Citation2022), efforts must go beyond optimizing algorithms to designing interactions that satisfy both technical and humanistic concerns (Cai, Reif, et al., Citation2019).
2.3.1. Redefining “usability”
Developing the capabilities for avoiding harms and enhancing experiences with AI requires a range of technical efforts. At the individual level, the scientific understanding of “usability” must expand significantly to address the unique features and impacts of AI and the special issues of undue influence, dynamic reliability, and errors of automation. In the face of tools that can appear intelligent, there is an urgent need for human controls, feedback, and affordances that allow users to calibrate their trust of AI outputs and exert control over its actions. AI also exhibits adaptive or learning behaviors which, while incredibly useful, also means that the performance can change over time, and it can react unpredictably to new inputs or stimuli. Designers must provide users with the ability to manage this with increasing capability, confidence, and comfort.
2.3.2. Human-centered evaluation and remediation
Perhaps the most urgent technical requirement involves evaluation and remediation. Given the potential harms along with the relative “newness” of these tools, there must be an ability to evaluate them from a human-centered standpoint, to consider the impacts on individuals as well as the collective, both short- and long-term, and to be able to correct them. The cost and difficulty of redesigning errant AI must be reduced significantly, and the cycles of improvement shortened. Naturally, the keystone of all these efforts is the ability to elevate their importance to designers, engineers, consumers, and regulators such that HCAI is both in high demand and insisted upon by all stakeholders. All stakeholders should be able to, at some basic level, evaluate and challenge the appropriateness of the AI tools they experience. Along with that must be a well described pathway towards satisfying this demand via human-centered, research-based design principles, processes, and evaluations.
2.3.3. Disambiguating accountability
While industries typically have existing standards and regulations that must be adhered to, they will require refinement to address the unique features and impacts of AI. As discussed earlier with risk shifting there can be an ambiguity of accountability in terms of what person or group is accountable, along with a lack of tools to successfully bear that accountability. It is crucial that designers explicitly consider these accountabilities, and that purchasers and users learn to expect clarity and fairness in terms of risks and responsibilities (see Section 6).
There is a need for researchers, designers, regulators, purchasers, business leaders, and users alike to develop a shared, clearly articulated vision of what “responsible” AI (see 3. Responsible Design of AI) is along with standards for security, privacy, interaction design, governance, all of which might be cohesively held within an overarching framework.
3. Responsible design of AI (challenge 2)
3.1. Definitions and rationale
Responsible design of AI is an umbrella term for various efforts to investigate legal, ethical and moral standpoints when using AI applications. Responsible design of AI possesses the systematic adoption of several AI principles (Barredo Arrieta et al., Citation2020), and is also often used to describe a governance framework documenting how a specific organization is addressing potential negative externalities around AI. In this case responsible AI is seen as a practice of designing, developing, and deploying AI with good intention, allowing companies to engender trust by employees, businesses, customers and society. The goal of a responsible AI framework can be to establish a governance structure to ensure a responsible use of AI technology but also to run an expanded marketing strategy to positively influence the corporate image, or a mixture of both.
Efforts in the area of responsible design of AI are increasing, as processes become more and more automated. The introduction of advanced ML methods leads to a moral wiggle room when it comes to questions concerning accountability and pivotality. Actions become increasingly unattributable to a single entity or person. In other words, the ultimate responsibilities for actions of AI implementations become increasingly opaque with the growing use of advanced ML methods. Due to this development, not only the technical responsibility but also the interaction in the legal and ethical context must be considered in order to ensure a responsible use of the technology. The technology must therefore be considered not only in terms of its efficiency but also in the context of its usage. With the introduction of advanced ML methods, it becomes increasingly important to understand how a decision was made and who is responsible for it. This broadening of the evaluation spectrum represents a new approach to the development and use of ML methods, prompting the need for a more strategic view on legal, ethical and behavioral topics defined and developed within a responsible AI design framework.
3.1.1. Subcomponents of responsible design of AI
Responsible design of AI coalesced around a set of different concepts covered by the term. Core concepts for responsible design of AI are summarized graphically in . While the concepts may go by different names, the key principles are the same. The guidelines behind Responsible design of AI establish that explainability, fairness, accountability and privacy should be considered when using AI models. Trustworthiness, data protection, reliability, security, and human-centeredness are also other terms that are frequently mentioned when it comes to responsible design of AI (Barredo Arrieta et al., Citation2020).
3.1.2. Summary of AI initiatives and standards
A growing number of non-profit organizations focused on governance (Responsible Artificial Intelligence Institute, Citation2022), technology companies developing and promoting tools and processes for responsible design of AI (Google AI, Citation2022; Microsoft, Citation2022), individual countries setting fiscal and research goals (Dumon et al., Citation2021; Roberts, Citation2018; Stanton & Jensen, Citation2021), as well as larger regions (Martin, Citation2021), and global organizations (Organisation for Economic Co-operation & Development, Citation2021; United Nations Educational Scientific and Cultural Organization (UNESCO), Citation2021) have recommended procedures for ensuring responsible design of AI. The most common principle recommended by these organizations is ensuring AI explainability, such that stakeholders including citizens, regulators, domain experts, or developers are able to understand AI predictions (Arya et al., Citation2020). Fairness, including accounting for potential bias (Mehrabi et al., Citation2021) and ensuring privacy through appropriate cybersecurity safeguards as prerequisites are also frequently recommended. Finally, ensuring that a thorough ethical analysis is performed throughout the AI development life cycle is also commonly recommended by these groups. The HCAI community has an opportunity to lead in the standardization of these recommendations and processes by coordinating across non-profit organizations, technology companies, countries, regions, and global organizations to ensure AI is responsibly developed for the world.
3.2. Main research issues and state of the art
3.2.1. Accountability and liability – moral and legal
During the last decade, a discussion concerning the possible accountability and liability of AI driven autonomous systems has gained momentum in the realms of the legal and philosophical literature. Legal accounts on this topic naturally concentrate on questions of possible liability gaps and how to ensure the compensation of victims following AI offenses. Scholars of philosophy and psychology meanwhile point out that responsibility and retribution gaps are likely to open up, if AI errs and causes harm, even if a human is found liable for an offense and victims are compensated.
The question of assigning legal liability in the event of a failure of AI is complex. The legal system distinguishes between private and criminal law. In private law, entities other than natural persons can be considered an actor and found liable. Therefore, private law can integrate the liability of AI more easily than criminal law (Gless et al., Citation2016). In recent years, a broad discussion has developed on whether AI can even be granted the legal status of a person under certain circumstances. This would equip AI also with rights, such as freedom of speech and freedom of religion, which might already be possible under current U.S. law (Bayern, Citation2016). In criminal law the liability of nonhuman agents is internationally contested (Gless et al., Citation2016). U.S. law allows for the prosecution of nonhuman agents such as corporations (Wellner, Citation2005). Cases in which an AI’s involvement in an offense may be considered under U.S. criminal law (Hallevy, Citation2010) include (1) an AI as an innocent agent of a perpetrator, (2) an AI committing an offense because a negligent human failed to act on the foreseeable consequences of its use, and (3) an AI itself being liable. However, whether it will ever really be possible to ascribe the criminal intent that is a prerequisite for criminal liability to an AI system is still contested (Osmani, Citation2020). Until now, robots are, despite certain degrees of freedom in their attributes (e.g., in communication, knowledge and creativity), frequently recognized as a product at law and are thus considered under product liability (Bertolini, Citation2013; Hubbard, Citation2014). Practical law lacks precedents of cases that involve highly sophisticated AI systems, and as such, many of these disputes have stayed theoretical for now. Corporations that create sophisticated AI systems normally are keen to settle cases stemming from accidents involving the AI systems (e.g., autonomous vehicles or advanced driverless assistance systems) outside the courtroom (Wigger, Citation2020).
Ethicists and psychologists focus their debate concerning the consequences of AI offenses on the possibility of responsibility and retribution gaps that might follow, or even exceed, liability gaps resulting from the delegation of decision making authority to AI systems. From a psychological perspective, when confronted with perceived injustice, people tend to want to identify the perpetrator of the harm they suffered and punish his or her wrongdoing (Carlsmith & Darley, Citation2008). The question arises whether a proclaimed liability and a compensation of some sort may satisfy the retributive needs of victims if the entity they experience as the perpetrator is an AI system. The human victim will most likely not accept the AI system as a suitable recipient of retributive blame or legal punishment due to an AI-system’s lack of self-awareness and moral consciousness. However, it is questionable whether the punishment of an entity responsible for the creation of the machine can compensate victims for the lack of direct retributive punishment, especially if the responsibility for the actions of the AI system on the part of its creators is diffused. One important factor in the diffusion of responsibility with respect to AI systems is the self-learning nature of modern systems and the creation of “black boxes.” In addition, technical goods are produced in the context of highly diversified transnational supply chains, which complicates the assignment of responsibility. From an ethical and psychological perspective, this creates a problematic disconnect between modern reality and the human urge to retaliate (Danaher, Citation2016).
3.2.2. Explainable AI
As AI increasingly shapes our view of the world, it influences individuals and social groups in their introspection and perception of others by: (1) providing information for human-human interactions, which is processed, aggregated and evaluated; and (2) interacting directly in human-machine interactions. Thus, explainable AI has – for good reason – become a prominent keyword in technology ethics (Mittelstadt et al., Citation2016; Wachter et al., Citation2017). Two main drivers of the opacity of AI generated results are the so-called “black box” of the self-learning algorithm and the influence of human subjectivity on the design process. HCAI will have to be designed in a way that takes all human factors of its different stakeholders into account. For instance, a socio-technical approach in which the technical AI development and the understanding of subconscious and implicit human factors evolve together, has been proposed to incorporate the “who” in a systems’ design (Ehsan & Riedl, Citation2020). The authors concentrate herein on “who” the user of an AI is and the social factors which surround the AI, once it is deployed. An explainable HCAI will also have to incorporate “who” influenced the system prior to its deployment, since an AI is already inherently value-laden in its design phase (Mittelstadt et al., Citation2016). Thus, as with any processed data, the information provided by an AI has to be interpretable in later life cycle stages through the lens of the influences it was exposed to in earlier stages.
Human-AI interactions will yield results that exceed human performance, to reach the expected added value, if AI is well calibrated to balance human trust and attentiveness in the interaction. HCAI will have to be designed so that users do not blindly trust the machine and carelessly ignore important information on the system’s performance and how the output is possibly biased or distorted by statistical variance. Humans must be enabled to critically question an AI’s output. On the other hand, human nature also requires that certain information not be disclosed openly. Behavioral sciences document the tendency to ignore information, if this helps to preserve a certain self-image (Grossman & van der Weele, Citation2017). In many cases the possibility of strategic ignorance might be just as important for the well-being of the user. Hence, for HCAI, transparency cannot be promoted without any limitations since a complete opaqueness and explainability may repulse people in some applications.
A distinction should be made between the transparency and explainability needs of end users and model developers. For example, while model developers are interested in the in-depth explanations of the underlying model attributes, end users are interested in more general explanations. The challenge is to satisfy both needs. The development of systems that satisfy the needs of both user groups is a major challenge and most interfaces are tailored either to model developers (Gunning & Aha, Citation2019) or end users (Mitchell et al., Citation2019). The development of such systems focusing on both groups is still in its infancy.
Without doubt, a high human need for explainability in AI applications based on interpretable transparency arises in the event of a failure of the system, as well as for AI applications that make ethically relevant decisions, as in the event of ethical dilemmas (e.g., a distribution of scarce resources) between different human parties. Here, affected people will want to be able to convince themselves that certain mistakes are not repeated in the former case. In the latter case, they will want to ensure that distribution outcomes are based on decision making mechanisms that are judged to be fair, legal and ethical in society. The importance of explainability in cases of ethically relevant decisions through AI becomes especially clear in light of possible responsibility gaps (see section 3.2.1 Accountability and liability – moral and legal). If AI systems fail and the need for explainability is left unanswered this may not just diminish trust in AI systems, but also in the institutions behind the AI (López-González, Citation2021). In other words, people’s societal trust may be challenged. To counteract such developments, institutions should be held as accountable for the actions of algorithms they deploy, or use, as they would be for the same events, if caused manually. This will set incentives to strive for explainability already in the development process of HCAI, which corresponds to the core concept of such applications.
3.2.3. Fairness
Fairness is defined as “the quality of treating people equally or in a way that is right or reasonable” (Cambridge University Press, Citation2022). In the legal domain, fairness is defined in two main areas: (1) disparate treatment (Zimmer, Citation1995) is a direct discrimination that happens when individuals are intentionally treated differently; and (2) disparate impact (Rutherglen, Citation1987) is an indirect discrimination that happens when individuals are unintentionally treated different under a neutral policy. Researchers categorize biases that create unfair AI deployment cases into three categories: data, model, and evaluation biases. Data biases comprise biases in the dataset due to unrepresentative data collection, defective sampling, and/or wrong data cleaning. The most common examples of data bias are historical bias, selection bias, representation bias, measurement bias, and self-report bias. Model biases, on the other hand, are observed when an AI algorithm does not neutrally extract or transform the data regardless of the biases in the dataset. Sources of model biases include several sources (Danks & London, Citation2017): AI algorithms might be inherently biased to differential use of information to maximize the accuracy rather than focusing on morally relevant and sensitive judgments, which yield the utilization of a statistically biased estimator; the use of transfer learning techniques in which AI is trained on a specific context but employed outside of its context without considering the new context’s feature space; and aggregation bias – wrongly assuming that the trends seen in aggregated data also apply to individual data points, algorithmic bias – wrongly extracting or transforming the data, and group attribution bias – assuming that a person’s traits always follow the ideologies of a group (Ho & Beyan, Citation2020). Further biases are the hot-hand fallacy – the tendency to believe that something that has worked in the past is more likely to be successful again in further even if there is no correlation, and the bandwagon bias – the tendency for people to adopt certain behaviors, styles, or attitudes simply because others are doing so. Lastly, evaluation biases can also arise when a thorough evaluation is not carried out or an inappropriate performance metric is chosen for the evaluation. The most common examples are rescue bias – selectively finding faults and discounting data, deployment bias – system interpreted improperly during deployment, and the Simpson’s paradox – biased analysis of heterogenous data by assuming associations, or characteristics observed in underlying subgroups are similar from one subgroup to another.
The most common measures of algorithmic fairness in AI tasks are disparate impact, demographic parity, and equalized odds. Among these, disparate impact and demographic parity aims to quantify the legal notion of disparate impact by considering true positive rates for different groups (Calders & Verwer, Citation2010; Feldman et al., Citation2015). Equalized odds, on the other hand, is proposed to quantify differences between predictions for different groups by considering both false-positive rates and true positive rates of the two groups (Hardt et al., Citation2016).
Since the unfairness in the deployment of AI algorithms may stem from a bias in data preparation, modelling, and/or evaluation parts, different pre-processing, in-processing, and post-processing strategies are proposed to enhance their fairness, respectively. In pre-process fairness-enhancing strategies, data is manipulated before training the AI algorithm to make it fairer. These preliminary manipulations can be either done by completely changing (Kamiran & Calders, Citation2012) or partially reweighing (Luong et al., Citation2011) the labels of training data which are closer to the decision boundaries to reduce the discrimination, or by modifying the feature representations rather than labels (Calmon et al., Citation2017). Recently, more sophisticated techniques such as generative adversarial networks are used to produce synthetic data to be augmented into the original dataset to improve demographic parity (Rajabi & Garibay, Citation2021). For in-process fairness-enhancing strategies fairness of the AI algorithm is yielded during the training time; thus, it requires modification in the architecture of the AI algorithm itself. In this context, one of the most intuitive strategies is to add a regularization parameter in the objective function to penalize the mutual information between sensitive variables and estimates (Kamishima et al., Citation2012). Similarly, numerous studies integrated fairness measures as a constraint in the optimization functions of logistic regression models (Zemel et al., Citation2013), variational autoencoder models (Louizos et al., Citation2015), kernel density estimation models (Cho et al., Citation2020), or through introducing a stability-focused regularization term in different AI algorithms to tackle the fairness-accuracy trade-off (Huang et al., Citation2019). Another recent study demonstrated that quantum-like techniques have a promise to prevent unfair decision making by amplifying the accuracy of extant AI algorithms, especially under uncertainty (Mutlu & Garibay, Citation2021). In post-process fairness-enhancing strategies, modifications are made after running the AI algorithm by assigning different thresholds to change the decision boundaries of algorithms (Corbett-Davies et al., Citation2017).
3.2.4. Ethical AI
To discuss artificial morality with a focus on responsible design of AI, there is a need to differentiate between two extremes of human-centeredness: the AI in an autonomous system (i.e., by definition an AI acting without direct human control) and recommender systems, which keep the human-in-the-loop by asking the human to make a final decision or action (see Section 7.2.2. Human-AI system interactions at work). As discussed in earlier (see Section 3.2.1. Accountability and liability – moral and legal) the deployment of HCAI should encompass the attribution of responsibility to human stakeholders of the AI, since an AI system does not offer any opportunity for meaningful retributive actions. While it may seem most concerning from an ethical, as well as psychological view that under certain legal models an AI may be considered itself as liable (Hallevy, Citation2010), already the responsibility ascription to human users of recommender systems may prove challenging, if not legally so, then at least in a moral sense. First, users who act upon the advice of a recommender system might themselves feel less responsible for the outcome of their actions. Individual human deciders can diffuse responsibility in a decision process with other people. This psychological response to a collaborative decision may prove to be even stronger, when people rely on recommender systems, than if they rely on human advisors. Human decision makers seem to adhere more to algorithmic advice (Logg et al., Citation2019) and are reluctant to acknowledge how strongly the machine’s advice influences them (Krügel et al., Citation2022). Secondly, society might encounter barriers to view human deciders, who follow the suggestion of a recommender system, as responsible as it would view a non- or merely human-advised decider (Braun et al., Citation2021; Nissenbaum, Citation1996).
The toughest challenge to responsibility ascription is, however, the deployment of autonomous systems. For instance, while some industries have specific regulations for safety critical devices (e.g., medical and pharmaceutical industry) the technology itself is not yet regulated, opening a moral wiggle room when it comes to responsibility attributions. Here, the “problem of many hands” becomes especially evident, when the acting entity is an AI, in which the prerequisites for its ethically relevant decisions are embedded a priori to the application by designers, engineers and adopters along its manufacturing process. Research has shown that when it comes to shared decision making, the type of partner with whom the decision is made affects the perceived responsibility for the decision, the perception of the choice, and the choice itself (Kirchkamp & Strobel, Citation2019). Therefore, it is advisable for HCAI to ensure that the responsibility for ethical decisions is clearly attributed to the production chain of the AI. While ML-based applications are not yet regulated, a first step is to clearly assign responsibilities and liabilities for the technology.
For the actual programming of moral capacities into AI systems, two approaches are mainly considered: a top-down and a bottom-up approach (Wallach & Allen, Citation2008). Bottom-up approaches, on the one hand, are based on the self-learning capacities of the AI, but may be strongly contested because of their unpredictability (Misselhorn, Citation2018) and the challenge to trace a morally relevant decision back to a human decision maker or programmer. A top-down approach, on the other hand, formulates moral principles, which are then implemented into the system. Discussions of which moral principles may be of relevance revolve mostly around the principles of deontological and utilitarian ethics, as well as Asimov’s laws of robotics (Misselhorn, Citation2018). A special interest lies in the decisions of autonomous systems in moral dilemmas. These have been discussed in the realm of autonomous vehicles during past years (Awad et al., Citation2020). Several scholars have argued that these kinds of dilemmas, in which an autonomous vehicle must decide whom to save and whom to harm in accident situations may not be technically applicable or at the forefront of autonomous vehicle ethics (De Freitas et al., Citation2020; Himmelreich, Citation2018). The COVID-19 pandemic has sadly shown that such life-and-death decisions might not be restricted to accident situations in civilian life (Bodenschatz et al., Citation2021; Truog et al., Citation2020); they may also arise for medical AI systems at some point, if these are to distribute scarce resources (Hao, Citation2020).
3.3. Summary of emerging requirements
3.3.1. Policy requirements
Implementing responsible design of AI is a growing concern with prodigious societal importance, and scholars from different disciplines try to identify what it entails and how it may serve to elevate human trust in AI systems and their ability to reap the benefits of AI, while they do not feel absolved of their own responsibility. Responsible AI itself is an umbrella term that encompasses many concepts, its boundaries are blurry, and concepts are often interchangeably labeled and used as semantic synonyms. It is therefore difficult to find a unique definition that covers the concepts of responsible design of AI in decision making. Establishing an appropriate definition of responsible design of AI and the concepts it encompasses however becomes increasingly important as it is imperative to define concepts to mitigate negative impacts of AI applications. Therefore, a better-contemplated taxonomy for a responsible AI definition is one of the most significant milestones in advances towards HCAI and to inform the development of policy guidelines.
Policymakers must also address the following issues, which are becoming increasingly important. Semantics is not only critical in defining a responsible AI framework, but as applications become more autonomous, the wording used to advertise the applications must determine the extent to which the human user can delegate their own responsibility to the system. It is necessary to examine, for different levels of applications, what terms lay people intuitively associate with the actual technical occurrence. For example, there is an ongoing legal discussion whether Tesla’s “Autopilot” may be labelled this way, because of the expectations towards the technical system that this word evokes, or whether this designation should be reserved for higher levels of automation (Taylor, Citation2020, Jul 14).
In addition, urgently pending policy implications can be derived from a discussion on the concept of ethicality within the responsible design of AI framework. Policy makers need to address societal concerns about moral dilemmas that AI systems may have to address in the near future. Especially in democracies, it may lead to societal unrest, if possible life-and-death decisions are not addressed by elected representatives but seemingly left to individuals and corporations to decide. Although responses to simplified dilemma situations and behavioral experiments should not be the basis for legal or ethical regulations, they may serve to gain insights into common intuitions on these matters (Awad et al., Citation2020; Bodenschatz et al., Citation2021; De Freitas & Cikara, Citation2021).
3.3.2. Technical requirements
Human nature makes it a challenge to encourage stakeholders along the AI life cycle to own their responsibility for their influence on the algorithms and decisions they make under the influence of an AI system. For this reason it is of utmost importance that certain technical requirements are fulfilled. These include requirements about (a) the UX design of AI interfaces, (b) a proper technical definitions of the fairness, explainability, and liability indicators, and (c) a holistic approach embedded into the system to make sure that explainability requirements are met.
More specifically, emphasis within a responsible design of AI framework should be on the interface for AI applications to meet the requirements of HCAI. The requirements need to evolve around physical and psychological human boundaries. Thus, they need to be defined by interdisciplinary consortia. The composition of the interface needs to interactively mitigate human biases and heuristics. A special emphasis should be on the mitigation of those biases that lead to a diffusion of responsibility with the autonomous system or between the human entities which the system connects.
Furthermore, especially for autonomous systems without human oversight, a determination of the appropriate measures and fairness indicators is of utmost importance. Since AI algorithms are trained to be fair with respect to a specific fairness measure, the selection of the proper measure may affect the disparity to a significant extent. As an analogy to the trade-off between sensitivity and specificity in statistics, it is critical to determine whether one should aim to maximize the equal probability of benefit or minimize the harm for the least advantaged populations. Additionally, understanding potential sources of unfairness is the key milestone to providing fair solutions in the deployment of AI; thus, an effective strategy can be employed to mitigate the unfair AI estimates for different subpopulations and individuals after the first challenge is overcome. Lastly, because AI and ML algorithms often rely on large amounts of data, availability of fairness-aware datasets to train these algorithms and benchmark datasets to test to ensure that AI systems produce fair and equitable outputs is important (Quy et al., Citation2021). There are efforts in government and private institutions to create and make these types of datasets available for training and testing of AI systems that impact various aspects of life such as finance, justice, health care, education and society in general (Brennan, Citation2021, Oct 8).
We acknowledge that humans are prone to bias and that algorithms designed by and using data collected by humans can preserve and exacerbate these biases. An important emergent requirement is to conduct research on how diverse teams of individuals can achieve designs that are as unbiased as possible or at least aware of the potential biases introduced.
Explainability must be ensured where users demand it. AI must be easily accessible and comprehensible in the sense of being understandable. For this, integration of explanation mechanisms into the direct user experience is indispensable. The integration into the UX design of an AI application must be intuitive, not only in its operation but also in its wording. It must be possible for the human user to bypass this information in certain applications (see Section 3.2.2 Explainable AI). The biggest challenge for an explanatory capability in HCAI applications will be to calibrate the trade-off between harmless individual system adaptations and harmful interventions promoting human weaknesses (e.g., the human tendency to diffuse responsibility).
In summary, the requirements emerging from a responsible design AI framework call for an interdisciplinary approach towards the technical design of HCAI. The systems of the future need to directly evolve from a framework that centers around considerations of human limitations. These limitations influence the way people make use of these systems. A framework for HCAI implementations also needs to take into consideration which social upheavals these systems’ deployment might entail if the human responsibility behind the AI is blurred. A taxonomy for a responsible design of AI definition needs to be established and semantics around the HCAI need to be adjusted to speak to human intuitions concerning the right interpretation of a machine’s capabilities. Social concerns need to be addressed in legal and ethical regulations. Legislative regulations for external audits of AI applications need to move from vague to concrete and applicable requirements and need to address the use of advanced and adaptive systems. They also need to ensure that methodological safeguards for the appropriate measures and fairness indicators are always met.
4. Privacy (challenge 3)
4.1. Definitions and rationale
The crucial foundation of a functioning AI is the data on which it is based. While AI may have broad and varying definitions, the applications that are most exciting for their capabilities in automation, enhancing human knowledge, and supplementing human actors, are those which draw most deeply on robust and representative datasets. This holds for the broad spectrum of AI applications – those embodied and non-embodied; for the practical or impractical; for life-saving measures or video games. There are few aspects of human life to which AI cannot or will not be applied, fuelled by the “big data” boom of the last decade.
Data is an abstraction of the fundamental building blocks that make up the way we perceive the world: the colors we detect, shapes we recognize, distances we travel. Narrow AI are trained, tested, and produced to act upon various data inputs – to “speak” to a child, assess optimal solar panel direction, or make tea. While the data does of course exist outside of the world of AI, it must be captured in order to train, test and produce the AI. Types of data are theoretically immeasurable, and while many may be largely independent of direct human interaction, such as the distance and travel of interplanetary bodies, the majority of AI applications are based around humans and their interactions. This is for multiple reasons: replacements and supplementation of labor; creation of new markets and products; and the sheer variety of human interaction. In any case where an AI is intended to interact with a human - be it physically, economically, or digitally - the AI must be trained on information regarding humans and human behavior. Human data comes, axiomatically, from humans.
HCAI presents the proposition of harnessing the potential power of AI in a way that benefits humanity, drawing on tools that “amplify human abilities, empowering people in remarkable ways,” calling for reliable, safe, and trustworthy design (Shneiderman, Citation2022). As evidenced by the other writings herewith, this is no simple task: ethical and sustainable collection, implementation, and use each present unique challenges and pitfalls. This is no less true for the data upon which AI depend: data about humans fundamentally affects both the humans about which the data is collected, and the humans of the system in which the AI will be deployed. This produces two major categories of impact: those relating to accuracy and robustness of data – bias and discrimination (see Section 3.2.3: Fairness).; and those relating to data subjects – privacy.
Conceptions of privacy vary from individual to individual, as well as by technical and legal definition, and have been summarized as six conceptions: the right to be left alone; the right to limit access to the self, including the ability to shield oneself from unwanted access by others; the right to secrecy, including the concealment of certain matters from others; the right to control over personal information, including the ability to exercise control over the information about oneself; the right to personhood, including the protection of one’s personality, individuality, and dignity; and the right to intimacy, including the control over, and limited access to one’s intimate relationships or aspects of life (Solove, Citation2002). Given the potentially broad characteristics of data for AI, each of these may be drawn upon: individuals or groups may not wish to have their data collected, nor others’ data used in relation to them. They may wish to conceal certain data, or to control its dissemination and audience. They may feel they are reduced to a set of data or a series of numbers by being incorporated into a larger system of data, or simply that they do not wish the multitudinous aspects of their life, including relationships, to be exposed to largely corporate entities to exploit. Most worryingly, individuals or groups may have a limited conception of what data is held about them, either siloed or in combination, and how that data might impact them. While all of these concerns are valid, for the purposes of HCAI, this discussion will focus on: how data is used by AI, and how it may be used against individuals and groups. These hinges on: the consent of collection and use; understanding of types and extent of impact both by the data subjects and the data holders; and what the data can be used, for what purpose, and for how long.
4.2. Main research issues and state of the art
4.2.1. Case study: social robots
How, then, could these concerns play out in real-time? This of course depends on the application of the AI. While harms of digital AI are equally as important as their embodied counterparts, their effects on privacy are best addressed in the context of their specific use and broader effects: for example, social media algorithms have been much discussed in the context of psychological harms such as addiction and self-esteem (Błachnio et al., Citation2016) alongside such concerns as political radicalization (Van Raemdonck, Citation2019) and sex trafficking (Latonero, Citation2011). However, there is considerable concern too alongside the physicalization of AI – its placement with and among humans. Beyond merely tripping over the Roomba, social robots provide an interesting case study for privacy.
Social robots are those AI-enabled digital counterparts with whom humans interact. This naturally encompasses a broad range of applications. Humanity’s proclivity to delegate labor is second perhaps only to its desire for companionship: beyond even the domestication of animals, humans anthropomorphize and are affectionate towards inanimate objects (Tahiroglu & Taylor, Citation2019), and have been since long before robots – one would be hard-pressed, for example, to find an unnamed boat docked at a marina, even though they may equally have an identifier similar to a license plate. Social robots appeal to many, either for their intended function or a fostered sense of affection.
Social robots are not, however, created equal. Depending on their intended purpose, social robots will have different methods of data gathering, processing, and storage, and even these can vary. For example, an AI lawn mower might have a complex machine-learning model to interpret a camera feed to precisely mow a new or previously-mapped lawn. Conversely, it might require a set of electrical boundary fences to “bump” into to turn. While a lawn mowing AI may raise few privacy concerns, particularly as there would be no reason to give it audio recording capabilities, robots in the home have this same variation but with far more potential information to gather.
Consider an entirely theoretical assistive living robot. The function of the robot is strictly human-centric: it is intended to help the human by bringing them water, reminding them to take medication, and alerting appropriate parties if the human has fallen. Consider, then, the functions that the robot requires: it must be able to be spoken to via natural language in order to be asked for water. It must be able to move, to bring the water. It must have data storage in order to record what medications and when they should be taken. It must have a camera to detect whether the human has fallen. This means the AI has at the very least, audio, visual, physical, and health data about the human which interacts with it, alongside, very likely, audio and visual data about the other humans who enter the living space. Such collection touches on many of the aforementioned privacy areas: while the owner or human occupant of the house may consent to be recorded and have their data used, they may have less understanding of the potential harms thereof; and visitors may not have consented at all. Humans recorded may or may not understand to whom access is limited, allowed, or insufficiently protected from. There may be an insufficient understanding of what the data collected reveals: while our innocuous toaster may struggle to harm a human physically, it may give information on their daily hours and habits, as well as their absence. Control over information is decided almost entirely by the manufacturer – there is not necessarily any way for a consumer to remove, revoke, or adjust data collected. Consumers are likely to be treated at best as an additional datapoint, and at worst as a target. Even the mobility of the robot can infringe upon privacy: it may enter private spaces of the home, or enter and record areas unexpectedly, limiting privacy unnoticed or unheralded.
Stakeholders – including users, policymakers, and courts – must demand answers of producers regarding robots’ design: how securely is data stored? Is it stored in its original form? Is it stored centrally, or de-centrally? Who has access to the data, and under what circumstances? Who is allowed, and how, to change data? Questions such as what privacy-enhancing technologies (PETs) have been used must be asked, and what aspects of privacy by design: is the design user-centric? Was it designed to minimize collection, or deal with data once collected? While privacy is an initial question in deciding what information the robot can collect, what data is collected comes to the mercy of what security measures have been implemented, and to what effect.
There are many privacy questions raised for the assistive living robot as described. The cited areas of privacy are a helpful lens with which to examine the potential implications, with the combination of several factors which can be read similarly (Solove, Citation2002); see .
Table 3. Implications of Solove’s privacy questions applied to social robots.
4.2.2. Data is the key
Data is omnipresent in the creation and use of AI. AI must be created, trained, and tested on applicable datasets. The usefulness of the dataset depends on four attributes: “its volume, velocity, variety, and veracity,” (Yanisky-Ravid & Hallisey, Citation2018) also known as size, whether data points remain relevant, combination of sources, and accuracy. While synthetic data may be created, there is an inevitable need for human-origin data, alongside, potentially, equipment-generated data. Data collection from humans is a regulated but varying area: each jurisdiction fields a different set of rules and regulations around its collection. The European Union General Data Protection Regulation (GDPR), for example, requires that consent be “opt-in,” rather than “opt out,” meaning that the data subject must actively consent to their data use rather than be passively informed of its use. Other GDPR requirements such as “legitimate interest,” which require that data be processed “only if and to the extent” that processing “is necessary for the purposes of the legitimate interests controlled by the controller or by a third party, except where such interests are overridden by the interests or fundamental rights and freedoms of the data subject,” (GDPR Article 6(1)(f)) suggest a greater level of protection for personal data. Leaving to more targeted papers the question of the effectiveness of such measures, it is important to note that there are concerns beyond first-level or direct collection, or even its denial: the metadata or secondary level data on the original can be abstracted into use for AI, to levels potentially unaddressed by data protection laws. This is naturally all the more problematic if done by actors with no legitimate or legal purpose in collection. In addition to jurisdictional differences in managing privacy for human data, sectors have varying degrees of sensitivity as well. For example, the healthcare domain has particular concerns around storing and processing personally identifiable information relating to an individual’s health.
Most concerningly, information can be used in ways that may harm or negatively influence the lives of the data subjects. While data gathering has affected human experience prior to AI, particularly in the digital era, the expansive types of data and computing power means that models are increasingly produced, accurately or inaccurately, based on human subjects. The slow erosion of various forms of personal information does not necessarily signpost the inherent loss of privacy it provides, and humans may not care, on an individual level, to protect their privacy were it to do so: the “privacy paradox” provides that while internet users purport to be “highly concerned about their privacy and the collection and use of their personal information,” they act in ways that show minimal care to protect it and reveal their information for disproportionately low reward (Kokolakis, Citation2017). Even lawfully obtained information can be used for purposes as mundane as marketing, to as concerning as spamming, hacking, transmitting viruses, and government monitoring (Yao et al., Citation2007).
This is not to say that data is on display for all to see. Privacy measures are taken to control access and control over data. While not all privacy measures are proportionate to the potential severity of a data leak, it would be fair to say that broad trends thereof exist – banks protect customer data with greater measures than arcade leader-boards. HCAI takes privacy very seriously – not only for the types of data gathered, but for the depth and breadth of that data. However, measures of protection will still vary between applications. For example, an AI child’s toy collecting only sensor data will need to require minimal privacy protections, as minimal personal data has been collected. More broadly, there are philosophies and design principles that put privacy at front and center, and which are beginning to be incorporated into HCAI, such as “privacy by design.” Privacy by design follows seven principles, and states that privacy should be (Cavoukian, Citation2009):
proactive rather than reactive, preventative rather than remedial
the default setting, rather than the exception
embedded into design
avoiding the false dichotomy of preventing function, but rather accommodating all legitimate interests
providing security across the course of the technology’s lifecycle
visible and transparent
user-centric
These are clearly compatible or directly parallel to the concerns of HCAI: the need to put the human in the equation first, by protecting their data and interests in a broad range of concerns. Beyond principles of design and their conceptual frameworks, PETs provide additional protections. A variety of PETs have been proposed and utilized, including “anonymizers, identity management systems, privacy proxies, encryption mechanisms, filters, anonymous credentials, commitment schemes, [and] sanitization techniques” (Le Métayer, Citation2013). PETs are not generalist applications, and must be taken on a case-by-case basis to determine the most appropriate; but most formal PET models can be grouped into three categories: language-based approaches; decentralized security models; and privacy metrics used to measure the level of security provided by the algorithm in question (Le Métayer, Citation2013). Appropriateness has a broad range of factors to consider, including the sensitivity of the data in question. For HCAI in particular, it must be emphasized that the individual in question’s choice and preferences around their data and its sensitivity form the bedrock of what appropriateness in privacy really means.
There are protections as to how data may be gathered, when, from whom, and under what conditions. However, the initial gathering stage is far from the only time at which privacy is at stake. Not only might the new assumptions and connections be made within the collected data by the AI, but the initial information might be combined with other datasets, or indeed by an AI itself that is “capable of independently searching for new relevant datasets from areas such as social networks, internet sites, blogs, and other data that exists online” (Yanisky-Ravid & Hallisey, Citation2018). Combination or recombination of data sources can provide an increasingly explicit picture of individuals or subgroups, with or without their knowledge. For example, ancestry DNA databases are now being used by police forces in forensic analysis of evidence (Van Ness, Citation2020, Feb 20).
Essentially, the initial capturer of data may or may not have had a lawful and ethical reason for doing so, and that information may be used for purposes which do or do not benefit the data subject, but which in all likelihood benefit the data capturer either directly or in further sale. However, they are not the only actor that matters to the data subject – other actors seek to access data either directly or indirectly. While security does not relate solely to privacy, the protection of data – and privacy thereof – is a crucial aspect of HCAI security.
4.3. Summary of emerging requirements
In summary, the very nature of AI makes it a threat to privacy throughout its lifecycle, including: the initial collection of human data; the meta-data extracted; the potential unheralded connections created by AI, particularly where more data is drawn; the need to safeguard stored and created data; and the collection of new data when the AI is put into the field. The many types of data collected increase the potential for more personal inferences to be drawn on top of the more personal data collected. This data can threaten the privacy of both the data subjects and others within the spheres of the AI’s use. Data must be carefully accumulated, strenuously protected, and, where possible, avoided – the less data that is necessary, the less data is at risk.
This naturally leads to the question of balancing challenges with use, including how to assess trade-offs within the realm of responsible innovation. This depends, as with so many cases, on the context.
4.3.1. Appropriateness
The first and most crucial question is whether the AI application in question is suitably human-centered. While arguments as to what constitutes a morally acceptable or ethical purpose will be waged elsewhere, it is appropriate to acknowledge that the purpose itself must be acceptable before moving to secondary, though not unimportant, questions. The second question is what types and level of data are needed to accomplish the stated purpose. What precisely does the AI need to accomplish its purpose? How can it best be designed to accomplish this purpose with minimal privacy impact? This includes discussions of considerations such as privacy by design, which consists of both privacy as the default and preventative rather than remedial protection. The reasons and reasonability for data may vary: a verbal translation AI would absolutely need access to natural language processing; a credit scoring model likely would not. The second aspect to fitness for purpose is the level of protection required, which depends both on the nature of the AI and the nature of the data. Level and type of protection depends on both what the data consists of, and how privacy-infringing it may be, as well as who may need access to the data. A radiological diagnostic AI is likely designed to be accessed by the patient’s healthcare system, whereas a child’s toy robot is not. Questions of proportionate protection further extend to context-appropriate PETs: what measures are suitable for the protection of the necessary data.
4.3.2. Control
However, privacy is not solely about the collection of data by another, but about control over one’s own data – secrecy or concealment, choice of dissemination or access, what aspects of life are proffered for consumption. There is no easy answer to resolving the privacy paradox – the relative value placed on privacy by individuals, but corresponding indifference to it in action. The human centricity of AI is limited by both levels of understanding and control – the level to which one is aware how one’s data is collected and used, and ability to affect that collection and use. Understanding of data collection and use is growing broadly within the public, as seen with the pushbacks on collection, but can and should be improved, particularly on a case-by-case basis. Elements of transparency and explainability go towards an understanding of data collection and use, but must be appropriately targeted to the data subject in question, and must not be obscured by data quantity in the information provided with a product. Even more complex, however, is the address of control over data. While legislation such as the General Data Protection Regulation (GDPR) purport to provide individuals with the ability to revoke consent over data use, there is a considerable ground still to cover as to what this looks like: how does an individual’s data affect a model? Can it be effectively revoked? This challenge exists even on a localized level – can an AI user or stakeholder effectively manage what data about them is used, how it is used, and how to block the AI from making forward connections with it? These questions too are context dependent – perhaps a user should not be able to change or block the results from a court-ordered blood draw – but are nonetheless incredibly important for the future of HCAI.
These, then, are the challenges of privacy and HCAI: the innovative potential of AI to be balanced against the humanistic desire and need for privacy in its many definitions. Privacy is a core part of responsible innovation; design; governance; interaction; and, perhaps above all, well-being. Certain steps can and should be taken: the minimization of collection, the protection of data, the consideration of context. Appropriateness must be weighed on an informed, human-centric, case-by-case basis: what is necessary, sufficient, and well protected. But there is not yet a balance between privacy, collection, and control – a novel and necessary avenue for ongoing research.
5. Design and evaluation framework (challenge 4)
5.1. Definitions and rationale
The HCI community has acknowledged the need to adapt the design practice to the specific challenges posed by AI (Yang et al., Citation2020). In this section, the challenges involved in the design of HCAI systems are reviewed and framework that can inform practitioners is introduced. The framework starts by drawing a distinction between two types of AI systems: those that have extremely low or high risks associated with them, and those with medium-high risks. It has been argued that AI systems with extremely low risks do not need special measures (e.g., those discussed in Section 6. Governance), whereas those with very high risks should not be allowed (Floridi et al., Citation2022). The systems that are most interesting to HCAI are, therefore, those involving medium to high risks. These systems have a great potential to improve human well-being, but at the same time pose serious risks, as documented by an increasing number of incidents (McGregor, Citation2022). The growing awareness of the risks associated with our emerging data-centered society has led to the rise of a number of movements with similar goals (see Section 3.1.2. Summary of initiatives and standards), which agree on the need to apply a more humane approach to the design of AI systems but differ in vocabulary and scope. Unlike the other movements that emphasize the human-in-the-loop concept, yet generally maintain a rationalistic perspective to the design, most HCAI definitions see the design of AI systems from a humanistic perspective where “enlightened trial and error” (Winograd, Citation2006) is the preferred way to address the complexities of real-world problems.
Drawing from these, a design approach is proposed for HCAI that considers both perspectives. In such a vision, human and societal well-being are at the center of the design process. Contrary to the standard AI paradigm which focuses on data and the maximization of an objective function, the HCAI vision relegates the AI objective function to an engineering requirement and promotes human objectives to the center of a design process with multiple stakeholders seeking ways to design socio-technical systems that account for the needs of various users while preserving human dignity, safety, and agency.
There are four main aspects that characterize such an approach (Olsson & Väänänen, Citation2021):
People: The HCAI design approach considers the needs, values, and desires of different user groups, cultures, and stakeholders. The AI product or service is designed for people and with people.
Process: The HCAI design process brings human-centered design phases to the traditional AI product development lifecycle. It includes observation of users, engagement with stakeholders, usability testing, iterative refinement, and continual monitoring of the performance of humans using AI algorithms.
Product: HCAI systems are designed to empower, augment, and amplify human performance. They emphasize human control while employing a high level of automation and AI/ML algorithms. Digital cameras and navigation systems, for instance, are designed to give humans control but have features supported by AI/ML.
Principles: The foundations of the HCAI design approach are the principles of ethical AI. These principles are followed at each step of the design process and allow designers to identify and leverage new opportunities that are socially acceptable or preferable while reducing the chances that the developed technology will be socially unacceptable and hence rejected (Floridi et al., Citation2018).
5.2. Main research issues and state of the art
5.2.1. Evaluating with human participants
A key challenge to consider when approaching the design and evaluation of HCAI systems is that human-centered systems reflect design objectives and evaluation criteria that cannot generally be met without the active involvement of human participants. Consider, for example, the difference between two AI challenges related to self-driving vehicles: (a) evaluating an algorithm that detects humans or animals in a video stream, and (b) evaluating an algorithm that computes comfortable acceleration bounds for different contexts in a vehicle with human passengers. In the first case, the vision algorithm can generally be evaluated against ground truths in an offline manner, allowing many different algorithms to be explored to optimize performance rapidly. In the second case, there is no “ground truth” established. The acceptable levels of acceleration may depend not only on the individual riders and their positions in the vehicles, but on other properties of the platform and algorithm, including speed, bumpiness, and the proximity of nearby traffic.
HCI research has a rich history of human evaluation and human research in the early stages of exploration and design. The techniques identified in classic HCI references (Shneiderman, Citation2016) and (Nielsen, Citation1994) depend on the active engagement of users in the evaluation of an interface. Even as the field has evolved to incorporate greater use of principle-driven and theory-based design (Steffen, Citation2021), new technologies and new interactions continue to need users in evaluation, whether to validate, re-calibrate, or differentiate from existing models and theories of user interaction.
5.2.2. Augmenting the traditional AI lifecycle with human-centered activities
From a methodological perspective, existing HCI design and evaluation methods are critical to enabling HCAI. Evaluating with human participants is of great importance as discussed above, but practitioners should see this only as a minimum requirement to consider when designing HCAI interventions. In fact, it may be difficult to recover from issues and missed opportunities discovered during the evaluation phase because a substantial amount of time has already been spent on building a complete prototype. Therefore, it is beneficial to incorporate additional human-centered design activities into the traditional AI lifecycle, especially during the earlier stages of the design.
illustrates how AI and HCI processes are typically integrated for addressing HCAI challenges. Here, a traditional AI system design process (Wirth & Hipp, Citation2000) is interconnected with a double diamond HCD process. With its distinction between concept design and realization, the double diamond representation is well suited for illustrating several HCD processes, such as interaction design, participatory design, or inclusive design, that are all considered to be helpful in designing HCAI interventions (Auernhammer, Citation2020). In both concept design and realization, the diamonds represent the need to alternate divergent phases of exploration with convergent phases of synthesis.
Figure 3. Integration of AI and HCI design processes. AI process steps are shown at the top and the HCI Double Diamond process is shown at the bottom. The middle layer connects AI and HCI design processes ensuring that the design and evaluation of AI systems are centered around humans through established principles and governance.
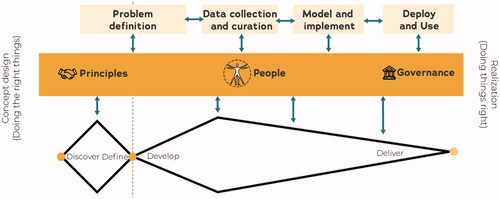
AI and HCI processes are interconnected by a middle layer, which serves as the framework’s communication layer. People, principles, and governance form the core of the layer. As we imagine this layer as a dense network of “highways” or interconnections, we can see how each stage of the design process is inextricably linked with the elements in the middle as well as with the other stages. The success of HCAI interventions will then depend on the quality of the communication layer. According to a recent study, it is typical for industry projects that pursue HCAI systems to divide the responsibilities between AI and HCI teams (Subramonyam et al., Citation2022). A common characteristic of such cases is the presence of an inefficient communication layer that prevents cross-disciplinary collaboration. Meanwhile, successful teams utilize more effective channels of communication that work beyond the boundaries of expertise, for example, via special abstractions that reveal low-level details of design and implementation (Subramonyam et al., Citation2022).
5.2.3. Ensuring universal accessibility
Forthcoming intelligent environments will be the smart home, workplace, hospitals, schools, and entire smart cities. In these emerging intelligent environments, AI technology will be a vital component of daily activities catering for human needs, well-being and prosperity. At the same time, despite its potential contributions, AI is being criticized regarding ethics, privacy, transparency and fairness. A major concern pertains to bias and exclusion that may be introduced by AI algorithms against individuals or entire social groups, including persons with disabilities, older adults, as well as vulnerable individuals.
Therefore, universal accessibility does not involve only individuals with impairments who are at risk of exclusion. Factors that have an impact on digital inequality, which are expected to be amplified in an AI context, include race and ethnicity, gender, socioeconomic status, age, education, occupational status, health, social connectedness, and availability of infrastructure. Under this perspective, universal accessibility is a fundamental pre-requisite as well as a main building block for the design and development of AI technologies which truly cater for human well-being both at the individual and the societal levels (see Section 2. Human Well-Being).
In this respect, universal accessibility of AI-enabled systems is expected to constitute a pillar of HCAI efforts, pursuing to alleviate, in a systematic manner, such risks of exclusion. Exclusion does not relate only to persons with disabilities and older persons, but refers equally to race, class, gender, and other dimensions of inequality, such as expertise with technology (Robinson et al., Citation2015). Exclusion may also refer to bias induced due to the data used for training an AI algorithm (Hoffmann, Citation2021), algorithmic bias and bias due to context of use (Ferrer et al., Citation2021), as well as due to the lack of following genuinely human-centered approaches when designing AI enabled systems (Margetis et al., Citation2021). In this respect, the concept of universal accessibility becomes both timely and critical, since access to AI-enabled technology will not only mean access to information, but pertains to fundamental human rights, such as education, health, well-being, security, and privacy (Stephanidis et al., Citation2019).
Design for universal accessibility requires design for diversity, whereby diversity refers to the broad range of user characteristics, the variety of contexts of use and human activities supported through a variety of technological platforms. The critical factor for achieving universal accessibility is to consciously and systematically apply principles and methods, and use appropriate tools, proactively, in order to develop systems, applications and services that are accessible and usable by potentially all citizens (Stephanidis, Citation2021).
An analysis of the suitability of interaction modalities encountered in intelligent environments highlights that different combinations of modalities may serve the needs of each user, and provides insights into the interaction challenges faced by each user category (Ntoa et al., Citation2021a). Besides the challenges faced with interaction techniques, individuals with physical disabilities may also encounter challenges with sensing systems, such as motion and biometric sensors, including premature timeouts, difficulty to set up systems, biometric failures, security vulnerabilities, or incorrect inferences (Kane et al., Citation2020).
In the context of adaptation-based accessibility, AI offers a great potential for fine-grained adaptation and personalization of user interfaces and interaction through ML-based user modelling and run-time interaction monitoring (Zouhaier et al., Citation2021). AI-based approaches to adaptation and personalization, by overcoming the bottlenecks of previous rule-based approaches, such as adaptation rules design and user proofing difficulties, bring significant potential to contribute to the wider practice and adoption of adaptation-based accessibility.
Fundamental principles regarding the role of AI to enhance the UX of people with disabilities already constitute the core of current research on the topic of e-Accessibility. Some groups have argued for the potential of AI in web content accessibility, highlighting the main role that AI can have in fostering assistive technologies and providing suitable content to people with disabilities including AI-based image and voice recognition, AI text processing, and affective computing (Abou-Zahra et al., Citation2018). However, potential caveats that may emerge by employing AI in web content accessibility approaches are also indicated, raising caution regarding the limitations of AI, such as accuracy, accountability, and sensitivity.
Overall, AI can offer ample novel opportunities to assist the everyday life of individuals with disabilities, with a considerable number of solutions being reported in the literature. For instance, a computer vision-based AI system provides acoustic information to individuals with visual impairments regarding the location, identity, and gaze-direction of nearby people (Grayson et al., Citation2020). Similarly, an automatic captioning smartphone-based platform employing deep learning (Makav & Kılıç, Citation2019) uses an image from the smartphone camera and generates a sentence to describe the visual content in natural language. Targeting people with severe upper limb impairments, a wheelchair that can be driven using facial expressions (Rabhi et al., Citation2018) employs neural networks and image processing, eliminating the need for a conventional joystick to specify the navigation path or move to a desired point.
Beyond the accessibility issues concerning users with disabilities, indicative of the importance of developing inclusive AI are the efforts reported in the literature across different contexts. The perspectives, challenges, and opportunities for inclusive education employing AI including the need for addressing ethical issues and accounting for cultural differences have been described (Mohammed & Nell’ Watson, Citation2019),. A strengths, weaknesses, opportunities, and threats (SWOT) analysis in the field of AI in education concluded that there are both promises and threats, and that the field seems to be at the moment in a state of hype, calling for action to open an informative discussion on this topic (Humble & Mozelius, Citation2019). In the field of inclusive healthcare, a considerable risk is that technologically advanced healthcare solutions are being developed mostly in high-income countries, which can be mitigated if a responsible and sustainable approach is followed for advancing AI-enabled healthcare systems also in middle- and low-income countries as well (Alami et al., Citation2020). Other scientific articles report on inclusive growth (Dubé et al., Citation2018; Fleissner, Citation2018), inclusive innovation and sustainability (Visvizi et al., Citation2018), and inclusive organizational environment (Miller et al., Citation2018), as these are shaped by the use of AI technology.
It is evident that developing inclusive AI-enabled environments is becoming a popular topic, but also that it is critical to promote coordinated and systematic efforts in this direction by building upon existing knowledge and practices for achieving universal access, and by actively involving end-users in the development lifecycle of AI-enabled systems, as mandated by the human-centered design process. At the same time, caution must be paid to avoid promoting the hype of AI, but instead to develop concrete methods, solutions, and tools for pursuing HCAI. In this respect, several challenges need to be addressed in order to elaborate a systematic approach to universal accessibility in AI-enabled environments (Margetis et al., Citation2012). Such challenges pertain to advancing knowledge regarding end-user requirements for universal access, researching the appropriateness of different solutions for the various combinations of user characteristics and environment characteristics and creating related ontological models. In addition, inclusiveness should become a primary concern of designers and engineers from the initial stages of the design of an AI-enabled system to data selection, model training, software development, and validation and testing.
5.2.4. Ensuring universal usability and improved user experience
Universal usability is tightly coupled with universal accessibility, aiming at successful usage of technology by the majority of the population (Shneiderman, Citation2000). This addresses the needs of all potential users, independently of their variable or invariable characteristics, as well as the technological and overall context, but also brings to the forefront the issue of usability (Lazar, Citation2007). But what does usability mean and what does it encompass in AI-enabled systems and environments?
Usability has been defined as the extent to which a system can be used by users to achieve goals with effectiveness, efficiency, and satisfaction (ISO 9241-11,11, Citation2018), while additional quality components of usability have been identified, such as the learnability of the system, the memorability it provides, as well as how many errors users make, how severe those are, and how easily can users recover from them (Nielsen, Citation1994). Apparently, no matter how technologically advanced a system may be, these concepts are influential on how usable it will be for its users. However, undoubtedly, the universal usability of AI environments goes well beyond these definitions.
Firstly, one should approach the problem following a more holistic approach, expanding from usability toward the notion of UX, and considering a person’s perceptions and responses resulting from the use and/or anticipated use of an AI-enabled system or environment, considering all the users’ emotions, beliefs, preferences, perceptions, physical and psychological responses, behaviors and accomplishments that occur before, during and after use (ISO 9241-210,210, Citation2019).
UX factors in intelligent environments have been identified to include natural interaction, accessibility, cognitive demands, emotions, well-being and quality of life, end-user programmability, safety and privacy, social aspects, cultural issues, and aesthetics (Stephanidis et al., Citation2021). A few sets of design guidelines for designing AI interactions have already been proposed in the literature (Amershi et al., Citation2019). The People + AI Guidebook (Google, Citation2022) provides guidelines organized in six chapters, namely, user needs and defining success, data collection and evaluation, mental models, explainability and trust, feedback and control, errors and graceful failure. Furthermore, a comprehensive framework has been proposed for the evaluation of UX in intelligent environments, identifying the following key UX constructs: intuitiveness, unobtrusiveness, adaptability and adaptivity, usability, appeal and emotions, safety and privacy, technology acceptance and user adoption (Ntoa et al., Citation2021b).
Focusing on the algorithmic autonomy of AI systems, additional concerns that are pivotal for UX include explainability, fairness, ethics, avoidance of automation bias and visualization of AI uncertainty, detection and mitigation of algorithmic bias, as well as responsible democratization of AI (Bond et al., Citation2019). At the same time, if the aforementioned UX aspects are well applied, the perils of inappropriate trust, loss of manual skills, and insufficient situation awareness, which constitute fundamental automation problems (Wallach et al., Citation2020) can be avoided.
AI explainability is a well-established topic in literature, mandating that users can understand machine’s decisions and actions (Gunning & Aha, Citation2019), which – from a UX perspective – calls for action to adopt effective visualization models, adaptive UIs, gesture recognition (Liu & Pan, Citation2022), and natural dialogue technologies that will contribute to comprehensible AI (Xu, Citation2019). When it comes to fairness and ethics, it is helpful that there are already several relevant principles and checklists available, however, it is very difficult for practitioners to successfully apply them in the numerous decisions they have to make on a daily basis (Madaio et al., Citation2020). As a result, additional research is required in order to make ethics and fairness easy to use in the daily routines of AI designers and engineers.
AI-induced bias is also a topic much discussed in research, but also in public discourse regarding fairness and ethics, but also regarding universal accessibility of AI. Automation bias occurs when users “over-trust” or inappropriately trust the automated decision making, but also expands beyond explainability to plausibility, reliability, predictability, and intervention possibility of the automated system (Strauß, Citation2021). Algorithmic bias, on the other hand, refers to systematic deviation in the algorithm output, performance, or impact, relative to some norm or standard, and can result in moral, statistical, social bias, or other, depending on the normative standard used as a reference point (Fazelpour & Danks, Citation2021).
Finally, besides all the aforementioned key concerns to the usability of AI-enabled systems and environments, additional issues should be addressed depending on the particular technology being developed, the target users, and application context. For instance, a particular focus on natural dialogue interaction should be placed when designing an intelligent personal assistant (Murad & Munteanu, Citation2019), whereas if the system addresses an educational context, domain-specific concerns should be catered for in order to make it usable (Langley, Citation2019; Luan et al., Citation2020).
In conclusion, as it has always been with usability, there cannot be a single recipe for the design and development of universally usable AI. Nevertheless, well-known principles for usable design will always constitute a solid and sturdy basis, which will support the entire construction. Additional constituents should also be added in order to achieve a truly universally usable AI.I It is important that traditional human-centered design practices are adhered to, putting humans-in control of the entire design and development of AI-enabled systems (Margetis et al., Citation2021), thus ensuring that the system addresses users’ needs in the best possible way. The endeavor is not trivial, requiring human-centered approaches to face new challenges, shifting in focus and methods, so that they can effectively address the critical issues that underlie a more trustful and beneficial relationship between humankind and technology (Stephanidis et al., Citation2019). It is the only viable approach that can guarantee that an AI-enabled system is usable and ensures high-quality UX, allowing researchers, designers, and engineers to safely navigate through the challenges entailed in the process and address them in the best possible way for the benefit of end-users.
5.2.5. Generative design
Generative design involves an algorithm that iteratively generate outputs that meet certain constrains, the design candidates, and a designer that select and fine tune these outputs (Kallioras & Lagaros, Citation2020). In fact, the designer itself can be an AI, resulting in a fully automated design process. For example, in generative adversarial networks, two different artificial neural networks take the roles of generator and designer (Goodfellow et al., Citation2020). Another approach uses Darwinian evolution algorithms (AI) to create neural networks algorithms (AI) that become progressively good at solving problems (Lipson & Pollack, Citation2000). In this context, on which an AI is designing another AI, the situation is more complex, but the human meta-designer should ascribe to the same human-centered design and evaluation principles as any other AI designer.
5.3. Summary of emerging requirements
The goal of establishing a human-centered AI design and evaluation framework is to ensure that the ethical AI principles are respected and human control and agency are maintained throughout the AI system life cycle: from data collection, and curation, to design, implementation, evaluation, deployment, use, and retirement. This framework is a collection of guidelines and as such, it cannot guarantee that the guidelines will be followed. As a result, there is a need for a governance structure to establish and enforce the human-centered AI design framework and assess the associated risks and societal impact of the designs. There are various ongoing efforts by governments, private institutions, and international organizations to establish guidelines and governance structures for continuous assessment and monitoring of AI system design, implementation, and deployment (see Section 6. Governance), but no universal adoption yet.
5.3.1. User communities: A new type of research infrastructure
Part of what is needed to advance research in HCAI is a set of research infrastructure that comprises stable user communities willing to use experimental systems. This “infrastructure” exists today primarily in the for-profit world. Companies like Google, Amazon, and others regularly carry out AI experiments on their users. Google evaluates new search engine algorithms, new recommendation systems in YouTube, new voice interaction in Android, and much more. Similarly, Amazon regularly experiments using its billions of customers to evaluate everything from product recommenders to interfaces to home interaction systems such as the Echo and Ring systems.
Academic researchers generally have no access to such infrastructures. And neither do entrepreneurs with early-stage start-ups. There have been a few examples of long-lived infrastructure including: the MovieLens research recommender system which has operated since 1997, though access has only been through co-operation with the University of Minnesota (Harper & Konstan, Citation2016); various universities operated Smart Homes, though only the recent HomeShare project attempted to provide a common infrastructure for operating and conducting experiments on a set of homes with willing residents aging in place. Faced with the lack of infrastructure, some researchers abandon human participant studies entirely. Others use one-time studies of students or crowd workers. But few are able to replicate the conditions of meaningful sustained use and carry out the controlled online field experiments that are needed to validate AI systems in context.
Thus, it is critical for the advancement of HCAI research that researchers come together to design, and that funders support, shared research infrastructure that includes intelligent systems, communities of users, and the mechanisms to permit effective experimentation, including: consent mechanisms and ethics review, subject management, detailed logging and reporting of results, and a flexible architecture to permit intelligent systems to co-exist and be tested across the user base.
5.3.2. The importance of community-derived metrics
Metrics drive research, and ML system metrics drive algorithms. As a result, a key challenge for advancing HCAI is development of sets of meaningful metrics that reflect the goals for such systems. In the absence of such community metrics development, too often researchers adopt metrics that are easy to compute, easy to optimize for, and not necessarily close enough to the goals of users or system developers.
An example of this challenge existed in the mid-1990s and early 2000s of the recommender systems field. Early researchers in this field were largely from the HCI community or the HCI-meets-AI community. These early works used a broad suite of metrics that included user satisfaction, impact on user behavior, predictive error, decision support metrics, and others. Fairly quickly, though, most researchers-particularly those conducting their experiments offline on datasets rather than online using human participants- converged on a single metric: predictive error. Researchers focused on minimizing the root mean squared error (RMSE) of predictions as compared with provided ratings. Such focus on a single limited metric had several problems. First, it counted errors in all predicted items the same, while most real applications had usage patterns where errors only mattered if they were in the top items that were being recommended and displayed. Second, it ignored the bias introduced by over-training around the items already rated by the user; accordingly, such algorithms were less likely to introduce users to new content which might be preferred over the already consumed content, and were simply overly conservative and potentially boring to users. The focus on a single algorithm did lead to tremendous advances in optimizing for accuracy, but this hyper-accurate system never proved to be practical or useful; the field moved toward broader metrics including top-n recommendation performance, diversity, serendipity, and other metrics better tied to user experience.
5.3.3. New HCAI programs in education
As the use of AI technologies grows, so does the need for a workforce with relevant skills to develop AI systems. Educating this growing workforce on human-centered and ethical AI emerges as a crucial requirement to ensure that AI systems are built that are beneficial to society while avoiding unintended harmful impacts. Stronger and coordinated efforts between academia, industry and government are needed to reorient program curriculum to align with human-centered AI design and evaluation principles, bring awareness to these topics and tools available to leverage for optimum design not only for performance but also for ethical outcomes and societal well-being.
5.3.4. Methodological requirements
Existing HCI design and evaluation methods provide a foundation for enabling HCAI from methodology perspective, but these methods were primarily defined for non-AI computing systems and may not effectively address the unique issues in AI systems. Recent research already reported that many challenges have been encountered in the process of developing HCAI systems (Yang et al., Citation2020). Research shows that there is a lack of effective methods for designing AI systems and HCI professionals have had difficulty performing the typical HCI activities of conceptualization, rapid prototyping, and testing (van Allen, Citation2018). Future HCAI design and evaluation work inevitably puts forward new requirements on these methods.
To effectively address the identified unique issues of AI systems as discussed earlier, over 20 existing methods of HCI, human factors, and other related disciplines from the HCAI perspective have been analyzed (Xu et al., Citation2022). As a result, summarizes a comparison between the existing design and evaluation methods (e.g., typical HCI methods being used in designing non-AI systems) and the selected 7 alternative methods that are presented by enhancing existing HCI methods and leveraging the methods from other disciplines (Jacko, Citation2012). As shown in , such alternative methods may be able to augment limitations of applying conventional HCI methods to the development of AI systems.
Table 4. Comparison between conventional HCI methods and the alternative methods [adapted from (Xu et al., Citation2022)].
6. Governance and independent oversight (challenge 5)
6.1. Definitions and rationale
The current moment represents a critical turning point in human history in which the governance of AI/ML needs to be more consciously shaped by ESG (environmental, social, governance) principles. Specifically, this means adopting an approach where human and environmental well-being are at the core of any AI regulatory framework. The Wellbeing Economic Alliance supported by the governments of Iceland, New Zealand, Scotland, and Wales, stands as a powerful model for how humans can build well-being economies (WEAll, Citation2021). This global movement, in tandem with the Doughnut Economics Action Lab methodology, posits that 21st century economic development depends on the recognition that the health of humans and the environment are interdependent (Raworth, Citation2017). More precisely, the 21st century’s grand challenge is “meeting the needs of all people within the means of the planet” for any economic growth targets moving forward (Raworth, Citation2018). In autonomous and intelligent systems, the mindful creation of such domains must subscribe to a higher purpose of sustainable well-being if there is to be a future for generations to come (see Section 2. Human well-being). As a consequence, greater ecological integrity and social justice, for example, must be accounted for in the governance of the entire AI lifecycle of design, development, and deployment (Falco et al., Citation2021). Integral to the success of a sustainable future with AI-enabled systems is the adoption of an HCAI approach that explicitly considers the well-being both of the human and of the environment. Central to the role of HCAI is further answering the question: how can development approaches be best integrated within a governance framework to enable and facilitate a maximum outcome of well-being? This section addresses: critical HCAI-informed guiding principles; forms of governance and the embedding of HCAI; examples of global AI frameworks being developed; and a proposal for a novel governance framework that integrates elements from current global frameworks as well as HCAI priorities.
6.1.1. Introduction
Expansion of AI-enabled use cases across a broad spectrum of domains has underscored the benefits and potential risks of AI (Brynjolfsson & McAfee, Citation2014; PricewaterhouseCoopers, Citation2017; West, Citation2018). Moreover, as climate change disruptions proliferate and demands for a sustainable future rise, corporations, non-profits, and countries are being forced to refocus their priorities (Business Roundtable, Citation2019, Aug 19; Cadbury, Citation2000). One such priority is to ensure the well-being of individuals, communities, and the environment as a result of acknowledging the interdependency of ecological balance and economic growth (Uddin et al., Citation2019). As a result, the three phases of the AI lifecycle – design, development, and deployment (Leslie et al., Citation2021) – call for a new outlook. The Silicon Valley model of “move fast and break things” (Taplin, Citation2017) is no longer tenable or even ethical; instead, technology needs to be responsibly and intentionally built with humanity and its role within and for the environment front and center (IEEE, Citation2019a).
6.1.2. Governance, trust, and guiding principles
For AI-enabled systems, this presents significant challenges given the self-regulatory mode of operation that has dominated the AI governance landscape until now. This has been seen in the asymmetrical standards utilized to introduce new technologies to market –specifically, a mindset change has been seen in the development of proposed guidelines for the responsible design, development, deployment, and regulation of AI/ML systems (Jillson, Citation2021; B. Mittelstadt, Citation2019; Smith & Director, Citation2020). Notwithstanding, the success of a possible future with an economic system that is accessible to and respectful of all depends on AI ethics that places trust at its core and AI governance that achieves user and environmental protection against specific AI-incurred risks. Trust is vital to underscore as an underlying quality of AI ethics and HCAI because it supports, in part, the very reason why we should engage with a technology in the first place (López-González, Citation2021). Regarding the role of trust in developing a governance framework, fundamental questions that need addressing include (see Section 3). Responsible Design of AI): Which guiding principles should inform regulation such that well-being is unquestionably ensured? What criteria need to be identified and standardized to establish confidence in what is being built? Who decides which principles are acceptable and which are not? What does consensus-based governance of AI look like? and Who carries out oversight and checks for accountability?
summarizes four identified guiding principles as a starting point, along with their unique role in ensuring well-being. These guiding principles (Fairness [see Section 3.2.3. Fairness], Integrity, Resilience, Explainability [see Section 3.2.1. Explainable AI], FIRE), inspired by the Federal Trade Commission’s guidelines on the use of AI tools (Smith & Director, Citation2020), are fundamental to building responsible and intentional technology, and adherence to them can elicit trust because of their due diligence to well-being standards, and their incorporation as a systemic factor. Emphasizing the value of trust becomes paramount to establishing a workable governance framework genuinely constructed to promote not just short-term but long-term human and ecological well-being. In fact, given the already rapid environmental changes occurring and the impacts on well-being as a result, FIRE becomes vital to supplying the flexibility required for continuous measurement and reevaluation of outcomes that an AI governance framework needs to succeed over time.
Table 5. Guiding principles of governance systems for the use of AI.
6.2. Research issues and state of the art
Governance can take many forms, can be highly granular and piecemeal, and can take place in many different locales, with the most familiar form being legislation. However, legislation is generally being proposed retrospectively, and technology is notoriously difficult to regulate. The example of the UK’s Online Harms White Paper, which was proposed in 2017 but which may not take effect until 2024 (Secretary of State for Digital C Media & Sport & Secretary of State for the Home Department by Command of Her Majesty, Citation2020), demonstrates that regulation – in addition to waiting for harm to occur – is difficult to frame and may well be equally difficult to enforce (Aynsley, Citation2020). As the Paper has progressed towards becoming a Bill (currently referred to as the Online Safety Bill), significant alterations have been made to reflect the changes that have occurred both in technology and society since it was framed. At the time of writing, discussions and amendments are still ongoing, and changes will certainly be required. Not only this, but governance that waits until harm has been caused runs the risk of society losing trust in a technology.
There are many ways to analyze different methods and layers of governance, but one possible framing is that different types of governance exist along a continuum. This continuum may also double as a continuum of consequences for non-compliance. For example, at one end of the continuum are legislation and regulation (sometimes characterized as “hard” governance). Here, a breach of governance is taken extremely seriously (e.g., breaking the law can have numerous effects, which are designed in most cases to be a deterrent). At the other end of the continuum are personal moral decisions; these may be entirely private and potentially consequence-free. In between are numerous varieties and nuances of governance including industrial standards, professional oversight, and codes of practice, among others; all these come from different sources and create a landscape of governance that operates at different levels of granularity. This granularity is also an indicator of the proximity of the governance type to the object of governance. For example, individual decisions happen at a “micro” level of granularity while codes of practice have a much broader “macro” remit and are more distant from the behavior being governed.
Following on from this characterization of granularity, and the “nearness” of some governance types to their object, it is possible to suggest that the more granular the type of governance, the greater the possibility that anticipatory, forward-looking governance, can be utilized. This has important implications because if anticipatory governance succeeds, then there may be less requirement for other types of governance at a greater distance.
This approach therefore seeks to try and prevent harms before they occur using forward-looking forms of governance to try and improve processes and thereby outcomes. These forms have been referred to as “tentative” governance because they are agile, non-prescriptive, and generally flexible (Kuhlmann et al., Citation2019). The aim is to use governance that is “dynamic … prudent and preliminary,” while also noting the “balancing act” with more rigid forms of governance such as legislation (Kuhlmann et al., Citation2019).
6.2.1. Prospective governance
There are several candidates for these prospective, non-regulatory types of governance, including standards and codes of conduct. Standards such as these, however, are often framed at a strategic level with particular purposes in mind. To date the authors have not found any codes of conduct that are shaped to address the societal issues that may arise, being largely framed around individual and sometimes organizational behavior, nor do the available codes of conduct usually provide any framework or encouragement to engage with society on a wider basis.
Some forms of prospective governance do address these issues of societal concerns and seek to reinforce the trust-relationship between science and society. One of these is Responsible Innovation (RI), a methodology that focuses on the processes shaping the innovation pathway: in addition to the developmental work being carried out on innovation, it draws in anticipation, reflection, engagement and responsiveness to try and ensure that innovations are aligned with societal needs (UK Research & Innovation, Citation2021, Oct 15). Concerns about prospective governance, societal acceptance and community engagement led to Responsible Innovation being incorporated into the most recent round of funding for the UK’s Doctoral Training Centers. In the US there has also been significant work on embedding responsible innovation approaches that include, for example: establishing agreed-upon frameworks to manage risks to individuals, organizations and society; creating education programs to amplify the workforce’s understanding of the potential role of AI within their jobs and across their organizations; identifying barriers to adoption (Kuzma, Citation2022); and calling for the advocacy and adoption of Responsible Innovation principles across businesses’ internal practices and governance (Business Roundtable, Citation2022, Jan 26; Microsoft News Center, Citation2022, Jan 13; NIST, Citation2021).
6.2.2. The global regulatory landscape
AI regulation as it stands today has been built via a piecemeal approach that is ultimately unsustainable as perspectives and priorities clash, and competition grows. Actions include shaping policies and establishing legislation vs. identifying frameworks of best practices. The nature of this patchwork is demonstrated by a snapshot of some global approaches, each with associated benefits and drawbacks, along with potential alternatives that leverage guiding principles (FIRE) critical to sustaining a well-being framework that can close resulting gaps ().
6.2.3. Research and development
Regulation is shaped around numerous drivers, which may include R&D priorities, national security and profit-seeking consumer demands. The American AI Initiative signed in 2019 proposes to develop AI capabilities within the U.S. through public and private partnerships and drive AI inventions in the country’s interests (Office N. A. I. I., Citation2022). The expectation of this approach rests on the assumption that prioritizing AI investment catalyzes technological breakthroughs, and thus spurs competitive leadership of the AI ecosystem both regionally and globally. Obvious impacts are the creation and attraction of talent, the building of productivity, the growth of jobs, and the cultural inspiration of an infinite number of experiential possibilities. By focusing on R&D, governments can reap the long-term benefits of strong public and private sector partnerships, including the development of an AI-trained workforce and the creation of intellectual property with the consequent economic rewards derived from countries needing to obtain copyrights and trademarks to use the technology. On the other hand, given the complexity and time-consuming requirements of R&D, efforts can be laborious and expensive, running the risk of innovating for months on end only to be upended by a cheaper alternative supported by volatile consumer demand and/or ending up with a host of innovative AI capabilities non-compliant with current regulatory requirements. Guided by such possible outcomes of innovation like economic growth and political leadership at large, this approach does not substantively address the well-being issue. In fact, because the impact on human and environmental well-being is left unmeasured and unaddressed, this approach demands a restatement of the necessary balance between innovation for economic prosperity and human empowerment beyond the ownership of tangible goods, and innovation for ecological longevity. Moreover, it indicates a critical opportunity for the business community and government to forge an adaptable and robust collaboration to ensure the positive impact of technology in as holistic a way as possible.
6.2.4. Steering groups and committees
Regulation may also be shaped around ethical guidelines and governing principles dictated by human rights requirements. The European Commission created the High-Level Expert Group on AI to generate the EU’s Ethics Guidelines for Trustworthy AI, released in 2019 (European Commission, Citation2019, April 8). Seven key requirements were identified: human agency and oversight; technical robustness and safety; privacy and data governance; transparency; diversity, non-discrimination and fairness; societal and environmental well-being; and accountability. The Organization for Economic Co-operation and Development (OECD) then launched its Principles on AI to promote trustworthiness and the respect of human rights and democratic values with specific recommendations for public policy and strategy, including the implementation of safeguards, responsible and transparent disclosures, and accountability across the development, deployment, and/or operation of AI-enabled systems (Organisation for Economic Co-operation & Development, Citation2021); the accompanying AI Policy Observatory is an online platform for the continuous sharing of new information and cross-country dialogue on AI. By focusing on the establishment of ethical guidelines and consequent governing principles, governments, organizations, companies, and AI subject matter experts can come together to discuss their unique perspectives and priorities and identify interdisciplinary solutions; in essence, it allows for the setup of a framework from which to establish a shared language for global AI norms and governance. However, who becomes part of these steering groups and committees invites the obvious questions of the tactics utilized to appoint group members, as well as who is accountable to whom and why; these are questions of power, and are therefore political (van Oudheusden, Citation2014). Moreover, without an overseeing body of the committee itself determining with transparency whether the group sufficiently represents the public’s good and not personal interests in the name of consumer welfare becomes a problematic situation where disclosing, for example, ties to corporate interests is insufficient. This approach is in theory built on the very concept of well-being, but without proper oversight its practice cannot yet be fully determined.
To further highlight the unknowns of this approach and the competitive atmosphere the field of AI has introduced at the geopolitical level (see Section 6.2.2), it is useful to mention the Artificial Intelligence Commission on Competition, Inclusion, and Innovation as introduced by the U.S. Chamber of Commerce in early 2022 (US Chamber of Commerce, Citation2022, Jan 18). Similar to the EU’s High-Level Expert Group on AI, this commission is tasked with shaping U.S. AI policy and specifically requests commentary from the public on the EU’s AI Act, Canada’s definition of AI, and the definition of AI as proposed by the OECD. The explicit reference to addressing already-proposed legal definitions of AI and respective regulation, and the fact that AI regulation is still in its early stages of development, suggests that both the setting of a global standard for AI regulation and the claim to leadership of such is still very much a realizable aim for the representative group that can best balance the short and long-term needs and goals of humanity.
6.2.5. Forums and discussion groups
Much like the creation of steering groups and committees, global thought leadership on the various societal implications of AI is shaped by such groups alongside key stakeholders from a variety of sectors, all seeking expert leaders in AI to collaborate (e.g., Canada-U.S.). The world’s first national AI strategy, the Pan-Canadian Artificial Intelligence Strategy, launched in 2017 with CIFAR (Canadian Institute for Advanced Research) leadership was funded by the Canadian Government, Facebook, and the RBC (Royal Bank of Canada) Foundation and immediately emphasized the imperative for interdisciplinary, international work around critical theme areas (i.e., Life & Health, Earth & Space, Individuals & Society, and Information & Matter) (CIFAR, Citation2022). This strategy stands as a laudable example of what global collaboration can look like across its AI & Society program that includes workshops with the public and policy conversations with the public policy community. While this effort opens the door to a wide variety of academic research perspectives, an even larger network of policymakers, and the potential for public input when meetings are made public, the curation of topic and project priorities remains unclear. Like the steering groups and committees’ approach, this approach is also built on the concept of well-being and depends on proper oversight to ensure efforts are indeed ethically aligned to the interests of all.
6.2.6. Hegemonic dominance
Regulation is also significantly shaped by the contexts and requirements of world power politics. The Next Generation Artificial Intelligence Development Plan published by China in 2017 is a roadmap of China’s stated intent to become the world’s leader in AI by 2030 (Chinese State Council, Citation2017). Motivated to lead the AI development trend of the world, multiple initiatives are proposed to establish an AI ecosystem for the entire economy and society by strengthening R&D; developing talent and skills; and establishing regulations, ethical norms, and national security practices. While such an ambitious national framework is already spurring aggressive competition in the current changing multipolar geopolitical landscape, such ambition underscores the massively coordinated and expensive nature of the endeavour, leaving unknown the covert intent behind the expansiveness and the potential for growth to increase unchecked.
A counter initiative is the Global Partnership on AI (GPAI) which was launched in 2020 by the G7 to provide a platform for cooperation between allied, democratic nations to better compete with China on AI (The Global Partnership on Artificial Intelligence, Citation2022). The U.S.’s launch of the National AI Initiative and National AI Initiative Act of 2020 is a sign of support for cooperation with strategic allies on R&D, assessment, and resources for trustworthy AI systems (Office N. A. I. I., Citation2022), with “International Cooperation” as one of six strategic pillars of the U.S.’s AI priorities. At the same time, the U.S. Department of Defense’s inauguration in early 2022 of the Chief Digital and Artificial Intelligence Office “to strengthen its technological superiority amidst a global race for technological advantage” (Office of the Under Secretary of Defense for Research & Engineering, Citation2022) underscores the nation’s continuing priorities around developing AI applications for warfare and defense to challenge China, a stark contrast to the EU’s lack of prioritization of the use of AI in defense (Heikkilä, Citation2021, March 29). In fact, such active pursuit by Europe on the issue of ethics and their equivalent legal outcomes has led some to argue for Europe’s leadership status in this domain and the counterproductive effects it could have on the bloc’s rise to power and its necessary industrial dependency on the U.S. or China (Miailhe, Citation2018). Nonetheless, the European Commission proposed a New EU-US Agenda for Global Change in 2020 precisely intended to reaffirm a joint commitment to transatlantic and international security, and to “intensify their cooperation at bilateral and multilateral level to promote regulatory convergence and facilitate free data flow with trust on the basis of high standards and safeguards” (European Commission & High Representative of the Union for Foreign Affairs & Security Policy, Citation2020, Dec 2). As the call for “digital sovereignty” or significant boosting of the local digital industry in the EU – which took over in 2021 (Fleming, Citation2021, March 15) – continues in early 2022 (European Parliament, Citation2022, Oct 2), the jury is out on whether the Global Partnership on AI, the U.S.’s National AI Initiative and most recent establishment of its own AI commission and Chief Digital and Artificial Intelligence Office, and the EU’s ethical stance on AI regulation will successfully lead transatlantic cooperation for competitive advantage in the wake of China’s continued progress across various AI capabilities (European Commission, Citation2021, December 15; Top500.org, Citation2020), and its rising status as a “near peer competitor” to the U.S. (Allison, Citation2021, Dec 7). Under this approach, the question of well-being and adherence to FIRE principles takes on second-tier status, if indeed any, to the premier priority of global AI primacy. Here, winning the title of global AI leader equates to treating AI development as a race dependent on achieving power through quantity and defense capability.
6.2.7. Governance case study: finding the right balance within a governance framework
In order to pursue governance approaches at high level, it is critical to agree on a definition of AI; this is most clearly seen in the exigencies of legislation. Definitions must have not only sufficient precision to be unambiguous, but must be neither too broadly nor too narrowly drawn. Such definitions must also be future-proof, as far as possible, to ensure that the legislation continues to be relevant as new applications are developed and deployed.
One example of legislation currently in draft is the EU Artificial Intelligence Act (European Commission, Citation2021 from the European Commission. Article 3 of the AI Act defines an AI system as “software that is developed with one or more of the techniques that can, for a given set of human defined objectives, generate outputs such as content, predictions, recommendations, or decisions influencing the environments they interact with.” The techniques identified include: (a) ML approaches, including supervised, unsupervised, and reinforcement learning, using a wide variety of methods including deep learning; (b) Logic- and knowledge-based approaches, including knowledge representation, inductive (logic) programming, knowledge bases, inference, and deductive engines, (symbolic) reasoning and expert systems; and (c) Statistical approaches, Bayesian estimation, search- and optimization methods.
The AI Act seeks to strike a balance between being too specific and not specific enough by describing a risk-based, use-cases framework for regulating AI with designated “high-risk” sectors subject to rules that regulate how companies can design, develop/train, and deploy AI systems. There is also a category of “unacceptable risk” (such as facial recognition in public places) where no possible implementation could be considered valid. Although the timeline for its rollout is not yet determined, there are already concerns that its definition of AI may be too expansive. For example, it would regulate a broad array of software, could create high costs for developers, and could potentially damage the digital economy (European Digital SME Alliance, Citation2021). Moreover, defining AI too narrowly risks alienating critical allies like the U.S., which has traditionally prioritized innovation in the name of expansive growth and national security (Bush, Citation2020). Other commentators point to the balance between innovating both for economic prosperity and for ecological longevity (Gupta, Citation2021, Sept 18; Klinova & Korinek, Citation2021), although the framers of the AI Act try to address possible constraints on innovation by providing for regulatory “sandboxes” that developers can use before deploying an AI product. The draft AI Act appears to be aiming at codification of “trustworthy” AI (see Section 3. Responsible Design of AI), that can recognize democratic values and human rights.
6.3. Summary of emerging requirements
Considering the various global governance frameworks in development and given the central importance of adhering to a sustainable well-being approach, this group has argued that an ideal AI governance framework is one that equally promotes well-being for humans and the planet in an intentional and responsible way (i.e., guided by FIRE principles). As such, competitive-advantage priorities (supported by the U.S.) and human rights’ principles (guiding the EU) may both be regarded as insufficient in the face of a global climate, biodiversity, and inequality crisis. In other words, good governance of AI necessitates the unpacking of several basic principles at the various levels of granularity, and using these basic principles as a shaping influence, rather than attempting to accommodate them as an afterthought.
Specifically, this group suggests the following checklist of ground rules to ensure that a holistic well-being approach is integrated within any governance framework. summarizes the role of the following ground rules across the “governance continuum.”
Table 6. The shaping influence of ground rules for AI-enabled technology across the levels and types of governance.
6.3.1. Environmental well-being
This entails a more sustainable and thus greener AI, which of necessity requires a change in process (e.g., less data and energy use) for a change in outcome/product (e.g., a lighter social and carbon footprint) (Gupta et al., Citation2020); otherwise, humans risk grave environmental and social harm and not having genuine social wealth (Ghosh, Citation2015) and a viable future. The training of some AI models requires enormous amounts of computational power and electricity use, resulting in high carbon emissions (Gupta, Citation2021, Sept 18; Lannelongue et al., Citation2021; OpenAI, Citation2018, May 16). This is in addition to the emissions that are already projected to increase unless there is meaningful intervention from information communication technologies (Freitag et al., Citation2021) more broadly. New methods for efficient deep learning deployment (Cai, Gan, et al., Citation2020) are one way to tackle the problem, although “rebound effects” may mean that efficiencies drive greater use (Martinuzzi et al., Citation2018). Users’ awareness of their emissions footprint in real time is another path that can lead to cleaner energy choices (WattTime, Citation2022). An early step is the need for effective and transparent carbon accounting for AI systems in development across the three phases of the AI lifecycle, including Scope 3 emissions (Samuel et al., Citation2022); independent oversight of this accounting, and the uncomplicated availability of users’ individual emissions reports. This underscores a dual need for significant groundbreaking clean energy investments as well as innovative educational campaigning.
6.3.2. Certification
AI-enabled products and services need to be independently certified, and users need to know what they are purchasing and using. Nutrition-like product labels for security and privacy have been proposed (Kelley et al., Citation2009) and prototyped (Emami-Naeini et al., Citation2020), and the use of Foundation for Responsible Robotics Quality Marks of responsible practices for AI-enabled robotics is currently being piloted (Foundation for Responsible Robotics, Citation2022). We embrace this nascent trend resulting from the myriad consequences of emerging technologies, and suggest significant development and mandated adoption at scale. For certification labels to have functional value, however, we further highlight both the imperative for explainable AI in which transparency is necessitated and interpretability is standardized across stakeholders’ needs, and for oversight and enforcement.
6.3.3. Independent oversight
No company should be permitted to “mark its own homework;” any claims of trustworthiness and compliance according to ethical principles and standards must be verifiable. Algorithms, the computational foundation of AI-enabled systems, are in effect socio-technical systems embedded within and across cultures with different meanings and points of interaction (Seaver, Citation2017) and self-regulation as a way to satisfy a moral imperative over a formal legal one is insufficient (Wieringa, Citation2020). Proposals for the adoption of traditional forms of oversight utilized within industry and government, including planning oversight, continuous monitoring by knowledgeable review boards, and retrospective analysis of system failures, have been proposed (Shneiderman, Citation2016). Following this requirement is the associated need for audit trails and their standardization (ForHumanity, Citation2022), as well as audit tools, methodologies, and resources at large (Mozilla, Citation2022). Audit trails that would include detailed logs of all actions pertaining to satisfying responsible guidelines can thus act as points of reference for not only the scrutiny of system design and the enhancement of system robustness as part of an established evaluation process for system measurement and assessment, but for legal purposes of accountability where decisions made and implementations carried out across the entire AI-enabled system’s lifecycle can and should be challenged.
6.3.4. Multiple stakeholders
The urgent demand for engagement with multiple stakeholders is heard not just across sectors but from all corners of the globe. In accordance with the general principle of “not about us without us,” those who are affected by a product or a product’s deployment must be included in its development. AI-enabled technology is neither confined to a single use case nor to a single market. As a result, everyone will be affected by AI at some point, if not already. The majority of WEIRD – Western, Educated, Industrialized, Rich, and Democratic (Henrich et al., Citation2010) – designers, developers, and regulators can no longer assume authority over non-WEIRD peoples as other cultures and other communities hold distinct values (IEEE, Citation2019b; Mhlambi, Citation2020) and are equally important to building technology that is respectful of others. This group argues that for the well-being of our humanity and our planet, as many perspectives as possible must be intentionally pursued and implemented for technological interoperability.
6.3.5. Documentation
Furthermore, the process of engagement with communities and stakeholders must be documented. It is imperative, regarding the oversight requirement above, that the documentation of such cross-engagement – local and/or global – is in place to ensure that decisions about who was consulted are transparent. Moreover, it is understood that not all parties’ concerns can be incorporated into final responses. Therefore, decisions about trade-offs and failure to accommodate particular viewpoints must also be documented. All of these pursuits certainly add to the length of documentation and the number of parties involved in independent oversight. As a result, and in keeping with the requirement for transparency, we must balance the stipulation for explainability of AI algorithms with the need for comprehensibility ().
In conclusion, together with the urgent need to safeguard the planet, the challenge of governance and HCAI lies in seeking a balance between the innovative potential of AI and the pressing necessity to provide a safe and just space for all of humanity.
7. Human-AI interaction (challenge 6)
7.1. Definitions and rationale
Today, computer-based (non-biological) artificially intelligent systems are capable of accomplishing a variety of complex goals and tasks, including image recognition, classification, autonomous decision making, logical reasoning, natural language processing, and many emerging capabilities that have been traditionally attributed mainly to humans. One of the critical questions for the HCAI systems designed for the benefit of society at large is the nature of the intended present and future human-AI interactions. Such interactions include the human-AI system’s interaction at work, at home, and at leisure, as well as the implications of the potential for AI technology to redefine humanity as we know it (Frischmann & Selinger, Citation2018; Karwowski, Citation2018, Aug 26; Karwowski & Zhang, Citation2021; Lee & Shin, Citation2020; Sawyer et al., Citation2021). Following the framework proposed by (Dwivedi et al., Citation2021), one can explore the impact of human-AI interactions given critical human activity domains, including: business and management; government and public sector; science and technology; arts, humanities, and law; and society-at-large.
7.2. Main research issues and state of the art
In the above context, the nature and scope of present and future human-AI interactions can be conceptualized in terms of the above application domains and main AI challenges. Technological issues include AI explainability and information-theoretic challenges of AI systems. The business and management considerations focus on decision making, work under partial and complete automation, and applications AI for digital marketing and sales. The government and public sector considerations include AI applications for small business and public sector organizations, public policy challenges of AI, and governance of AI and connected systems. The arts, humanities and law issues encompass people-centered perspectives on AI and fear and cultural proximity in demand for AI goods and services. Finally, the science and technology arena offers perspectives on role of AI in the fundamental sciences.
The specific examples of the human-AI interaction challenges in these categories, as applied to human-AI interaction across the above domains, are as follows (Dwivedi et al., Citation2021):
Social challenges: education of customers; cultural barriers; human rights; geographic or regional differences; unrealistic expectations towards AI technology; country-specific practices; and insufficient knowledge on values and advantages of AI technologies.
Economic challenges: affordability of required computational expenses; varied costs; and impact on revenue.
Ethical challenges: lack of trust towards AI-based decision making; and unethical use of shared data.
Data challenges: lack of data to validate benefits of AI solutions; transparency and data reproducibility, dimensionality obstacles; the insufficient size of available data pool; and lack of data collection standards format and quality.
Organizational and managerial challenges: the realism of AI; better understanding of needs of the technical systems; organizational resistance to data sharing; lack of in-house AI talent; the threat of replacement of human workforce; lack of strategy for AI development; lack of interdisciplinary talent; and threat to replacement of human workforce.
Technological and technology implementation challenges: variations in decision making; adversarial attacks; lack of transparency and interpretability; AI safety; specialization and expertise; big data; architecture issues; and complexities in interpreting unstructured data.
Political, legal, and policy challenges: copyright issues; governance of autonomous intelligence systems; responsibility and accountability; privacy/safety; lack of rules of accountability in the use of AI; costly human resources still legally required to account for AI-based decisions; and lack of official industry standards of AI use and performance evaluation.
Ethical challenges: responsibility and explanation of the decision made by AI; processes relating to AI and human behavior; compatibility of machine versus human value judgment; moral dilemmas; and AI discrimination.
7.2.1. Human-AI technology interactions: economy and business
It has been postulated that the large-scale application of AI systems might lead to unprecedented economic and social implications, disrupting the job market (Vochozka et al., Citation2018) irrespective of whether these applications are substitutes, complements, or extensions of existing jobs (Ernst et al., Citation2019; Hodge et al., Citation2020). For example, according to (Muro et al., Citation2019), implementation of AI systems could affect work in virtually every occupational group, and professional and better-educated, better-paid employees (along with transportation, manufacturing and production workers) might be the most affected by the new AI technologies. Furthermore, the above study also suggested that bigger, higher-tech metro areas and communities that are heavily involved in manufacturing sector are likely to experience the most AI-related job market disruptions.
Recently, Kelley et al. (Citation2021) conducted a large survey of over 10,000 respondents regarding public sentiment about AI in eight countries across six continents (Kelley et al., Citation2021). The results revealed that AI would significantly impact society in public view, with four distinct groups of sentiment towards AI (i.e., exciting, useful, worrying, and futuristic). The exciting (18.9%) category included positive feelings about AI with general excitement or enthusiasm. The useful (12.2%) category expressed the belief that AI will be helpful and assist humans in completing many tasks. The worrying (22.7%) category contained a wide range of negative emotional responses with various forms of concern and fear. The futuristic (24.4%) category referred to the futuristic nature of AI, concerning AI in the context of robots or science-fiction concepts or referencing the future in general. Given that the general nature of these effects was quite uncertain, the referenced study pointed out the pressing need for interventions and communications regarding the responsible design, development, and use of AI technologies.
One of the main worries of the public about AI and the future of work is increasing AI substitution for employees’ tasks, responsibilities, and decision making and the high potential for worker replacement (Ernst et al., Citation2019; Strich et al., Citation2021). For example, the McKinsey report (Manyika & Sneader, Citation2018) projected that under the fastest AI progression model scenario, as much as 30 percent of the global workforce that accounts for approximately 800 million workers could be displaced by AI applications in the period 2016–2030. However, as discussed by the report, accelerating progress in AI technologies will also create opportunities for the economy, businesses, and society at large. Indeed, it is widely predicted that using AI at a larger scale will change how companies create value and compete on the global markets and add as much as $15.7 trillion to the global economy by 2030 (De Cremer & Kasparov, Citation2021). Wired magazine also presented strong arguments that AI technology will provide new business tools for entrepreneurs and create new lines of business, as AI will empower workers, businesses, and industries rather than replace employees (Wired magazine, Citation2018). AI can also improve humans’ performance and productivity (Ikwuegbu, Citation2021). For example, the call center employees could get instant intelligence about what the caller needs and do their work faster and better. In life sciences, businesses employ deep learning and neural networks to bring medical treatments to market faster. Concerning the AI-human interactions at work, one of the promising new capabilities of AI is chatbot-based communication systems that can exhibit empathy through an understanding of human behavior and psychology, allowing the chatbot to interact with customers at an emotional level to assure their satisfaction and AI systems acceptance (Wired magazine, Citation2018). Indeed, a recent survey of human-AI collaboration in managerial professions including interviews and laboratory simulation, assessed various modes of collaboration between humans and virtual assistants, and showed increased task productivity due to enhanced human-AI interaction (Sowa et al., Citation2021). The study results, indicate that the future of AI in knowledge work should be based on collaborative approaches where humans and AI systems work closely together.
7.2.2. Human-AI system interactions at work
In general, the human–AI interactions at work can be conceptualized by using a scheme of four basic levels of collaboration between AI and humans in a working environment (Sowa et al., Citation2021). At the first level, the human–AI collaboration does not exist as employees either directly compete with or work independently from the AI systems. This is especially true with the use of substitutive decision making AI systems that offer employees no possibility of interacting with these system (Lindebaum et al., Citation2020). At the second level, humans and AI systems complement each other, with AI systems handling complex computations or processing massive amounts of data, while humans engage in complex decision making, using their social and emotional skills. At the third level of collaboration, humans and AI systems become interdependent on each other’s unique abilities in task performance. At the fourth level of collaboration, AI systems become a true extension of the human brain and the two agents engage in fully collaborative work ().
Figure 4. The levels of human-AI (H–AI) system interactions: level 1 = working separately or competing); level 2 (supplementing each other work); level 3 = interdependent on each other; level 4: full collaboration – adapted from (Sowa et al., Citation2021).
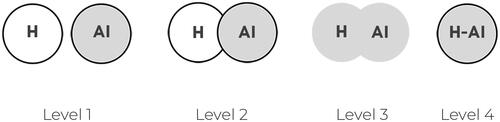
One of the critical issues in the human-AI system collaboration is the trust and responsibility shared between the human and AI system and associated with the notion of human control and AI autonomy expressed by the level of risk (Abbass, Citation2019). describes the nature of such a risk in human-AI system interactions regarding the four components of function allocation (i.e., sense-making, decision making, execution ability, and execution authority).
Table 7. The nature risk in human-AI (H-AI) system interactions; adapted from (Abbass, Citation2019).
7.2.3. The impact of AI technology on occupations
One of the critical abilities of AI systems is cognitive computing, including the ability to learn and exercise algorithmic decision making. However, there are significant unintended consequences of introducing AI systems for decision making (Hodge et al., Citation2020; Mayer et al., Citation2020). For example (Strich et al., Citation2021) investigated the impact of substitutive decision making AI systems on employees’ professional role identity in the banking system. They postulated that the implementation of substitutive decision making AI systems can significantly restrict employees’ ability to apply their skills, knowledge, and expertise to these substituted tasks. The results showed that introducing substitutive decision making AI systems can empower less qualified employees by enabling them to complete tasks they would otherwise not be able to perform, but also deskill well-qualified employees by reducing the required skills needed for their jobs.
Others have argued that while ML is poised to transform numerous occupations and industries, the successful application of ML depends on a variety of task characteristics and contextual factors of work activities (Brynjolfsson et al., Citation2018). To answer the question of which tasks will be most affected by ML, the Occupational Information Network (O*NET) content model for 964 occupations in the US economy was used (Brynjolfsson et al., Citation2018). These occupations were linked to 18,156 specific tasks at the occupation level and mapped to 2,069 direct work activities shared across the investigated occupations. Each direct work activity was scored for its suitability for ML using its modified task evaluation rubric, with higher values indicative of the greatest potential to impact jobs. The results showed that ML could transform many jobs in the economy, but it will affect very different parts of the workforce than earlier automation efforts. Also, while most occupations are suitable for some ML tasks, few occupations can be fully automated at this time using ML. It was concluded that realizing the potential of ML will require considerable reengineering of processes and the reorganization of tasks.
Recently, another group developed a comprehensive framework for assessing the impact of AI on a variety of business occupations based on the analysis of work tasks, the required human cognitive abilities, and a large set of AI capability benchmarks (Tolan et al., Citation2021). A total of 59 generic work tasks were mapped to 14 human cognitive abilities (as an intermediate layer) and linked with a list of 328 AI intensity benchmarks. The explored human cognitive abilities that are subject to AI exposure at work environments included the following categories: memory processes; sensorimotor interactions; visual processing; auditory processing; attention and search; planning, decision making, and acting; comprehension and expression; communication; emotion and self-control; navigation; learning; reasoning; social interaction; and metacognition.
Furthermore, since all human activities require the use of some cognitive ability, the high-level categorization of work tasks with respect to required cognitive abilities was also introduced by sorting each cognitive ability according to the objects that they operate on. This high-level taxonomy included the following three main categories of human-AI interactions:
Dealing with people: Emotion and self-control, mind modelling and social interaction, metacognition and confidence assessment.
Dealing with ideas or information: comprehension and expression, planning, sequential decision making and acting, memory processes, attention and search, conceptualization, learning, and abstraction, and quantitative and logical reasoning.
Dealing with (physical or virtual) objects or things: sensorimotor interaction, navigation, visual processing, and auditory processing.
The above set of cognitive abilities was mapped to a comprehensive repository of AI systems benchmarks that were identified from a large number of AI capability domains, including, but not limited to, ML, computer vision, speech recognition, machine translation, text summarization, information retrieval, robotic navigation, and interaction, automated vehicles, game playing, prediction, estimation, planning, automated deduction or music analysis. Such a mapping process enabled the assessment of which AI features would most likely affect the analyzed jobs and allowed for ranking occupations concerning AI exposure. The conducted mapping also allowed for identifying the potential AI exposure for tasks for which AI applications are yet to be developed.
The reported study results showed that most AI research activity could currently be attributed to visual processing, attention and search, comprehension and expression, learning, and reasoning. The results also indicate that with the rapid development of new AI capabilities, many traditional occupations might be subject to much higher AI exposure in the near future. Finally, it was pointed out that currently AI is used to perform tasks with comparatively limited human labor input in advanced economies, such as visual and auditory processing using deep learning and sensorimotor interaction through (deep) reinforcement learning (Tolan et al., Citation2021), while other groups have demonstrated increasing engagement with AI through the use of virtual, augmented, and mixed reality systems (Sung et al., Citation2021). Through an integration of human-AI interaction categories with cognitive abilities, occupations relying more on interpersonal skills and social decision making could soon be affected.
7.2.4. The social viability and benefits of AI systems
Based on the growing needs to explicitly assess the AI systems with respect to their social viability and expected benefits, the DEEP-MAX scorecard was developed as the transparent point-based rating system for AI systems applications using seven key parameters: diversity (D), equity (E), ethics (E), privacy and data protection (P), misuse protection (M), audit and transparency (A), and digital divide and data deficit (X) (Dwivedi et al., Citation2021). The main components of the proposed scorecard system are shown in .
Table 8. DEEP-MAX scorecard rating for AI systems; from (Dwivedi et al., Citation2021).
7.3. Summary of emerging requirements
It is widely predicted that applications of AI technology in business will fundamentally change the nature of work and the workforce as we know it today (De Cremer & Kasparov, Citation2021; Desouza et al., Citation2020; Ernst et al., Citation2019; Hodge et al., Citation2020). Recently, over 3,100 senior business leaders, managers, and IT staff working globally in financial services, healthcare, insurance, manufacturing, communications, and the public sector were surveyed on the evolving role of technology in changing nature of the modern workforce (PEGA, Citation2020). The report concluded that AI is already a major player in the workplace, as most of the surveyed organizations have been deploying deep learning (70%) and ML (68%) technologies. Sixty-seven percent of the organizations participating in the survey reported using AI to support decision making, and 64% are using AI to reach decisions without human input. Furthermore, 73% percent of respondents agreed that the contemporary definition of “workforce” includes both human employees and AI-based systems, while 84% of respondents stated that they were comfortable working alongside AI-based systems. Regarding the impact of AI systems on the current jobs, 64% of study participants also believed that most employees would need to learn how to apply AI systems within the next five years, while 56% agreed they would also need to understand how to train AI systems. Finally, the majority of the respondents also agreed that an application of AI-based intelligent automation would prove beneficial in terms of increasing customer satisfaction (74%), decreasing stress levels in the workplace (72%), and improving employee satisfaction (71%).
However, it should also be pointed out that in the opinion of over 10,000 respondents from eight countries across six continents, in the long-term AI will be “either good or bad for society, depending on what happens” (Kelley et al., Citation2021). This sentiment was shared by 43% of the respondents from Australia, 39% from Canada, 40% from the United States, 60% from S. Korea, 42% from France, 41% from Brazil, 26% from India, and 48% of participants from Nigeria. The above discussion provides another critical point as to the pressing need for addressing the human-centered design of AI systems in the near future for the benefit of the global society.
8. Discussion and conclusions
The consensus position of this group of international experts is to ensure that humans remain at the center of the AI lifecycle (Bond et al., Citation2019), and that AI is implemented to improve human performance in ways that are reliable, safe, trustworthy, compatible with human values, protect human safety, and assure human agency (Shneiderman, Citation2022). To accomplish this vision, AI should be developed that promotes human well-being by supporting human cognitive capabilities and emotion management, preventing negative side effects, being inclusive, avoiding bias, and being transparent and accountable. As AI becomes ingrained ever more deeply in society, AI should support the wide-reaching goals of improving medical outcomes, helping to end epidemics, providing more efficient commerce and safer transportation, and improving the environment (United Nations Department of Economic & Social Affairs, Citation2018, Apr 20). Supporting this vision requires AI that is fair and transparent to users, ethical, and respectful to the privacy of both individuals which contribute to the underlying data and end-users of the AI. Ensuring that AI is implemented which respects human values and promotes human well-being also requires careful algorithm and UI design, and oversight from individual developers and industries to sectors and global organizations. Ultimately the products of HCAI development should be compatible with human values.
Significant overlap and interrelations exist within the challenge areas discussed in this article. Ensuring that AI systems improve human well-being requires human-centered processes to design and test algorithms, safeguard privacy, and the implementation of independent oversight, especially in cases where AI will have broad use. Achieving the vision of responsible design of AI also requires the establishment of serious governance structures across the continuum of individual developers, communities, institutions, industries, sectors, and international organizations, and may consist of HCAI training, codes of practice, standards, regulations, and/or legislation. Since data used to train AI algorithms is obtained from humans, processes must be in place to ensure that such data is robust against bias and discrimination, and that data remains private and secure. Such processes should be directed by environmental and social governance principles with human well-being at the center and should include a focus on FIRE principles (Smith & Director, Citation2020). Finally, AI products and how humans interact with them should be promote human well-being. For these interrelated challenges to be addressed successfully, there is a need for shared concepts and terminology, and established routes of information sharing such as integrated guidelines, multidisciplinary workgroups and conferences, and promoting an HCAI focus on international efforts.
8.1. Research directions
While there are many existing research efforts in the area of human-centered AI, from individual grants to research institutions, internal R&D efforts at technology companies, and government investments such as DARPA’s Explainable AI, there is much that remains to be done. presents a summary of research directions that are essential to address the six grand challenges of HCAI. Throughout this article, identified research directions have ranged broadly from human-AI interaction to design and evaluation methodologies. They also include research on responsible AI design principles, privacy and human-centered policy guidelines for governance, independent oversight, and auditing.
Table 9. Research directions for HCAI
8.2. Recommendations
In order to address the six grand challenges put forth in this paper, outlines a list of recommendations, and delineates responsible stakeholders. Responsible stakeholders (Shneiderman, Citation2020a) are the individuals who take active part in the research, development, design, implementation, regulation of AI technologies. These recommendations set out the essential steps and measures to ensure a human-centered approach to AI. These recommendations serve as a call for action to the responsible stakeholders in order to create a future in which AI is designed, implemented and used in a human-centered way and contributes to the well-being and betterment of humankind and the environment.
Table 10. Call for action recommendations for HCAI by stakeholder.
8.3. Conclusion
The grand challenges discussed in this study, the recommendations, and research directions provided reflect the perspectives of 26 expert researchers from diverse academic disciplines and institutional backgrounds. However, the implementation of these recommendations and the exploration of the presented research directions will require sustained collaboration across all stakeholder communities, including researchers, developers, business leaders, and policy makers.
These recommendations serve as a guide to prioritize and plan coordinated efforts to integrate the knowledge and perspectives of all the stakeholders as we advance AI technologies in a more human-centered way. also It is hoped that the research directions proposed here inspire current and future scientists to continually innovate and promote AI to improve overall human well-being and support the wide-reaching goals of increasing equality, reducing poverty, improving medical outcomes, expanding and individualizing education, helping to end epidemics, providing more efficient commerce and safer transportation, promoting sustainable communities, and improving the environment (United Nations Department of Economic & Social Affairs, Citation2018, Apr 20).
The time of reckoning for Artificial Intelligence is now. The six grand challenges, research directions and recommendations presented in this work will help the scientific community develop AI that is centered in human values and well-being. Rather than leading to an uncertain and potentially unpredictable socio-technological future, the HCAI vision is that research and advances in AI will lead humanity confidently towards a future of prosperity, fairness, and well-being.
Disclosure statement
No potential conflict of interest was reported by the author(s).
Additional information
Notes on contributors
Ozlem Ozmen Garibay
Ozlem Ozmen Garibay is an assistant professor in Industrial Engineering and Management Systems, where she directs the Human-Centered Artificial Intelligence Research Lab. Prior to that, she served as the Director of Research Technology.
Brent Winslow
Brent Winslow is a Chief Scientist at Design Interactive and leads a cross-functional team of data scientists, engineers, and developers in leveraging non-invasive technology to assess human cognitive, physical, and health status. He is now with Google.
Salvatore Andolina
Salvatore Andolina is an Assistant Professor at the University of Palermo, Italy. His research focuses on human-computer interaction, information retrieval, and creativity. His current research interests include the design of Human-Centered AI systems for empowering humans in a variety of ubiquitous, social, and collaborative settings.
Margherita Antona
Margherita Antona is a Principal Researcher at the HCI Laboratory of ICS-FORTH. She has participated in more than 25 European and national R&D projects and has co-authored more than 160 scientific publications. She is Co‐Chair of the International Conference on Universal Access in Human-Computer Interaction (UAHCI).
Anja Bodenschatz
Anja Bodenschatz is a researcher associate in the field of behavioral economics. She holds an M.Sc. in Corporate Development and is a fellow at the Joachim Herz Foundation. Currently, she investigates ethical boundaries for the programming of autonomous systems.
Constantinos Coursaris
Constantinos Coursaris is Associate Professor of Information Technology and Academic Director of the User Experience program at HEC Montréal. Constantinos was elected Chair of the Association for Information Systems Special Interest Group in Human-Computer Interaction (AIS SIGHCI) in 2019 and was appointed AIS Assistant Vice-President for Research Resources in 2020.
Gregory Falco
Gregory Falco is a Professor in the Department of Civil and Systems Engineering at Johns Hopkins University, with an appointment at the Applied Physics Laboratory. He is also a member of the American Institute of Aeronautics and Astronautics (AIAA) and the Institute of Electrical and Electronics Engineers (IEEE).
Stephen M. Fiore
Stephen M. Fiore is the Director, Cognitive Sciences Laboratory, and Professor with the University of Central Florida’s Cognitive Sciences Program. He was a member of the expert panel for the Organisation for Economic Co-operation and Development’s 2015 Programme for International Student Assessment (PISA).
Ivan Garibay
Ivan Garibay is an Associate Professor in the Industrial Engineering and Management Systems at the University of Central Florida (UCF). He is the director of the Artificial Intelligence and Big Data Initiative, the Complex Adaptive Systems Laboratory (CASL), and the Master of Science in Data Analytics (MSDA) at UCF.
Keri Grieman
Keri Grieman holds a Juris Doctorate from the University of Calgary, and a Master of Laws from Queen Mary University of London (QMUL), where she received the Computer and Communications Law prize. Keri was the Google Policy Fellow at the Canadian Internet Policy and Public Interest Clinic.
John C. Havens
John C Havens is Executive Director of the Council on Extended Intelligence and The IEEE Global Initiative on Ethics of Autonomous and Intelligent Systems. He founded the IEEE 7010 Well-being Metric for Autonomous Systems, and The IEEE Global Initiative and Founding/co-chair of the Wellbeing Committee of the IEEE Global Initiative.
Marina Jirotka
Marina Jirotka is a Professor of human-centered Computing in the Department of Computer Science at the University of Oxford and Governing Body Fellow of St Cross College. She is Director of the Responsible Technology Institute at Oxford and co-director of the Observatory for Responsible Research and Innovation in ICT (ORBIT).
Hernisa Kacorri
Hernisa Kacorri is an Assistant Professor in the College of Information Studies. She holds an affiliate appointment in the Department of Computer Science and the Human-Computer Interaction Lab at the University of Maryland, College Park and serves as a core faculty at the Trace R&D Center.
Waldemar Karwowski
Waldemar Karwowski is Pegasus Professor and Chairman, Department of Industrial Engineering and Management Systems, University of Central Florida. He served on the Board on Human Systems Integration, National Research Council, USA. He currently is Co-Editor-in-Chief of Theoretical Issues in Ergonomics Science journal and Editor-in-Chief of Human-Intelligent Systems Integration journal.
Joe Kider
Joe Kider is an assistant professor at the Institute for Simulation and Training (IST) at the University of Central Florida, and co-Director of the SENSEable Design Lab at IST in the School for Modeling, Simulation, and Training.
Joseph Konstan
Joseph Konstan is Distinguished McKnight Professor of Computer Science and Engineering at the University of Minnesota where he has also served as the College of Science and Engineering’s Associate Dean for Research since 2019.
Sean Koon
Sean Koon received his MD at Linda University in 1998 and maintains board certifications in Family Medicine and Addiction Medicine. Along with providing patient care at Kaiser Permanente, he has served in various leadership and educational roles since 2003, including a current role as a Senior Innovation Advisor.
Monica Lopez-Gonzalez
Monica Lopez-Gonzalez is a business executive, cognitive scientist, entrepreneur, and public speaker. She is the Co-Founder & CEO at the Institute for Human Intelligence, and faculty at Johns Hopkins University in the Department of Cognitive Science, the Department of Neurology, and the AI Program of the Whiting School of Engineering.
Iliana Maifeld-Carucci
Illiana Maifeld-Carucci is a doctoral student in Systems Engineering at Johns Hopkins University focused on developing trust metrics for AI-enabled systems. She received her M.S. in Data Science at The George Washington University in 2019. Since that time, she has worked as a Machine Learning Engineer at Data Machines Corp.
Sean McGregor
Sean McGregor is an ML architect at Syntiant and technical lead for the IBM Watson AI XPRIZE. Sean also organizes a series of workshops at major academic AI conferences on the topic of “AI for Good” and develops the AI Incident Database with the Partnership on AI.
Gavriel Salvendy
Gavriel Salvendy is a distinguished professor at the College of Engineering and Computer Science at the University of Central Florida and the founding president of the Academy of Science, Engineering, and Medicine of Florida, and a member of the U.S. National Academy of Engineering.
Ben Shneiderman
Ben Schneiderman is a Distinguished Professor in the Department of Computer Science and Founding Director of the HCI Laboratory at the University of Maryland, College Park. He is a Fellow of the AAAS, ACM, IEEE, National Academy of Inventors, and the U.S. National Academy of Engineering.
Constantine Stephanidis
Constantine Stephanidis is a Professor at the Department of Computer Science of the University of Crete. He is Founder and Head of the HCI Laboratory, and Founder and Head of the Ambient Intelligence (AMI) Programme of the Institute of Computer Science of the Foundation for Research and Technology – Hellas (ICS-FORTH).
Christina Strobel
Christina Strobel is a Postdoctoral Researcher at the Hamburg University of Technology. She is a digital economist & behavioral scientist and has worked as a visiting researcher at Yale University and also is a Lecturer at the International School of Management (ISM).
Carolyn Ten Holter
Carolyn Ten Holter is a doctoral student and research assistant in the Responsible Technology Institute at the University of Oxford. Her doctoral research is investigating responsible research and innovation practice in emerging technologies, in particular quantum technologies.
Wei Xu
Wei Xu is a Professor of HCI/Human Factors at the Center for Psychological Sciences of Zhejiang University, China. He is a member of the Technical Advisory Group for the ISO Human-System Interaction Technical Committee and developed the ISO standards (9241-810: Ergonomics of Human-System Interaction: Robotic, Intelligent, and Autonomous Systems).
References
- Abbass, H. A. (2019). Social integration of artificial intelligence: Functions, automation allocation logic and human-autonomy trust. Cognitive Computation, 11(2), 159–171. https://doi.org/10.1007/s12559-018-9619-0
- Abou-Zahra, S., Brewer, J., & Cooper, M. (2018). Artificial intelligence (AI) for web accessibility: Is conformance evaluation a way forward? [Paper presentation]. Proceedings of the 15th International Web for All Conference, 1–4, Lyon, France. https://doi.org/10.1145/3192714.3192834
- AI for Good. (2021). https://aiforgood.itu.int/about/
- Alami, H., Rivard, L., Lehoux, P., Hoffman, S. J., Cadeddu, S. B. M., Savoldelli, M., Samri, M. A., Ag Ahmed, M. A., Fleet, R., & Fortin, J. P. (2020). Artificial intelligence in health care: Laying the foundation for responsible, sustainable, and inclusive innovation in low- and middle-income countries. Globalization and Health, 16(1), 52. https://doi.org/10.1186/s12992-020-00584-1
- Allen, C., Smit, I., & Wallach, W. (2005). Artificial morality: Top-down, bottom-up, and hybrid approaches. Ethics and Information Technology, 7(3), 149–155. https://doi.org/10.1007/s10676-006-0004-4
- Allison, G. (2021, Dec 7). The great rivalry: China vs. the U.S. in the 21st century https://www.belfercenter.org/publication/great-rivalry-china-vs-us-21st-century.
- Amershi, S., Weld, D., Vorvoreanu, M., Fourney, A., Nushi, B., Collisson, P., Suh, J., Iqbal, S., Bennett, P. N., Inkpen, K., Teevan, J., Kikin-Gil, R., & Horvitz, E. (2019). Guidelines for human-AI interaction [Paper presentation]. Proceedings of the 2019 CHI Conference on Human Factors in Computing Systems, 1–13, Glasgow Scotland UK. https://doi.org/10.1145/3290605.3300233
- Amershi, S., Cakmak, M., Knox, W. B., & Kulesza, T. (2014). Power to the people: The role of humans in interactive machine learning. AI Magazine, 35(4), 105–120. https://doi.org/10.1609/aimag.v35i4.2513
- Anderson, J., Rainie, L., & Luchsinger, A. (2018). Artificial intelligence and the future of humans (pp. 10). Pew Research Center.
- Araujo, T., Helberger, N., Kruikemeier, S., & De Vreese, C. H. (2020). In AI we trust? Perceptions about automated decision-making by artificial intelligence. AI & Society, 35(3), 611–623. https://doi.org/10.1007/s00146-019-00931-w
- Arya, V., Bellamy, R. K., Chen, P.-Y., Dhurandhar, A., Hind, M., Hoffman, S. C., Houde, S., Liao, Q. V., Luss, R., & Mojsilovic, A. (2020). AI explainability 360: An extensible toolkit for understanding data and machine learning models. Journal of Machine Learning Research, 21(130), 1–6. https://doi.org/10.1145/3430984.3430987
- Auernhammer, J. (2020, 11–14 August). Human-centered AI: The role of human-centered design research in the development of AI. In S. Boess, M. Cheung, and R. Cain (Eds.), Synergy – DRS International Conference 2020. https://doi.org/10.21606/drs.2020.282
- Awad, E., Anderson, M., Anderson, S. L., & Liao, B. (2020). An approach for combining ethical principles with public opinion to guide public policy. Artificial Intelligence, 287, 103349. https://doi.org/10.1016/j.artint.2020.103349
- Aynsley, C. (2020). The online harms bills cannot wait. Infosecurity Magazine. https://www.infosecurity-magazine.com/opinions/online-harms-bill-wait/
- Balaji, T., Annavarapu, C. S. R., & Bablani, A. (2021). Machine learning algorithms for social media analysis: A survey. Computer Science Review, 40, 100395. https://doi.org/10.1016/j.cosrev.2021.100395
- Barredo Arrieta, A., Díaz-Rodríguez, N., Del Ser, J., Bennetot, A., Tabik, S., Barbado, A., Garcia, S., Gil-Lopez, S., Molina, D., Benjamins, R., Chatila, R., & Herrera, F. (2020). Explainable Artificial Intelligence (XAI): Concepts, taxonomies, opportunities and challenges toward responsible AI. Information Fusion, 58, 82–115. https://doi.org/10.48550/arXiv.1910.10045
- Bayern, S. (2016). The implications of modern business–entity law for the regulation of autonomous systems. European Journal of Risk Regulation, 7(2), 297–309. https://doi.org/10.1017/S1867299X00005729
- Becerra-Perez, M. M., Menear, M., Turcotte, S., Labrecque, M., & Legare, F. (2016). More primary care patients regret health decisions if they experienced decisional conflict in the consultation: A secondary analysis of a multicenter descriptive study. BMC Family Practice, 17(1), 156. https://doi.org/10.1186/s12875-016-0558-0
- Bellamy, R. K., Dey, K., Hind, M., Hoffman, S. C., Houde, S., Kannan, K., Lohia, P., Martino, J., Mehta, S., & Mojsilovic, A. (2018). AI Fairness 360: An extensible toolkit for detecting, understanding, and mitigating unwanted algorithmic bias. arXiv preprint arXiv:1810.01943. https://doi.org/10.48550/arXiv.1810.01943
- Berghel, H. (2017). Net neutrality reloaded. Computer Magazine. 50(10), 68–72. https://doi.org/10.1109/mc.2017.3641632
- Berndt, J. O., Rodermund, S. C., Lorig, F., & Timm, I. J. (2017). Modeling user behavior in social media with complex agents [Paper presentation]. Third International Conference on Human and Social Analytics (HUSO 2017), Nice, France.
- Bertolini, A. (2013). Robots as products: The case for a realistic analysis of robotic applications and liability rules. Law, Innovation and Technology, 5(2), 214–247. https://doi.org/10.5235/17579961.5.2.214
- Bessi, A., & Ferrara, E. (2016). Social bots distort the 2016 US Presidential election online discussion. First Monday, 21, 11–17. https://doi.org/10.5210/fm.v21i11.7090
- Bhutta, N., Hizmo, A., & Ringo, D. (2021). How much does racial bias affect mortgage lending? Evidence from human and algorithmic credit decisions. SSRN Electronic Journal. https://doi.org/10.2139/ssrn.3887663
- Błachnio, A., Przepiorka, A., & Pantic, I. (2016). Association between Facebook addiction, self-esteem and life satisfaction: A cross-sectional study. Computers in Human Behavior, 55, 701–705. https://doi.org/10.1016/j.chb.2015.10.026
- Bodenschatz, A., Uhl, M., & Walkowitz, G. (2021). Autonomous systems in ethical dilemmas: Attitudes toward randomization. Computers in Human Behavior Reports, 4, 100145. https://doi.org/10.1016/j.chbr.2021.100145
- Bond, R. R., Mulvenna, M. D., Wan, H., Finlay, D. D., Wong, A., Koene, A., Brisk, R., Boger, J., & Adel, T. (2019). Human centered artificial intelligence: Weaving UX into algorithmic decision making. RoCHI.
- Braun, M., Hummel, P., Beck, S., & Dabrock, P. (2021). Primer on an ethics of AI-based decision support systems in the clinic. Journal of Medical Ethics, 47(12), e3–e3. https://doi.org/10.1136/medethics-2019-105860
- Brennan, P. F. (2021, October 8). Bridge to artificial intelligence (Bridge2AI). https://commonfund.nih.gov/bridge2ai
- Brown, B., Bødker, S., & Höök, K. (2017). Does HCI scale? Scale hacking and the relevance of HCI. Interactions, 24(5), 28–33. https://doi.org/10.1145/3125387
- Brynjolfsson, E., & McAfee, A. (2014). The second machine age: Work, progress, and prosperity in a time of brilliant technologies. WW Norton & Company.
- Brynjolfsson, E., Mitchell, T., & Rock, D. (2018). What can machines learn, and what does it mean for occupations and the economy? AEA Papers and Proceedings, 108, 43–47. https://doi.org/10.1257/pandp.20181019
- Burzagli, L., Emiliani, P. L., Antona, M., & Stephanidis, C. (2022). Intelligent environments for all: A path towards technology-enhanced human well-being. Universal Access in the Information Society, 21(2), 437–456. https://doi.org/10.1007/s10209-021-00797-0
- Bush, V. (2020). Science, the Endless Frontier. Princeton University Press.
- Business Roundtable (2019, August 19). Statement on the purpose of a corporation. https://s3.amazonaws.com/brt.org/BRT-StatementonthePurposeofaCorporationJuly2021.pdf.
- Business Roundtable (2022, January 26). Roadmap for responsible artificial intelligence (AI) https://www.businessroundtable.org/policy-perspectives/technology/ai.
- Cadbury, A. (2000). Foreword (corporate governance: A framework for implementation. The World Bank Group.
- Cai, C. J., Reif, E., Hegde, N., Hipp, J., Kim, B., Smilkov, D., Wattenberg, M., Viegas, F., Corrado, G. S., Stumpe, M. C., & Terry, M. (2019). Human-centered tools for coping with imperfect algorithms during medical decision-making [Paper presentation]. Proceedings of the 2019 CHI Conference on Human Factors in Computing Systems, 1–14, Glasgow, Scotland, UK. https://doi.org/10.1145/3290605.3300234
- Cai, H., Gan, C., Wang, T., Zhang, Z., & Han, S. (2020). Once for all: Train one network and specialize it for efficient deployment [Paper presentation]. International Conference on Learning Representations. https://arxiv.org/pdf/1908.09791.pdf
- Calders, T., & Verwer, S. (2010). Three naive Bayes approaches for discrimination-free classification. Data Mining and Knowledge Discovery, 21(2), 277–292. https://doi.org/10.1007/s10618-010-0190-x
- Calmon, F., Wei, D., Vinzamuri, B., Natesan Ramamurthy, K., & Varshney, K. R. (2017). Optimized pre-processing for discrimination prevention. Advances in Neural Information Processing Systems, 30. https://doi.org/10.48550/arXiv.1704.03354
- Calvo, R. A., & Peters, D. (2014). Positive computing. MIT Press.
- Calvo, R. A., Peters, D., Vold, K., & Ryan, R. M. (2020). Supporting human autonomy in AI systems: A framework for ethical enquiry. In Ethics of digital well-being (pp. 31–54). Springer.
- Cambridge University Press, Ed. (2022). Cambridge dictionary.
- Carlsmith, K. M., & Darley, J. M. (2008). Psychological aspects of retributive justice. Advances in Experimental Social Psychology, 40, 193–236. https://doi.org/10.1016/S0065-2601(07)00004-4
- Carr, S. (2020). ‘AI gone mental’: Engagement and ethics in data-driven technology for mental health (Vol. 29, pp.125–130). Taylor & Francis.
- Cavoukian, A. (2009). Privacy by design: The 7 foundational principles. Information and Privacy Commissioner of Ontario, Canada, (5), 12.
- Chander, A., & Krishnamurthy, V. (2018). The myth of platform neutrality. Georgetown Law Technology Review, 2, 400.
- Cheatham, B., Javanmardian, K., & Samandari, H. (2019). Confronting the risks of artificial intelligence. McKinsey Quarterly, 2, 38.
- Chen, Y., Mark, G., Ali, S., & Ma, X. (2017). Unpacking happiness: Lessons from smartphone photography among college students. 429–438. https://doi.org/10.1109/ICHI.2017.25
- Chinese State Council. (2017). Notice of the state council issuing the new generation of artificial intelligence development plan. https://flia.org/wp-content/uploads/2017/07/A-New-Generation-of-Artificial-Intelligence-Development-Plan-1.pdf.
- Cho, J., Hwang, G., & Suh, C. (2020). A fair classifier using kernel density estimation. In H. Larochelle, M. Ranzato, R. Hadsell, M. F. Balcan, & H. Lin (Eds.), Advances in neural information processing systems (Vol. 33, pp. 15088–15099). Curran Associates, Inc. https://proceedings.neurips.cc/paper/2020/file/ac3870fcad1cfc367825cda0101eee62-Paper.pdf
- Chouldechova, A. (2017). Fair prediction with disparate impact: A study of bias in recidivism prediction instruments. Big Data, 5(2), 153–163. https://doi.org/10.1089/big.2016.0047
- CIFAR. (2022). Pan-Canadian AI strategy. https://cifar.ca/ai/
- Corbett-Davies, S., Pierson, E., Feller, A., Goel, S., & Huq, A. (2017). Algorithmic decision making and the cost of fairness [Paper presentation]. Proceedings of the 23rd ACM SIGKDD International Conference on Knowledge Discovery and Data Mining, 797–806, Halifax NS Canada. https://doi.org/10.1145/3097983.3098095
- Creemers, R. (2018). China’s social credit system: An evolving practice of control. SSRN Electronic Journal, https://doi.org/10.2139/ssrn.3175792
- Croskerry, P., Singhal, G., & Mamede, S. (2013). Cognitive debiasing 1: Origins of bias and theory of debiasing. BMJ Qual Saf, 22 (Suppl 2), ii58–ii64. https://doi.org/10.1136/bmjqs-2012-001712
- Danaher, J. (2016). Robots, law and the retribution gap. Ethics and Information Technology, 18(4), 299–309. https://doi.org/10.1007/s10676-016-9403-3
- Danks, D., & London, A. J. (2017). Algorithmic bias in autonomous systems. IJCAI.
- De Cremer, D., & Kasparov, G. (2021). AI should augment human intelligence, not replace it. Harvard Business Review
- De Freitas, J., & Cikara, M. (2021). Deliberately prejudiced self-driving vehicles elicit the most outrage. Cognition, 208, 104555. https://doi.org/10.1016/j.cognition.2020.104555
- De Freitas, J., Anthony, S. E., Censi, A., & Alvarez, G. A. (2020). Doubting driverless dilemmas. Perspectives on Psychological Science, 15(5), 1284–1288. https://doi.org/10.1177/1745691620922201
- Desouza, K. C., Dawson, G. S., & Chenok, D. (2020). Designing, developing, and deploying artificial intelligence systems: Lessons from and for the public sector. Business Horizons, 63(2), 205–213. https://doi.org/10.1016/j.bushor.2019.11.004
- Diener, E., Scollon, C. N., & Lucas, R. E. (2009). The evolving concept of subjective well-being: The multifaceted nature of happiness. https://doi.org/10.1007/978-90-481-2354-4_4
- Dietrich, E., & Fields, C. (1989). Experimental and theoretical artificial intelligence. Journal of Experimental & Theoretical Artificial Intelligence, 1(1), 1–4. https://doi.org/10.1080/09528138908953690
- Dubé, L., Du, P., McRae, C., Sharma, N., Jayaraman, S., & Nie, J.-Y. (2018). Convergent innovation in food through big data and artificial intelligence for societal-scale inclusive growth. Technology Innovation Management Review, 8(2), 49–65. https://doi.org/10.22215/timreview/1139
- Dumon, J., Goodman, B., Kirechu, P., Smith, C., Van Deusen, A. (2021). Responsible AI guidelines in practice. Lessons learned from the DIU portfolio. Defense Innovation Unit. https://www.diu.mil/responsible-ai-guidelines.
- Dwivedi, Y. K., Hughes, L., Ismagilova, E., Aarts, G., Coombs, C., Crick, T., Duan, Y., Dwivedi, R., Edwards, J., Eirug, A., Galanos, V., Ilavarasan, P. V., Janssen, M., Jones, P., Kar, A. K., Kizgin, H., Kronemann, B., Lal, B., Lucini, B., … Williams, M. D. (2021). Artificial Intelligence (AI): Multidisciplinary perspectives on emerging challenges, opportunities, and agenda for research, practice and policy. International Journal of Information Management, 57, 101994. https://doi.org/10.1016/j.ijinfomgt.2019.08.002
- Ehsan, U., & Riedl, M. O. (2020, 19–24 July). Human-centered explainable AI: Towards a reflective sociotechnical approach [Paper presentation]. HCI International 2020 – Late Breaking Papers: Multimodality and Intelligence: 22nd HCI International Conference, HCII 2020, Copenhagen, Denmark, 449–466. https://doi.org/10.1007/978-3-030-60117-1_33
- Emami-Naeini, P., Agarwal, Y., Faith Cranor, L., & Hibshi, H. (2020). Ask the experts: What should be on an IoT privacy and security label? [Paper presentation]. 2020 IEEE Symposium on Security and Privacy (SP), 447–464, San Francisco, California. https://doi.org/10.1109/SP40000.2020.00043
- Engelmann, S., Chen, M., Fischer, F., Kao, C., & Grossklags, J. (2019). Clear sanctions, vague rewards: How China’s social credit system currently defines “good” and “bad” behavior [Paper presentation]. Proceedings of the Conference on Fairness, Accountability, and Transparency, 69–78. https://doi.org/10.1145/3287560.3287585
- Ernst, E., Merola, R., & Samaan, D. (2019). Economics of artificial intelligence: Implications for the future of work. IZA Journal of Labor Policy, 9(1). https://doi.org/10.2478/izajolp-2019-0004
- European Commission (2019, April 8). Ethics guidelines for trustworthy artificial intelligence. FUTURIUM – European Commission. https://ec.europa.eu/futurium/en/ai-alliance-consultation
- European Commission (2021, December 15). EU industrial R&D investment scoreboard 2020. https://iri.jrc.ec.europa.eu/scoreboard/2020-eu-industrial-rd-investment-scoreboard
- European Commission. (2021). Proposal for a regulation of the European Parliament and of the Council laying down harmonised rules on artificial intelligence (Artificial Intelligence Act) and amending certain Union legislative acts. The Artificial Intelligence Act. https://artificialintelligenceact.eu/
- European Commission and High Representative of the Union for Foreign Affairs and Security Policy. (2020, December 2). A new EU-US agenda for global change. https://ec.europa.eu/info/sites/info/files/joint-communication-eu-us-agenda_en.pdf.
- European Digital SME Alliance. (2021). Feedback from: European Digital SME (small and medium enterprises) alliance. https://ec.europa.eu/info/law/better-regulation/have-your-say/initiatives/12527-Artificial-intelligence-ethical-and-legal-requirements/F2665574_en.
- European Parliament. (2022, October 2). Special committee on artificial intelligence in a digital age. https://multimedia.europarl.europa.eu/en/webstreaming/aida-committee-meeting_20220210-0900-COMMITTEE-AIDA
- Falco, G., Shneiderman, B., Badger, J., Carrier, R., Dahbura, A., Danks, D., Eling, M., Goodloe, A., Gupta, J., Hart, C., Jirotka, M., Johnson, H., LaPointe, C., Llorens, A. J., Mackworth, A. K., Maple, C., Pálsson, S. E., Pasquale, F., Winfield, A., & Yeong, Z. K. (2021). Governing AI safety through independent audits. Nature Machine Intelligence, 3(7), 566–571. https://doi.org/10.1038/s42256-021-00370-7
- Falk-Krzesinski, H. J., Contractor, N., Fiore, S. M., Hall, K. L., Kane, C., Keyton, J., Klein, J. T., Spring, B., Stokols, D., & Trochim, W. (2011). Mapping a research agenda for the science of team science. Research Evaluation, 20(2), 145–158. https://doi.org/10.3152/095820211X12941371876580
- Fazelpour, S., & Danks, D. (2021). Algorithmic bias: Senses, sources, solutions. Philosophy Compass, 16(8), e12760. https://doi.org/10.1111/phc3.12760
- Feldman, M., Friedler, S. A., Moeller, J., Scheidegger, C., & Venkatasubramanian, S. (2015). Certifying and removing disparate impact [Paper presentation]. Proceedings of the 21th ACM SIGKDD International Conference on Knowledge Discovery and Data Mining. Sydney NSW Australia.
- Fernandez-Caballero, A., Martinez-Rodrigo, A., Pastor, J. M., Castillo, J. C., Lozano-Monasor, E., Lopez, M. T., Zangroniz, R., Latorre, J. M., & Fernandez-Sotos, A. (2016). Smart environment architecture for emotion detection and regulation. Journal of Biomedical Informatics, 64, 55–73. https://doi.org/10.1016/j.jbi.2016.09.015
- Ferrer, X., Nuenen, T. v., Such, J. M., Cote, M., & Criado, N. (2021). Bias and discrimination in AI: A cross-disciplinary perspective. IEEE Technology and Society Magazine, 40(2), 72–80. https://doi.org/10.1109/mts.2021.3056293
- Fleissner, C. (2018). Inclusive capitalism based on binary economics and positive international human rights in the age of artificial intelligence. Washington University Global Studies Law Review, 17(1), 201.
- Fleming, S. (2021, March 15). What is digital sovereignty and why is Europe so interested in it? https://www.weforum.org/agenda/2021/03/europe-digital-sovereignty/.
- Floridi, L., Cowls, J., Beltrametti, M., Chatila, R., Chazerand, P., Dignum, V., Luetge, C., Madelin, R., Pagallo, U., Rossi, F., Schafer, B., Valcke, P., & Vayena, E. (2018). AI4People-an ethical framework for a good AI society: Opportunities, risks, principles, and recommendations. Minds and Machines, 28(4), 689–707. https://doi.org/10.1007/s11023-018-9482-5
- Floridi, L., Holweg, M., Taddeo, M., Amaya Silva, J., Mökander, J., & Wen, Y. (2022). capAI – a procedure for conducting conformity assessment of AI systems in line with the EU Artificial Intelligence Act. SSRN Electronic Journal, https://doi.org/10.2139/ssrn.4064091
- ForHumanity. (2022). Independent Audit of AI Systems (IAAIS) https://forhumanity.center/blog/auditing-ai-and-autonomous-systems-building-an-infrastructureoftrust/.
- Foundation for Responsible Robotics. (2022). FRR quality mark for (AI based) robotics. https://responsiblerobotics.org/quality-mark/.
- Freitag, C., Berners-Lee, M., Widdicks, K., Knowles, B., Blair, G. S., & Friday, A. (2021). The real climate and transformative impact of ICT: A critique of estimates, trends, and regulations. Patterns, 2(9), 100340. https://doi.org/10.1016/j.patter.2021.100340
- Frischmann, B., & Selinger, E. (2018). Re-engineering humanity. Cambridge University Press.
- Garibay, O., & Winslow, B. (2021, 26–27 July). HCII2021 special thematic sessions on “human-centered AI”. HCI International 2021, Washington DC, USA. https://2021.hci.international/Human-Centered_AI_Thematic_Sessions.html
- General Data Protection Regulation 679 CFR 2016. (2016). Regulation (EU) 2016/679 of the European Parliament and of the Council of 27 April 2016 on the protection of natural persons with regard to the processing of personal data and on the free movement of such data, and repealing Directive 95/46/EC (General Data Protection Regulation) (Text with EEA relevance). Official Journal, L 119, 1–88. ELI: http://data.europa.eu/eli/reg/2016/679/oj[legislation
- Ghassemi, M., Oakden-Rayner, L., & Beam, A. L. (2021). The false hope of current approaches to explainable artificial intelligence in health care. The Lancet Digital Health, 3(11), e745–e750. https://doi.org/10.1016/S2589-7500(21)00208-9
- Ghosh, I. (2015). Social wealth economic indicators for a caring economy. Interdisciplinary Journal of Partnership Studies, 1(1). https://doi.org/10.24926/ijps.v1i1.90
- Gibbons, R. (1998). Incentives in organizations. Journal of Economic Perspectives, 12(4), 115–132. https://doi.org/10.1257/jep.12.4.115
- Gless, S., Silverman, E., & Weigend, T. (2016). If robots cause harm, who is to blame? Self-driving cars and criminal liability. New Criminal Law Review, 19(3), 412–436. https://doi.org/10.1525/nclr.2016.19.3.412
- Gong, J., Currano, R., Sirkin, D., Yeung, S., & Holsinger, F. C. (2021, 08–09 May). NICE: Four human-centered AI principles for bridging the AI-to-clinic translational gap [Paper presentation]. Virtual ’21: ACM CHI Workshop on Realizing AI in Healthcare: Challenges Appearing in the Wild, 202, Virtual. ACM.
- Goodfellow, I., Pouget-Abadie, J., Mirza, M., Xu, B., Warde-Farley, D., Ozair, S., Courville, A., & Bengio, Y. (2020). Generative adversarial networks. Communications of the ACM, 63(11), 139–144. https://doi.org/10.1145/3422622
- Google. (2022). People + AI guidebook. https://pair.withgoogle.com/guidebook/.
- Google AI. (2022). Responsible AI practices. https://ai.google/responsibilities/responsible-ai-practices.
- Grace, K., Salvatier, J., Dafoe, A., Zhang, B., & Evans, O. (2018). Viewpoint: When will AI exceed human performance? Evidence from AI experts. Journal of Artificial Intelligence Research, 62, 729–754. https://doi.org/10.1613/jair.1.11222
- Grayson, M., Thieme, A., Marques, R., Massiceti, D., Cutrell, E., & Morrison, C. (2020). A dynamic AI system for extending the capabilities of blind people [Paper presentation]. Extended Abstracts of the 2020 CHI Conference on Human Factors in Computing Systems, 1–4, Honolulu, Hawaiʻi, USA. https://doi.org/10.1145/3334480.3383142
- Groce, A., Kulesza, T., Zhang, C., Shamasunder, S., Burnett, M., Wong, W.-K., Stumpf, S., Das, S., Shinsel, A., Bice, F., & McIntosh, K. (2014). You are the only possible oracle: Effective test selection for end users of interactive machine learning systems. IEEE Transactions on Software Engineering, 40(3), 307–323. https://doi.org/10.1109/TSE.2013.59
- Grossman, Z., & van der Weele, J. J. (2017). Self-image and willful ignorance in social decisions. Journal of the European Economic Association, 15(1), 173–217. https://doi.org/10.1093/jeea/jvw001
- Gunning, D., & Aha, D. (2019). DARPA’s explainable artificial intelligence (XAI) program. AI Magazine, 40(2), 44–58. https://doi.org/10.1145/3301275.3308446
- Gupta, A. (2021, September 18). The imperative for sustainable AI systems. The Gradient. https://thegradient.pub/sustainable-ai/.
- Gupta, A., Lanteigne, C., & Kingsley, S. (2020). SECure: A social and environmental certificate for AI systems. https://doi.org/10.48550/arXiv.2006.06217
- Guy, I. (2015). Social recommender systems (recommender systems handbook) (pp. 511–543). Springer.
- Hallevy, G. (2010). I, Robot-I, criminal: When science fiction becomes reality: Legal liability of AI robots committing criminal offenses. Syracuse Journal of Science & Technology Law Reporter, 1
- Han, S., Kelly, E., Nikou, S., & Svee, E.-O. (2022). Aligning artificial intelligence with human values: Reflections from a phenomenological perspective. AI & Society, 37(4), 1383–1395. https://doi.org/10.1007/s00146-021-01247-4
- Hao, K. (2020). Doctors are using AI to triage covid-19 patients. The tools may be here to stay. MIT Technology Review, 27, 1–12.
- Hardt, M., Price, E., & Srebro, N. (2016). Equality of opportunity in supervised learning. Advances in Neural Information Processing Systems, 29. https://doi.org/10.48550/arXiv.1610.02413
- Harper, F. M., & Konstan, J. A. (2016). The MovieLens datasets. ACM Transactions on Interactive Intelligent Systems, 5(4), 1–19. https://doi.org/10.1145/2827872
- He, X., Hong, Y., Zheng, X., & Zhang, Y. (2022). What are the users’ needs? Design of a user-centered explainable artificial intelligence diagnostic system. International Journal of Human–Computer Interaction, 1–24. https://doi.org/10.1080/10447318.2022.2095093
- Heikkilä, M. (2021, March 29). NATO wants to set AI standards. If only its members agreed on the basics. Politico. https://www.politico.eu/article/nato-ai-artificial-intelligence-standards-priorities.
- Henrich, J., Heine, S. J., & Norenzayan, A. (2010). The weirdest people in the world? The Behavioral and Brain Sciences, 33(2–3), 61–83. https://doi.org/10.1017/S0140525X0999152X
- Himmelreich, J. (2018). Never mind the trolley: The ethics of autonomous vehicles in mundane situations. Ethical Theory and Moral Practice, 21(3), 669–684. https://doi.org/10.1007/s10677-018-9896-4
- Ho, D.-A., & Beyan, O. (2020). Biases in data science lifecycle. arXiv preprint arXiv:2009.09795. https://doi.org/10.48550/arXiv.2009.09795
- Hodge, R., Rotner, J., Baron, I., Kotras, D., & Worley, D. (2020). Designing a new narrative to build an AI-ready workforce. MITRE Center for Technology and National Security. https://www.mitre.org/sites/default/files/2021-11/prs-20-0975-designing-a-new-narrative-to-build-an-AI-ready-workforce.pdf
- Hoffman, R. R., Mueller, S. T., Klein, G., & Litman, J. (2018). Metrics for explainable AI: Challenges and prospects. arXiv preprint arXiv:1812.04608. http://arxiv.org/abs/1812.04608
- Hoffmann, A. L. (2021). Terms of inclusion: Data, discourse, violence. New Media & Society, 23(12), 3539–3556. https://doi.org/10.1177/1461444820958725
- Holmquist, L. E. (2017). Intelligence on tap: Artificial intelligence as a new design material. Interactions, 24(4), 28–33. https://doi.org/10.1145/3085571
- Huang, L., Jiang, S., & Vishnoi, N. (2019). Coresets for clustering with fairness constraints. Advances in Neural Information Processing Systems, 32. https://doi.org/10.48550/arXiv.1906.08484
- Hubbard, F. P. (2014). Sophisticated robots: Balancing liability, regulation, and innovation. Florida Law Review, 66(5), 1803.
- Humble, N., & Mozelius, P. (2019, 31 October–1 November 2019). Artificial intelligence in education – A promise, a threat or a hype? [Paper presentation]. Proceedings of the European Conference on the Impact of Artificial Intelligence and Robotics, EM-Normandie Business School Oxford, UK, 149–156. https://doi.org/10.34190/ECIAIR.19.005
- IEEE. (2019a). Classical ethics in A/IS. In M. I. Aldinhas Ferreira, J. Silva Sequeira, G. Singh Virk, M. O. Tokhi, & E. E. Kadar (Eds.), The IEEE global initiative on ethics of autonomous and intelligent systems (Vol. 95, pp. 11–16). Springer International Publishing. https://doi.org/10.1007/978-3-030-12524-0_2
- IEEE. (2019b). Ethics in action in autonomous and intelligent systems|IEEE SA. [Paper presentation]. Ethics in Action | Ethically Aligned Design. https://ethicsinaction.ieee.org/
- Ikwuegbu, I. (2021). AI, automation and the future of work (a research essay).
- ISO 9241-11. (2018). Ergonomics of human-system interaction—part 11: Usability: Definitions and concepts. International Organization for Standardization Geneva.
- ISO 9241-210. (2019). Ergonomics of human-system interaction: Part 210: Human-centred design for interactive systems. ISO.
- Iyengar, S., & Massey, D. S. (2019). Scientific communication in a post-truth society. Proceedings of the National Academy of Sciences of the United States of America, 116(16), 7656–7661. https://doi.org/10.1073/pnas.1805868115
- Jacko, J. A. (2012). Human computer interaction handbook: Fundamentals, evolving technologies, and emerging applications. CRC press.
- Jillson, E. (2021). Aiming for truth, fairness, and equity in your company’s use of AI. FTC Bureau of Consumer Protection https://www.ftc.gov/news-events/blogs/business-blog/2021/04/aiming-truth-fairness-equity-your-companys-use-ai.
- Johnson, M., & Vera, A. (2019). No AI is an island: The case for teaming intelligence. AI Magazine, 40(1), 16–28. https://doi.org/10.1609/aimag.v40i1.2842
- Joseph-Williams, N., Edwards, A., & Elwyn, G. (2011). The importance and complexity of regret in the measurement of 'good’ decisions: A systematic review and a content analysis of existing assessment instruments. Health Expectations, 14(1), 59–83. https://doi.org/10.1111/j.1369-7625.2010.00621.x
- Jun, S., Yuming, W., & Cui, H. (2021). An integrated analysis framework of artificial intelligence social impact based on application scenarios. Science of Science and Management of Science & Technology, 42(05), 3.
- Kallioras, N. A., & Lagaros, N. D. (2020). DzAIℕ: Deep learning based generative design. Procedia Manufacturing, 44, 591–598. https://doi.org/10.1016/j.promfg.2020.02.251
- Kamiran, F., & Calders, T. (2012). Data preprocessing techniques for classification without discrimination. Knowledge and Information Systems, 33(1), 1–33. https://doi.org/10.1007/s10115-011-0463-8
- Kamishima, T., Akaho, S., Asoh, H., & Sakuma, J. (2012). Fairness-aware classifier with prejudice remover regularizer. In Flach, P.A., De Bie, T., Cristianini, N. (Eds.), Machine learning and knowledge discovery in databases. ECML PKDD 2012. Lecture Notes in Computer Science (Vol 7524). Springer. https://doi.org/10.1007/978-3-642-33486-3_3
- Kane, S. K., Guo, A., & Morris, M. R. (2020). Sense and accessibility: Understanding people with physical disabilities’ experiences with sensing systems [Paper presentation]. Proceedings of the 22nd International ACM SIGACCESS Conference on Computers and Accessibility, 1–14, Virtual Event Greece. https://doi.org/10.1145/3373625.3416990
- Karwowski, W. (2018, August 26). The human use of artificial intelligence. Keynote address. 20th Congress of the International Ergonomics Association. Florence, Italy.
- Karwowski, W., & Zhang, W. (2021). Human factors and ergonomics (handbook of human factors and ergonomics). Wiley.
- Kelley, P. G., Bresee, J., Cranor, L. F., & Reeder, R. W. (2009). A “nutrition label” for privacy [Paper presentation]. Proceedings of the 5th Symposium on Usable Privacy and Security. Mountain View, CA.
- Kelley, P. G., Yang, Y., Heldreth, C., Moessner, C., Sedley, A., Kramm, A., Newman, D. T., & Woodruff, A. (2021). Exciting, useful, worrying, futuristic: Public perception of artificial intelligence in 8 countries [Paper presentation]. Proceedings of the 2021 AAAI/ACM Conference on AI, Ethics, and Society. Virtual Event, USA.
- Keyes, C. L. (2002). The mental health continuum: From languishing to flourishing in life. Journal of Health and Social Behavior, 43(2), 207–222. https://doi.org/10.2307/3090197
- Kirchkamp, O., & Strobel, C. (2019). Sharing responsibility with a machine. Journal of Behavioral and Experimental Economics, 80, 25–33. https://doi.org/10.1016/j.socec.2019.02.010
- Kleppe, M., & Otte, M. (2017). Analysing and understanding news consumption patterns by tracking online user behaviour with a multimodal research design. Digital Scholarship in the Humanities, 32(suppl_2), ii158–ii170. https://doi.org/10.1093/llc/fqx030
- Klinova, K., & Korinek, A. (2021). AI and shared prosperity [Paper presentation]. Proceedings of the 2021 AAAI/ACM Conference on AI, Ethics, and Society. Virtual Event, USA.
- Kokolakis, S. (2017). Privacy attitudes and privacy behaviour: A review of current research on the privacy paradox phenomenon. Computers & Security, 64, 122–134. https://doi.org/10.1016/j.cose.2015.07.002
- Komal, S. (2014). Comparative assessment of human intelligence and artificial intelligence. International Journal of Computer Science and Mobile Computing, 3, 1–5.
- Kramer, A. D., Guillory, J. E., & Hancock, J. T. (2014). Experimental evidence of massive-scale emotional contagion through social networks. Proceedings of the National Academy of Sciences of the United States of America, 111(24), 8788–8790. https://doi.org/10.1073/pnas.1320040111
- Krügel, S., Ostermaier, A., & Uhl, M. (2022). Zombies in the loop? Humans trust untrustworthy AI-advisors for ethical decisions. Philosophy & Technology, 35(1), 1–37. https://doi.org/10.1007/s13347-022-00511-9
- Kuhlmann, S., Stegmaier, P., & Konrad, K. (2019). The tentative governance of emerging science and technology—a conceptual introduction. Research Policy, 48(5), 1091–1097. https://doi.org/10.1016/j.respol.2019.01.006
- Kuzma, J. (2022). Implementing responsible research and innovation: A case study of US biotechnology oversight. Global Public Policy and Governance, 2(3), 306–325. https://doi.org/10.1007/s43508-022-00046-x
- Lambrecht, A., & Tucker, C. (2019). Algorithmic bias? An empirical study of apparent gender-based discrimination in the display of STEM career ads. Management Science, 65(7), 2966–2981. https://doi.org/10.1287/mnsc.2018.3093
- Langley, P. (2019). An integrative framework for artificial intelligence education [Paper presentation]. Proceedings of the AAAI Conference on Artificial Intelligence. Honolulu, HI, USA.
- Lannelongue, L., Grealey, J., Bateman, A., & Inouye, M. (2021). Ten simple rules to make your computing more environmentally sustainable (vol. 17, p. e1009324). Public Library of Science San Francisco.
- Latonero, M. (2011). Human trafficking online: The role of social networking sites and online classifieds. SSRN Electronic Journal, https://doi.org/10.2139/ssrn.2045851
- Lazar, J. (2007). Universal usability: Designing computer interfaces for diverse user populations. John Wiley & Sons.
- Lazer, D. M. J., Baum, M. A., Benkler, Y., Berinsky, A. J., Greenhill, K. M., Menczer, F., Metzger, M. J., Nyhan, B., Pennycook, G., Rothschild, D., Schudson, M., Sloman, S. A., Sunstein, C. R., Thorson, E. A., Watts, D. J., & Zittrain, J. L. (2018). The science of fake news. Science, 359(6380), 1094–1096. https://doi.org/10.1126/science.aao2998
- Le Métayer, D. (2013). Privacy by design: A formal framework for the analysis of architectural choices [Paper presentation]. Proceedings of the Third ACM Conference on Data and Application Security and Privacy. San Antonio, TX, USA.
- Le, Q., Miralles-Pechuán, L., Kulkarni, S., Su, J., & Boydell, O. (2020). An overview of deep learning in industry. Data Analytics and AI, 65–98. https://doi.org/10.1201/9781003019855-5
- Lee, I., & Shin, Y. J. (2020). Machine learning for enterprises: Applications, algorithm selection, and challenges. Business Horizons, 63(2), 157–170. https://doi.org/10.1016/j.bushor.2019.10.005
- Leonidis, A., Korozi, M., Sykianaki, E., Tsolakou, E., Kouroumalis, V., Ioannidi, D., Stavridakis, A., Antona, M., & Stephanidis, C. (2021). Improving stress management and sleep hygiene in intelligent homes. Sensors, 21(7), 2398. https://doi.org/10.3390/s21072398
- Leslie, D., Burr, C., Aitken, M., Cowls, J., Katell, M., & Briggs, M. (2021). Artificial intelligence, human rights, democracy, and the rule of law: A primer. The Council of Europe.
- Liang, F., Das, V., Kostyuk, N., & Hussain, M. M. (2018). Constructing a data-driven society: China’s social credit system as a state surveillance infrastructure. Policy & Internet, 10(4), 415–453. https://doi.org/10.1002/poi3.183
- Lieberman, H. (2009). User interface goals, AI opportunities. AI Magazine, 30(4), 16–16. https://doi.org/10.1609/aimag.v30i4.2266
- Lindebaum, D., Vesa, M., & den Hond, F. (2020). Insights from “the machine stops” to better understand rational assumptions in algorithmic decision making and its implications for organizations. Academy of Management Review, 45(1), 247–263. https://doi.org/10.5465/amr.2018.0181
- Lipson, H., & Pollack, J. B. (2000). Automatic design and manufacture of robotic lifeforms. Nature, 406(6799), 974–978. https://doi.org/10.1038/35023115
- Liu, X., & Pan, H. (2022). The path of film and television animation creation using virtual reality technology under the artificial intelligence. Scientific Programming, 2022, 1–8. https://doi.org/10.1155/2022/1712929
- Logg, J. M., Minson, J. A., & Moore, D. A. (2019). Algorithm appreciation: People prefer algorithmic to human judgment. Organizational Behavior and Human Decision Processes, 151, 90–103. https://doi.org/10.1016/j.obhdp.2018.12.005
- López-González, M. (2021). Applying human cognition to assured autonomy. International Conference on Human-Computer Interaction. Virtual Event, USA.
- Louizos, C., Swersky, K., Li, Y., Welling, M., & Zemel, R. (2015). The variational fair autoencoder. arXiv preprint arXiv:1511.00830.
- Luan, H., Geczy, P., Lai, H., Gobert, J., Yang, S. J. H., Ogata, H., Baltes, J., Guerra, R., Li, P., & Tsai, C. C. (2020). Challenges and future directions of big data and artificial intelligence in education. Frontiers in Psychology, 11, 580820. https://doi.org/10.3389/fpsyg.2020.580820
- Luong, B. T., Ruggieri, S., & Turini, F. (2011). k-NN as an implementation of situation testing for discrimination discovery and prevention [Paper presentation]. Proceedings of the 17th ACM SIGKDD International Conference on Knowledge Discovery and Data Mining. San Diego, CA, USA.
- Madaio, M. A., Stark, L., Wortman Vaughan, J., & Wallach, H. (2020). Co-designing checklists to understand organizational challenges and opportunities around fairness in AI [Paper presentation]. Proceedings of the 2020 CHI Conference on Human Factors in Computing Systems. Honolulu, HI, USA.
- Makav, B., & Kılıç, V. (2019). Smartphone-based image captioning for visually and hearing impaired [Paper presentation]. 2019 11th International Conference on Electrical and Electronics Engineering (ELECO). Bursa, Turkey.
- Manyika, J., & Sneader, K. (2018). AI, automation, and the future of work: Ten things to solve for. McKinsey & Company. https://www.mckinsey.com/featured-insights/future-of-work/ai-automation-and-the-future-of-work-ten-things-to-solve-for
- Margetis, G., Antona, M., Ntoa, S., & Stephanidis, C. (2012). Towards accessibility in ambient intelligence environments. International Joint Conference on Ambient Intelligence. Pisa, Italy.
- Margetis, G., Ntoa, S., Antona, M., & Stephanidis, C. (2021). Human‐centered design of artificial intelligence. Handbook of Human Factors and Ergonomics, 1085–1106. https://doi.org/10.1002/9781119636113.ch42
- Martelaro, N., & Ju, W. (2017). WoZ Way: Enabling real-time remote interaction prototyping & observation in on-road vehicles [Paper presentation]. Proceedings of the 2017 ACM Conference on Computer Supported Cooperative Work and Social Computing. Portland, OR, USA.
- Martin, B. (2021). CLAIRE – Confederation of Laboratories for Artificial Intelligence. Research in Europe. https://claire-ai.org/
- Martinuzzi, A., Blok, V., Brem, A., Stahl, B., & Schönherr, N. (2018). Responsible research and innovation in industry—challenges, insights and perspectives (VOl. 10, pp.702). MDPI.
- Mayer, A.-S., Strich, F., & Fiedler, M, University of Passau (Germany). (2020). Unintended consequences of introducing AI systems for decision making. MIS Quarterly Executive, 19(4), 239–257. https://doi.org/10.17705/2msqe.00036
- McGregor, S. (2022). AI incident database. https://incidentdatabase.ai.
- Mehrabi, N., Morstatter, F., Saxena, N., Lerman, K., & Galstyan, A. (2021). A survey on bias and fairness in machine learning. ACM Computing Surveys, 54(6), 1–35. https://doi.org/10.1145/3457607
- Mhlambi, S. (2020). From rationality to relationality: Ubuntu as an ethical and human rights framework for artificial intelligence governance [Paper presentation]. Carr Center for Human Rights Policy Discussion Paper Series, vol. 9. Cambridge, MA, USA.
- Miailhe, N. (2018). The geopolitics of artificial intelligence: The return of empires? Politique Étrangère, 83(3), 105–117.
- Microsoft. (2022). Responsible AI. https://www.microsoft.com/en-us/ai/responsible-ai.
- Microsoft News Center. (2022, January 13). Leaders across healthcare, academia and technology form new coalition to transform healthcare journey through responsible AI adoption https://news.microsoft.com/2022/01/13/leaders-across-healthcare-academia-and-technology-form-new-coalition-to-transform-healthcare-journey-through-responsible-ai-adoption/.
- Milano, S., Taddeo, M., & Floridi, L. (2020). Recommender systems and their ethical challenges. AI & Society, 35(4), 957–967. https://doi.org/10.1007/s00146-020-00950-y
- Miller, F. A., Katz, J. H., & Gans, R. (2018). The OD imperative to add inclusion to the algorithms of artificial intelligence. OD Practitioner, 50(1), 8.
- Miller, T. (2019). Explanation in artificial intelligence: Insights from the social sciences. Artificial Intelligence, 267, 1–38. https://doi.org/10.1016/j.artint.2018.07.007
- Misselhorn, C. (2018). Artificial morality. concepts, issues and challenges. Society, 55(2), 161–169. https://doi.org/10.1007/s12115-018-0229-y
- Mitchell, M., Wu, S., Zaldivar, A., Barnes, P., Vasserman, L., Hutchinson, B., Spitzer, E., Raji, I. D., & Gebru, T. (2019). Model cards for model reporting [Paper presentation]. Proceedings of the Conference on Fairness, Accountability, and Transparency. Atlanta, GA, USA.
- Mittelstadt, B. (2019). AI ethics – too principled to fail? SSRN Electronic Journal, 1, 501–507. https://doi.org/10.2139/ssrn.3391293
- Mittelstadt, B. D., Allo, P., Taddeo, M., Wachter, S., & Floridi, L. (2016). The ethics of algorithms: Mapping the debate. Big Data & Society, 3(2), 205395171667967. https://doi.org/10.1177/2053951716679679
- Mohammed, P. S., & Nell’ Watson, E. (2019). Towards inclusive education in the age of artificial intelligence: Perspectives, challenges, and opportunities (artificial intelligence and inclusive education) (pp. 17–37). Springer.
- Mozilla. (2022). Open source audit tooling (OAT) project. https://foundation.mozilla.org/en/what-we-fund/fellowships/oat/.
- Murad, C., & Munteanu, C. (2019). I don’t know what you’re talking about, HALexa” the case for voice user interface guidelines [Paper presentation]. Proceedings of the 1st International Conference on Conversational User Interfaces. Dublin, Ireland.
- Muro, M., Whiton, J., & Maxim, R. (2019). What jobs are affected by AI? Better-paid, better-educated workers face the most exposure. Metropolitan Policy Program Report.
- Murphy, E., Walsh, P. P., & Banerjee, A. (2021). Framework for achieving the environmental sustainable development goals. Environmental Protection Agency.
- Mutlu, E., & Garibay, O. O. (2021). A quantum leap for fairness: Quantum Bayesian approach for fair decision making [Paper presentation]. International Conference on Human-Computer Interaction. Virtual Event, USA.
- Neary, M., & Schueller, S. M. (2018). State of the field of mental health apps. Cognitive and Behavioral Practice, 25(4), 531–537. https://doi.org/10.1016/j.cbpra.2018.01.002
- Nielsen, J. (1994). Usability engineering. Morgan Kaufmann.
- Nissenbaum, H. (1996). Accountability in a computerized society. Science and Engineering Ethics, 2(1), 25–42. https://doi.org/10.1007/bf02639315
- NIST. (2021). AI risk management framework concept paper. https://www.nist.gov/itl/ai-risk-management-framework.
- Ntoa, S., Margetis, G., Antona, M., & Stephanidis, C. (2021a). Digital accessibility in intelligent environments (human-automation interaction: mobile computing). Springer.
- Ntoa, S., Margetis, G., Antona, M., & Stephanidis, C. (2021b). User experience evaluation in intelligent environments: A comprehensive framework. Technologies, 9(2), 41. https://doi.org/10.3390/technologies9020041
- Ntoutsi, E., Fafalios, P., Gadiraju, U., Iosifidis, V., Nejdl, W., Vidal, M. E., Ruggieri, S., Turini, F., Papadopoulos, S., & Krasanakis, E. (2020). Bias in data‐driven artificial intelligence systems—AN introductory survey. Wiley Interdisciplinary Reviews, 10(3), e1356. https://doi.org/10.1002/widm.1356
- Office of the Under Secretary of Defense for Research and Engineering. (2022). USD(R&E) technology vision for an era of competition. https://www.cto.mil/wp-content/uploads/2022/02/usdre_strategic_vision_critical_tech_areas.pdf.
- Office N. A. I. I. (2022). National artificial intelligence initiative https://www.ai.gov/.
- Okamura, K., & Yamada, S. (2020). Adaptive trust calibration for human-AI collaboration. PLOS One, 15(2), e0229132. https://doi.org/10.1371/journal.pone.0229132
- Oliva, E. I. P. N., Gherardi-Donato, E. C. d S., Bermúdez, J. Á., & Facundo, F. R. G. (2018). Use of Facebook, perceived stress and alcohol consumption among university students. Ciencia & Saude Coletiva, 23(11), 3675–3681. https://doi.org/10.1590/1413-812320182311.27132016
- Oliveira, J. D., Couto, J. C., Paixão-Cortes, V. S. M., & Bordini, R. H. (2022). Improving the design of ambient intelligence systems: Guidelines based on a systematic review. International Journal of Human–Computer Interaction, 38(1), 19–27. https://doi.org/10.1080/10447318.2021.1926114
- Olsson, T., & Väänänen, K. (2021). How does AI challenge design practice? Interactions, 28(4), 62–64. https://doi.org/10.1145/3467479
- OpenAI. (2018, May 16). AI and compute. https://openai.com/blog/ai-and-compute/.
- Organisation for Economic Co-operation and Development. (2021). OECD AI principles. https://oecd.ai/en/ai-principles.
- Osmani, N. (2020). The complexity of criminal liability of AI systems. Masaryk University Journal of Law and Technology, 14(1), 53–82. https://doi.org/10.5817/mujlt2020-1-3
- Park, S., Kim, I., Lee, S. W., Yoo, J., Jeong, B., & Cha, M. (2015). Manifestation of depression and loneliness on social networks: A case study of young adults on Facebook [Paper presentation]. Proceedings of the 18th ACM Conference on Computer Supported Cooperative Work & Social Computing. Vancouver, Canada.
- PEGA. (2020). The future of work. https://www.pega.com/future-of-work.
- Peters, D., Calvo, R. A., & Ryan, R. M. (2018). Designing for motivation, engagement and wellbeing in digital experience. Frontiers in Psychology, 9, 797. https://doi.org/10.3389/fpsyg.2018.00797
- Pomerantsev, P. (2019). This is not propaganda: Adventures in the war against reality. PublicAffairs.
- Poquet, O., & Laat, M. (2021). Developing capabilities: Lifelong learning in the age of AI. British Journal of Educational Technology, 52(4), 1695–1708. https://doi.org/10.1111/bjet.13123
- PricewaterhouseCoopers. (2017). Sizing the prize: What’s the real value of ai for your business and how can you capitalise? https://www.pwc.com/gx/en/news-room/docs/report-pwc-ai-analysis-sizing-the-prize.pdf.
- Quy, T. L., Roy, A., Iosifidis, V., & Ntoutsi, E. (2021). A survey on datasets for fairness-aware machine learning. 12(3), e1452. https://doi.org/10.1002/widm.1452
- Rabhi, Y., Mrabet, M., & Fnaiech, F. (2018). A facial expression controlled wheelchair for people with disabilities. Computer Methods and Programs in Biomedicine, 165, 89–105. https://doi.org/10.1016/j.cmpb.2018.08.013
- Rajabi, A., & Garibay, O. O. (2021, July 24–29). Towards fairness in AI: Addressing bias in data using GANs [Paper presentation]. HCI International 2021 – Late Breaking Papers: Multimodality, EXtended Reality, and Artificial Intelligence: 23rd HCI International Conference, HCII 2021, Virtual Event, 509–518. https://doi.org/10.1007/978-3-030-90963-5_39
- Ramsay, G., & Robertshaw, S. (2019). Weaponising news: RT, Sputnik and targeted disinformation. King’s College London Centre for the Study of Media, Communication & Power.
- Raworth, K. (2017). Doughnut economics: Seven ways to think like a 21st-century economist. Chelsea Green Publishing.
- Raworth, K. (2018). A healthy economy should be designed to thrive, not grow. https://www.ted.com/speakers/kate_raworth
- Renz, A., & Vladova, G. (2021). Reinvigorating the discourse on human-centered artificial intelligence in educational technologies. Technology Innovation Management Review, 11(5), 5–16. https://doi.org/10.22215/timreview/1438
- Responsible Artificial Intelligence Institute. (2022). Responsible Artificial Intelligence Institute. https://www.responsible.ai/
- Riedl, M. O. (2019). Human‐centered artificial intelligence and machine learning. Human Behavior and Emerging Technologies, 1(1), 33–36. https://doi.org/10.1002/hbe2.117
- Riva, G., Banos, R. M., Botella, C., Wiederhold, B. K., & Gaggioli, A. (2012). Positive technology: Using interactive technologies to promote positive functioning. Cyberpsychology, Behavior and Social Networking, 15(2), 69–77. https://doi.org/10.1089/cyber.2011.0139
- Roberts, M. (2018). Censored. Princeton University Press.
- Robinson, L., Cotten, S. R., Ono, H., Quan-Haase, A., Mesch, G., Chen, W., Schulz, J., Hale, T. M., & Stern, M. J. (2015). Digital inequalities and why they matter. Information, Communication & Society, 18(5), 569–582. https://doi.org/10.1080/1369118x.2015.1012532
- Rudin, C., & Radin, J. (2019). Why are we using black box models in AI when we don’t need to? A lesson from an explainable AI competition. Harvard Data Science Review, 1(2), 1.2. https://doi.org/10.1162/99608f92.5a8a3a3d
- Rutherglen, G. (1987). Disparate impact under title VII: An objective theory of discrimination. Virginia Law Review, 73(7), 1297. https://doi.org/10.2307/1072940
- Ryan, M. (2022). The social and ethical impacts of artificial intelligence in agriculture: Mapping the agricultural AI literature. AI & Society, https://doi.org/10.1007/s00146-021-01377-9
- Ryan, R. M., & Deci, E. L. (2017). Self-determination theory: Basic psychological needs in motivation, development, and wellness. Guilford Publications.
- Ryff, C. D., & Singer, B. H. (2008). Know thyself and become what you are: A eudaimonic approach to psychological well-being. Journal of Happiness Studies, 9(1), 13–39. https://doi.org/10.1007/s10902-006-9019-0
- Saleiro, P., Kuester, B., Hinkson, L., London, J., Stevens, A., Anisfeld, A., Rodolfa, K. T., & Ghani, R. (2018). Aequitas: A bias and fairness audit toolkit. arXiv preprint arXiv:1811.05577
- Samuel, G., Lucivero, F., & Somavilla, L. (2022). The environmental sustainability of digital technologies: Stakeholder practices and perspectives. Sustainability, 14(7), 3791. https://doi.org/10.3390/su14073791
- Sarakiotis, V. (2020). Human-centered AI: Challenges and opportunities. UBIACTION
- Sawyer, B. D., Miller, D. B., Canham, M., & Karwowski, W. (2021). Human factors and ergonomics in design of A3: Automation, autonomy, and artificial intelligence. In G. Salvendy & W. Karwowski (Eds.), Handbook of human factors and ergonomics (pp. 1385–1416). Hoboken, NJ, USA: Wiley.
- Seaver, N. (2017). Algorithms as culture: Some tactics for the ethnography of algorithmic systems. Big Data & Society, 4(2), 205395171773810. https://doi.org/10.1177/2053951717738104
- Secretary of State for Digital, C., Media and Sport and Secretary of State for the Home Department by Command of Her Majesty. (2020, December 15). Online harms white paper: Full government response to the consultation. https://www.gov.uk/government/consultations/online-harms-white-paper/outcome/online-harms-white-paper-full-government-response.
- Seligman, M. E. (2012). Flourish: A visionary new understanding of happiness and well-being. Simon and Schuster.
- Shearer, E., & Mitchell, A. (2021). News use across social media platforms in 2020. Pew Research Center. https://www.pewresearch.org/journalism/2021/01/12/news-use-across-social-media-platforms-in-2020/
- Shneiderman, B, University of Maryland, College Park. (2020b). Human-centered artificial intelligence: Three fresh ideas. AIS Transactions on Human-Computer Interaction, 12(3), 109–124. https://doi.org/10.17705/1thci.00131
- Shneiderman, B. (2000). Universal usability. Ubiquity, 2000(August), 1–91. https://doi.org/10.1145/347634.350994
- Shneiderman, B. (2016). Opinion: The dangers of faulty, biased, or malicious algorithms requires independent oversight. Proceedings of the National Academy of Sciences of the United States of America, 113(48), 13538–13540. https://doi.org/10.1073/pnas.1618211113
- Shneiderman, B. (2020a). Bridging the Gap Between Ethics and Practice. ACM Transactions on Interactive Intelligent Systems, 10(4), 1–31. https://doi.org/10.1145/3419764
- Shneiderman, B. (2022). Human-Centered AI. Oxford University Press.
- Smith, A. & Director, F. (2020). Using artificial intelligence and algorithms. US Federal Trade Commission, FTC Business Blog, April, https://www.ftc.gov/news-events/blogs/business-blog/2020/04/using-artificial-intelligence-algorithms.
- Solove, D. J. (2002). Conceptualizing privacy. California Law Review, 90(4), 1087. https://doi.org/10.2307/3481326
- Sowa, K., Przegalinska, A., & Ciechanowski, L. (2021). Cobots in knowledge work: Human–AI collaboration in managerial professions. Journal of Business Research, 125, 135–142. https://doi.org/10.1016/j.jbusres.2020.11.038
- Spielkamp, M. (2017). Inspecting algorithms for bias. Technology Review, 120(4), 96–98.
- Stanton, B., & Jensen, T. (2021). Trust and artificial intelligence (Preprint). NIST Interagency/Internal Report (NISTIR) – 8332. https://www.nist.gov/publications/trust-and-artificial-intelligence
- Steffen, D. (2021). Taking the next step towards convergence of design and HCI: Theories, principles, methods [Paper presentation]. In G. Salvendy & W. Karwowski (Eds.), International Conference on Human-Computer Interaction. Hoboken, NJ, USA: Wiley.
- Stephanidis, C. (2021). Design for all in digital technologies. In G. Salvendy & W. Karwowski (Eds.), Handbook of human factors and ergonomics (pp. 1187–1215). Hoboken, NJ, USA: Wiley.
- Stephanidis, C., Antona, M., & Ntoa, S. (2021). Human factors in ambient intelligence environments. In G. Salvendy & W. Karwowski (Eds.), Handbook of human factors and ergonomics (pp. 1058–1084). Hoboken, NJ, USA: Wiley.
- Stephanidis, C., Salvendy, G., Antona, M., Chen, J. Y. C., Dong, J., Duffy, V. G., Fang, X., Fidopiastis, C., Fragomeni, G., Fu, L. P., Guo, Y., Harris, D., Ioannou, A., Jeong, K-a., Konomi, S. i., Krömker, H., Kurosu, M., Lewis, J. R., Marcus, A., … Zhou, J. (2019). Seven HCI grand challenges. International Journal of Human–Computer Interaction, 35(14), 1229–1269. https://doi.org/10.1080/10447318.2019.1619259
- Strauß, S. (2021). Deep automation bias: How to tackle a wicked problem of AI? Big Data and Cognitive Computing, 5(2), 18. https://doi.org/10.3390/bdcc5020018
- Strich, F., Mayer, A.-S., & Fiedler, M, University of Bayreuth, Germany (2021). What do I do in a world of artificial intelligence? Investigating the impact of substitutive decision-making AI systems on employees’ professional role identity. Journal of the Association for Information Systems, 22(2), 304–324. https://doi.org/10.17705/1jais.00663
- Subramonyam, H., Im, J., Seifert, C., & Adar, E. (2022, April 29–May 5, 2022). Human-AI guidelines in practice: The power of leaky abstractions in cross-disciplinary teams [Paper presentation]. CHI Conference on Human Factors in Computing Systems (CHI ’22). New Orleans, LA, USA.
- Sung, E. C., Bae, S., Han, D.-I D., & Kwon, O. (2021). Consumer engagement via interactive artificial intelligence and mixed reality. International Journal of Information Management, 60, 102382. https://doi.org/10.1016/j.ijinfomgt.2021.102382
- Szalavitz, M., Rigg, K. K., & Wakeman, S. E. (2021). Drug dependence is not addiction-and it matters. Annals of Medicine, 53(1), 1989–1992. https://doi.org/10.1080/07853890.2021.1995623
- Tahiroglu, D., & Taylor, M. (2019). Anthropomorphism, social understanding, and imaginary companions. The British Journal of Developmental Psychology, 37(2), 284–299. https://doi.org/10.1111/bjdp.12272
- Taplin, J. (2017). Move fast and break things: How Facebook, Google, and Amazon have cornered culture and what it means for all of us. Pan Macmillan.
- Taylor, M. (2020, July 14). German court bans Tesla 'Autopilot’ name for misleading customers. Forbes https://www.forbes.com/sites/michaeltaylor/2020/07/14/german-court-bans-tesla-autopilot-name-for-misleading-customers/.
- The Global Partnership on Artificial Intelligence. (2022). GPAI. https://www.gpai.ai/
- Thorson, K., Cotter, K., Medeiros, M., & Pak, C. (2021). Algorithmic inference, political interest, and exposure to news and politics on Facebook. Information, Communication & Society, 24(2), 183–200. https://doi.org/10.1080/1369118x.2019.1642934
- Tolan, S., Pesole, A., Martínez-Plumed, F., Fernández-Macías, E., Hernández-Orallo, J., & Gómez, E. (2021). Measuring the occupational impact of AI: Tasks, cognitive abilities and AI benchmarks. Journal of Artificial Intelligence Research, 71, 191–236. https://doi.org/10.1613/jair.1.12647
- Top500.org. (2020). November 2020 Top500. https://www.top500.org/lists/top500/2020/11/.
- Truog, R. D., Mitchell, C., & Daley, G. Q. (2020). The toughest triage - allocating ventilators in a pandemic. The New England Journal of Medicine, 382(21), 1973–1975. https://doi.org/10.1056/NEJMp2005689
- Uddin, G. A., Alam, K., & Gow, J. (2019). Ecological and economic growth interdependency in the Asian economies: An empirical analysis. Environmental Science and Pollution Research International, 26(13), 13159–13172. https://doi.org/10.1007/s11356-019-04791-1
- UK Research and Innovation. (2021, October 15). Responsible innovation. https://www.ukri.org/about-us/policies-standards-and-data/good-research-resource-hub/responsible-innovation/
- United Nations Department of Economic and Social Affairs. (2018, April 20). The 17 goals. https://sdgs.un.org/goals
- United Nations Educational Scientific and Cultural Organization (UNESCO). (2021). Recommendation on the ethics of artificial intelligence. https://en.unesco.org/artificial-intelligence/ethics.
- US Chamber of Commerce. (2022, January 18). U.S. chamber launches bipartisan commission on artificial intelligence to advance U.S. leadership. https://www.uschamber.com/technology/u-s-chamber-launches-bipartisan-commission-on-artificial-intelligence-to-advance-u-s-leadership.
- van Allen, P. (2018). Prototyping ways of prototyping AI. Interactions, 25(6), 46–51. https://doi.org/10.1145/3274566
- Van Ness, L. (2020, February 20). DNA databases are boon to police but menace to privacy, critics say. www.pewtrusts.org/en/research-and-analysis/blogs/stateline/2020/02/20/dna-databases-are-boon-to-police-but-menace-to-privacy-critics-say.
- van Oudheusden, M. (2014). Where are the politics in responsible innovation? European governance, technology assessments, and beyond. Journal of Responsible Innovation, 1(1), 67–86. https://doi.org/10.1080/23299460.2014.882097
- Van Raemdonck, N. (2019). The echo chamber of anti-vaccination conspiracies: Mechanisms of radicalization on Facebook and Reddit. Institute for Policy, Advocacy and Governance (IPAG) Knowledge Series, Forthcoming.
- Vinichenko, M. V., Melnichuk, A. V., & Karácsony, P. (2020). Technologies of improving the university efficiency by using artificial intelligence: Motivational aspect. Entrepreneurship and Sustainability Issues, 7(4), 2696–2714. https://doi.org/10.9770/jesi.2020.7.4(9)
- Vinuesa, R., Azizpour, H., Leite, I., Balaam, M., Dignum, V., Domisch, S., Fellander, A., Langhans, S. D., Tegmark, M., & Fuso Nerini, F. (2020). The role of artificial intelligence in achieving the sustainable development goals. Nature Communications, 11(1), 233. https://doi.org/10.1038/s41467-019-14108-y
- Visvizi, A., Lytras, M. D., Damiani, E., & Mathkour, H. (2018). Policy making for smart cities: Innovation and social inclusive economic growth for sustainability. Journal of Science and Technology Policy Management, 9(2), 126–133. https://doi.org/10.1108/jstpm-07-2018-079
- Vochozka, M., Kliestik, T., Kliestikova, J., & Sion, G. (2018). Participating in a highly automated society: How artificial intelligence disrupts the job market. Economics, Management, and Financial Markets, 13(4), 57–62. http://doi.org/10.22381/EMFM13420185
- Wachter, S., Mittelstadt, B., & Floridi, L. (2017). Transparent, explainable, and accountable AI for robotics. Sci Robot, 2(6), eaan6080. https://doi.org/10.1126/scirobotics.aan6080
- Wallach, D. P., Flohr, L. A., & Kaltenhauser, A. (2020). Beyond the buzzwords: on the perspective of AI in UX and vice versa [Paper presentation]. International Conference on Human-Computer Interaction. Virtual Event, Denmark.
- Wallach, W., & Allen, C. (2008). Moral machines: Teaching robots right from wrong. Oxford University Press.
- Wang, W., & Siau, K. (2018). Artificial intelligence: A study on governance, policies, and regulations [Paper presentation]. MWAIS 2018 Proceedings, vol. 40. St. Louis, MO, USA.
- WattTime. (2022). WattTime. https://www.watttime.org/
- WEAll. (2021). Wellbeing economy alliance. https://weall.org/
- Wellbeing AI Research Institute. (2022). Wellbeing AI Research Institute. https://wellbeingairesearchinstitute.com/.
- Wellner, P. A. (2005). Effective compliance programs and corporate criminal prosecutions. Cardozo Law Review, 27, 497.
- West, D. M. (2018). The future of work: Robots, AI, and automation. Brookings Institution Press.
- West, D. M., & Allen, J. R. (2020, July 28). Turning point. Policymaking in the era of artificial intelligence. https://www.brookings.edu/book/turning-point/.
- Wieringa, M. (2020). What to account for when accounting for algorithms: A systematic literature review on algorithmic accountability [Paper presentation]. Proceedings of the 2020 Conference on Fairness, Accountability, and Transparency. Barcelona, Spain.
- Wigger, D. (2020). Automatisiertes Fahren und strafrechtliche Verantwortlichkeit wegen Fahrlässigkeit (vol. 2). Nomos Verlag.
- Williams, B. A., Brooks, C. F., & Shmargad, Y. (2018). How algorithms discriminate based on data they lack: Challenges, solutions, and policy implications. Journal of Information Policy, 8(1), 78–115. https://doi.org/10.5325/jinfopoli.8.1.0078
- Winfield, A. F., Michael, K., Pitt, J., & Evers, V. (2019). Machine ethics: The design and governance of ethical AI and autonomous systems. Proceedings of the IEEE, 107(3), 509–517. https://doi.org/10.1109/JPROC.2019.2900622
- Wing, J. M. (2021). Trustworthy AI. Communications of the ACM, 64(10), 64–71. https://doi.org/10.1145/3448248
- Winograd, T. (2006). Shifting viewpoints: Artificial intelligence and human–computer interaction. Artificial Intelligence, 170(18), 1256–1258. https://doi.org/10.1016/j.artint.2006.10.011
- Winslow, B., Chadderdon, G. L., Dechmerowski, S. J., Jones, D. L., Kalkstein, S., Greene, J. L., & Gehrman, P. (2016). Development and clinical evaluation of an mHealth application for stress management. Frontiers in Psychiatry, 7, 130. https://doi.org/10.3389/fpsyt.2016.00130
- Winslow, B., Kwasinski, R., Hullfish, J., Ruble, M., Lynch, A., Rogers, T., Nofziger, D., Brim, W., & Woodworth, C. (2022). Automated stress detection using mobile application and wearable sensors improves symptoms of mental health disorders in military personnel. Frontiers in Digital Health, 4. https://doi.org/10.3389/fdgth.2022.919626
- Wired Magazine. (2018). AI and the future of work. https://www.wired.com/wiredinsider/2018/04/ai-future-work/
- Wirth, R., & Hipp, J. (2000). CRISP-DM: Towards a standard process model for data mining [Paper presentation]. Proceedings of the 4th International Conference on the Practical Applications of Knowledge Discovery and Data Mining. Manchester, UK.
- Wu, W., Huang, T., & Gong, K. (2020). Ethical principles and governance technology development of AI in China. Engineering, 6(3), 302–309. https://doi.org/10.1016/j.eng.2019.12.015
- Xu, W. (2019). Toward human-centered AI. Interactions, 26(4), 42–46. https://doi.org/10.1145/3328485
- Xu, W., Dainoff, M. J., Ge, L., & Gao, Z. (2022). Transitioning to human interaction with AI systems: New challenges and opportunities for HCI professionals to enable human-centered AI. International Journal of Human–Computer Interaction, 1–25. https://doi.org/10.1080/10447318.2022.2041900
- Yang, Q., Scuito, A., Zimmerman, J., Forlizzi, J., & Steinfeld, A. (2018). Investigating how experienced UX designers effectively work with machine learning [Paper presentation]. Proceedings of the 2018 Designing Interactive Systems Conference. Hong Kong, China.
- Yang, Q., Steinfeld, A., Rosé, C., & Zimmerman, J. (2020). Re-examining whether, why, and how human-AI interaction is uniquely difficult to design [Paper presentation]. Proceedings of the 2020 Chi Conference on Human Factors in Computing Systems. Virtual Event, USA.
- Yanisky-Ravid, S., & Hallisey, S. (2018). ‘Equality and privacy by design’: Ensuring artificial intelligence (AI) is properly trained & Fed: A new model of AI data transparency & certification as safe harbor procedures. SSRN Electronic Journal, https://doi.org/10.2139/ssrn.3278490
- Yao, M. Z., Rice, R. E., & Wallis, K. (2007). Predicting user concerns about online privacy. Journal of the American Society for Information Science and Technology, 58(5), 710–722. https://doi.org/10.1002/asi.20530
- Završnik, A. (2017). Big data, crime and social control. Routledge.
- Zemel, R., Wu, Y., Swersky, K., Pitassi, T., & Dwork, C. (2013). Learning fair representations [Paper presentation]. International conference on machine learning. Atlanta, GA, USA.
- Zimmer, M. J. (1995). Emerging uniform structure of disparate treatment discrimination litigation. Georgia Law Review, 30, 563.
- Zouhaier, L., Hlaoui, Y. B. D., & Ayed, L. B. (2021). A reinforcement learning based approach of context-driven adaptive user interfaces [Paper presentation]. 2021 IEEE 45th Annual Computers, Software, and Applications Conference (COMPSAC). Madrid, Spain.