Abstract
Augmented Reality (AR) could improve maritime collaboration by facilitating human factors like situation awareness and decision-making. However, it is not clear in which ways AR can be beneficial and for which maritime operations. This paper addresses the need for a systematic literature review of state-of-the-art ways AR can facilitate maritime collaboration. Following the PRISMA statement, we searched for the constructs “AR,” “maritime operation,” and “collaboration” in the Web of Science and IEEE Xplore databases. Out of 691 search results, we retained 32 publications for analysis. Ten publications from other sources were included. Our results provide a review of AR applications for the maritime operations of ship navigation, personal navigation, maritime construction, maritime maintenance and inspection, and other. We suggest that research focuses on bringing promising AR applications to higher technology readiness levels, learning from other industries, applying robust evaluation methods, and grounding more on human factors like decision-making, situation awareness, and communication.
1. Introduction
The role of human collaboration has been raised as a challenge for maritime safety (see Batalden & Sydnes, Citation2014; Chauvin et al., Citation2013; Lee et al., Citation2020; Sánchez-Beaskoetxea et al., Citation2021; Zhang et al., Citation2016). In a study of 39 maritime vessel collisions, (Chauvin et al., Citation2013) pointed out crucial causal factors such as decision-making, inter-ship communication, and Bridge Resource Management. In a study of 94 cases of maritime vessel accidents, Batalden and Sydnes (Citation2014) identified several causes related to collaboration such as shipboard management and the planning of shipboard operations. Lee et al. (Citation2020) discusses the role of collaboration for safe operations in vessel traffic systems and on offshore platforms. According to Sampson et al. (Citation2022) collaboration also influences safety in maritime evacuations.
Maritime safety becomes more relevant as the maritime industry grows (Nilsson, van Overloop, Ali Mehdi, & Pålsson, Citation2018). Further, as climate change leads to more intense and frequent extreme weather events, maritime safety should be further emphasized (United Nations Economic Commission for Europe (UNECE), Citation2020). Improved collaboration has been shown to increase energy efficiency (Waterborne Technology Platform, Citation2021). Collaboration plays a role in a wide spectrum of maritime operations, such as ship operations (Sánchez-Beaskoetxea et al., Citation2021), offshore platform operations (Lee et al., Citation2020), offshore windmill maintenance (Preisser et al., Citation2019), and ship construction (Blanco-Novoa et al., Citation2018). In this publication, we focus on maritime operations in which humans play a role; we use the definition of collaboration proposed by (Bedwell et al., Citation2012, p. 3): “An evolving process whereby two or more social entities actively and reciprocally engage in joint activities aimed at achieving at least one shared goal.” Given the importance of safe and efficient collaboration in the evolving field of maritime operations, there would be great value in identifying ways to improve this collaboration.
Various factors have been argued to be important for high-quality collaboration. For example, Warner et al. (Citation2005) emphasized team decision-making and related cognitive factors, such as shared mental models and communication. Similar factors were identified by Cooke et al. (Citation2013), namely: team planning, team decision-making, team problem-solving, team performance, shared mental models, team mental models, shared situation awareness (SSA) and team situation awareness, shared understanding, team coordination, and team communication. These collaborative factors are underpinned by individual cognitive factors such as decision-making and situation awareness (SA). Collaboration is also influenced by contextual factors, such as time constraints, risk, and task characteristics (Reader, Citation2017).
Augmented reality (AR) is a relatively new technology in the field of maritime operations, but it is seen to have the potential for facilitating collaboration (see Blanco-Novoa et al., Citation2018; Davidson & Sanderson, Citation2022; Merino et al., Citation2020; Rowen, Grabowski, Rancy, et al., Citation2019). In this publication, we use the definition of AR proposed by Azuma (Citation1997) and refined by Azuma et al. (Citation2001): “An AR system supplements the real world with virtual (computer-generated) objects that appear to coexist in the same space as the real world.” AR can be displayed on different types of hardware, such as immersive headsets (Frydenberg et al., Citation2021) and fixed displays (Oh et al., Citation2016). Often, the virtual objects are visual; in some cases, other senses are also involved, such as hearing (Lyons et al., Citation2000).
Some research has been done on AR for maritime collaboration. For example, AR can display a ship’s heading and speed (Frydenberg et al., Citation2021) or camera images on fixed displays showing traffic information (Oh et al., Citation2016). Alternatively, AR can be provided through hand-held tablets showing objects obscured by other objects (Stylianidis et al., Citation2020). While there are some studies of AR applications for maritime operations (e.g., Blanco-Novoa et al., Citation2018; Frydenberg et al., Citation2021; Rowen et al., Citation2019), their prototypes generally were developed to a low technology readiness level (TRL). TRLs are a method for estimating the maturity of a technology during its development by grading them on a scale (Héder, Citation2017, p. 11). Another reason we think AR may be useful for collaboration in maritime operations is that it is being studied and implemented for collaboration in other fields, such as product design, factory planning, and maintenance (Lukosch et al., Citation2015), and manufacturing (Marques et al., Citation2022). Similar operations can be carried out in maritime contexts. While AR has been implemented in several industries, its effects on operator performance are understudied (Grabowski et al., Citation2018), particularly in the maritime industry (De la Peña Zarzuelo et al., Citation2020; Gernez et al., Citation2020). Furthermore, there is a need to understand which categories of maritime operations may benefit from AR (Gernez et al., Citation2020). Finally, we see a need for understanding the potential of AR for collaboration (Billinghurst, Citation2021) including in maritime operations (Davidson & Sanderson, Citation2022; Merino et al., Citation2020; Pidel & Ackermann, Citation2020).
While AR may have the potential to facilitate maritime collaboration, it is not clear in which ways AR can be beneficial and for which maritime operations. A review of the existing research and development of AR for collaboration in maritime operations is needed to create an overview of the state-of-the-art ways in which AR can facilitate collaboration in maritime operations. Therefore, a systematic literature review could advance the knowledge base of ways AR can facilitate collaboration in maritime operations. Thus, we present a systematic literature review with the aim of guiding researchers and developers in understanding and developing AR for collaboration in maritime operations and other safety-critical operations. To achieve this aim, this study has three objectives: (1) creating an overview of the state-of-the-art ways in which AR can facilitate collaboration in maritime operations; (2) creating a categorization of these maritime operations; (3) giving insight into cognitive and collaborative challenges in these maritime operations.
Previous literature reviews have identified valuable knowledge on AR applications in maritime operations. For example, Gernez et al. (Citation2020) and Laera et al. (Citation2021) reviewed AR applications for ship navigation, and Vasiljević, Borović, and Vukić (Citation2011) and Von Lukas, Vahl, and Mesing (Citation2014) reviewed AR applications for several maritime operations, including ship construction. Our current review contributes to these existing reviews in several ways. Firstly, it includes the most recent developments. Secondly, compared to Gernez et al. (Citation2020) and Laera et al. (Citation2021), it includes maritime operations other than ship navigation, such as inspection and maintenance. Thirdly, compared to Vasiljević et al. (Citation2011) and Von Lukas et al. (Citation2014), it presents a more systematic approach and includes more recent developments. Lastly, it focuses on collaborative and cognitive challenges related to collaboration in maritime operations. This review is timely because AR has passed the top of the Gartner hype curve in 2010 (?). This is shown in , which depicts where AR was on the Gartner hype curve in the years 2005–2018, indicating that, around 2010, proposed and tested use cases became realistic and industry adoption of AR started (Gartner, Citationn.d.a). For examples of AR implementation for collaboration see Blanco-Novoa et al. (Citation2018); Lukosch et al. (Citation2015); Marques et al. (Citation2022).
Figure 1. Augmented reality plotted on the Gartner hype curve from 2005 until 2018. Data from (Gartner, Citationn.d.b), figure made by (Wikitude, Citationn.d.)
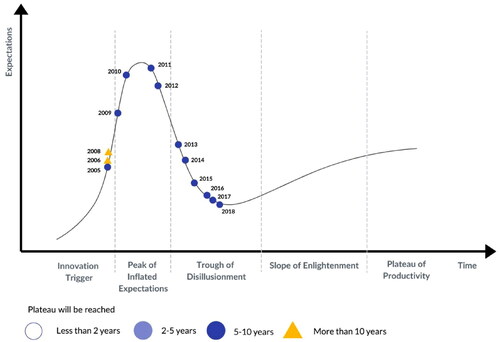
2. Method
We made a systematic literature review of scientific publications on the topic of AR applications relevant to collaboration in maritime operations. Literature reviews are vulnerable to researcher bias. Therefore, it is important to follow guidelines to reduce the impact of bias (Drucker, Fleming, & Chan, Citation2016). Following such guidelines also makes it easier to assess the trustworthiness of a literature review and allows others to replicate and update the literature review. Our review was done in accordance with the guidelines of the PRISMA statement (Page, McKenzie, et al., Citation2021). We choose the PRISMA guidelines because it is endorsed by many journals and organizations from various disciplines (Page, McKenzie, et al., Citation2021) and its focus on interventions translates well to our focus on prototypes. Other guidelines we considered were the Synthesis Without Meta-analysis (SWiM) and the Cochrane guidelines. PRISMA was preferred over other guidelines such as Synthesis Without Meta-analysis (SWiM) which is intended to examine quantitative effects of interventions (Campbell et al., Citation2020), as we were mainly interested in prototype development and evaluation studies, which often do not report quantitative effects. PRISMA was also preferred over the Cochrane guidelines, which are focused on randomized clinical trials of medical interventions, and are less comprehensive for the synthesis of methods and study characteristics (Arteaga et al., Citation2022). This chapter describes the process of selecting inclusion and exclusion criteria, the search for publications, the screening and reviewing process, the data collection process, and our attempts to avoid and mitigate biases.
2.1. Inclusion and exclusion criteria
Our aim was to identify literature related to AR, maritime operations, and collaboration. We only included publications that related to all three of these topics. The selected publications used various methods, such as empirical studies, reviews, prototype designs, and theoretical discussions. We think research on AR for collaboration in other industries may be relevant to collaboration in maritime operations. Therefore, when AR for collaboration was studied in maritime operations, a similar AR application studied in another industry could be included. As such, Stylianidis et al. (Citation2020, Citation2016) and Oskiper et al. (Citation2013, Citation2015) are included despite their lack of discussion of maritime operations because others developed similar AR applications for maritime operations (e.g., Lee et al., Citation2016; Morgere et al., Citation2014b). We minimized the bias introduced by including these publications as described in the section 2.4. Publications used for our review are restricted to those written in English. The reasons for exclusion of each publication found is given in our data sheet available on the Open Science Framework (OSF).
2.2. Search, screening, and full-text review
We followed the guidelines of the PRISMA statement for systematic reviews (Page, McKenzie, et al., Citation2021) in an approach similar to that of Laera et al. (Citation2021) and to some of our previous work (Van den Oever, Citation2019). We searched literature in the Web of Science and IEEE Xplore databases and added ten publications from other sources. We believe our review satisfactorily covers its topic with these databases and by including these ten publications from other sources. We choose these databases because the Web of Science is one of the most comprehensive academic literature databases (Chadegani et al., Citation2013) and IEEE Xplore specializes in computer science (About IEEE Xplore, Citation2023). Based on the Gartner hype curve AR passed the peak of inflated expectations in 2010, as depicted in , which depicts where AR was on the Gartner hype curve in the years 2005 to 2018, indicating that around 2010, proposed and tested use cases became realistic and industry adoption started (Gartner, Citationn.d.a). Thus, we limited our search to literature published after 2010. The last inclusion date was September 22, 2022. The search string was based on the constructs “augmented reality,” “maritime operations” and “collaboration,” and synonyms thereof. We used negative keywords to exclude search results that were researched in a medical context. For example, we excluded “blood” and “vascular” because these terms led to results about blood vessels. We searched the same search terms in both databases. To further filter out irrelevant publications, search results from subject areas that did not have any clear relevance to maritime operations involving humans were excluded, such as radiology, physics, and biology. The exact search strings, full list of filters of the respective databases, and instructions for replicating the literature search are available on OSF. Here, we present a readable version of the search string:
Title, abstract, and keywords: “augmented reality” OR AR OR HMD OR “immersive augmented reality” OR “head mounted display” OR HUD OR “head up display” OR MR OR “mixed reality” OR HWD OR “head-worn display”
AND Title, abstract, and keywords: collaboration OR cooperation OR communication OR operation OR “team communication” OR “situation awareness” OR “situational awareness” OR decision* OR decision-making OR “decision making” OR joint OR “shared situation awareness” OR “team situation awareness” OR “shared decision-making” OR “shared decision making” OR “team decision-making” OR “team decision making” OR “joint decision making” OR “joint decision-making” OR orientation
AND Title, abstract, and keywords: “Maritime operation” OR “offshore operation” OR offshore OR maritime OR ship OR “ship bridge” OR vessel OR marine OR navigation OR “Safety-critical system” OR” safety critical” OR “safety-critical”
NOT Title, abstract, and keywords: blood OR vascular OR surgery OR surgical OR medical
2.3. Data collection process
Publications included after the full-text review were structurally summarized in an Excel spreadsheet, similar to Schraagen and Verhoeven (Citation2013) and Van den Oever (Citation2019), available in our data sheet. Each publication was classified according to the following categories: publication type, study method, AR hardware type, visualized information, validation level, TRL, maritime operation type, and methodological limitations. explains the categories by showing their classification format and the rationale for them. Column one lists the categories we summarized in our data sheet; column two explains how we filled in cells for each publication for the category listed in column one, often including standardized answer options; column three explains why and how we summarized publications in the category listed in column one and why and how we applied the classification format described in column two.
Table 1. Categories of the structured summaries of individual publications.
The first author estimated the TRL of the AR applications by comparing the full-text description in the manuscript with the TRL criteria shown in row five of . To verify this assessment, the inter-rater reliability of the TRL judgments were assessed for a subset of 15 of the publications. This was done by a research assistant who separately estimated the TRLs for a random selection of the publications. A linear weighted Cohen’s Kappa showed an inter-rater reliability of 0.81, which is conventionally considered to be acceptable (McHugh, Citation2012). TRL estimations that differed between the first author and the research assistant were resolved by discussion of the criteria by which we estimated TRLs. The estimation criteria that came forth from this discussion were used for the final TRL estimations in our review.
Data from individual publications selected for review were synthesized and numerical summaries were made when relevant (see our data sheet on OSF). Distributions were summarized in tables for several data categories: reasons for exclusion in the screening and full-text review, publication types, chronological and geographical distribution of publications, maritime operations, TRLs, and hardware used. Summary statistics, such as means and modes, were calculated when possible. Chronological distribution and TRL data are summarized in graphs. Structured summaries were grouped to synthesize their characteristics per maritime operation category.
2.4. Bias avoidance and mitigation
Literature reviews carry a risk of promoting a biased view of knowledge on a topic Page, Moher, et al. (Citation2021). In the current study, we attempted to minimize this risk of bias in several ways. In terms of bias in individual publications, we evaluated and discussed elements that add to the risks of bias within each publication (i.e., research methods, and reporting).
In terms of bias across publications, the perspectives of the authors may lead to bias. The ten publications found through other sources than the database search may have introduced some bias in favor of research known to the authors. In addition, the authors’ judgments on the relevance of publications to collaboration, maritime operations, and AR may have been biased in favor of topics that are better known to the authors. Namely, Stylianidis et al. (Citation2020, Citation2016) and Oskiper et al. (Citation2013, Citation2015) are included despite their lack of discussion of maritime operations because other researchers developed similar AR applications for maritime operations (e.g., Lee et al., Citation2016; Morgere et al., Citation2014b). We attempted to minimize the risk of this bias by limiting the number of publications from other sources and by ensuring their thematic relatedness to publications found through the structured search.
Another risk of bias comes from using scientific databases as our main source, which creates a blind spot for developments that are not published in scientific literature. Some of the publications we found (e.g., Şakar & Sürücü, Citation2018) discuss non-academic sources, which implies there are more AR applications under development than is reflected in the scientific literature. Besides that, research institutes like the Maritime Research Institute Netherlands (MARIN), the German Aerospace Center (DLR), and companies such as Kongsberg develop and study maritime AR applications beyond those discussed in publicly available publications. This bias is partly resolved by including three publications from other sources which were published by DLR. Nevertheless, there may remain some bias in favor of scientific works published in scientific journals. We accept this bias because our target readers are in the scientific community. As such, readers should assume our literature review falls short concerning the representation of non-scientific and non-public developments of AR for maritime collaboration.
3. Results
We performed a systematic literature review of scientific publications on the topic of AR applications relevant for collaboration in maritime operations, following the guidelines of the PRISMA guidelines (Page, McKenzie, et al., Citation2021). The next section describes our search results and the characteristics of the publications we found. After that, we discuss AR applications for each of the maritime operation categories we delineated.
3.1. Search results and publication characteristics
We searched in the Web of Science (WOS) and IEEE XPlore (IEEEX) databases. We filtered publications following the PRISMA statement (Page, McKenzie, et al., Citation2021), as depicted in . The top row shows how many publications were identified in the databases and how many publications we excluded for being duplicates; the second row shows how many publications we screened based on their titles and abstracts; the third row shows how many publications we full-text reviewed; the bottom row shows how many publications we included in our review. In the screening and full-text review, publications were excluded for being insufficiently related to maritime operations (e.g., Mouri et al., Citation2012; Tran et al., Citation2021), to AR (e.g., Tsivgoulis et al., Citation2018), to human collaborations (e.g., Bang et al., Citation2016; Rogers et al., Citation2015), or combinations thereof. Exact numbers per reason of exclusion in the screening and full-text review are listed in . Column one gives a list of reasons for excluding publications; column two gives the number of publications excluded in the screening for each reason in column one; column three gives the number of publications excluded in the full-text review for each reason in column one; the first row gives the total of publications considered in each selection phase; the last row gives the total publications included in each selection phase;” NA” means not applicable. Aside from the structured database search, Ten additional publications from other sources than the database search were included (i.e., Falk et al., Citation2020; Laera et al., Citation2021; Şakar & Sürücü, Citation2018; Stylianidis et al., Citation2020; Von Lukas et al., Citation2014) because they were known to the authors, were found via citations in publications in the database search, or suggested by the reviewers. Thus, we retained 42 publications for analysis. displays the chronological distribution of publications, with the x-axis giving the years 2010 to 2022 and the y-axis showing the number of publications in each year as a bar with a label. We see a mild trend towards increased publication on the topic. We think the dip in publications in 2021 and 2022 may be explained by the Corona pandemic and our latest inclusion date being September 9 2022, rather than a waning of interest in the subject. Included publications come from Europe (21) North America (9), Asia (8), Oceania (3), and South America (1).
Table 2. Reason for exclusion of publications in screening and full-text review.
Examining the publications along the categories by which we summarized them gives insight into publication characteristics. Firstly, we found four different publication types. There were 26 publications that developed a prototype and evaluated it. 11 publications developed a prototype without evaluating it. One publication was a theoretical discussion. Four publications were review articles. Secondly, these 42 publications discussed 57 unique AR applications. Thirdly, researchers used different hardware for their prototypes. As shown in , in which the x-axis gives the different hardware types we identified, and the y-axis shows how many of the prototypes were made using each hardware type with a bar and a label. the most popular devices were optical see-through head-mounted displays (HMD), followed by fixed displays. Eight studies did not clearly specify the hardware they used. Fourthly, TRLs ranged from 1 to 7, with a mode of 2 as visible in , which shows on the x-axis the TRLs and on the y-axis how many of the prototypes were on each TRL according to our estimation. Structured summaries of all selected publications and calculations for the figures and tables in this paper are available in our data sheet on OSF.
3.2. AR applications for maritime operations
Our literature review showed that AR application prototypes have been developed for various maritime operations. shows how many times AR applications for the different maritime operations were discussed in the literature we found. Column one lists the maritime operation categories we made; column two gives the number of publications in each category. To see which publication was in each category, we refer to the column” Maritime operation type” in our data sheet available on OSF. In each of the following subsections, we discuss a group of maritime operations. For each operation, we will list the following: how many AR applications are relevant for this type of operation, the cognitive and collaborative challenges that the AR applications attempt to address, what kind of information is visualized in the AR applications, the hardware that was used, and the TRLs of the AR applications.
Table 3. Prevalence of maritime operations discussed in included publications.
3.2.1. AR for ship navigation
In terms of implementing AR, ship navigation is the most discussed operation. We found AR applications intended for four types of ship operation: (1) normal operations such as sailing, (2) traffic control, (3) control of remotely operated vehicles (ROV) on the surface, and (4) control of ROV underwater.
Ship navigation in normal operations using AR was the most discussed; 22 prototypes were made for this operation, as shown in . The AR application sometimes addresses more specific tasks, like navigating ships in narrow waterways (Rowen et al., Citation2021), in coastal waters (Morgere et al., Citation2014b), or while docking (Okazaki, Takaseki, et al., Citation2017). The cognitive and collaborative challenges that these operations pose include maintaining SA (Grabowski et al., Citation2018; Okazaki, Takaseki, et al., Citation2017; Rowen et al., Citation2019; Şakar & Sürücü, Citation2018), SSA (Rowen, Grabowski, Rancy, et al., Citation2019), decision-making (Grabowski et al., Citation2018; Morgere et al., Citation2014a, Citation2014b; Rowen et al., Citation2019; Wisernig et al., Citation2015), collaborative decision-making (Grabowski et al., Citation2018; Rowen, Grabowski, Rancy, et al., Citation2019), communication (Grabowski et al., Citation2018; Rowen, Grabowski, Rancy, et al., Citation2019; Satriadi et al., Citation2019), operator mobility, self-efficacy, and trust (Rowen et al., Citation2021), and mental workload (Morgere et al., Citation2014a, Citation2014b; Oh et al., Citation2016; Rowen et al., Citation2019). The AR applications visualize information such as the following: collision warnings, position, rudder information (Oh et al., Citation2016), roll and pitch, engine data (Laera et al., Citation2021), speed over ground (SOG), course over ground (COG), heading (Oh et al., Citation2016; Rowen et al., Citation2019; Rowen et al., Citation2021; Rowen, Grabowski, Rancy, et al., Citation2019), Automatic Identification System (AIS) information, bearing, closest point of approach (CPA) and time to closest point of approach (TCPA) of other ships or buoys (Leite et al., Citation2022; Oh et al., Citation2016; Rowen et al., Citation2019, Citation2021; Rowen, Grabowski, Rancy, et al., Citation2019), information about objects and areas of interest (Laera et al., Citation2021, Citation2020; Leite et al., Citation2022; Morgere et al., Citation2014a, Citation2014b; Oh et al., Citation2016; Okazaki, Takaseki, et al., Citation2017; Şakar & Sürücü, Citation2018), tide and sea current information (Laera et al., Citation2021), track lines, sea route lines, course lines (Leite et al., Citation2022; Oh et al., Citation2016; Okazaki, Takaseki, et al., Citation2017), cross track errors (Rowen et al., Citation2019, Citation2021; Rowen, Grabowski, Rancy, et al., Citation2019), maps (Satriadi et al., Citation2019), markers in geological locations (Leite et al., Citation2022; Okazaki, Takaseki, et al., Citation2017; Oskiper et al., Citation2013, Citation2015), speeds of bow and stern, distance of bow and stern to quay, wind information (Falk et al., Citation2020), and no-go zones (Laera et al., Citation2020). AR hardware used included: HMD optical see-through (e.g., Rowen et al., Citation2021), HMD video see-through (Satriadi et al., Citation2019), unspecified HMD (e.g., Şakar & Sürücü, Citation2018), handheld optical see-through in the form of binoculars (Oskiper et al., Citation2013, Citation2015), fixed display video see-through (e.g., Okazaki et al., Citation2017). The AR applications had TRLs that ranged from 1 to 7, but the majority of these applications were developed to TRL 2 (18 out of 25 prototypes).
Maritime traffic control using AR was discussed three times (Nad et al., Citation2019; Şakar & Sürücü, Citation2018; Von Lukas et al., Citation2014). All three publications focused on surveilling ships in ports. Such surveillance poses challenges such as the following: mental workload (Nad et al., Citation2019), communicating, remotely collaborating with ship operators (Şakar & Sürücü, Citation2018), and SA (Şakar & Sürücü, Citation2018; Nad et al., Citation2019). AR applications have also been proposed for remotely observing situations (Şakar & Sürücü, Citation2018), for overlaying AIS information on the world (Nad et al., Citation2019; Von Lukas et al., Citation2014), and for providing communication tools such as virtual pointers, markers, and pose indicators (Nad et al., Citation2019; Von Lukas et al., Citation2014). The hardware used were: HMD video see-through (Nad et al., Citation2019), fixed displays (Von Lukas et al., Citation2014), and HMD optical see-through (Şakar & Sürücü, Citation2018). We judged these prototypes to be at a TRL of 2 (Nad et al., Citation2019; Şakar & Sürücü, Citation2018) and 3 (Von Lukas et al., Citation2014).
We found three AR applications for navigating ROV on the water surface (Burmeister et al., Citation2020; Byeon, Grundmann, & Burmeister, Citation2021; Laera et al., Citation2021). Burmeister et al. (Citation2020) developed a prototype for remotely operating a simulated tugboat, which Byeon et al. (Citation2021) improved to remotely operate a physical model tugboat. In a literature review, Laera et al. (Citation2021) described Hong et al.’s (Citation2015) prototype for remote vessel navigation. Remote vehicle operations pose challenges of maintaining sufficient SA with reduced sensory perception and remotely collaborating with other vessels (Byeon et al., Citation2021; Laera et al., Citation2021). Visualized information in the prototype of Byeon et al. (Citation2021) included: live 360° video, an Electronic Chart Display and Information System (ECDIS), virtual representations of control elements, such as joysticks, measured forces on the tug lines, distance to other vessels, AIS information about other vessels, and a laser pointer. The AR application described by (Laera et al., Citation2021) visualized the following information: forward and rear 180° video with AIS information of other ships, a range ring, compass, and heading. The hardware used was: HMD video see-through. We judged these prototypes to be at TRL 4 (Burmeister et al., Citation2020; Laera et al., Citation2021) and TRL 5 (Byeon et al., Citation2021).
AR for underwater navigation of ROV was discussed four times (Candeloro et al., Citation2015; He et al., Citation2019; Shih et al., Citation2018; Vasilijevic et al., Citation2013). Such operations pose challenges related to: maintaining SA (Candeloro et al., Citation2015; Vasilijevic et al., Citation2013), reduced sensory perception (He et al., Citation2019; Shih et al., Citation2018), mental workload (Vasilijevic et al., Citation2013), and collaboration between ROV operators (Candeloro et al., Citation2015). Visualized information included: dehazed live video streaming (He et al., Citation2019; Shih et al., Citation2018), depth, distances to targets, heading (Candeloro et al., Citation2015), and virtual targets presented with an auditory display (Vasilijevic et al., Citation2013). The hardware types used were: HMD video see-through (Candeloro et al., Citation2015), HMD optical see-through (Shih et al., Citation2018), fixed display video see-through with headphones (Vasilijevic et al., Citation2013). The hardware used by He et al. (Citation2019) was not specified. We judged these prototypes to be at TRL 2 (Vasilijevic et al., Citation2013), TRL 3 (He et al., Citation2019; Shih et al., Citation2018), and TRL 6 (Candeloro et al., Citation2015).
3.2.2. AR for personal navigation
During maritime operations, people have to find their way around ships, windmills, and shipyards, and while diving, for example, to find ship parts or evacuation routes. To improve safety and efficiency, AR tools are under development to support personal navigation in maritime operations. We found AR applications for three types of personal navigation: normal operations such as finding ship parts in a shipyard, crisis operations such as evacuations, and diving operations such as salvaging.
We found two instances of AR for personal navigation in normal operations (Blanco-Novoa et al., Citation2018; Şakar & Sürücü, Citation2018). When people have to navigate in large and unfamiliar areas such as shipyards and ports, they encounter challenges to SA and remote collaboration (Blanco-Novoa et al., Citation2018). The prototypes aimed to help operators by means of geotags (Blanco-Novoa et al., Citation2018; Şakar & Sürücü, Citation2018), a map-like overview of the area (Blanco-Novoa et al., Citation2018), and live video of the point of view of local operators to help remote experts orientate (Blanco-Novoa et al., Citation2018). The hardware types proposed were: handheld video see-through and HMD optical see-through (Blanco-Novoa et al., Citation2018). Şakar and Sürücü (Citation2018) did not specify hardware. We judged these prototypes to be at TRL 2 (Şakar & Sürücü, Citation2018) and TRL 3 (Blanco-Novoa et al., Citation2018).
We found four AR applications for personal navigation in crisis operations (Bhattarai et al., Citation2020; Chusetthagarn et al., Citation2018; Şakar & Sürücü, Citation2018; Sampson et al., Citation2022). Bhattarai et al. (Citation2020) and Şakar and Sürücü (Citation2018) proposed AR to improve the sensory perception of emergency personnel in critical situations. For example, during a fire, people have to cope with limited visibility and high time pressure, which poses challenges to SA, decision-making, and remote collaboration. The information that was visualized in these AR applications highlighted important points of interest, such as other emergency personnel, civilians and doors (Bhattarai et al., Citation2020), and an overlay to improve vision(Şakar & Sürücü, Citation2018). It also shared sensor data with commanding officers and other emergency personnel (Bhattarai et al., Citation2020). Sampson et al. (Citation2022) developed an AR application for evacuating people from large passenger ships. Such operations pose challenges to SA, complex problem-solving, and the coordination of emergency personnel and passengers. The AR application visualized a map, directional features, a route, and information about passengers and emergency personnel in need of assistance. Chusetthagarn et al. (Citation2018) developed an AR application prototype to manage crowds during evacuations by visualizing how many people are located in particular crisis areas. This prototype is useful for supporting SSA, communication, and collaborative decision-making in evacuations of, for example, offshore structures, ports, and ships. The visualized information was a map of crisis areas and sensor data per area. The hardware used were: HMD optical see-through (Bhattarai et al., Citation2020; Chusetthagarn et al., Citation2018) and handheld video see-through (Sampson et al., Citation2022). Şakar and Sürücü (Citation2018) did not specify the hardware for the AR application they discussed. We judged these prototypes to be at TRL 2 (Bhattarai et al., Citation2020; Şakar & Sürücü, Citation2018), TRL 3 (Chusetthagarn et al., Citation2018), and TRL 6 (Sampson et al., Citation2022).
We found three AR applications for personal navigation while diving (Gallagher et al., Citation2017; Oppermann et al., Citation2013; Şakar & Sürücü, Citation2018). These applications can be used in diving operations such as search and rescue, construction, maintenance, and salvage. Such operations pose challenges to communication (Gallagher et al., Citation2017; Oppermann et al., Citation2013) and SA (Gallagher et al., Citation2017). The visualized information included images and outlines of objects (Şakar & Sürücü, Citation2018), high-resolution sonar video, sector sonar images, navigation aids, underwater night vision, text messages (Gallagher et al., Citation2017), a crosshair in the center of the display, a mission description, and virtual search targets (Oppermann et al., Citation2013). All three prototypes used HMD optical see-through. We judged these prototypes to be at TRL 3 (Şakar & Sürücü, Citation2018; Gallagher et al., Citation2017) and TRL 2 (Oppermann et al., Citation2013).
3.2.3. AR for maritime construction
AR has been proposed for use in maritime construction of, for example, ships, ports, and offshore structures. We found five AR applications for construction in the maritime industry (Lee et al., Citation2016; Şakar & Sürücü, Citation2018; Stylianidis et al., Citation2020, Citation2016; Von Lukas et al., Citation2014). Maritime construction operations pose challenges to SA, decision-making (Stylianidis et al., Citation2020, 2016), communication, collaborative decision-making, and complex problem-solving in highly parallel and time-sensitive construction processes (Von Lukas et al., Citation2014). Both Lee et al. (Citation2016) and Von Lukas et al. (Citation2014) developed TRL 2 prototypes to design piping in ships or offshore structures. Lee et al.’s (Citation2016) prototype converted pipe drawing data to 3D models and connected sensor data of the physical piping to the 3D models to be viewed on-site. Von Lukas et al.’s (Citation2014) prototype also visualized 3D models of pipes on-site, focusing on the design and placement of new pipes in the context of a bundle of previously placed pipes. Both these prototypes used handheld video see-through AR devices (tablets). Stylianidis et al. (Citation2020, Citation2016) developed a similar application using CAD models to visualize hidden objects, such as pipes and electricity cables, and their characteristics to increase SA for decision-making in maritime construction projects. The AR hardware used was handheld video see-through. We judged this prototype to be at TRL 7. Von Lukas et al. (Citation2014) developed another prototype to support collaborative decision-making in retrofitting a rescue boat and davit crane on a ship, which we judged to be at TRL 5. The prototype visualized a davit crane and rescue boat in different positions with characteristics such as size and maximum load. The hardware used for this prototype was a handheld video see-through device. Şakar and Sürücü (Citation2018) discussed a prototype for planning terminals and port expansions. Such operations pose challenges to collaboration, efficiency, and decision-making. While the used hardware was not specified, the visualized information included virtual models of objects, such as new buildings projected in their planned location within the physical port or terminal. We judged this prototype to be at TRL 2.
3.2.4. AR for maritime maintenance and inspection
AR has been proposed for use in maritime maintenance and inspection of, for example, ships, offshore windmills, and shipyards. AR applications for maintenance and inspection in maritime contexts were discussed six times (Blanco-Novoa et al., Citation2018; Hou et al., Citation2014; Von Lukas et al., Citation2014), focused on shipyards, ships, and offshore structures. AR applications like these can support maintenance personnel through remote human support and computer support. Such operations pose challenges related to: SSA, collaborative decision-making (Von Lukas et al., Citation2014), SA, remote collaboration (Blanco-Novoa et al., Citation2018), mental workload, efficiency, and complex problem-solving (Hou et al., Citation2014; Von Lukas et al., Citation2014). Visualized information included point-of-view sharing, descriptive textual information about objects under inspection, historical data about maritime maintenance and inspection (Blanco-Novoa et al., Citation2018), object locations, visual maintenance guides, diagrams and schematics, a mini-map (Hou et al., Citation2014), and on-site visualized CAD data with information about objects (Blanco-Novoa et al., Citation2018; Hou et al., Citation2014; Von Lukas et al., Citation2014). The AR hardware devices used were: handheld video see-through (Blanco-Novoa et al., Citation2018; Hou et al., Citation2014; Von Lukas et al., Citation2014) and fixed display video see-through (Hou et al., Citation2014). We judged two of Blanco-Novoa et al.’s (Citation2018) prototypes to be at TRL 1, Hou et al.’s (Citation2014) prototype to be on TRL 2. Another prototype of Blanco-Novoa et al. (Citation2018) and Von Lukas et al.’s (Citation2014) prototype we judged to be on TRL 3.
3.2.5. AR for other maritime operations
We found AR applications for the maritime operations of helicopter piloting in an offshore wind park (Ernst et al., Citation2019; Maibach, Jones, et al., Citation2022; Maibach, Walko, et al., Citation2022; Walko & Schuchardt, Citation2021), drone operation for large-scale maritime construction, such as offshore windmills and port terminals (Piumsomboon et al., Citation2018), and handling cargo in ports (Şakar & Sürücü, Citation2018).
Flying during maritime operations makes it possible to reach locations hard to reach by ship. Helicopters are used to transfer equipment and technicians to windmills in offshore wind parks (Maibach, Jones, et al., Citation2022). Due to increasing maritime activity, the number of offshore helicopter operations is growing (Walko & Schuchardt, Citation2021). For helicopter pilots, operations pose high demands on mental workload, SA, orientation, attention, collaboration with co-pilots, coordination with ship crew (Maibach, Jones, et al., Citation2022; Maibach, Walko, et al., Citation2022; Walko & Schuchardt, Citation2021), and communication with the hoist operators and persons to be hoisted (Ernst et al., Citation2019). To support pilots, Ernst et al. (Citation2019) developed an application prototype for HMD video see-through (Oculus Rift), which we judged to be at TRL 3. The visualized information included virtual flight displays, a chase-view perspective (behind and above the helicopter), an unobstructed view of the surroundings by making the cockpit see-through, and a synthetic ocean surface that gave more valuable information than the real ocean surface by identifying wave types. Walko and Schuchardt (Citation2021) developed an HMD video see-through (HoloLens) prototype for helicopter pilots that was further developed by Maibach, Jones, et al. (Citation2022). Maibach, Walko, et al. (Citation2022) continued the development on HoloLens 2. This prototype visualized information in three categories: world fixed (heading indicator, artificial horizon, highlighted obstacles), helicopter fixed (attitude indicator, drift indicator), and head fixed (airspeed, First Limit Indicator, altitude, vertical speed, flight path marker). We judged this prototype to be at TRL 4.
In large-scale maritime construction, drones can be used for remote collaboration and multi-scale collaboration. Such operations pose challenges to remote collaboration, communication, and SSA. Partly, these challenges are posed because collaborators have different visual perspectives on a structure under construction. The visualized information included augmented interpupillary distance and movement gain in the virtual presence to give the user the impression of either flying or being a giant. The hardware used was HMD video see-through (Piumsomboon et al., Citation2018). We judged this prototype to be at TRL 3.
Much of the maritime industry is directed at handling cargo and warehousing cargo. These operations pose challenges related to SA and efficiency. In addition, small increments in time spent and errors avoided can create large improvements in efficiency (Şakar & Sürücü, Citation2018). Şakar and Sürücü (Citation2018) discuss an AR application to support cargo handling by visualizing picking lists, best routes to cargo, loading instructions, and characteristics of the cargo. The hardware was head-mounted optical see-through. We judged this prototype to be at TRL 2.
4. Discussion
To advance research and development into AR for collaboration in maritime operations, we have provided an overview of recent and related scientific work. Following the PRISMA statement for structured literature reviews (Page, McKenzie, et al., Citation2021), we searched the Web of Science and IEEE Xplore databases. Through a filtering process, we selected 34 publications for in-depth analysis and comparison and added ten publications from other sources. With this review, we address a knowledge gap of an overview of the state-of-the-art knowledge base of ways AR can be used to facilitate collaboration in maritime operations.
In section 3 we have presented a structured overview of the publications we examined. Our overview showed that AR applications for collaboration in maritime operations have been studied for various maritime operations. We categorized these operations into ship navigation, personal navigation, maritime construction, maritime maintenance and inspection, and other. Our work addresses Gernez et al. (Citation2020) call for categorizing maritime operations that could be supported by AR. For each operation, we have discussed what tasks it consists of, the AR applications that have been developed for it, the cognitive and collaborative challenges the AR applications have addressed, the hardware used, and the information visualized in AR. Most of the publications we examined were based on work from Europe, North America, and Asia. Most prototypes were at TRL 2. In this section, we will discuss (1) important recurring themes across publications we analyzed, with an emphasis on what research and development should focus on in the future, (2) limitations of our study, and (3) recommendations for further research.
4.1. Recurring themes
We will now discuss some of the common themes we discovered. Recognizing and understanding these themes may help focus and structure further research and development. The themes we discuss are: TRLs of AR application prototypes were rather low; AR applications were developed more for some maritime operations than for others; certain cognitive and collaborative challenges recur often; most studies lack explicit discussion of and grounding in cognitive and collaborative challenges.
In general, the AR application prototypes that were identified had been developed and described at rather low TRLs. Most prototypes were at TRL 2 (technology concept formulated), with some being on slightly higher TRLs (see ). The prototypes described in the publications we examined may have been further developed to higher levels of TRL, but we were not able to identify such instances. That prototypes were developed to low TRLs could indicate that the field appears to be more interested in proof-of-concept than in continued development. However, we think the field would benefit from higher TRL development of AR applications that were already tested on lower TRLs with positive results, i.e., ship navigation. Therefore, we agree with Gernez et al. (Citation2020) and Laera et al. (Citation2021) who suggested that developing and testing AR applications for ship navigation at higher TRLs may accelerate the growth of our understanding of the topic. AR development could also be extended to the other categories of maritime operations we have discussed. Besides the low TRLs, the methods sections in most of the publications we reviewed were inadequate for reproduction or validation because they focused on the design and development of prototypes rather than on evaluation. Although this has been previously addressed by, among others, Martini et al. (Citation2022), we believe this shortcoming should be mentioned. We would therefore also suggest more focus on scientific standards for methods and reporting when testing prototypes, including discussing the limitations of the evaluation.
There were more AR applications developed for some maritime operations than for others. Most AR applications were developed for ship navigation operations; several other categories of maritime operations did not receive much attention (see ), even though AR could improve collaboration. We found no publications discussing AR for other maritime operations, such as maritime crane operations and aquaculture. This is an interesting finding since AR is being researched for crane operations (Sitompul & Wallmyr, Citation2019) and agriculture (de Oliveira & Correa, Citation2020). Based on our findings, it appears that AR has significant potential for ship navigation, as also mentioned by Gernez et al. (Citation2020) and Laera et al. (Citation2021). Maritime crane operations may have challenges relating, for example, to SSA, communication, and decision-making. At the same time, there are research opportunities for AR for other maritime operations, including operations not listed in our review.
We discussed several cognitive and collaborative challenges in the publications we reviewed. Cognitive challenges that were repeatedly mentioned were: SA, reduced sensory perception, decision-making, mental workload, complex problem-solving, and efficiency. SA especially was frequently named as a cognitive challenge to be addressed by AR. Recurring collaboration challenges were SSA, communication, collaborative decision-making, and remote collaboration. These cognitive and collaborative challenges could be interesting to explore in further research as well. We suggest focusing on these collaborative challenges when answering Brandao and Pinho (Citation2017) call to do more academic research on AR for collaboration.
While cognitive and collaborative challenges were discussed in most publications, many publications lacked discussion of how these challenges had been addressed by their prototypes. For example, Şakar and Sürücü (Citation2018) suggested implementing AR for traffic control to support communication and SA without discussing SSA. Other researchers discussed SA, but not decision-making (e.g., Blanco-Novoa et al., Citation2018), even though these challenges are usually interconnected (Endsley & Garland, Citation2000). This may reflect the fact that SA has become a popular concept across both academic and practical fields, almost on the verge of passing into layman’s language. At the same time, the concept of SA has also been criticized for lacking specificity and having an unclear theoretical basis (Hoffman, Citation2015). Thus, simply stating that an AR application could” enhance SA” for a given maritime operation may have limited value.
Several publications focused on cognitive and collaborative challenges without a discussion of their relationship to the relevant theory. For example, Okazaki, Takaseki, et al. (Citation2017) developed a prototype without explicitly discussing any cognitive or collaborative challenge, while others developed similar prototypes clearly aimed at addressing cognitive and collaborative challenge (e.g., Rowen et al., Citation2021). In future AR research, we suggest putting more emphasis on relating prototype development and evaluation to relevant cognition and collaboration theories. This should improve the prototype’s applied value for maritime operations, facilitate academic research, and increase human-centeredness in design. Following this advice may also help in improving scientific methods and reporting when testing prototypes, as exemplified by Rowen et al. (Citation2021). This advice is in concurrence with Aylward’s (Citation2022) suggestion for more human-centered design.
4.2. Limitations
Some limitations should be considered when interpreting our current findings. As we mentioned in section 2.4, our filtering judgment may have introduced bias in favor of publications reporting on topics better known to us. As also mentioned in section 2.4, our literature review focuses on academic developments of AR for maritime collaboration. Thus, readers should assume our literature review falls short in its representation of non-scientific, non-public developments. Another limitation to consider is that, due to resource limitations, the TRL inter-rater reliability has been checked for only 15 out of 42 publications. However, since the inter-rater reliability score was high for that sample, we may assume that the rest of the TRL judgments are also fairly reliable. This impression was supported by the way the few cases of inter-rater disagreement were resolved. A third limitation is that we searched literature in only two databases: the Web of Science and IEEE Xplore. For example, we also could have repeated the search in the SCOPUS database. However, we believe that performing the same search in the broad Web of Science database as well as the IEEE Xplore which specializes in computer science will satisfactorily cover our topic of interest. A fourth limitation is that the wording in our search terms may have favored visual AR. For example, we searched for head-mounted display and head-up display and did not explicitly search for tactile AR and audio AR. This may have resulted in the omission of AR with tactile and audio displays. However, tactile and audio displays did appear in our search results, but these prototypes were insufficiently related to maritime operations to include in our review. Only two of the publications in our review discussed non-visual displays (e.g., Burmeister et al., Citation2020; Vasilijevic et al., Citation2013). This indicates a possible research gap in audio and tactile displays for maritime AR.
4.3. Implications for further AR research
To our knowledge, our study is the first to perform a structured literature review of AR applications in maritime operations, while dealing with cognitive and collaborative challenges and categorizing AR applications according to types of maritime operations. AR promises to be a useful tool for improving collaboration and therefore safety and efficiency in maritime operations. Our findings can guide such development and evaluation of AR for collaboration in maritime operations. Firstly, like Gernez et al. (Citation2020) and Laera et al. (Citation2021), we think AR applications for maritime collaboration are ready to be developed and evaluated at higher TRLs, especially for operations for which many prototypes have been developed at lower TRLs, i.e., ship navigation. Secondly, we agree with Aylward (Citation2022) that AR development should apply a human-centered design approach to a larger extent. Thirdly, in concurrence with Brandao and Pinho (Citation2017), we have noticed a shortage of academic research on AR for collaborative challenges, to which we add a shortage of attention to cognitive challenges. Therefore, we suggest research and development of AR applications attend to cognitive and collaborative challenges. Fourthly, future studies would benefit from task analyses of the maritime operations we have discussed in order to identify further cognitive and collaborative challenges that AR can address. Fifthly, our overview of maritime operations can help researchers choose operations to research for which there is a lack of AR development. This includes operations for which we found no academic publications, such as maritime crane operations and aquaculture operations. Studying the applicability of AR in these operations may help increase safety and efficiency.
Our results and findings may also be useful for wider AR research and development. Some aspects of maritime operations have similarities to operations in other industries. For example, AR to support the handover of control from automation to human operator (Langlois & Soualmi, Citation2016) and between human operators (Sampaio et al., Citation2020); AR for maintenance and inspection (e.g., Le Chenechal et al., Citation2016; Park et al., Citation2020). AR is also used in the design and construction of other things than ships and harbors (see Lukosch et al., Citation2015; Marques et al., Citation2022). Insights may even be relevant to AR applications that appear dissimilar to the maritime AR applications we treated in this paper, as cognitive and collaborative challenges may be similar (Billinghurst, Citation2021). For example, AR may support surgeons in decision-making and collaboration (Vávra et al., Citation2017). If the cognitive and collaborative challenges are the same, similar AR features may be useful, such as highlighting areas of interest. Transferring knowledge from the maritime industry to other sectors and vice versa can stimulate innovation. This may especially be the case for safety-critical operations in which cognitive and collaborative challenges are similar.
Further research into AR for maritime operations could attend to other challenges besides cognitive and collaborative challenges. Billinghurst (Citation2021) discusses other” grand challenges for AR” such as technological challenges (e.g., developing displays and tracking), interaction challenges (e.g., natural user interfaces and multi-modality), and social and ethical challenges (e.g., acceptance and privacy). Organizational, environmental, and ergonomic challenges are also important to understand when implementing AR (Masood & Egger, Citation2019). Further research into AR for maritime operations could attend to these challenges. More foundational research into the grand challenges of implementing AR may also benefit the maritime and other sectors.
Further research can use our data sheet available on OSF to review topics we summarized in the data sheet but did not cover in this review. We chose to focus our review on identifying maritime operations in which AR can support collaboration, estimating TRLs of developed prototypes, identifying cognitive and collaborative challenges AR can address, and relating prototype development and evaluation to relevant theory. We did not extensively treat information visualization, and prototype evaluation methods, which are summarized in our data sheet. Our summary of information visualized in AR can be used to suggest design guidelines for developing AR applications for collaboration in safety-critical operations (maritime and in other industries) similar to existing design guidelines for ship bridges (Nordby et al., Citation2019). Our summary of prototype evaluation methods may be used for making an overview and assessment of prototype evaluation methods in the maritime and other industries. As mentioned in section 4.1, we think evaluation methods could be more scientific, which has been addressed by, among others, Martini et al. (Citation2022). A study reflecting on common issues in prototype evaluation methods may inform the research and development of prototypes.
4.4. Conclusion
Our systematic review found a thriving literature on AR prototypes for maritime operations. Our evaluation of the literature has led us to conclude that it would be more beneficial to spend more resources on developing the prototypes to higher TRLs, rather than developing new prototypes that overlap with previous functionality. At the same time, we conclude that it would be beneficial to develop prototypes for a wider array of maritime operations. For this, design guidelines would be useful. Further, grounding AR development in cognitive, collaborative, and other” grand challenges” (Billinghurst, Citation2021) may facilitate the transfer of AR applications to new contexts with similar challenges. De la Peña Zarzuelo et al. (Citation2020) envisioned that AR can become a pillar of the maritime industry. We believe that, if our recommendations are followed, their vision can be made true.
Acknowledgements
We wish to thank Anna Kartnes Andersson for helping with the inter-rater reliability checking of the TRLs.
Disclosure statement
No potential conflict of interest was reported by the author(s).
Data availability statement
We have made our data available in a data sheet on the Open Science Framework: https://osf.io/5eur3/?viewonly=7eadfa2f2e85446ea8c4dcb8d6f917de for evaluating the validity and reliability of our work and to facilitate follow-up research.
Additional information
Notes on contributors
Floris van den Oever
Floris van den Oever is a Ph.D. researcher in Psychology and Human-Computer Interaction at the University of Bergen, Norway. His work focuses on AR and collaboration. He holds an MSc in Human Factors and Engineering Psychology with honors in design from the University of Twente, The Netherlands.
Morten Fjeld
Morten Fjeld is a professor of Human-Computer Interaction at the University of Bergen, Norway, and Chalmers, Sweden. His work focuses on tangible user interfaces and founded the t2i Interaction Lab. He holds a dual MSc in applied mathematics from NTNU, Norway, and ENSIMAG, France, and a Ph.D. from ETH, Switzerland.
Bjørn Sætrevik
Bjørn Sætrevik is a professor of general psychology at the University of Bergen, Norway. His work focuses on decision-making, perceived risk, team-processes, and human factors. He is a clinical psychologist (2003) with a Ph.D. in cognitive neuroscience (2007), both from the University of Bergen, Norway.
References
- About Ieee Xplore (2023). IEEE Xplore Resources and Help. Retrieved February 13, 2023, from https://ieeexplore.ieee.org/Xplorehelp/overview-of-ieee-xplore/about-ieee-xplore
- Arteaga, M. C., Workentin, M., Alamgir, A. K. M., & Kupka, C. F. (2022). PRISMA statement and cochrane reviews. https://doi.org/10.13140/RG.2.2.13610.29121
- Aylward, K. (2022). Towards an understanding of the consequences of technology-driven decision support for maritime navigation [Doctoral dissertation]. Chalmers University of Technology. Retrieved 2022-09-13, from https://doi.org/10.13140/RG.2.2.32082.02243
- Azuma, R. (1997). A survey of augmented reality. Presence: Teleoperators and Virtual Environments, 6(4), 355–385. https://doi.org/10.1162/pres.1997.6.4.355
- Azuma, R., Baillot, Y., Behringer, R., Feiner, S., Julier, S., & MacIntyre, B. (2001). Recent advances in augmented reality. IEEE Computer Graphics and Applications, 21(6), 34–47. https://doi.org/10.1109/38.963459
- Bang, J., Lee, D., Lee, H., & Son, W. (2016). Network assistance to localization and mapping for outdoor augmented reality in cellular network (pp. 1–4). IEEE.
- Batalden, B.-M., & Sydnes, A. K. (2014). Maritime safety and the ISM code: A study of investigated casualties and incidents. WMU Journal of Maritime Affairs, 13(1), 3–25. https://doi.org/10.1007/s13437-013-0051-8
- Bedwell, W. L., Wildman, J. L., DiazGranados, D., Salazar, M., Kramer, W. S., & Salas, E. (2012). Collaboration at work: An integrative multilevel conceptualization. Human Resource Management Review, 22(2), 128–145. https://doi.org/10.1016/j.hrmr.2011.11.007
- Bhattarai, M., Jensen-Curtis, A. R., & Martinez-Ramon, M. (2020, December). An embedded deep learning system for augmented reality in firefighting applications. In 2020 19th IEEE International Conference on Machine Learning and Applications (ICMLA), Miami, FL (pp. 1224–1230). IEEE. https://doi.org/10.1109/ICMLA51294.2020.00193
- Billinghurst, M. (2021, March). Grand challenges for augmented reality. Frontiers in Virtual Reality, 2 (SPECIALTY GRAND CHALLENGE article), 578080. https://doi.org/10.3389/frvir.2021.578080
- Blanco-Novoa, O., Fernandez-Carames, T. M., Fraga-Lamas, P., & Vilar-Montesinos, M. A. (2018). A practical evaluation of commercial industrial augmented reality systems in an industry 4.0 shipyard. IEEE Access, 6(2018), 8201–8218. https://doi.org/10.1109/ACCESS.2018.2802699
- Brandao, W. L., & Pinho, M. S. (2017). Using augmented reality to improve dismounted operators’ situation awareness. In 2017 IEEE Virtual Reality (VR), Los Angeles, CA (pp. 297–298). IEEE. https://doi.org/10.1109/VR.2017.7892294
- Burmeister, H.-C., Grundmann, R., & Schulte, B. (2020, October). Situational awareness in AR/VR during remote maneuvering with MASS: The tug case. In Global Oceans 2020: Singapore – U.S. Gulf Coast, Biloxi, MS (pp. 1–6). IEEE. https://doi.org/10.1109/IEEECONF38699.2020.9389455
- Byeon, S., Grundmann, R., & Burmeister, H.-C. (2021). Remote-controlled tug operation via VR/AR: Results of an in-situ model test. TransNav, the International Journal on Marine Navigation and Safety of Sea Transportation, 15(4), 801–806. https://doi.org/10.12716/1001.15.04.12
- Campbell, M., McKenzie, J. E., Sowden, A., Katikireddi, S. V., Brennan, S. E., Ellis, S., Hartmann-Boyce, J., Ryan, R., Shepperd, S., Thomas, J., Welch, V., & Thomson, H. (2020, January). Synthesis without meta-analysis (SWiM) in systematic reviews: Reporting guideline. BMJ, 368(2020), l6890. https://doi.org/10.1136/bmj.l6890
- Candeloro, M., Valle, E., Miyazaki, M. R., Skjetne, R., Ludvigsen, M., & Sorensen, A. J. (2015, October). HMD as a new tool for telepresence in underwater operations and closed-loop control of ROVs. In OCEANS 2015 - MTS/IEEE Washington, Washington, DC (pp. 1–8). IEEE. https://doi.org/10.23919/OCEANS.2015.7404466
- Chadegani, A. A., Salehi, H., Yunus, M. M., Farhadi, H., Fooladi, M., Farhadi, M., Ebrahim, N. A. (2013). A comparison between two main academic literature collections: Web of science and scopus databases. https://doi.org/10.48550/ARXIV.1305.0377
- Chauvin, C., Lardjane, S., Morel, G., Clostermann, J.-P., & Langard, B. (2013, October). Human and organisational factors in maritime accidents: Analysis of collisions at sea using the HFACS. Accident; Analysis and Prevention, 59(2013), 26–37. https://doi.org/10.1016/j.aap.2013.05.006
- Chusetthagarn, D., Visoottiviseth, V., & Haga, J. (2018, November). A prototype of collaborative augment reality environment for HoloLens. In 2018 22nd International Computer Science and Engineering Conference (ICSEC), Chiang Mai, Thailand (pp. 1–5). IEEE. https://doi.org/10.1109/ICSEC.2018.8712803
- Cooke, N. J., Gorman, J. C., Myers, C. W., & Duran, J. L. (2013). Interactive team cognition. Cognitive Science, 37(2), 255–285. https://doi.org/10.1111/cogs.12009
- Davidson, T. J., & Sanderson, P. M. (2022). A review of the effects of head-worn displays on teamwork for emergency response. Ergonomics, 65(2), 188–218. https://doi.org/10.1080/00140139.2021.1968041
- De la Peña Zarzuelo, I., Freire Soeane, M. J., & López Bermúdez, B. (2020). Industry 4.0 in the port and maritime industry: A literature review. Journal of Industrial Information Integration, 20(2020), 100173. https://doi.org/10.1016/j.jii.2020.100173
- de Oliveira, M. E., & Correa, C. G. (2020, November). Virtual Reality and Augmented reality applications in agriculture: A literature review. In 2020 22nd Symposium on Virtual and Augmented Reality (SVR), Porto de Galinhas, Brazil (pp. 1–9). IEEE. https://doi.org/10.1109/SVR51698.2020.00017
- Directorate-General for Research and Innovation (2014, July). Horizon 2020 Work Programme 2014–2015 general annexes revised. https://ec.europa.eu/research/participants/data/ref/h2020/wp/2014_2015/annexes/h2020-wp1415-annex-g-trl_en.pdf
- Drucker, A. M., Fleming, P., & Chan, A.-W. (2016). Research techniques made simple: Assessing risk of bias in systematic reviews. The Journal of Investigative Dermatology, 136(11), e109–e114. https://doi.org/10.1016/j.jid.2016.08.021
- Endsley, M. R., & Garland, D. J. (2000). Situation awareness analysis and measurement. CRC Press.
- Ernst, J. M., Ebrecht, L., & Schmerwitz, S. (2019, September). Virtual cockpit instruments displayed on head-worn displays – Capabilities for future cockpit design. In 2019 IEEE/AIAA 38th Digital Avionics Systems Conference (DASC), San Diego, CA (pp. 1–10). IEEE. https://doi.org/10.1109/DASC43569.2019.9081733
- Falk, M., Saager, M., Harre, M.-C., & Feuerstack, S. (2020). Augmented berthing support for maritime pilots using a shore-based sensor infrastructure (pp. 553–559). Springer. https://doi.org/10.1007/978-3-030-60703-6_71
- Frydenberg, S., Aylward, K., Nordby, K., & Eikenes, J. O. H. (2021). Development of an augmented reality concept for icebreaker assistance and convoy operations. Journal of Marine Science and Engineering, 9(9), 996. https://doi.org/10.3390/jmse9090996
- Gallagher, D. G., Manley, R. J., Hughes, W. W., & Pilcher, A. M. (2017). Divers augmented vision display (DAVD) emerging technology development (pp. 1–7). IEEE. https://ieeexplore.ieee.org/abstract/document/8232042
- Gartner (n.d.a). Gartner hype cycle. Retrieved February 21, 2023, from https://www.gartner.com/en/research/methodologies/gartner-hype-cycle
- Gartner (n.d.b). Smarter with Gartner. Retrieved March 14, 2023, from gartner.com/SmarterWithGartner
- Gernez, E., Nordby, K., Eikenes, J. O., & Hareide, O. S. (2020). A review of augmented reality applications for ship bridges. Necesse, 5(3), 159–186. https://www.researchgate.net/publication/349968857_A_review_of_augmented_reality_applications_for_ship_bridges
- Grabowski, M., Rowen, A., & Rancy, J.-P. (2018). Evaluation of wearable immersive augmented reality technology in safety-critical systems. Safety Science, 103(2018), 23–32. https://doi.org/10.1016/j.ssci.2017.11.013
- He, H., Huang, M., Wu, D., Wang, X., Wang, H., Zhu, M., Wang, H., & Kang, J. (2019, June). An augmented reality visualization algorithm of the lightweight ROV. In OCEANS 2019 - Marseille, Marseille, France (pp. 1–9). IEEE. https://doi.org/10.1109/OCEANSE.2019.8867433
- Héder, M. (2017). From NASA to EU: The evolution of the TRL scale in Public Sector Innovation. The Innovation Journal, 22(2), 1–23. https://eprints.sztaki.hu/9204/
- Hoffman, R. (2015). Origins of situation awareness: cautionary tales from the history of concepts of attention. Journal of Cognitive Engineering and Decision Making, 9(1), 73–83. https://doi.org/10.1177/1555343414568116
- Hong, T. C., Andrew, H. S. Y., & Kenny, C. W. L. (2015). Assessing the situation awareness of operators using Maritime Augmented Reality System (MARS). Proceedings of the Human Factors and Ergonomics Society Annual Meeting, 59(1), 1722–1726. https://doi.org/10.1177/1541931215591372
- Hou, L., Wang, Y., Wang, X., Maynard, N., Cameron, I., Zhang, S., & Maynard, Y. (2014). Combining photogrammetry and augmented reality towards an integrated facility management system for the oil industry. Proceedings of the IEEE, 102(2), 204–220. https://doi.org/10.1109/JPROC.2013.2295327
- Laera, F., Fiorentino, M., Evangelista, A., Boccaccio, A., Manghisi, V. M., Gabbard, J., Gattullo, M., Uva, A. E., & Foglia, M. M. (2021). Augmented reality for maritime navigation data visualisation: A systematic review, issues and perspectives. Journal of Navigation, 74(5), 1073–1090. https://doi.org/10.1017/S0373463321000412
- Laera, F., Foglia, M. M., Evangelista, A., Boccaccio, A., Gattullo, M., Manghisi, V. M., Gabbard, J. L., Uva, A. E., & Fiorentino, M. (2020, November). Towards sailing supported by augmented reality: Motivation, methodology and perspectives. In 2020 IEEE International Symposium on Mixed and Augmented Reality Adjunct (ISMAR-Adjunct), Porto de Galinhas, Brazil (pp. 269–274). IEEE. https://doi.org/10.1109/ISMAR-Adjunct51615.2020.00076
- Langlois, S., & Soualmi, B. (2016, November). Augmented reality versus classical HUD to take over from automated driving: An aid to smooth reactions and to anticipate maneuvers. In 2016 IEEE 19th International Conference on Intelligent Transportation Systems (ITSC), Rio de Janeiro, Brazil (pp. 1571–1578). IEEE. https://doi.org/10.1109/ITSC.2016.7795767
- Le Chenechal, M., Duval, T., Gouranton, V., Royan, J., & Arnaldi, B. (2016, March). Vishnu: Virtual immersive support for HelpiNg users an interaction paradigm for collaborative remote guiding in mixed reality. In 2016 IEEE Third VR International Workshop on Collaborative Virtual Environments (3DCVE), Greenville, SC (pp. 9–12). IEEE. https://doi.org/10.1109/3DCVE.2016.7563559
- Lee, J., Yoon, W. C., & Chung, H. (2020). Formal or informal human collaboration approach to maritime safety using FRAM. Cognition, Technology & Work, 22(4), 861–875. https://doi.org/10.1007/s10111-019-00606-y
- Lee, J., Lee, K., Nam, B., & Wu, Y. (2016, September). IoT Platform-based iAR: A Prototype for Plant O&M Applications. In 2016 IEEE International Symposium on Mixed and Augmented Reality (ISMAR-Adjunct), Merida, Yucatan, Mexico (pp. 149–150). IEEE. https://doi.org/10.1109/ISMAR-Adjunct.2016.0063
- Leite, B. G., Sinohara, H. T., Maruyama, N., & Tannuri, E. A. (2022). Maritime navigational assistance by visual augmentation. Journal of Navigation, 75(1), 57–75. https://doi.org/10.1017/S0373463321000795
- Lukosch, S., Billinghurst, M., Alem, L., & Kiyokawa, K. (2015). Collaboration in Augmented Reality. Computer Supported Cooperative Work (CSCW), 24(6), 515–525. https://doi.org/10.1007/s10606-015-9239-0
- Lyons, K., Gandy, M., & Starner, T. (2000). Guided by voices: An audio augmented reality system. Springer.
- Maibach, M.-J., Jones, M., & Walko, C. (2022). Using augmented reality to reduce workload in offshore environments. CEAS Aeronautical Journal, 13(2), 559–573. https://doi.org/10.1007/s13272-022-00578-2
- Maibach, M.-J., Walko, C., Kalra, A. (2022, September). Evaluation of a head-mounted display and advanced flight control laws for helicopter ship deck landing. In Proceedings of the 48th European Rotorcraft Forum. Springer. https://elib.dlr.de/190747/
- Marques, B., Silva, S., Alves, J., Rocha, A., Dias, P., & Santos, B. S. (2022). Remote collaboration in maintenance contexts using augmented reality: Insights from a participatory process. International Journal on Interactive Design and Manufacturing (IJIDeM), 16(1), 419–438. https://doi.org/10.1007/s12008-021-00798-6
- Martini, T., Mevenkamp, P., Peinecke, N., & Schmidt, J. (2022). Investigation and evaluation of a helicopter pilot assistance system for offshore missions in degraded visual environment. In M. S. Dennison, D. M. Krum, J. J. N. Sanders-Reed, & J. T. J. Arthur (Eds.), Virtual, augmented, and mixed reality (XR) technology for multi-domain operations III (p. 7). SPIE. https://doi.org/10.1117/12.2618786
- Masood, T., & Egger, J. (2019). Augmented reality in support of Industry 4.0—Implementation challenges and success factors. Robotics and Computer-Integrated Manufacturing, 58(2019), 181–195. https://doi.org/10.1016/j.rcim.2019.02.003
- McHugh, M. L. (2012). Interrater reliability: The kappa statistic. Biochemia Medica, 22(3), 276–282. https://pubmed.ncbi.nlm.nih.gov/23092060
- Merino, L., Schwarzl, M., Kraus, M., Sedlmair, M., Schmalstieg, D., & Weiskopf, D. (2020, November). Evaluating mixed and augmented reality: A systematic literature review (2009–2019). In 2020 IEEE International Symposium on Mixed and Augmented Reality (ISMAR), Porto de Galinhas, Brazil (pp. 438–451). IEEE. https://doi.org/10.1109/ISMAR50242.2020.00069
- Morgere, J. C., Diguet, J.-P., & Laurent, J. (2014a, September). Electronic navigational chart generator for a marine mobile augmented reality system. In 2014 Oceans - St. John’s (pp. 1–9). IEEE. https://doi.org/10.1109/OCEANS.2014.7003021
- Morgere, J. C., Diguet, J. P., & Laurent, J. (2014b, August). Mobile augmented reality system for marine navigation assistance. In 12th IEEE International Conference on Embedded and Ubiquitous Computing, Milano, Italy (pp. 287–292). IEEE. https://doi.org/10.1109/EUC.2014.49
- Mouri, K., Ogata, H., Li, M., Hou, B., & Uosaki, N. (2012). Learning log navigator: Augmented awareness past learning experiences (pp. 159–162). IEEE.
- Nad, D., Miskovic, N., & Omerdic, E. (2019, June). Multi-modal supervision interface concept for marine systems. In OCEANS 2019 - Marseille, Marseille, France (pp. 1–5). IEEE. https://doi.org/10.1109/OCEANSE.2019.8867226
- Nilsson, H., van Overloop, J., Ali Mehdi, R., & Pålsson, J. (2018). Transnational maritime spatial planning in the North Sea: The shipping context. https://maritime-spatial-planning.ec.europa.eu/practices/transnational-maritime-spatial-planning-north-sea-shipping-context
- Nordby, K., Gernez, E., & Mallam, S. (2019). OpenBridge: Designing for consistency across user interfaces in multi-vendor ship bridges. Proceedings of Ergoship 2019, 60–68. http://hdl.handle.net/11250/2638416
- Oh, J., Park, S., & Kwon, O.-S. (2016). Advanced navigation aids system based on augmented reality. International Journal of e-Navigation and Maritime Economy, 5(2016), 21–31. https://doi.org/10.1016/j.enavi.2016.12.002
- Okazaki, T., Kitagawa, R., Matsubara, K., & Kashima, H. (2017, June). Development of maneuvering support system for ship docking. In 2017 Joint 17th World Congress of International Fuzzy Systems Association and 9th International Conference on Soft Computing and Intelligent Systems (IFSA-SCIS), Otsu, Japan (pp. 1–5). IEEE. https://doi.org/10.1109/IFSA-SCIS.2017.8023252
- Okazaki, T., Takaseki, R., Shoji, R., & Matsubara, K. (2017, October). Development of sea route display system by using augmented reality. In 2017 IEEE International Conference on Systems, Man, and Cybernetics (SMC), Banff, AB (pp. 3403–3408). IEEE. https://doi.org/10.1109/SMC.2017.8123156
- Oppermann, L., Blum, L., Lee, J.-Y., & Seo, J.-H. (2013, September). AREEF Multi-player Underwater Augmented Reality experience. In 2013 IEEE International Games Innovation Conference (IGIC), Vancouver, BC, Canada (pp. 199–202). IEEE. https://doi.org/10.1109/IGIC.2013.6659137
- Oskiper, T., Sizintsev, M., Branzoi, V., Samarasekera, S., & Kumar, R. (2013, October). Augmented Reality binoculars. In 2013 IEEE International Symposium on Mixed and Augmented Reality (ISMAR), Adelaide, Australia (pp. 219–228). IEEE. https://doi.org/10.1109/ISMAR.2013.6671782
- Oskiper, T., Sizintsev, M., Branzoi, V., Samarasekera, S., & Kumar, R. (2015, September). Augmented reality scout: Joint unaided-eye and telescopic-zoom system for immersive team training. In 2015 IEEE International Symposium on Mixed and Augmented Reality, Fukuoka, Japan (pp. 25–30). IEEE. https://doi.org/10.1109/ISMAR.2015.11
- Page, M. J., McKenzie, J. E., Bossuyt, P. M., Boutron, I., Hoffmann, T. C., Mulrow, C. D., Shamseer, L., Tetzlaff, J. M., Akl, E. A., Brennan, S. E., Chou, R., Glanville, J., Grimshaw, J. M., Hróbjartsson, A., Lalu, M. M., Li, T., Loder, E. W., Mayo-Wilson, E., McDonald, S., … Moher, D. (2021). The PRISMA 2020 statement: An updated guideline for reporting systematic reviews. BMJ, n71(2021), n71. https://doi.org/10.1136/bmj.n71
- Page, M. J., Moher, D., Bossuyt, P. M., Boutron, I., Hoffmann, T. C., Mulrow, C. D., Shamseer, L., Tetzlaff, J. M., Akl, E. A., Brennan, S. E., Chou, R., Glanville, J., Grimshaw, J. M., Hróbjartsson, A., Lalu, M. M., Li, T., Loder, E. W., Mayo-Wilson, E., McDonald, S., … McKenzie, J. E. (2021). PRISMA 2020 explanation and elaboration: Updated guidance and exemplars for reporting systematic reviews. BMJ, n160(2021), n160. https://doi.org/10.1136/bmj.n160
- Park, K.-B., Kim, M., Choi, S. H., & Lee, J. Y. (2020). Deep learning-based smart task assistance in wearable augmented reality. Robotics and Computer-Integrated Manufacturing, 63(2020), 101887. https://doi.org/10.1016/j.rcim.2019.101887
- Pidel, C., & Ackermann, P. (2020). Collaboration in virtual and augmented reality: A systematic overview. In L. T. De Paolis & P. Bourdot (Eds.), Augmented reality, virtual reality, and computer graphics (Vol. 12242, pp. 141–156). Springer International Publishing. https://doi.org/10.1007/978-3-030-58465-8_10
- Piumsomboon, T., Lee, G. A., Ens, B., Thomas, B. H., & Billinghurst, M. (2018). Superman vs giant: A study on spatial perception for a multi-scale mixed reality flying telepresence interface. IEEE Transactions on Visualization and Computer Graphics, 24(11), 2974–2982. https://doi.org/10.1109/TVCG.2018.2868594
- Preisser, A. M., McDonough, R. V., & Harth, V. (2019). The physical performance of workers on offshore wind energy platforms: Is pre-employment fitness testing necessary and fair? International Archives of Occupational and Environmental Health, 92(4), 513–522. https://doi.org/10.1007/s00420-018-1385-5
- Reader, T. W. (2017). Team decision making. In E. Salas, R. Rico, & J. Passmore (Eds.), The Wiley Blackwell handbook of the psychology of team working and collaborative processes (1st ed., pp. 271–296). Wiley. https://doi.org/10.1002/9781118909997.ch12
- Rogers, K., Frommel, J., Breier, L., Celik, S., Kramer, H., Kreidel, S., Brich, J., Riemer, V., & Schrader, C. (2015). Mobile augmented reality as an orientation aid: A scavenger hunt prototype (pp. 172–175). IEEE.
- Rowen, A., Grabowski, M., & Rancy, J.-P. (2019). Through the looking glass(es): Impacts of wearable augmented reality displays on operators in a safety-critical system. IEEE Transactions on Human-Machine Systems, 49(6), 652–660. https://doi.org/10.1109/THMS.2019.2944384
- Rowen, A., Grabowski, M., & Rancy, J.-P. (2021). Moving and improving in safety-critical systems: Impacts of head-mounted displays on operator mobility, performance, and situation awareness. International Journal of Human-Computer Studies, 150(2021), 102606. https://doi.org/10.1016/j.ijhcs.2021.102606
- Rowen, A., Grabowski, M., Rancy, J.-P., & Crane, A. (2019). Impacts of wearable augmented reality displays on operator performance, situation awareness, and communication in safety-critical systems. Applied Ergonomics, 80(2019), 17–27. https://doi.org/10.1016/j.apergo.2019.04.013
- Şakar, G. D., & Sürücü, E. (2018). Augmented reality as blue ocean strategy in port industry. Dokuz Eylül Üniversitesi Denizcilik Fakültesi Dergisi, 10(1), 127–153. https://doi.org/10.18613/deudfd.428196
- Sampaio, R. S., Jones, M., & Walko, C. (2020). Evaluation of novel concepts for takeover control using electronically coupled sidesticks. Journal of the American Helicopter Society, 65(1), 1–15. https://doi.org/10.4050/JAHS.65.012004
- Sampson, O., Bolierakis, S., Krommyda, M., Karagianidis, L., & Amditis, A. (2022, June). AR Crew Rescue Assistant and AR Passenger Assistant Application for emergency scenarios on large passenger ships. In 2022 IEEE International Conference on Imaging Systems and Techniques (IST), Kaohsiung, Taiwan (pp. 1–6). IEEE. https://doi.org/10.1109/IST55454.2022.9827754
- Sánchez-Beaskoetxea, J., Basterretxea-Iribar, I., Sotés, I., & Machado, M. D. L. M. M. (2021). Human error in marine accidents: Is the crew normally to blame? Maritime Transport Research, 2(2021), 100016. https://doi.org/10.1016/j.martra.2021.100016
- Satriadi, K. A., Ens, B., Cordeil, M., Jenny, B., Czauderna, T., & Willett, W. (2019, March). Augmented reality map navigation with freehand gestures. In 2019 IEEE Conference on Virtual Reality and 3D User Interfaces (VR), Osaka, Japan (pp. 593–603). IEEE. https://doi.org/10.1109/VR.2019.8798340
- Schraagen, J. M., & Verhoeven, F. (2013). Methods for studying medical device technology and practitioner cognition: The case of user-interface issues with infusion pumps. Journal of Biomedical Informatics, 46(1), 181–195. https://linkinghub.elsevier.com/retrieve/pii/S1532046412001591https://doi.org/10.1016/j.jbi.2012.10.005
- Shih, K.-T., Lin, K.-E., & Chen, H. H. (2018, July). Dehazing with a see-through near-eye display. In 2018 IEEE International Conference on Multimedia & Expo Workshops (ICMEW), San Diego, CA (pp. 1–1). IEEE. https://doi.org/10.1109/ICMEW.2018.8551537
- Sitompul, T. A., & Wallmyr, M. (2019, November). Using augmented reality to improve productivity and safety for heavy machinery operators: State of the art. In The 17th International Conference on Virtual-Reality Continuum and Its Applications in Industry, Brisbane, QLD, Australia (pp. 1–9). ACM. https://doi.org/10.1145/3359997.3365689
- Stylianidis, E., Valari, E., Pagani, A., Carrillo, I., Kounoudes, A., Michail, K., & Smagas, K. (2020). Augmented reality geovisualisation for underground utilities. PFG – Journal of Photogrammetry, Remote Sensing and Geoinformation Science, 88(2020), 173–185. https://doi.org/10.1007/s41064-020-00108-x
- Stylianidis, E., Valari, E., Smagas, K., Pagani, A., Henriques, J., Garca, A., Jimeno, E., Carrillo, I., Patias, P., Georgiadis, Ch., Kounoudes, A., & Michail, K. (2016, October). LARA: A location-based and augmented reality assistive system for underground utilities’ networks through GNSS. In 2016 22nd International Conference on Virtual System & Multimedia (VSMM), Kuala Lumpur, Malaysia (pp. 1–9). IEEE. https://doi.org/10.1109/VSMM.2016.7863170
- Tran, T-a., Ruppert, T., Eigner, G., & Abonyi, J. (2021). Real-time locating system and digital twin in lean 4.0. (pp. 000369–000374). IEEE.
- Tsivgoulis, G. I., Gkonis, P. K., Kaklamani, D. I., Venieris, I. S., & Telonis, A. G. (2018). Tanker vessel inner spaces wlan development and quality of experience study for video streaming (pp. 1–4). IEEE.
- United Nations Economic Commission for Europe (UNECE) (2020). Climate change impacts and adaptation for transport networks and nodes. https://unece.org/sites/default/files/2021-01/ECE-TRANS-283e_web.pdf
- Van den Oever, F. (2019). Changes in team communication patterns in critical situations: using REM to study the difference in communication patterns between critical and non-critical situations [Master’s thesis]. University of Twente. https://essay.utwente.nl/79340/
- Vasilijevic, A., Miskovic, N., & Vukic, Z. (2013, June). Comparative assessment of human machine interfaces for ROV guidance with different levels of secondary visual workload. In 21st Mediterranean Conference on Control and Automation, Platanias, Chania - Crete, Greece (pp. 1292–1297). IEEE. https://doi.org/10.1109/MED.2013.6608886
- Vasiljević, A., Borović, B., & Vukić, Z. (2011). Augmented reality in marine applications. SHIPBUILDING An International Journal of Research and Development, 62(2), 136–142. https://hrcak.srce.hr/71509
- Vávra, P., Roman, J., Zonča, P., Ihnát, P., Němec, M., Kumar, J., Habib, N., & El-Gendi, A. (2017). Recent development of augmented reality in surgery: A review. Journal of Healthcare Engineering, 2017(2017), 4574172. https://doi.org/10.1155/2017/4574172
- Von Lukas, U., Vahl, M., & Mesing, B. (2014). Maritime applications of augmented reality–experiences and challenges. In International conference on virtual, augmented and mixed reality (pp. 465–475). Springer.
- Walko, C., & Schuchardt, B. (2021). Increasing helicopter flight safety in maritime operations with a head-mounted display. CEAS Aeronautical Journal, 12(1), 29–41. https://doi.org/10.1007/s13272-020-00474-7
- Warner, N., Letsky, M., & Cowen, M. (2005). Cognitive model of team collaboration: Macro-cognitive focus. Proceedings of the Human Factors and Ergonomics Society Annual Meeting, 49(3), 269–273. https://doi.org/10.1177/154193120504900312
- Waterborne Technology Platform. (2021, January). Zero-emission waterborne transport. https://ec.europa.eu/info/sites/default/files/research_and_innovation/funding/documents/european_partnership_for_zero-emission_waterborne_transport.pdf
- Wikitude (n.d). Wikitude. Retrieved March 14, 2023, from https://www.wikitude.com/
- Wisernig, E., Sadhu, T., Zilinski, C., Wyvill, B., Albu, A. B., & Hoeberechts, M. (2015, October). Augmented Reality Visualization for Sailboats (ARVS). In 2015 International Conference on Cyberworlds (CW), Visby, Sweden (pp. 61–68). IEEE. https://doi.org/10.1109/CW.2015.74
- Zhang, W., Goerlandt, F., Kujala, P., & Wang, Y. (2016). An advanced method for detecting possible near miss ship collisions from AIS data. Ocean Engineering, 124(2016), 141–156. https://doi.org/10.1016/j.oceaneng.2016.07.059