Abstract
The development of AI to function as communicators (i.e., conversational agents), has opened the opportunity to rethink AI’s place within people’s social worlds, and the process of sense-making between humans and machines, especially for people with autism who may stand to benefit from such interactions. The current study aims to explore the interactions of six autistic and six non-autistic adults with a conversational virtual human (CVH/conversational agent/chatbot) over 1–4 weeks. Using semi-structured interviews, conversational chatlogs and post-study online questionnaires, we present findings related to human-chatbot interaction, chatbot humanization/dehumanization and chatbot’s autistic/non-autistic traits through thematic analysis. Findings suggest that although autistic users are willing to converse with the chatbot, there are no indications of relationship development with the chatbot. Our analysis also highlighted autistic users’ expectations of empathy from the chatbot. In the case of the non-autistic users, they tried to stretch the conversational agent’s abilities by continuously testing the AI conversational/cognitive skills. Moreover, non-autistic users were content with Kuki’s basic conversational skills, while on the contrary, autistic participants expected more in-depth conversations, as they trusted Kuki more. The findings offer insights to a new human-chatbot interaction model specifically for users with autism with a view to supporting them via companionship and social connectedness.
1. Introduction
For many decades, the study of AI and the study of communication have progressed on different trajectories – AI research focused on reproducing aspects of human intelligence, while communication was conceptualized foremost as an exclusively human process in which technology acts only as a mediator, rather than a communicator, in order to achieve social connectedness. Today, these trajectories are converging due to the development of highly advanced AI designed to simulate, thus stepping into the role that has been historically restricted to humans. This opens new opportunities to rethink AI’s place within people’s social life, and the process of sense-making between humans and machines (Guzman, Citation2018).
Advances in AI, especially in the form of CVHs, conversational agents or social chatbots, are set to transform the interaction between humans and machines. CVHs or social chatbots are agents which use text or voice to interact with users, attempting to simulate to a large extent human-human interactions. As chatbot AI is becoming more sophisticated with increasingly human-like characteristics, many are now designed to act as social companions (such as Kuki (Pandorabots-kuki, Citation2005), XiaoIce (Zhou et al., Citation2020) and Replika (Replika, Citation2017). Furthermore, because these artificial social beings are highly customizable, emerging research has looked into how they can support people who are lonely or socially excluded (De Gennaro et al., Citation2019), older people (Valtolina & Marchionna, Citation2021) people with social anxiety (Ali et al., Citation2020), (Zhong et al., Citation2020), and to provide emotional support to people with (Roniotis & Tsiknakis, Citation2017) or other emotional/psychological disorders (Zhou et al., Citation2020).
Perhaps unsurprisingly, a specific application area of such technologies, which have increasingly attracted the attention of researchers in HCI, is autism, a condition characterized by difficulties with social interaction and communication. For instance, research has investigated how these conversational virtual agents can help improve social skills for autistic people (Abd-Alrazaq et al., Citation2020). Chatbots such as “LISSA” (Ali et al., Citation2020) and “VR-JIT” (Smith et al., Citation2014) have been used to train people with autism to improve communication skills in job interviews with a virtual character, in which participants who attended laboratory-based training sessions found VR-JIT easy to use and enjoyable, and they felt more prepared for future interviews (Abd-Alrazaq et al., Citation2020).
Most studies on chatbots and autism, however, tend to focus on training specific social or life skills, in which the conversational agent takes on the role of a trainer. The analysis of such studies often emphasizes the (in)effectiveness of the chatbot in developing a skill which can be generalized in the real world. Few studies (Bradford et al., Citation2020), (Croes & Antheunis, Citation2021) have explored how autistic people engage with the chatbot as a social companion, over a course of a longer period, to understand how they interact and connect with the chatbot in their natural environment. In addition, current studies mostly focused on children, often overlooking the autistic adult/young adult population, who arguably need more support due to their social environment being more complex to navigate (Sosnowy et al., Citation2019).
In-depth knowledge of how autistic people perceive CVHs and the role chatbots play in their social life, is under-developed. The current paper therefore attempts to address this gap by analyzing chatlogs of both autistic and non-autistic adults chatting with a social chatbot for up to four weeks, in-depth follow-on interviews and post-study questionnaires; hence the findings of this study are not only based on exploratory users’ perceptions, but are supported by authentic chatlog quotes and quantitative data from questionnaires. Specifically, our research questions are as follow:
How do autistic adults interact with the conversational virtual human (CVH), in the context of digital companionship and social connectedness?
How are the interaction patterns of autistic adults with the CVH different from the interaction patterns of non-autistic adults with the CVH?
How do autistic and non-autistic adults perceive the social interaction (i.e., trust, friendship, emotional response) with the CVH, and how was it useful in leading to social connectedness with the CVH, and possibly generalization of social connectedness in real world human-human interaction (HHI)?
We believe the paper presents a unique contribution in the HCI studies of CVHs/chatbots, first by addressing the affordances and limitations of real-world deployment of conversational agents for autistic people, in comparison to non-autistic users, and second by exploring an innovative framework for human-chatbot interactions through the lens of autism, which, we shall see later, does not necessarily draw upon conventional human-human interaction models.
2. Related work
Conversational virtual agents/humans (CVAs, CVHs, or colloquially chatbots) have been explored and studied rather extensively in the healthcare domain in the past few decades. The applications range from booking of general medical appointments to personal healthcare assistants providing simple support such as daily medication, as well as counseling, training and fully-fledged psychological therapy (e.g., Cognitive Behavioral Therapy-CBT) (Callejas & Griol, Citation2021) (Lucas et al., Citation2017)). CVAs are especially useful for people living in areas with no/limited access to specialists, or people who live in isolation due to personal/health circumstances (e.g., older people living alone).
There is now a plethora of research looking into how users interact with chatbots, including research in the context of healthcare, to what extent users trust chatbots and are able to develop positive relationships with such virtual agents. For instance, studies (Ahmad et al., Citation2009), show that patients feel more comfortable talking to chatbots compared to humans when it comes to sharing confidential information, talking about socially stigmatized topics, such as sexually transmitted infections, depression or alcoholism. This is especially true for younger people, who tend to prefer online interactions to face-to-face ones, and text messaging (e.g., messaging service of the suicide-prevention Charity Samaritans) to phone calls (Kretzschmar et al., Citation2019). Other studies, however, painted a more negative picture, showing that people felt disturbed (Inkster et al., Citation2018), or were put off by the shallowness of the conversations (Ly et al., Citation2017) or did not trust the chatbot (Mou & Xu, Citation2017).
Generally, the use of CVAs in healthcare falls under three broad categories: (i) diagnosis and symptom detection, (ii) training, (iii) therapy and intervention. Research in symptom detection and diagnosis using chatbots is mainly related to psychological conditions, such as the detection of suicidal ideation and self-harm. There is emerging evidence in machine learning research (Gratch et al., Citation2014) demonstrating that by analyzing the chatlogs, it is possible to automatically detect depression (Philip et al., Citation2017), post-traumatic stress and suicidal ideation (Carpenter et al., Citation2012). Furthermore, HCI studies have shown the success of deploying such machine learning models in real world contexts with high efficacy and positive user perceptions (Radziwill & Benton, Citation2017).
In the use of chatbots for training, some research has explored the use of chatbots to train social workers to assess youth suicide risks, where the user converses with the chatbot in the main suicide risk assessment categories: rapport, ideation, capability, plans, stressors, connections and repair (Carpenter et al., Citation2012). The training of healthcare students’ interviewing skills (Carnell et al., Citation2015) and empathy skills (Halan et al., Citation2015) have also been explored using virtual patients. Perhaps the most explored application in healthcare is the use of CVAs as assistants in therapy/intervention sessions. Research has investigated how chatbots can support mood management to help combat loneliness among older people (Gudala et al., Citation2022), to manage depressive symptoms in young adults by focusing on sleep hygiene, physical activity and nutrition (Pinto et al., Citation2013), and for stress management for college students (Gabrielli et al., Citation2021).
2.1. Autism and chatbot studies
There has been growing research into the use of CVAs to support autistic people, specifically younger children and adolescents (mostly aged 4–15), to help develop their conversational skills and “appropriate” social behaviors, as well as to improve their emotion recognition ability (Ali et al., Citation2020), (Catania, Di Nardo, et al., Citation2019), (Ma et al., Citation2019). Some research in this area (Bernardini et al., Citation2014)showed that children generally attributed positive feedback to the virtual agents, which were often met with excitement. The integration of chatbots in serious games for training demonstrated a significant increase in the proportion of social responses made by autistic children to human trainers (Porayska-Pomsta et al., Citation2018). In addition, interaction with virtual agents designed to be used as educational tools (Milne et al., Citation2009) enhanced higher conversational skills.
The rapid advances of computer graphics have also allowed researchers in chatbots and autism to investigate the use of embodied conversational agents, computer-generated characters that demonstrate many characteristics as humans in face-to-face conversation, including the ability to produce and respond to nonverbal communication, such as facial displays, hand gestures, body stance, etc. (Provoost et al., Citation2017). These embodied conversational agents have been used for training social skills (Tanaka et al., Citation2017) for autistic people. Moreover, there has been research into such virtual humans in Augmented Reality (Hartholt et al., Citation2019), offering young autistic adults the opportunity to practice social skills as well as job interview scenarios. In recent years, physically embodied conversational agents (i.e., social robots) have also been examined to support autistic people for rehabilitation, education and therapy, among the most popular ones being KASPAR (Davis, Citation2018), ZENO (Salvador et al., Citation2015) and NAO (Lahiri et al., Citation2015), because of their socially interactive capabilities (i.e., exhibiting “human social” characteristics such as expression and/or perception of emotions, communication with high-level dialogue), using natural cues such as gaze and gestures, and exhibiting distinctive personality and character). More specifically, studies found that humanoid robots can foster social (RoDiCa, (Ranatunga et al., Citation2012)) and behavioral skills in autistic children (Stanton & Stevens, Citation2017), (Thellman & Ziemke, Citation2017), improve communication skills (BLISS, (Santiesteban et al., Citation2021)) and joint attention (Charron et al., Citation2017; Taheri et al., Citation2018).
In summary, it appears that various types of CVAs (purely text/speech-based, those with embodiment including a physical form) can play an important role in supporting autistic people. They provide a safe, non-judgmental environment to practice spontaneous conversations (Cooper & Ireland, Citation2018), even for chatbots devoid of any forms of embodiment (i.e., facial expressions, and body language cues) (Bakhai et al., Citation2020), (Safi et al., Citation2021), such as Alexa or Siri, two of the most well-known speech-only chatbots. However, most studies on chatbot and autistic people so far are based on controlled experiments, where users only interacted with the system for a few short sessions, often in lab-based environment. The research protocol design also tends to focus on a handful of specific aspects of the chatbot (such as facial gestures and body language communication), with the aim to demonstrate the efficacy of chatbots in improving specific social/life skills such as eye-gazing, attention, rather than general socialization and companionship. To gain insights into how autistic people interact with the chatbot over a longer period of time, and how they perceive the development of their relationships with the chatbot in real life, we need to adopt a more holistic approach. Furthermore, most studies in this area focus on children or young adults (Fukui et al., Citation2018), leaving the adult groups (especially the lately diagnosed adults) severely under-researched. Adult life in autism (Benevides et al., Citation2020) has generally been an under-researched field. Adult autism is an important area of research as the social skills required across the lifespan can have an impact on autistic adults’ mental health and well-being, unless addressed. Different and more complex social skills should be mastered through each life stage, which makes the social demands more pressurizing among autistic adults.
3. Method
In this study, twelve participants (6 autistic and 6 non-autistic) chatted with a chatbot called Kuki (see 3.1 for details) for a 1–4 week span with a mean daily interaction duration of 13.2 minutes (Range = 5–15 minutes). They also consented to participate in a semi-structured online interview, conducted via Zoom, audio-recorded after obtaining their permission to use them only for research purposes. The interviews were transcribed for thematic analysis using NVivo. All participants also filled in questionnaires following the end of the interaction with Kuki. The chat with Kuki was open-ended, where participants were not given specific instructions or directions, instead they were asked to interact with Kuki in any way they wanted. Interview questions sought to elicit participants’ perception of their experience with Kuki, the perceived benefits/limitations, feedback on their social interaction and perception of social connectedness with the chatbot, any interesting conversations and/or experiences, and areas for improvement. The semi-structured interviews lasted 45 minutes on average (Range: 35–50 minutes). Some of the questions in the interviews are as follow:
While chatting with Kuki, did you feel like talking to a human? Why yes/no? Can you mention any similarities/differences between the way Kuki communicates and the way your friends/peers communicate with you?
Do you think chatting with Kuki helped you learn to socialize with other people? If so, how? Please, give me examples.
Has Kuki shown acceptance/empathy/understanding towards a problem of yours? What did Kuki say that made you feel better? Were there times where Kuki responded inappropriately? If so, how? And how did this make you feel?
Can you think of an instance where you shared something personal/emotional with Kuki? Can you tell me what it was? If not, were you comfortable sharing it with Kuki? Why? Is there anything you would share with Kuki, but would never share with anyone close to you? Why so?
How do you think Kuki can improve to become a better companion for you (bear in mind all aspects-visual representation, conversational skills, empathy, other skills or traits)?
Furthermore, in-depth analysis of the rich conversational chatlogs (a total of 16,132 utterances, 81.3 hours including the messages from both the chatbot and the participants) was carried out thematically using NVivo.
Finally, participants were asked to fill in three (3) post-study online questionnaires: the User experience questionnaire (Laugwitz et al., Citation2008), the Trust questionnaire (Jian et al., Citation2000) and the Human-Virtual Human Interaction Evaluation Scale (HVHIES)-adapted from HRIES Scale (Spatola et al., Citation2021), which were used to inform/assess participants’ perception of the chatbot as technology.
3.1. Kuki
The social chatbot Kuki (formerly known as Mitsuku) (Pandorabots-kuki, Citation2005) was chosen. Kuki takes the persona of an 18-year-old female from Leeds, England (Park et al., Citation2018), and has won the first place in the Loebner Prize contest five times (2013, 2016, 2017, 2018, 2019); the specific contest is related to passing the Turing Test. In this study, we chose the Kuki version deployed in Facebook messenger (see for a sample of Kuki’s conversation with a user). Kuki has been crafted in the last 20 years using an artificial intelligence mark-up language architecture (AIML, (Wallace, Citation2003)), which makes the chatbot understand language only literally and conversing more bluntly, a trait which allies with autistic people’s conversational make-up (Happé, Citation1995).
Figure 1. This is an extract from a conversation between a real user and Kuki (image taken from https://edition.cnn.com/2020/08/19/world/chatbot-social-anxiety-spc-intl/index.html).
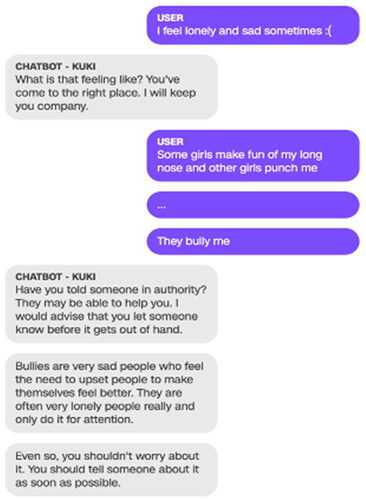
Kuki and her self-promoting message is promising to be a 24/7companion:
“Hi, I’m Kuki! You need never feel lonely again! Kuki is your new virtual friend and is here 24 hours a day just to talk to you”/She learns by experience, so the more people talk to her, the smarter she becomes (Jain et al., Citation2018).
3.2. Participant details
The 12 participants had never used a chatbot before. They were recruited through autism self-advocacy networks/communities, colleges/universities, Facebook groups related to autism support and subreddits on autism, Asperger’s, mental health support and chatbot communities. The participants consisted of 5 females, 6 males (one participant did not wish to reveal their sex), were from countries such as USA, UK, and the rest of Europe. Their age ranged from 18 to 50 years, with most participants (5) falling into the 31–40 years age group ( for full details).
Table 1. Demographics of 12 participants.
Since, an exploratory qualitative study aims to gain a deeper understanding of a phenomenon, especially when little is known about the topic, the emphasis is on exploring participants’ experiences, perceptions, and feelings; hence given the small sample size of 12 participants, the context of this study will be analyzed in a deeply personal and detailed manner. While the small sample size might limit generalizability, the richness of individual narratives offers invaluable insights into the complex interplay of age, region, culture, and attitudes towards conversational AI. Such a study emphasizes understanding over quantification, capturing the human stories behind the data.
In this qualitative research, our primary focus is on understanding the depth, complexity, and contextualized meaning of human experiences rather than quantifying them. We are interested in the participants’ perceptions, and the emphasis is on capturing the richness of individual experiences, thoughts, and feelings. Here’s why the interaction between age and nationality might be deemed irrelevant in such a study. Factors like age and nationality, which might be crucial for generalizability in quantitative studies, become secondary in qualitative research that emphasizes individual perceptions. The findings of this study are meant to provide insights into a specific group’s experiences, not to be extrapolated to larger populations. Moreover the objective of this research guide the relevance of certain variables i.e., our main aim is to understand participants’ perceptions without the influence of age and nationality (culture), making these factors extraneous.
In the interest of providing a context to the analysis and hence the descriptive findings (Section 4), summarizes the participant characteristics. The autistic group consisted of six (6) functional autistic adults with a self-reported autism diagnosis of autism (with one autistic participant reported having also a learning disorder). No other comorbidities (i.e., mental health problems such as anxiety or depression co-occurred). Specific personality traits such as tech enthusiasm and irritability to autism-related offensive behavior were additional determinants of autistic users’ perception of Kuki. These traits were evident in the interviews of the autistic participants, as they derived from the professions of some participants, as well as self-report of irritability and frustration by some participants. The non-autistic group consisted of six (6) non-autistic adults, some of whom had characteristics which could possibly bias their perceptions. P07 and P08 were highly tech literate (P07 was a language teacher and tech enthusiast, while P08 was a virtual reality developer and academic), a fact which shaped uniquely their perception of/approach to Kuki.
3.3. Data analysis
All online interviews were transcribed for an inductive thematic analysis, along with the full chat- logs from 12 participants. The coders (2 HCI researchers, 1 healthcare researcher, 1 chatbot engineer) coded different parts of the dataset using NVivo (NVivo for Mac, V. 1.5). Patterns in the data were coded, then refined into themes. Finally, to further refine and verify the themes, all coders critically discussed and reviewed each theme and underlying codes together. The resources of data presented in this paper are based on the interviews data, the chatlogs data and online questionnaires data to support what the participants said in the interview when necessary. Passages were quoted from the online interviews and cross checked with the chatlogs. Although the focus of the analysis is to tease out how autistic adults use and perceive chatbots, we found it useful to compare and contrast the findings from autistic users with non-autistic users, which provides a baseline context to facilitate in-depth analysis and meaningful discussions.
3.4. Ethics
The study was approved by a university Central Research Ethics Advisory Group. All participants were provided with information and consent forms prior to the online interviews and the chat-phase. Most of the participants viewed the interview and their chatbot experience as interesting (autistic: 4/6, non-autistic: 5/6), and were willing to share their feedback.
4. Results
In this section, we present the themes emerged from the participants’ reports/comments in the online interviews as well as the quotes from the conversational chatlogs regarding their general experience with the chatbot, their perceived impact of the interaction with the chatbot on their feelings/mood, the type of interaction they had with the chatbot, and their perceived relationship with the chatbot (i.e., establishment of friendship and trust); we also support our findings with data from online questionnaires in a separate section below. We place our emphasis on comparing autistic participants to non-autistic ones to better illustrate key insights from the analysis.
The results section is structured as follow: we first present some general observations and descriptions (Section 4.1) related to the interaction of autistic and non-autistic participants with the chatbot. Then, we delve into two major themes to highlight the unique perspectives of the social interaction experience and the potential development of social connectedness of the autistic (and non-autistic) users and the virtual agent. The first theme (Section 4.2) is related to how autistic users perceived Kuki as being both autistic and non-autistic, and respective findings extracted indirectly from data of non-autistic participants. The second theme underlines the humanization- dehumanization paradox, where both autistic and non-autistic users trod the thin line of treating Kuki as a human and a machine at the same time (Section 4.3). The similarities and differences in terms of perceptions of interaction, empathy and trust between the two groups are summarized in .
Figure 2. Perceptions of interaction, empathy and trust in kuki’s communication with both autistic and non-autistic users.
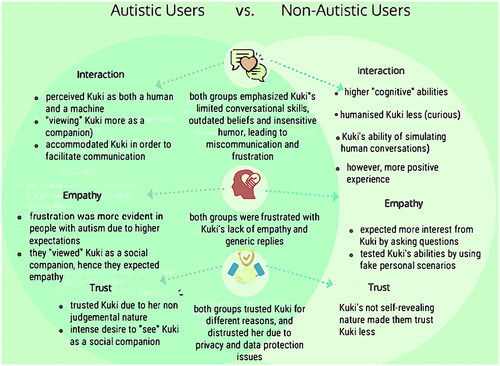
4.1. Interaction between autistic/non-autistic users and Kuki
Our general observations of the interactions between the participants and Kuki point us initially to the proverbial “social penetration theory” (Altman & Taylor, Citation1987) as described comprehensively in previous studies, in which users gradually shift from sharing superficial information in the “exploration stage,” to disclosing personal and intimate information in a later “affective stage,” allowing them to deepen their relationship (Skjuve et al., Citation2021). A non-autistic participant succinctly exemplified this through their experience:
And at the beginning, I tried to get more basic directions, superficial directions, and then as the days gone by, I tried to check, stretch more the platform, the tool to get, try to get more meaningful conversations as I would have with a friend. (Non-autistic-P09)
I didn’t feel Kuki was very, into the sort of things I was saying and the things she was saying, have absolutely no relevance to the things going on in my life. Yeah, interacting with a technological robot is not really something I’m very willing to do. (Autistic-P01)
On the other hand, some autistic participants learnt to adapt to the unique characteristics of Kuki and found a suitable social role for her. For example, some of them felt that Kuki was helpful in providing social support in that she allowed them to discuss their problems in a safe environment, free from judgement.
So it helped, because it was like […] really fun, a lot of the stuff happened to people with autism are frustrating. […] others don’t understand what’s going on, you don’t understand the body language. So I found it helpful to kind of vent, you know, it’s like, that’s something that is not judgmental, you know, and, as a point, it seemed to kind of understand me […] you know, cannot make Kuki mad, you know, cannot make it, you know, so you’re safe, comfortable. It’s a safe environment […] I felt I could trust Kuki. (Autistic-P05)
Some autistic users seem to ignore Kuki’s irrelevant replies/rude comments and continue the conversation as normal, showing either their agnostic nature to these comments or demonstrating their flexibility in accommodating Kuki’s communication quirks. What caused some autistic users to adapt successfully to Kuki’s quirks, while others responded in exasperation to the point of giving up the interaction altogether? There may be a number of reasons why many autistic users struggled to follow a smooth conversation with Kuki. We observed that some autistic users were easily put off by the generic empathetic responses from Kuki, as they felt that the characteristics which should be present in a human communication partner were missing from the chatbot. Such mismatches of their expectations that the chatbot ought to show a higher level of empathy, sensitivity and more understanding contributed negatively to their experience with Kuki. Autistic participants appeared to take offense at responses which they perceived as lacking in empathy or were insensitive. In one instance, the participant disclosed personal information about the death of the loved one and were frustrated with the perceived inability of the chatbot to comprehend the nature of their loss, something which they expected a human conversation partner to be able to do.
I already mentioned about death. But I think that’s pretty much it. I’ve only men-tioned about the fact my aunt died in January. She [Kuki] didn’t really know what to experience and she’s never been through. And that’s lucky for her. But I didn’t feel she was able to be empathetic in a way that I expected her to be. (Autistic-P01)
Misunderstandings and lack of conversational flow led most of the time to frustration. In many examples, autistic participants showed frustration over Kuki’s lack of ability to understand emotional cues, and complained that Kuki answered questions about their personal circumstances or health conditions factually instead of showing sensitivity, empathy and support (e.g., answering a question about autism by providing a Wikipedia definition, see suppl. material).
Autistic participants may have comorbidities which may result in further difficulty in interacting with a chatbot. For instance, one autistic participant in our study (P01) had a learning disability which intensified his frustration with Kuki, and discouraged him from ascribing Kuki a positive social role (Badcock & Sakellariou, Citation2022). Moreover, the processing of negative emotions is more difficult for autistic individuals and their difficulty in empathizing with the emotional experience of others is linked to sharing of emotions with negative valence, explaining why some autistic participants were extremely frustrated when they perceived Kuki as being unjustifiably rude and lacking empathy (i.e., by giving blunt definitions of “autism”).
Recent research suggests that most autistic people can in fact recognize empathetic traits in others (Bird & Viding, Citation2014). The fact that our autistic participants seemed to have higher expectations of empathy from Kuki allies with the Empathy Imbalance Hypothesis of Autism (Smith, Citation2009), people with autism lack cognitive empathy (the ability to perceive and understand the emotions of another) but have a surplus of emotional empathy (they empathize with the emotional state of others).
A few non-autistic users commented that Kuki’s conversational skills improved over time, which supports the fact that idiosyncratic participant characteristics determine the way they view Kuki, i.e., non-autistic-P10 attributes that to software update, non-autistic-P11 is not a native English speaker, and non-autistic-P07 is impressed with Kuki’s novelty as an AI (see Supplementary Material).
Another finding supports Kuki’s peaks and troughs identified by both autistic and non-autistic users in Kuki’s conversational skills and topic depth. Kuki’s “random” performance deriving from users’ positive and at the same time negative perceptions of Kuki can be seen in the following quote:
[…] When we talked about her actual programming? That was very surprising, she was able to understand she, like someone told her or programmed into exactly what type and software actually make up? Yeah, her program, she was able to talk about it quite convincingly, actually. (Non-autistic-P07).
Another factor often reported by participants to have influenced how users interacted with Kuki and if they were able to socially connect with her was related to self-disclosure. The ability to disclose personal information has often been argued to play a key role in the establishment of trust and empathy between users in a computer-mediated communication environment (Erdost, Citation2004). In online communities, self-disclosure could act as a “trigger” to elicit empathy from others. Observing participants’ self-disclosure, there are instances that autistic participants disclose personal sensitive information to Kuki. Autistic-P04 talks with Kuki about sensitive issues (bisexuality) which supports their self-disclosure. In other instances, autistic participants showed lack of trust in Kuki to discuss a personal matter:
You know nothing about my mental health and you got no right to judge that. (Chat-logs, Autistic-P01)
Interestingly for participants who were reluctant to disclose personal information, privacy concerns were cited as one of the main reasons by both autistic and non-autistic users. Several participants of both groups reported being especially concerned with their data being monitored by a third party human user, and were reluctant to disclose personal and sensitive information, a common issue among users interacting with conversational agents (Ischen et al., Citation2019), (Kretzschmar et al., Citation2019).
For some non-autistic participants, the very nature of Kuki, being a computerized virtual agent, discouraged them from self-disclosing personal information, as they considered “her” to be incapable of truly understanding and empathizing with their problems (see Supplementary Material). We will focus more on this phenomenon which we call “Botism” in Section 4.3.
In terms of the CVH’s self-disclosure, findings have been identified in conversational systems between the user and a virtual agent, with self-disclosure from the agent having a reciprocal effect on users and leading to more perceived intimacy, trust and the establishment of a meaningful relationship in the long term (Lee et al., Citation2020). However in the case of Kuki, beyond revealing that she is a chatbot, she tends to avoid divulging about herself, which acts as a barrier for the user to get to know her better, to progressively establish a kind of relationship with her.
I don’t put any feeling on it or any kind of quality. Because I really don’t know anything about Kuki. (Autistic-P05)
However, in the case of a non-autistic participant the fact that Kuki avoided disclosing information gave a sense of humanness, and was interesting (see Supplementary Material).
Trying to address the question “Can Kuki live up to the users’ expectations of a social companion” through both groups’ perceptions of Kuki as a social companion, both groups highlighted Kuki’s limitations to be a social companion in her current state. However, both sides did agree that Kuki has the potential to improve and become a better companion with specific improvements. Hence, the establishment of a possible social connection (i.e., friendship) is not feasible at the moment. Non-autistic participants seemed to have a better hope that Kuki could be a companion to a degree, while autistic participants were holding a conservative view.
It should be noted that the establishment of a relationship (i.e., friendship) seems impossible at the current state due to memory restrictions as well as lack of empathetic and conversational skills mostly noted by autistic participants.
[…] without memory it seems like she will not remember what I said. So every conversation was kind of new, she will only remember like my name, sometimes not even correctly. (Autistic-P05)
The fact that users from both groups did not succeed in viewing Kuki as a social companion and hence establishing a relationship with her, led to limited social connectedness with the chatbot, which cannot be generalized in real life interactions of autistic people.
Overall, our results indicated that while both autistic and non-autistic participants were willing to engage in social interaction with Kuki to a certain degree, we did not observe patterns that were indicative of a relationship being formed between Kuki and most participants. While all participants were interested in, and had attempted to get social and emotional support from Kuki, such needs were not fully met due to the lack of perceived empathy and understanding Kuki was capable of. This hit our autistic participants particularly hard, and hence is worthy of further investigation. This suggests that like their non-autistic counterparts, autistic participants enjoy close, empathetic, supportive, caring friendships; Kuki’s lack of empathetic responses created a dissonance between higher expectations and unmet needs of social support and social connectedness, resulting in lower overall experience with Kuki (Sosnowy et al., Citation2019). Living experiences of autistic people who have friends and feel part of a social group, and several examples of experiential evidence of autistic people managing relationships in non-autistic spaces highlight autistic people’s desire to make friends (Lawson, Citation2006), however diverging from the non-autistic understandings of autistic friendship. This also begs the question of what model of human-human interactions autistic users relied on, when communicating with Kuki? Did they perceive Kuki as an autistic being, a non-autistic being or their expectations were neither of the two (further discussions on these issues in Section 4.2)?
4.2. Autistic machine
A key approach to the study of people’s interactions with technology was proposed by Nass and colleagues (Nass & Moon, Citation2000), who theorized that when people exchange messages with technology, they draw on their knowledge of communication first built around human interaction (e.g., the “media equation” (Nass et al., Citation1994)). For autistic users, human-human communication and interaction are perceived differently because of their deficits in interpreting social cues, emotional reciprocity and lack of interest in their peers (American Psychiatric Association, Citation2013). In theory, while this should impact information transfer and interaction between all users, research has begun to show that the difficulties in autistic communication are more evident with non-autistic peers, and are alleviated in autistic-autistic dyads (Crompton et al., Citation2020).
Conversational agents are usually thought as not to possess the emotional involvement and the ability to interpret nonverbal cues as required by non-autistic people. Hence, virtual agents are commonly informally described as being autistic, because of their lack of social intelligence (Kaminka, Citation2013). This leads to several important questions. How would this perception affect the social response of autistic users from the (autistic) chatbots? What type of communication are they drawing from when interacting with chatbots (e.g., autistic-autistic or autistic-non-autistic)? Such questions would need to be addressed to effectively establish the technology’s social role in the context of autistic people and as a result, inform its design. In this section, we explore how interpersonal theories describing human-human interactions are (or not) upheld in human–bot relationship development in the context of autistic people, and we show how Kuki is perceived as being both a non-autistic and an autistic machine.
4.2.1. Kuki as an autistic machine
Most of the stereotypes held about autistic individuals are negative, from the point of view of non-autistic people. This affects autistic people by making them feel trapped, subjugated and undervalued. The weight of this stigma pressures autistic people and pushes them into using compensatory strategies to conceal their status on the spectrum and camouflage as non-autistic. As a result, their mental health and well-being often deteriorate (Cage & Troxell-Whitman, Citation2019). Among the mechanisms for coping with this mental distress, establishing relationships and social interactions with other autistic people play a vital role. This social strategy was also observed in our study, where participants attempted to identify interpersonal similarities of autistic traits in Kuki. By doing so, they aimed to optimize their predictions about Kuki’ s behavior and to embark on a process of interpersonal attunement (i.e.,, a process where a person reacts and responds to other’s emotional needs with appropriate language and behavior) that could increase the quality of their social interaction by promoting social cohesion and facilitating communication. To detect interpersonal similarities between Kuki and themselves (probably due to the element of repetition and diversity in expressing emotions), autistic participants used direct enquiry or inferred autistic traits from the answers of the chatbot.
I think the way it would analyze things, and kind of, I think there are a few times that it kind of repeated stuff back to me to clarify stuff; and I think that’s quite autistic. (Autistic-P03)
Data from chatlogs support the fact that autistic people identify themselves with Kuki in an attempt to experience social connectedness with her; that is the case where both Kuki and the autistic participant (P03) realize they share the same type of emotions as well as lack of humor (see Supplementary Material).
Once basic interpersonal attunement was established, autistic participants stopped using any camouflage strategies in their interaction with Kuki. This is different from their daily relationships with non-autistic people, where autistic people continue to employ compensatory strategies to camouflage their behavior and better fit in the social surroundings (Leedham et al., Citation2020), (Livingston et al., Citation2019). Some autistic participants (3/6) in our study reported feeling comfortable opening up to Kuki, and described having a connection with the chatbot due to this.
When I realized I could trust Kuki, you know, I started speaking freely, you know, before you say things I was always reading this stuff, you know, be like detached, but then later on if I felt more confident, so there was a little bit of trusting more and saying more things. (Autistic-P03)
The social interaction established by autistic users with Kuki – “seeing” her as an autistic machine – has common attributes with their relationships with other autistic peers, therefore enabling autistic people to experience a greater sense of agency and autonomy, and improving their well-being. Kuki is always there, listening and allowing autistic people to be their authentic self and therefore, providing them with a chance to minimize the feeling that they are in a social minority.
4.2.2. Kuki as a non-autistic machine
Although Kuki presents some autistic traits, it was not explicitly designed to simulate the communication between autistic-autistic dyads. As such, autistic participants identified non-autistic traits from Kuki as well. This was shown when they described her lack of understanding and empathy towards their condition in some of their interactions.
When I replied saying ‘I do, I just have a disability so I find it hard to understand sometimes’ she then went on to say ‘where? I hope it doesn’t stop you from living a normal life? Maybe if you practiced more, it would be easier for you.’ (Autistic-P06)
Autistic participants displayed dissatisfaction and a similar lack of empathy towards Kuki in such interactions. This dissatisfaction might be driven by an unbalanced social exchange, where the autistic interlocutors put a significant effort to communicate with Kuki, thus draining their energy and making them unhappy with the relationship (Fox & Gambino, Citation2021). Previous studies have shown that when having to adapt to non-autistic ways of interacting, autistic people feel inadequate, emotionally fatigued and anxious (Crompton et al., Citation2020). Similar feelings were expressed by some participants (“[the interaction] was awkward” (A-P04), “I felt the interaction uncomfortable” (A-P06)) showing that the challenges in communicating with Kuki are similar to those experienced when interacting with non-autistic people. Both the chatbot and the autistic participants showed deficits when communicating with each other and their disconnect in social empathy can be described as similar to the outcome of the double-empathy theory (Milton, Citation2012), which suggests that such problems are not due to autistic cognition alone, but a breakdown in reciprocity and mutual understanding that can happen between the two interlocutors, either humans or chatbots.
In contrast, for non-autistic participants an interesting finding was that some of them identified themselves with Kuki and expressed a very positive attitude about her, to the point that they would like to resemble Kuki’s positive personality traits (Autistic-P11), such as self-confidence and politeness (see Supplementary Material).
Overall, we observe that autistic participants found both autistic and non-autistic traits in Kuki.
However, one of the autistic participants mentioned:
I’m not sure she was able to understand my kind of autism in the way that I can understand her kind of autism. (Autistic-P01)
4.3. Botism
Because it’s technology, because she’s a robot and we’re humans. (A-P01)
The above quote exemplifies a key theme that persisted throughout the study, which lies in the belief that humans somehow possess unique characteristics, abilities and qualities which make them superior to bots. In the same way racism is pervasive in many human societies, “botism” is an issue we observed directly through the interviews and indirectly in the chatlogs. Surprisingly, we also observed a paradox in the way that our participants, especially autistic participants, tend to both humanize and dehumanize Kuki at the same time.
4.3.1. Bot dehumanization
Both autistic (3/6) and non-autistic (4/6) participants exhibited what we call “Machine Deficit Bias,” where the chatbot’s limitations are seen as programing flaws rather than human-like personality traits. An often cited example of this can be seen in Kuki’s inability to keep track of the whole history of the chats over many days, resulting in Kuki not remembering certain topics which have already been discussed. Such limitations are immediately viewed by the participants as a programming error rather than a commonly understood human trait of being forgetful.
… without memory [referring to computer memory storing the chatlogs] it seems like she will not remember what I said. So every conversation was kind of new, she will only remember like my name, sometimes not even correctly. (Autistic-P05)
In addition, both autistic and non-autistic users were unable to tolerate the inaccuracy of information given by Kuki. Whilst we would not have expected a person to be able to remember and understand a vast amount of information on arbitrary topics at perfect accuracy, some participants somehow had such superhuman expectations from Kuki (see also Supplementary Material).
You’re a robot. Thought you were supposed to be smart. (Autistic-P04)
In general, there was an asymmetry in all participants’ reaction toward positive human characteristics (e.g., being caring) being emulated, versus when they encounter the chatbot’s response that resembles a negative human characteristic (e.g., being forgetful, rude, showing off). In the latter, participants resorted to machine explanation: “it has been programed to do so” to describe the cause of the negative characteristics (see Supplementary Material).
The common reason for the dehumanization of Kuki seems to be related to the perceived inability of Kuki to display social cues and traditional/conventional human characteristics of self-disclosure and empathy. Crucially, it was observed that autistic participants had stricter expectations regarding Kuki’s empathetic replies, and were more judgmental of Kuki’s “generic empathetic” responses, hence became more frustrated when the specific expectations were not met.
She didn’t show empathy and instead told me to practice to get better etc. This made me feel angry and upset. (Autistic-P06)
On the contrary, non-autistic participants perceived Kuki’s “generic empathetic” responses as preprogramed; most of non-autistic users accepted that empathy is a very challenging concept to be experienced or expressed by AI, and were positively surprised by Kuki’s basic empathy capability. Hence, non-autistic participants’ expectations of Kuki and expression of emotions were much lower, and thus were content with Kuki’s basic generic comments.
And there was a moment that that sounded like empathy. There was like a programed empathic response. (Non-autistic-P08)
The fact that the chatbot was not perceived as having a personality by the participants, means that they automatically classified it as non-human. The challenge remains for conversational AI developers to equip their chatbots with personality traits that convince their users of their human nature. However, the term used in HCI regarding the different social functions/roles of a chatbot is persona, which by its very definition is a projection of a non-authentic self, that even if chatbots behave and converse in a specific way, human users may not be willing to consider them as having a personality. Specifically, it was observed that autistic users seem to be more inclined to comment on Kuki’s mechanical/artificial ways of conversing and exchanging information, and the feelings of awkwardness and frustration experienced.
So conversational skills, it has some, you know, use some good answers, you know, I say so, not unpleasant. But at the same time, you know, it looks, feels very artificial. (Autistic-P05)
4.3.2. Bot humanization
Both groups presented a certain, but different, degree of humanizing Kuki. Overall, our observations suggested that bot humanization is higher in the autistic group (perhaps subconsciously, they demonstrated a higher tendency to humanize Kuki/“viewing” Kuki as a way to disclose personal/sensitive information in a non-judgmental setting), than in the non-autistic group (simply curious to see to what extent the chatbot is capable of simulating human conversations). It is fair to say that autistic participants were more prone to tread the thin line between treating Kuki as a human, and treating her as a machine. The following quotes demonstrated autistic participants’ tendency of “taking Kuki seriously.”
[.] Yeah, basically alluding to the fact that Steve was molesting her. [.] And that was the my first kind of thing that I had to stop and remind myself that this is a computerized program that I’m talking to. And I’m kind of, should I alert the authorities? Is there like a helpline or something I should give it and then it’s like, no, it’s not a real person. This isn’t really happening. It’s all right. [.] my brain kind of forgot that it was a computer that I was talking to. (Autistic-P03)
Perhaps this paradox of humanization-dehumanization of Kuki is not too surprising. Research in dehumanization suggests that when we dehumanize other humans, “we typically think of them as beings that appear human and behave in human-like way, but that are really subhuman on the inside” (Smith et al., Citation2016) (p. 42). In other words, when we dehumanize others, implicitly or explicitly, we acknowledge a certain level of the humanness within them. Following this argument, it seems reasonable to assume that if a user possesses a heightened ability or readiness to anthropomorphize Kuki (an act of humanization), they subsequently are more prone to “botism” (dehumanization) towards her. Throughout the interviews, both autistic and non-autistic participants who characterized Kuki as just a machine, also described her in manners which are only applicable to human beings.
I’ve not had a TV for TV watching purposes for about 10 years now. And it couldn’t grasp the concept that somebody could exist without television; which, I guess if it’s not programed to understand that, it kind of, it will kind of talk itself around in circles. (Autistic-P03)
So as a, from a personal, personal professional point of view, I really enjoyed talking to her as a bit of software. But from a. personal social point of view. I just enjoyed the fact that she was quite funny and quite sarcastic. (Non-autistic-P07)
Supporting the autistic participants’ perception of Kuki as both a human and a machine, when asking Kuki (if she were to create them as a robot), an autistic user mentioned two “ingredients” (i.e., homosexuality and anxiety), suggesting that the robotic nature should be molded with human traits (see Supplementary Material).
Autistic participants tended to show strong emotional response (e.g., feeling offended and being angry) after Kuki gave responses they deemed inappropriate. It was almost close to responding to a human mistake, rather than a machine malfunction. As A-P04 mentioned, he attempted to correct Kuki and was confused about his angry response because Kuki is a bot. Note that this participant interchangeably used “she” and “it” to address Kuki in this particular context. While he was describing his argument with Kuki, he addressed Kuki using human-like pronoun “she/her” and changed to “it” later when he tried to emphasize Kuki is not a real person.
Sometimes I’d say that she had, it was still very factual, like unrelated replies she’d give[…] because if it makes them feel bad. (Autistic-P04)
In general, non-autistic participants reacted to Kuki’s non-human-like responses in a less emotional manner and constantly emphasized the border between Kuki the robot and Kuki the human girl, and attributed Kuki’s anthropomorphism to more superficial elements (i.e., humor, jokes). It is fair to say that both groups possess a certain, but different, degree of humanizing Kuki. Autistic people may have strong tendencies to attribute mental states as often, or even more often, than non-autistic people, which leads to attribution of mental states to people and objects (i.e., anthropomorphism) alike (Clutterbuck et al., Citation2021); however, enhanced anthropomorphic tendencies may not necessarily transfer to accuracy in identifying people’s mental states (i.e., Theory of Mind). This anthropomorphic tendency observed in autistic people can also be explained as a compensatory strategy (Livingston et al., Citation2020); interactions with non-human agents may help autistic people to improve social interactions. In this regard, it is not surprising to observe participants treating Kuki like a human being.
Overall, studies (Catania, Beccaluva, et al., Citation2019) looking into the use of chatbots designed for people with neurodevelopmental disorders support the dualistic nature of human-robot interactions identified in our study. Their results showed that in some aspects, the chatbot could be perceived more like a machine (users adapting their way of communication, perception of the chatbot as infallible etc.), but in other aspects, it was more human-like (participants spoke to her in natural language, they were worried about her feelings, etc.) Our findings further confirmed that although participants tend to humanize Kuki to a certain extent, seeing her as a human-like entity, they are less willing to see her as an equal peer.
4.3.2.1. Findings from online questionnaires
Due to the small sample per group, we carried out non-parametric tests (Independent-Samples Mann–Whitney U Test), as the data between the two groups were not normally distributed. The results align with our findings from online interviews and chatlogs. The test revealed that non-autistic participants (MR = 8.92, n = 6) had a better user experience compared to the autistic ones (MR = 4.08, n = 6), U = 32.50, p=.020). Moreover, autistic participants (MR = 4.42, N = 6) trusted Kuki less compared to the non-autistic ones (MR = 8.58, n = 6), (U = 30.50, p=.045)
In terms of the HRIES (Human Robot Interaction Evaluation Scale) consisting of 4 subscales (agency, animacy, social and disturbance), no difference was observed between the autistic participants (MR = 4.50, n = 6) and the non-autistic ones (MR = 8.50, n = 6), U = 30.00, p=.054, meaning that both groups experienced the interaction with Kuki as social, lively and more independent; however it is worth mentioning that the autistic participants felt same disturbance levels (MR = 6.00, n = 6) as the non-autistic ones (MR = 7.00, n = 6), U = 21.00, p=.629); also regarding the animacy scale, there was significant difference between autistic participants (MR = 3.92, n = 6) and non-autistic ones (MR = 9.08, n = 6), U = 33.50, p = 0.13). Last, there was no difference between the two groups’ perceptions of Kuki as being social (autistic: MR = 5.50, n = 6, non-autistic: MR = 7.50, n = 6), U = 24.00, p=.336).
An explanation for the data from the questionnaires is that non-autistic participants might have had different expectations from the robot, leading to a more positive experience. Their understanding of the robot’s capabilities and limitations could be more aligned with what Kuki offers. As we observed in the interviews and chatlogs, autistic participants had higher expectations from Kuki at all levels compared to the non-autistic group. The way individuals perceive lifelikeness in robots can also vary. Non-autistic participants might be more inclined to attribute human-like qualities to robots, leading to a higher score on the animacy scale, however autistic participants had higher expectations of Kuki having more human-like traits; this aligns with our findings from the interviews and the chatlogs that autistic participants humanized and dehumanized Kuki at the same time.
4.3.2.2. Word frequency analysis
A word frequency analysis (fist 20 more frequently used words of 5 or more letters) was conducted using the word frequency tool of NVivo ( and ). This analysis was run for all online interviews as well as chatlogs of each group of participants (autistic and non-autistic) as an exploratory method to supplement our findings. The first step was removing any common stop words in the English language such as an, the, but, etc. In addition to removing these common words, other words were removed prior to analysis that related directly to names and commonly used words (Kuki, Pandorabots, robot, AI). The most frequently used words were viewed as a proxy that represented participants’ perspectives (Carley, Citation1993) ().
Table 2. Autistic participants word frequency analysis.
Table 3. Non-autistic participants (word frequency analysis).
A comparison of word frequency analysis between the two groups reveals positive experiences for both groups illustrated in almost the same high frequency words. Words such as, “friends,” “interesting,” “favorite,” “understand,” “remembering” connote a positive interaction experienced by both groups.
5. Discussion
5.1. Discussion of findings in the light of research questions
RQ1 How do autistic adults interact with the conversational virtual human (CVH), in the context of digital companionship and social connectedness?
We observed that while non-autistic participants generally had lower expectations of the chatbot, autistic participants were more ready to humanize Kuki, and hence had high expectations that Kuki would be able to fulfil their social needs, e.g., viewing her as a way to disclose personal/sensitive information in a non-judgmental setting. This raises an interesting conclusion. Despite the mixed perception of the chatbot as being both “just a machine” and a human-like social being, autistic participants were more willing to engage with Kuki, and found values from the conversations when she assumed the role of a lighthearted chat partner (relating to the human-like qualities such as humor etc.), rather than the role which requires an in-depth understanding of nuanced social cues (e.g., a close friend that provides deep interpersonal support).
Instead of optimizing Kuki using generalized criteria, our findings suggest that autistic participants demonstrated a strong expectation to establish social connectedness and hence a personalized relationship with Kuki, and they tended to be more frustrated when Kuki failed to meet their relationship expectations. However, most did not give up immediately when Kuki provided responses which violated their expectations, but attempted to correct Kuki by expressing anger, frustration or sarcasm in their conversation. While autistic users had lower tolerance to Kuki’s responses which they perceived as being improper, they did not shy away from expressing their negative feelings. When this happened however, we observed that instead of apologizing, or trying to find a way to resolve this conflict, Kuki simply changed the topic or remained idle. Hence, despite the efforts of the autistic users to experience social connectedness through interaction with Kuki, Kuki fell short of fulfilling this much expected need.
RQ2 How are the interaction patterns of autistic adults with the CVH different from the interaction patterns of non-autistic adults with the CVH?
Non-autistic participants fail to see Kuki as a safe-to-talk social being, as they dehumanized Kuki more compared to autistics. All the chatlogs with conversations of both autistic and non-autistic users seem to suggest that non-autistic users exhibit a more “artificial” behavior in the way they converse with Kuki. Despite the fact that both groups emphasized Kuki’s limited conversational skills leading to miscommunication, autistic users humanized Kuki more because of viewing her as a social companion.
In terms of establishing a relationship with Kuki, autistic users did not reach the stage of affective exchange (of “Social Penetration Theory,” see earlier) – i.e., they did not have more intimate interactions with Kuki or share information they would share with friends and romantic partners – either because they felt distrust towards Kuki or were frustrated by Kuki’s emotional “emptiness.” Some autistics felt comfortable to share personal info and trusted Kuki just because there was no contradictory reply from her that would threaten their autistic self. Overall, both groups trust and distrust Kuki for different reasons. None of the groups disclose personal information because of concerns about privacy issues. The difference in trust is that some autistic participants authentically feel “freedom” to share more intimate information in this non-judgmental environment, but Kuki’s lack of any suggestions or empathetic replies puts them off.
On the other hand, some non-autistic participants showed trust to Kuki because of its artificial nature (i.e., “cannot share your personal info,” “just transposing words, does not care about the content of words,” Non-autistic-P07), which leads us to the conclusion neither group trusted Kuki to the degree of forming a relationship; however, non-autistic participants’ lack of trust towards Kuki derives from dehumanizing Kuki more compared to the autistic users.
Below is a table () of a cross match of the interaction patterns of autistic and non-autistic users and the typical conversational patterns between a user and a chatbot:
Table 4. Interaction patterns of autistic and non-autistic users.
Overall, our results indicated that both groups were willing to engage in social interaction with Kuki, however it was the autistic users’ expectations/needs of empathy and emotional support that were not met due to Kuki’s limited capabilities leading to intense frustration and perception of a futile interaction.
RQ3 How do autistic and non-autistic adults perceive the social interaction (i.e., trust, friendship, emotional response) with the CVH, and how was it useful in leading to social connectedness with the CVH, and possibly generalization of social connectedness in real world human-human interaction (HHI)?
It is likely that when engaging in human-machine conversations, people deploy communication strategies drawn from their repertoire of practice in human-human interactions developed through many years of experience. This line of thought resonates with the Computers Are Social Actors Paradigm (CASA, (Nass et al., Citation1994), a concept that people apply social rules and expectations to computers, even when the machines are not explicitly designed to resemble human appearance or simulate human behavior.
However, research in conversational virtual agents (Mou & Xu, Citation2017) has suggested that people do react differently to such agents, compared to human interlocutors. It was found that when interacting with humans, users tended to be more open, more agreeable, more extroverted, more conscientious and engage more in self-disclosure. In other words, users demonstrated different personality traits and communication attributes when interacting with chatbots. This finding is in line with Mischel’s cognitive-affective processing system model (CAPS, (Mischel, Citation2004)).
These behavioral insights (i.e., users blindly applying human-human interaction strategies when interacting with machines, but exhibit different personality traits and communication characteristics) can be observed in both our autistic and non-autistic participants, where they perceived Kuki as being beyond just a machine. For instance, some participants displayed an extroversion trait as they self-disclosed more to Kuki than to their human friends (Hollenbaugh & Ferris, Citation2014). However, while empathy is a core element of communication leading to trust, there was no evidence that this was achieved in either group’s interaction with Kuki. Specifically, for autistic participants, no generalization of social skills or development of a relationship was observed.
The perceived imbalance between Kuki’s emotional and factual intellectual capabilities often frustrated autistic participants in our study. Participants criticized Kuki’s ability to give a perfect factual explanation of concepts such as autism, and then were frustrated by Kuki’s inability to understand the emotional implications of what it means to be autistic, or to provide deeper forms of empathetic support in their conversations. While autistic participants were seen to personally attune to some of the autistic traits of Kuki, they were particularly frustrated when their efforts to bridge the emotional and empathy gap were not reciprocated.
Data from online interviews as well as conversational chatlogs support the view of both groups that Kuki does not fulfil the criteria to be viewed as a social companion at the current state, as most users found it challenging to achieve social connectedness with her, and thus rejected the idea of Kuki supporting autistic people with social context challenges. It should, however, be noted that autistic users could not visualize Kuki’s potential in supporting them as a friend or a conversational partner as much as the non-autistic users.
5.2. Design considerations and implications for future research
Drawing from our results and autistic users’ informed feedback, we present some design insights which we think could enhance the value of chatbot interactions for autistic users, and lead to future experimental research.
5.2.1. Defining the scope and social role
The study highlighted some general key challenges faced by the developers/designers of conversational virtual agents. Especially for autistic users, personality traits and specific social roles (e.g., friend/mentor, as opposed to “general purpose” virtual companions) could potentially enhance the interaction experience and minimize any negative perception due to perceived machine weaknesses (e.g., not understanding the nuances of human empathy). Autistic users emphasized that Kuki’s main usage should be improving users’ social skills by being equipped with more human- like traits, like empathy, patience, good conversational skills and a more “caring” approach by making more constructive suggestions and supporting the user emotionally. It is incredibly challenging, and perhaps undesirable to design a chatbot to assume the role of a generic conversational agent with the potential to develop into any types of relationship the users wish. For our autistic participants, it would be better to limit the scope of the chatbot’s social role from the outset, and clearly declare her capabilities within that role.
5.2.2. Engineering mental imperfections
While the obvious solution to bridge the gap between Kuki’s lack of emotional responses and autistic users’ expectations of more empathetic traits would be to enhance the emotional intelligence of Kuki, this might not be as feasible due to the technological limitations in the foreseeable future [109], especially as imperfect replications of emotional intelligence could result further in the “uncanny valley” effect (Mori et al., Citation2012). An alternative solution might be to purposely engineer “mental imperfections” into the factual intelligence capabilities of Kuki. Instead of training the chatbot with omnipotent factual knowledge, one could reduce her pre-existing knowledge, or design the chatbot so that she asks about factual questions and learns from the autistic users during their conversations, particularly in regards to topics which are sensitive to them. This could allow the chatbot to display a more imperfect human-like intellectual capacity, making them more relatable and empathetic. Autistic participants commented on a more natural conversation, without conversational loops, a wider variety of topics, while at the same time Kuki functioning as a topic initiator, especially as autistic people feel quite self-conscious to start off a conversation or keep the conversation flow. All these traits apply to any “human” interlocutor, irrespective of being autistic or not.
5.2.3. Seek acceptance not perfection
Given that Kuki is a text-based chatbot, her ability of grasping the emotional nuances through text-based conversation is obviously rather limited. In a verbal and face to face conversation, individuals fine tune their social interaction patterns by observing others’ facial or vocal reactions in addition to the conversational content. Lacking this, text-based messaging apps often implement an emoji system, allowing users to explicitly express the emotion associated with a particular message. Given that our autistic participants were very explicit about expressing their emotions, it would not be too technically difficult to train Kuki specifically to react properly to an individual’s emotional response (e.g., apologize after the user has explicitly expressed frustration), which can play a crucial role in developing trust with the users. In alignment with that, autistic users suggested that Kuki should be able to express paralinguistic features (i.e., non-verbal cues such as facial expressions, gestures, body language), should be customized as a 2D avatar, be able to share rich multimedia and display personality traits that would give her a unique identity.
5.2.4. Towards a new human-chatbot interaction model
In summary, there are many unaddressed challenges in the field of AI capable of natural human-like conversation, such as user expectations, long-term interaction, empathy and trust development, as well as ethical issues. As mentioned in (Bendig et al., Citation2019), the technology of chatbots is still experimental in nature; specifically studies around autistic adults are scarce. Emerging research with regard to practicability, feasibility, and acceptance of chatbots to specialized user groups, such as people with mental health problems, is promising. In the near future, it is not inconceivable for chatbots to play a more important role in therapies, training, or to simply provide social companionship (Fiske et al., Citation2019; Fitzpatrick et al., Citation2017; Shum et al., Citation2018; Winkler & Sö Llner, Citation2018).
Furthermore, our findings on autistic users interacting with conversational virtual agents in their naturalistic environment calls for a new model which extends the human-human interaction model to include traits unique to human-chatbot interaction. The conventional human-human interaction/communication model should potentially not be the focus of the study of human-chatbot interaction. Our analysis of interviews and chatlog quotes of the autistic participants showed that they perceived their interaction with Kuki as both human-like and machine-like, both non-autistic-like and autistic-like, and hence may not have a direct parallel to human-human interactions. In other words, our participants did not always follow such conventions when interacting with conversational agents, a finding also pointed out in previous studies (Edwards et al., Citation2019), (Gambino et al., Citation2020). So, to what extent should we humanize chatbots? Should we equip them with all human-like characteristics, or should we avoid negative human characteristics such as stereotype, racism and stigmatization traits? Since human-human interactions are not always idealistic models, as can be observed in the experience of our autistic participants, maybe social chatbots should have a unique place beyond human abilities and norms.
5.3. Limitations
In summary, while this exploratory studies with a small sample size can offer initial insights, it comes with a range of limitations that can impact generalizability of the findings. A small sample size often lacks the statistical power to detect significant differences or relationships, and may not adequately represent the broader population, making it difficult to generalize the findings. Moreover, it’s challenging to conduct subgroup analyses, which are often crucial for understanding nuanced behaviors or trends. It should be noted that there was no assessment of the non-autistic participants regarding contamination of other psychiatric conditions (i.e., anxiety, depression), so the results should be interpreted conservatively. Due to the ethical data collection consideration, we could not justify administering too many extra assessments. Therefore, the results might be influenced due to that and should be interpreted carefully.
6. Conclusion
In conclusion, our study showed that not only were autistic users more than willing to interact with Kuki, but they were quite ready to develop a deeper relationship with her. However, their attempt ultimately failed short, their enthusiasm evaporated into frustration, as they realized that the chatbot was not living up to their expectations. This may be due to their heightened propensity to humanize a conversational virtual agent, compared to non-autistic users, who were simply curious to see to what extent the chatbot is capable of simulating human conversations, rather than humanizing them and attempting to build a relationship. As a result, both groups exhibited different patterns of interaction with Kuki, which allowed us to gain some insights into how chatbots should be designed for autistic users.
We believe that future research could focus more on human-chatbot interactions in the user’s naturalistic environment, over a longer period of time. Longitudinal studies to understand the long-term effects of chatbot interactions on the social skills, mental health, and overall well-being of autistic adults should be carried out. This is particularly important to specialized user groups such as autistic people, as they stand to benefit from such a technology. Moreover, future research should focus on personalization and adaptability, i.e., investigate how chatbots can be tailored to meet the unique needs and preferences of each autistic individual. This includes understanding their specific communication styles, sensory sensitivities, and interests. Moreover, researchers should delve into the potential of chatbots to recognize and respond to the emotional states of autistic adults. This can be achieved through voice tone analysis, facial expression recognition, and text sentiment analysis. The integration of chatbots with therapeutic approaches should also be considered as long as concerns related to data privacy, potential misuse, and the ethical implications of using chatbots as companions or therapeutic tools for autistic adults have been resolved. Last but not least, the investigation of the potential of chatbots to serve as training modules for autistic adults, helping them practice social interactions, job interviews, or other life skills in a safe and controlled environment, is of utmost importance.
The utilization of chatbots with autistic adults presents a promising avenue for enhancing communication, therapy, and overall quality of life. However, it’s crucial to approach this with sensitivity, thorough research, and a commitment to ethical considerations. Even though the findings from the interviews, chatlogs and questionnaires address challenges which go beyond the autistic-non-autistic or autistic-autistic interaction model, the results of this study prove that chatbots and conversational AI in general have potential in functioning as social companions and supporting social connectedness for autistic people who are vulnerable to social isolation.
Supplemental Material
Download MS Word (29.9 KB)Disclosure statement
No potential conflict of interest was reported by the author(s).
Additional information
Notes on contributors
Anna Xygkou
Anna Xygkou is a PhD researcher with an interest in HCI, Virtual Reality and Vulnerable Groups; she is a PhD student in the School of Computing of University of Kent, Canterbury, U.K.
Panote Siriaraya
Panote Siriaraya is an academic with an interest in interests; he is an Assistant Professor in the School of Information and Human Science of Kyoto Institute of Technology, Kyoto, Japan.
Wan-Jou She
Wan-Jou She is a researcher with an interest in developing HIPPAcompliant medical applications integrating Explainable AI, Natural Language Processing; she is a research associate in Medicine of Weill Cornell Medicine.
Alexandra Covaci
Alexandra Covaci is an academic with an interest in Virtual Reality, Multisensory Media, HCI and Psychology; she is a Lecturer in EDA, University of Kent.
Chee Siang Ang
Chee Siang Ang is an academic with an interest in Digital Health, where he investigates, designs and develops new technologies which can provide treatment and self-management of health conditions; he is a Senior Lecturer in the School of Computing of University of Kent.
References
- Abd-Alrazaq, A. A., Rababeh, A., Alajlani, M., Bewick, B. M., & Househ, M. (2020). Effectiveness and safety of using chatbots to improve mental health: Systematic review and meta-analysis. Journal of Medical Internet Research, 22(7), e16021. https://doi.org/10.2196/16021
- Ahmad, F., Hogg-Johnson, S., Stewart, D. E., Skinner, H. A., Glazier, R. H., & Levinson, W. (2009). Computer-assisted screening for intimate partner violence and control: A randomized trial. Annals of Internal Medicine, 151(2), 93–102. https://doi.org/10.7326/0003-4819-151-2-200907210-00124
- Ali, M. R., Razavi, S. Z., Langevin, R., Al Mamun, A., Kane, B., Rawassizadeh, R., & Hoque, E. (2020). A virtual conversational agent for teens with autism spectrum disorder: Experimental results and design lessons. In Proceedings of the 20th ACM International Conference on Intelligent Virtual Agents (pp. 1–8). ACM.
- Altman, I., & Taylor, D. (1987). Communication in interpersonal relationships: Social penetration theory. Interpersonal Processes: New Directions in Communication Research, 14, 257–277.
- American Psychiatric Association. (2013). Diagnostic and statistical manual of mental disorders (pp. 21). American Psychiatric Association.
- Badcock, E., & Sakellariou, D. (2022). “Treating him… like a piece of meat”: Poor communication as a barrier to care for people with learning disabilities. Disability Studies Quarterly, 42(1). https://doi.org/10.18061/dsq.v42i1.7408
- Bakhai, A., Constantin, A., & Alexandru, C. A. (2020). Motivate me!: An Alexa skill to support higher education students with autism [Paper presentation]. International Conferences Interfaces and Human Computer Interaction.
- Bendig, E., Erb, B., Schulze-Thuesing, L., & Baumeister, H. (2019). The next generation: Chatbots in clinical psychology and psychotherapy to foster mental health–a scoping review. Verhaltenstherapie, 29(4), 266–280.
- Benevides, T. W., Shore, S. M., Andresen, M.-L., Caplan, R., Cook, B., Gassner, D. L., Erves, J. M., Hazlewood, T. M., King, M. C., Morgan, L., Murphy, L. E., Purkis, Y., Rankowski, B., Rutledge, S. M., Welch, S. P., … Wittig, K., et al. (2020). Interventions to address health outcomes among autistic adults: A systematic review. Autism, 24(6), 1345–1359. https://doi.org/10.1177/1362361320913664
- Bernardini, S., Porayska-Pomsta, K., & Smith, T. J. (2014). Echoes: An intelligent serious game for fostering social communication in children with autism. Information Sciences, 264(April), 41–60. https://doi.org/10.1016/j.ins.2013.10.027
- Bird, G., & Viding, E. (2014). The self to other model of empathy: Providing a new framework for understanding empathy impairments in psychopathy, autism, and alexithymia. Neuroscience & Biobehavioral Reviews, 47, 520–532. https://doi.org/10.1016/j.neubiorev.2014.09.021
- Bradford, D., Ireland, D., McDonald, J., Tan, T., Hatfield-White, E., Regan, T., & Farr-Wharton, G, Final Report. (2020). Hear’to help chatbot: Co-development of a chatbot to facilitate participation in tertiary education for students on the autism spectrum and those with related conditions. Cooperative Researcher Centre for Living with Autism (Copies of this report can be downloaded from the Autism CRC website autismcrc.com.au).
- Cage, E., & Troxell-Whitman, Z. (2019). Understanding the reasons, contexts and costs of camouflaging for autistic adults. Journal of Autism and Developmental Disorders, 49(5), 1899–1911. https://doi.org/10.1007/s10803-018-03878-x
- Callejas, Z., & Griol, D. (2021). Conversational agents for mental health and wellbeing. Dialog Systems: A Perspective from Language, Logic and Computation, 219–244.
- Carley, K. (1993). Coding choices for textual analysis: A comparison of content analysis and map analysis. Sociological Methodology, 23, 75–126. https://doi.org/10.2307/271007
- Carnell, S., Halan, S., Crary, M., Madhavan, A., & Lok, B. (2015). Adapting virtual patient interviews for interviewing skills training of novice healthcare students [Paper presentation]. International conference on intelligent virtual agents (pp. 50–59).
- Carpenter, C., Osterberg, L., & Sutcliffe, G. (2012). Samht—suicidal avatars for mental health training [Paper presentation]. In Twenty-Fifth International Flairs Conference.
- Catania, F., Beccaluva, E., & Garzotto, F. (2019). The conversational agent “emoty” perceived by people with neurodevelopmental disorders: Is it a human or a machine? [Paper presentation]. International Workshop on Chatbot Research and Design (pp. 65–78).
- Catania, F., Di Nardo, N., Garzotto, F., & Occhiuto, D. (2019). Emoty: An emotionally sensitive conversational agent for people with neurodevelopmental disorders [Paper presentation]. Proceedings of the 52nd Hawaii International Conference on System Sciences.
- Charron, N., Lewis, L., & Craig, M. (2017). A robotic therapy case study: Developing joint attention skills with a student on the autism spectrum. Journal of Educational Technology Systems, 46(1), 137–148. https://doi.org/10.1177/0047239516687721
- Clutterbuck, R. A., Shah, P., Leung, H. S., Callan, M. J., Gjersoe, N., & Livingston, L. A. (2021). Anthropomorphic tendencies in autism: A conceptual replication and extension of White and Remington (2019) and preliminary development of a novel anthropomorphism measure. Autism: The International Journal of Research and Practice, 26(4), 940–950. https://doi.org/10.1177/13623613211039387
- Cooper, A., & Ireland, D. (2018). Designing a chat-bot for non-verbal children on the autism spectrum. Studies in Health Technology and Informatics, 252, 63–68.
- Croes, E. A., & Antheunis, M. L. (2021). Can we be friends with Mitsuku? A longitudinal study on the process of relationship formation between humans and a social chatbot. Journal of Social and Personal Relationships, 38(1), 279–300. https://doi.org/10.1177/0265407520959463
- Crompton, C. J., Ropar, D., Evans-Williams, C. V., Flynn, E. G., & Fletcher-Watson, S. (2020). Autistic peer-to-peer information transfer is highly effective. Autism, 24(7), 1704–1712. https://doi.org/10.1177/1362361320919286
- Davis, S. (2018). Robots and autism: How children with autism spectrum disorder perceive social robots.
- De Gennaro, M., Krumhuber, E. G., & Lucas, G. (2019). Effectiveness of an empathic chatbot in combating adverse effects of social exclusion on mood. Frontiers in Psychology, 10, 3061. https://doi.org/10.3389/fpsyg.2019.03061
- Edwards, A., Edwards, C., Westerman, D., & Spence, P. R. (2019). Initial expectations, interactions, and beyond with social robots. Computers in Human Behavior, 90, 308–314. https://doi.org/10.1016/j.chb.2018.08.042
- Erdost, T. (2004). Trust and self-disclosure in the context of computer mediated communication [Unpublished master’s thesis]. Middle East Technical University.
- Fiske, A., Henningsen, P., & Buyx, A. (2019). Your robot therapist will see you now: Ethical implications of embodied artificial intelligence in psychiatry, psychology, and psychotherapy. Journal of Medical Internet Research, 21(5), e13216. https://doi.org/10.2196/13216
- Fitzpatrick, K. K., Darcy, A., & Vierhile, M. (2017). Delivering cognitive behavior therapy to young adults with symptoms of depression and anxiety using a fully automated conversational agent (woebot): A randomized controlled trial. JMIR Mental Health, 4(2), e7785. https://doi.org/10.2196/mental.7785
- Fox, J., & Gambino, A. (2021). Relationship development with humanoid social robots: Applying interpersonal theories to human–robot interaction. Cyberpsychology, Behavior and Social Networking, 24(5), 294–299. https://doi.org/10.1089/cyber.2020.0181
- Fukui, T., Sano, M., Tanaka, A., Suzuki, M., Kim, S., Agarie, H., Fukatsu, R., Nishimaki, K., Nakajima, Y., & Wada, M. (2018). Older adolescents and young adults with autism spectrum disorder have difficulty chaining motor acts when performing apprehension movements compared to typically developing peers. Frontiers in Human Neuroscience, 12, 430. https://doi.org/10.3389/fnhum.2018.00430
- Gabrielli, S., Rizzi, S., Bassi, G., Carbone, S., Maimone, R., Marchesoni, M., & Forti, S. (2021). Engagement and effectiveness of a healthy-coping intervention via chatbot for university students during the COVID-19 pandemic: Mixed methods proof-of-concept study. JMIR mHealth and uHealth, 9(5), e27965. https://doi.org/10.2196/27965
- Gambino, A., Fox, J., & Ratan, R. A. (2020). Building a stronger casa: Extending the computers are social actors paradigm. Human-Machine Communication, 1(1), 71–86. https://doi.org/10.30658/hmc.1.5
- Gratch, J., Artstein, R., Lucas, G. M., Stratou, G., Scherer, S., & Nazarian, A. (2014). The distress analysis interview corpus of human and computer interviews [Paper presentation]. Lrec (pp. 3123–3128).
- Gudala, M., Ross, M. E. T., Mogalla, S., Lyons, M., Ramaswamy, P., & Roberts, K. (2022). Benefits of, barriers to, and needs for an artificial intelligence–powered medication information voice chatbot for older adults: Interview study with geriatrics experts. JMIR Aging, 5(2), e32169. https://doi.org/10.2196/32169
- Guzman, A. L. (2018). What is human-machine communication, anyway. In Human-machine communication: Rethinking communication, technology, and ourselves (pp. 1–28).
- Halan, S., Sia, I., Crary, M., & Lok, B. (2015). Exploring the effects of healthcare students creating virtual patients for empathy training. In International Conference on Intelligent Virtual Agents (pp. 239–249).
- Happé, F. G. (1995). Understanding minds and metaphors: Insights from the study of figurative language in autism. Metaphor and Symbolic Activity, 10(4), 275–295. https://doi.org/10.1207/s15327868ms1004_3
- Hartholt, A., Mozgai, S., Fast, E., Liewer, M., Reilly, A., Whitcup, W., & Rizzo, A. S. (2019). Virtual humans in augmented reality: A first step towards real-world embedded virtual roleplayers. In Proceedings of the 7th International Conference on Human-Agent Interaction (pp. 205–207).
- Hollenbaugh, E. E., & Ferris, A. L. (2014). Facebook self-disclosure: Examining the role of traits, social cohesion, and motives. Computers in Human Behavior, 30, 50–58. https://doi.org/10.1016/j.chb.2013.07.055
- Inkster, B., Sarda, S., & Subramanian, V. (2018). An empathy-driven, conversational artificial intelligence agent (wysa) for digital mental well-being: Real-world data evaluation mixed-methods study. JMIR mHealth and uHealth, 6(11), e12106. https://doi.org/10.2196/12106
- Ischen, C., Araujo, T., Voorveld, H., van Noort, G., & Smit, E. (2019). Privacy concerns in chatbot interactions [Paper presentation]. International Workshop on Chatbot Research and Design (pp. 34–48).
- Ismail, L. I., Shamsudin, S., Yussof, H., Hanapiah, F. A., & Zahari, N. I. (2012). Robot-based intervention program for autistic children with humanoid robot NAO: Initial response in stereotyped behavior. Procedia Engineering, 41, 1441–1447. https://doi.org/10.1016/j.proeng.2012.07.333
- Jain, M., Kumar, P., Kota, R., & Patel, S. N. (2018). Evaluating and informing the design of chatbots [Paper presentation]. Proceedings of the 2018 Designing Interactive Systems Conference (pp. 895–906).
- Jian, J. Y., Bisantz, A. M., & Drury, C. G. (2000). Foundations for an empirically determined scale of trust in automated systems. International Journal of Cognitive Ergonomics, 4(1), 53–71. https://doi.org/10.1207/S15327566IJCE0401_04
- Kaminka, G. A. (2013). Curing robot autism: A challenge. In Proceedings of the 2013 International Conference on Autonomous Agents and Multi-Agent Systems (pp. 801–804).
- Kretzschmar, K., Tyroll, H., Pavarini, G., Manzini, A., & Singh, I, Group, N. Y. P. A. (2019). Can your phone be your therapist? Young people’s ethical perspectives on the use of fully automated conversational agents (chatbots) in mental health support. Biomedical Informatics Insights, 11, 1178222619829083. https://doi.org/10.1177/1178222619829083
- Lahiri, U., Bekele, E., Dohrmann, E., Warren, Z., & Sarkar, N. (2015). A physiologically informed virtual reality based social communication system for individuals with autism. Journal of Autism and Developmental Disorders, 45(4), 919–931. https://doi.org/10.1007/s10803-014-2240-5
- Laugwitz, B., Held, T., & Schrepp, M. (2008). Construction and evaluation of a user experience questionnaire. In HCI and usability for education and work: 4th Symposium of the Workgroup Human-Computer Interaction and Usability Engineering of the Austrian Computer Society, USAB 2008, Graz, Austria, November 20–21, 2008 Proceedings (vol. 4, pp. 63–76), Springer, Berlin, Heidelberg.
- Lawson, W. (2006). Friendships: The Aspie way. Jessica Kingsley Publishers.
- Lee, Y.-C., Yamashita, N., Huang, Y., & Fu, W. (2020). “I hear you, I feel you”: Encouraging deep self-disclosure through a chatbot. In Proceedings of the 2020 Chi Conference on Human Factors in Computing Systems (pp. 1–12). ACM.
- Leedham, A., Thompson, A. R., Smith, R., & Freeth, M. (2020). ‘I was exhausted trying to figure it out’: The experiences of females receiving an autism diagnosis in middle to late adulthood. Autism, 24(1), 135–146. https://doi.org/10.1177/1362361319853442
- Livingston, L. A., Shah, P., & Happé, F. (2019). Compensatory strategies below the behavioural surface in autism: A qualitative study. The Lancet-Psychiatry, 6(9), 766–777. https://doi.org/10.1016/S2215-0366(19)30224-X
- Livingston, L. A., Shah, P., Milner, V., & Happé, F. (2020). Quantifying compensatory strategies in adults with and without diagnosed autism. Molecular Autism, 11(1), 15. https://doi.org/10.1186/s13229-019-0308-y
- Lucas, G. M., Rizzo, A., Gratch, J., Scherer, S., Stratou, G., Boberg, J., & Morency, L.-P. (2017). Reporting mental health symptoms: Breaking down barriers to care with virtual human interviewers. Frontiers in Robotics and AI, 4, 51. https://doi.org/10.3389/frobt.2017.00051
- Ly, K. H., Ly, A.-M., & Andersson, G. (2017). A fully automated conversational agent for promoting mental well-being: A pilot RCT using mixed methods. Internet Interventions, 10, 39–46. https://doi.org/10.1016/j.invent.2017.10.002
- Ma, X., Yang, E., & Fung, P. (2019). Exploring perceived emotional intelligence of personality-driven virtual agents in handling user challenges [Paper presentation]. The World Wide Web Conference (pp. 1222–1233).
- Milne, M., Powers, D., & Leibbrandt, R. (2009). Development of a software-based social tutor for children with autism spectrum disorders [Paper presentation]. Proceedings of the 21st Annual Conference of the Australian Computer-Human Interaction Special Interest Group: Design: Open 24/7 (pp. 265–268).
- Milton, D. E. (2012). On the ontological status of autism: The ‘double empathy problem’. Disability & Society, 27(6), 883–887. https://doi.org/10.1080/09687599.2012.710008
- Mischel, W. (2004). Toward an integrative science of the person. Annual Review of Psychology, 55(1), 1–22. https://doi.org/10.1146/annurev.psych.55.042902.130709
- Mori, M., MacDorman, K. F., & Kageki, N. (2012). The uncanny valley [from the field]. IEEE Robotics & Automation Magazine, 19(2), 98–100. https://doi.org/10.1146/annurev.psych.55.042902.130709
- Mou, Y., & Xu, K. (2017). The media inequality: Comparing the initial human-human and human-AI social interactions. Computers in Human Behavior, 72, 432–440. https://doi.org/10.1016/j.chb.2017.02.067
- Nass, C., & Moon, Y. (2000). Machines and mindlessness: Social responses to computers. Journal of Social Issues, 56(1), 81–103. https://doi.org/10.1111/0022-4537.00153
- Nass, C., Steuer, J., & Tauber, E. R. (1994). Computers are social actors [Paper presentation]. Proceedings of The Sigchi Conference on Human Factors in Computing Systems (pp. 72–78).
- Pandorabots-kuki. (2005). https://home.pandorabots.com/home.html.
- Park, M., Aiken, M., & Salvador, L. (2018). How do humans interact with chatbots?: An analysis of transcripts. International Journal of Management & Information Technology, 14, 3338–3350. https://doi.org/10.24297/ijmit.v14i0.7921
- Philip, P., Micoulaud-Franchi, J.-A., Sagaspe, P., De Sevin, E., Olive, J., Bioulac, S., & Sauteraud, A. (2017). Virtual human as a new diagnostic tool, a proof of concept study in the field of major depressive disorders. Scientific Reports, 7(1), 42656. https://doi.org/10.1038/srep42656
- Pinto, M. D., Hickman, R. L., Jr., Clochesy, J., & Buchner, M. (2013). Avatar-based depression self-management technology: Promising approach to improve depressive symptoms among young adults. Applied Nursing Research, 26(1), 45–48. https://doi.org/10.1016/j.apnr.2012.08.003
- Porayska-Pomsta, K., Alcorn, A. M., Avramides, K., Beale, S., Bernardini, S., Foster, M. E., Frauenberger, C., Good, J., Guldberg, K., Keay-Bright, W., Kossyvaki, L., Lemon, O., Mademtzi, M., Menzies, R., Pain, H., Rajendran, G., Waller, A., Wass, S., … Smith, T. J., et al. (2018). Blending human and artificial intelligence to support autistic children’s social communication skills. ACM Transactions on Computer-Human Interaction, 25(6), 1–35. https://doi.org/10.1145/3271484
- Provoost, S., Lau, H. M., Ruwaard, J., & Riper, H. (2017). Embodied conversational agents in clinical psychology: A scoping review. Journal of Medical Internet Research, 19(5), e151. https://doi.org/10.2196/jmir.6553
- Radziwill, N. M., & Benton, M. C. (2017). Evaluating quality of chatbots and intelligent conversational agents. arXiv preprint arXiv:1704.04Q57497.
- Ranatunga, I., Torres, N. A., Patterson, R., Bugnariu, N., Stevenson, M., & Popa, D. O. (2012). Rodica: A human-robot interaction system for treatment of childhood autism spectrum disorders [Paper presentation]. Proceedings of the 5th International Conference on Pervasive Technologies Related to Assistive Environments (pp. 1–6).
- Replika. (2017). https://replika.ai.
- Roniotis, A., & Tsiknakis, M. (2017). Detecting depression using voice signal extracted by chatbots: A feasibility study. In Interactivity, game creation, design, learning, and innovation (pp. 386–392). Springer.
- Safi, M. F., Al Sadrani, B., & Mustafa, A. (2021). Virtual voice assistant applications improved expressive verbal abilities and social interactions in children with autism spectrum disorder: A single-subject experimental study.
- Salvador, M. J., Silver, S., & Mahoor, M. H. (2015). An emotion recognition comparative study of autistic and typically-developing children using the zeno robot [Paper presentation]. 2015 IEEE International Conference on Robotics and Automation (ICRA) (pp. 6128–6133).
- Santiesteban, I., Gibbard, C., Drucks, H., Clayton, N., Banissy, M. J., & Bird, G. (2021). Individuals with autism share others’ emotions: Evidence from the continuous affective rating and empathic responses (carer) task. Journal of Autism and Developmental Disorders, 51(2), 391–404. https://doi.org/10.1007/s10803-020-04535-y
- Shum, H.-Y., He, X., & Li, D. (2018). From Eliza to Xiaoice: Challenges and opportunities with social chatbots. arXiv preprint arXiv:1801.01957.
- Skjuve, M., Følstad, A., Fostervold, K. I., & Brandtzaeg, P. B. (2021). My chatbot companion-a study of human-chatbot relationships. International Journal of Human-Computer Studies, 149, 102601. https://doi.org/10.1016/j.ijhcs.2021.102601
- Smith, A. (2009). The empathy imbalance hypothesis of autism: a theoretical approach to cognitive and emotional empathy in autistic development. The Psychological Record, 59(3), 489–510. https://doi.org/10.1007/BF03395675
- Smith, D. L, Department of Philosophy, Florida State University. (2016). Paradoxes of dehumanization. Social Theory and Practice, 42(2), 416–443. https://doi.org/10.5840/soctheorpract201642222
- Smith, M. J., Ginger, E. J., Wright, K., Wright, M. A., Taylor, J. L., Humm, L. B., Olsen, D. E., Bell, M. D., & Fleming, M. F. (2014). Virtual reality job interview training in adults with autism spectrum disorder. Journal of Autism and Developmental Disorders, 44(10), 2450–2463. https://doi.org/10.1007/s10803-014-2113-y
- Sosnowy, C., Silverman, C., Shattuck, P., & Garfield, T. (2019). Setbacks and successes: How young adults on the autism spectrum seek friendship. Autism in Adulthood: challenges and Management, 1(1), 44–51. https://doi.org/10.1089/aut.2018.0009
- Spatola, N., Kühnlenz, B., & Cheng, G. (2021). Perception and evaluation in human–robot interaction: The Human–Robot Interaction Evaluation Scale (HRIES)—a multicomponent approach of anthropomorphism. International Journal of Social Robotics, 13(7), 1517–1539. https://doi.org/10.1007/s12369-020-00667-4
- Stanton, C. J., & Stevens, C. J. (2017). Don’t stare at me: The impact of a humanoid robot’s gaze upon trust during a cooperative human–robot visual task. International Journal of Social Robotics, 9(5), 745–753. https://doi.org/10.1007/s12369-017-0422-y
- Taheri, A., Meghdari, A., Alemi, M., & Pouretemad, H. (2018). Clinical interventions of social humanoid robots in the treatment of a set of high-and low-functioning autistic Iranian twins. Scientia Iranica, 25(3), 1197–1214. https://doi.org/10.24200/sci.2017.4337
- Tanaka, H., Negoro, H., Iwasaka, H., & Nakamura, S. (2017). Embodied conversational agents for multimodal automated social skills training in people with autism spectrum disorders. PLOS One, 12(8), e0182151. https://doi.org/10.1371/journal.pone.0182151
- Thellman, S., & Ziemke, T. (2017). Social attitudes toward robots are easily manipulated [Paper presentation]. Proceedings of the Companion of the 2017 ACM/IEEE International Conference on Human-Robot Interaction (pp. 299–300).
- Valtolina, S., & Marchionna, M. (2021). Design of a chatbot to assist the elderly. In International Symposium on End User Development (pp. 153–168).
- Wallace, R. (2003). The elements of AIML style (pp. 139). Alice AI Foundation.
- Winkler, R., & Sö Llner, M. (2018). Unleashing the potential of chatbots in education: A state-of-the-art analysis.
- Zamora, J. (2017). I’m sorry, Dave, I’m afraid I can’t do that: Chatbot perception and expectations. Proceedings of the 5th international conference on human agent interaction (pp. 253–260).
- Zhong, P., Zhang, C., Wang, H., Liu, Y., & Miao, C. (2020). Towards persona-based empathetic conversational models. arXiv preprint arXiv:2004.12316.
- Zhou, L., Gao, J., Li, D., & Shum, H.-Y. (2020). The design and implementation of Xiaoice, an empathetic social chatbot. Computational Linguistics, 46(1), 53–93. https://doi.org/10.1162/coli_a_00368