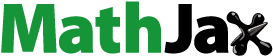
Abstract
Autonomous personal mobility vehicle (APMV) is a miniaturized autonomous vehicle designed for short-distance mobility to everyone. Due to its open design, APMV’s passengers are exposed to communications between the external human-machine interface (eHMI) on APMV and pedestrians. Therefore, effective eHMI designs for APMV need to consider potential impacts of APMV-pedestrian interactions on passengers’ subjective feelings. This study from the perspective of APMV passengers discussed three eHMI designs: (1) graphical user interface (GUI)-based eHMI with text message (eHMI-T), (2) multimodal user interface (MUI)-based eHMI with neutral voice (eHMI-NV), and (3) MUI-based eHMI with affective voice (eHMI-AV). In a riding field experiment (N = 24), eHMI-T made passengers feel awkward during the “silent time” when eHMI-T conveyed information exclusively to pedestrians, not passengers. MUI-based eHMIs with voice cues showed advantages, with eHMI-NV excelling in pragmatic quality and eHMI-AV in hedonic quality. Considering passengers’ personalities and genders in APMV eHMI design is also highlighted.
1. Introduction
1.1. Autonomous personal mobility vehicle
Autonomous personal mobility vehicle (APMV) is a miniaturized vehicle with automation functions ranging from the SAE levels 3–5 automated driving systems that can decide their own driving behavior without passenger involvement. Currently, most well-known APMVs are indeed developed based on electric wheelchairsFootnote1 or semi-open small vehicles.Footnote2 It is important to note that these APMVs are not only developed for the elderly people or people with disabilities; they can be used by anyone for short-distance mobility.
As shown in , unlike autonomous cars, APMVs can be widely used in shared spaces with other traffic participants, such areas include sidewalks, shopping centers, stations, school campuses, and other mixed traffic areas (Ali et al., Citation2019; Kobayashi et al., Citation2013; Liu et al., Citation2020, Citation2023), to facilitate the mobility of passengers. As a result, APMVs will inevitably interact with more vulnerable road users like pedestrians without any protection.
1.2. Interaction issues of APMV in shared spaces
In the aforementioned pedestrian-rich shared spaces, pedestrians may have a low perceived safety when an APMV uses implicit communication, such as changes in maneuvers, to interact with them. For example, Liu et al. (Citation2020, Citation2023) highlighted that pedestrians, when encountering an APMV, were inclined to perceive danger due to confusion in comprehending the driving intentions of the APMV. Furthermore, we consider that this confusion can result in undesirable consequences, such as lower acceptance levels, or even dangers like crashes. Therefore, it is crucial to explore effective ways of communication between APMVs and pedestrians, in order to accurately convey their intentions to each other and ensure safe and efficient interactions between them. One solution to improve APMV–pedestrian communications is to equip an external human–machine interface (eHMI) on the APMV.
1.3. External human–machine Interface and its challenge in user experience for APMV passengers
Based on the literature review, we found that current eHMI designs and research primarily focus on the interactions between autonomous vehicles (AVs) and pedestrians. These eHMIs often rely on the visual cues through light bars, icons, text, and ground projections (Bazilinskyy et al., Citation2021; Dey et al., Citation2021; Li et al., Citation2021, Citation2023; Liu et al., Citation2021). As mentioned in the review article (Brill et al., Citation2023), auditory-based eHMI has been under-studied within the eHMI literature when compared to vision-based eHMIs. A few works comparing the effects of vision-based and auditory-based eHMIs of AVs on pedestrians’ user experience (UE; Dou et al., Citation2021) and reactions to warning messages (Ahn et al., Citation2021), as well as Haimerl et al. (Citation2022) explored the feasibility of auditory eHMI of AVs for pedestrians with intellectual disabilities. Moreover, Kreißig et al. (Citation2023) assess the impact of vision-based and auditory-based eHMIs on the perception of surrounding pedestrians during the autonomous parking of a driver-less E-Cargo bike. To the best of our knowledge, there is presently no research addressing the design of eHMI for APMVs and investigating the UEs of vision-based and auditory-based eHMI for APMVs.
To apply eHMI designs from AVs to APMVs, we must first carefully consider the distinct characteristics that set AVs and APMVs apart. As shown in , unlike AV–pedestrian interactions, APMVs have unique characteristics in their interactions with pedestrians: (i) APMVs are often in closer proximity to pedestrians, given their much smaller sizes and lower driving speeds than AVs, especially in indoor areas such as shopping centers and airports, where pedestrians are populated; and (ii) in addition to close proximity, passengers on the APMV are exposed to the communication between the eHMI deployed on APMVs and pedestrians by the characteristic of the open design. Therefore, to ensure an optimal passenger experience, eHMI designs for APMVs must consider the potential impact of APMV–pedestrian communications on passengers’ subjective feelings.
Based on the characteristics mentioned above, a few studies have used eHMI to improve the interactions between pedestrians and APMVs. For example, Watanabe et al. (Citation2015) used a projector to project the trajectory of an APMV’s movement onto the ground, in order to communicate the motion intentions of the APMV to both pedestrians and passengers. Zhang et al. (Citation2022) conducted a series of interviews with wheelchair users, and revealed the existence of two distinct interaction loops, i.e., APMV–passenger and APMV–pedestrian. Moreover, they suggested a range of design concepts, derived from a virtual workshop, for those two interactions, i.e., projecting information, such as planned path, on the ground for APMV–passenger interaction, and showing trajectory on a display and using vibrotactile feedback for APMV–passenger interactions. Furthermore, based on the related works above, we can clearly see that the eHMIs of APMVs could impact the experience of both passengers and pedestrians. However, most of the current eHMI designs for APMVs only aim to improve pedestrian experience, passengers’ experience has not yet received much attention or been widely discussed.
1.4. Purpose and research questions
To address the research gaps mentioned in the previous section, this article explores communication designs in the interactions between APMVs and pedestrians from the perspective of APMV passengers. As the APMV is for single-passenger use, which is highly associated with the passenger’s own preference, we further consider the passenger’s personality for the eHMI designs. Additionally, considering that gender differences may have an impact on user preference for eHMIs (Chang, Citation2020), we will further discuss the effects of gender differences among passengers on the UE of APMV’s eHMI.
In addition to commonly used graphical user interface (GUI)-based eHMI designs, voice user interface (VUI) can be a useful addition to the GUI-based eHMI (Sodnik et al., Citation2008). Furthermore, considering the perspective of APMV passengers, compared to only GUI-based eHMIs, the voice cues from multimodal user interface (MUI)-based eHMIs can provide confirmative feedback to passengers about the APMV’s communications with pedestrians, avoiding feelings of ignorance. The voice cues can also serve as an alternative communication channel to broadcast the APMV’s intentions to other road users in the vicinity who may be visually occupied.
Therefore, we explored MUI-based eHMIs that incorporate both GUI and verbal message-based VUI on APMVs to communicate with pedestrians. To the best of our knowledge, we are the first to conduct a field study investigating UE of APMV passengers using MUI-based eHMIs with voice cues.
To sum up, in this article, we aim to answer the following three questions through a field study:
RQ 1: To what extent does the silent GUI-based eHMI for AVs apply to APMVs in terms of passenger’s UE?
RQ 2: To what extent do the voice cues of MUI-based eHMI apply to APMVs in terms of passenger’s UE?
RQ 3: Does APMV’s eHMI design need to fit the passenger’s personality?
2. Method
We conducted a passenger-centric experiment using a robotic wheelchair as the APMV. The purpose of the experiment was to investigate the impact of different visual and auditory eHMIs on passengers’ subjective feelings when the APMV communicates with pedestrians. This study has been carried out with the approval of the Research Ethics Committee of Nara Institute of Science and Technology (NAIST) [No. 2022-I-55].
2.1. Participants
A total of 24 participants (12 males and 12 females) with an age range of 22 to 29 years (mean: 23.9, standard deviation: 1.7) participated in this experiment as APMV passengers. All participants reported no previous experience with eHMI and APMVs. It took about one hour for each participant to complete the experiment.
Each participant received 1,000 Japanese Yen as reward.
2.2. Autonomous personal mobility vehicle
A robotic wheelchair WHILL Model CR with an autonomous driving system was used as the APMV. As shown in , the APMV was equipped with a LiDAR (Velodyne VLP-16) and a controlling PC allowing it to drive autonomously on a pre-designed route. In this experiment, its maximum speed was limited to 1 m/s for safety considerations.
Table 1. The basic design of APMV’s eHMI including LED lights and a display is used to show its driving behaviors.
2.3. The eHMI for visualizing APMV’s driving behaviors
Visualizing APMV’s driving behaviors can be considered a method of transforming implicit communication into explicit communication in pedestrian–APMV interactions. This approach represents a non-directed information provision method, making it easy for enabling multiple pedestrians around APMV to comprehend the driving behaviors of the APMV. To implement this visualization method on APMVs, as shown in , the APMV is equipped with a GUI to show the vehicle’s driving behaviors via two parts: LED lights on the sides and chassis, and a display above the bodywork.
According to the eHMI design concept in study (Li et al., Citation2021), the chassis is equipped with LED lights that show green to move, yellow to decelerate, and red to stop by projecting the respective colored lights onto the ground. In addition, the yellow LED lights on both sides of the APMV’s body and wheels are used as turn signals.
In addition, cartoonish eyes are shown in the display to mimic the observational behaviors of a human driver driving a vehicle. The eye color is designed to be cyan, showing the autonomous driving mode is ON. In particular, when turning, the eyes look in the corresponding direction. Also, the eyes show a relaxing gaze when stopping, a serious gaze when moving forward, and a concentrated gaze when slowing down.
2.4. Three eHMIs for communicating with pedestrians
In situations where the APMV interacts with specific pedestrians, both the directionality and content of information cues are crucial for their communication and negotiation. Therefore, in the design concept of our APMV’s eHMI, we convey the direction of information delivery through the gaze direction of the eyes on the eHMI screen and convey the content of the information using voice or text to ensure clear communication.
In this study, one GUI-based eHMI and two MUI-based eHMIs are designed for the APMV to communicate with a pedestrian while they encounter each other. In particular, they are (a) GUI-based eHMI with text messages (eHMI-T), (b) MUI-based eHMI with neutral voice (eHMI-NV), and (c) MUI-based eHMI with affective voice (eHMI-AV) as shown in . The eHMI-T uses a smiley face together with a piece of text to indicate its yielding intention, and uses grateful eyes together with a piece of text to express its appreciation, i.e., thanks. Both the eHMI-NV and eHMI-AV have the same GUI design for their eHMI as that for the eHMI-T, but they differ in their VUIs. To be more specific, the eHMI-NV utilizes a neutral voiceFootnote3 to say “After you” and “Thank you” to pedestrians, while the eHMI-AV utilizes an affective voice (see note 3) to express “Oh please, after you!” and “You are so kind. Thank you so much!”. Both of the voice cues are in Japanese as all participants are native Japanese speakers.
Table 2. Three types of eHMI designs to communicate with pedestrians.
Note that although the basic eHMI can be activated automatically by the APMV’s movements (see ), to ensure the performance for communication with pedestrians, a trained experimenter who followed the APMV remotely controlled the eHMI and activated the designated GUI and VUIs at a certain moment.
2.5. Driving scenarios
For our experimental site, we selected an indoor 55 m × 30 m area, as shown in . It is a walkway located on the ground floor of the Information Science Complex, at NAIST. An approximately 150 m long circular driving route has been established at the experimental site. This site was chosen based on a common usage scenario in which APMVs drove in a shared indoor area and frequently encountered pedestrians. Of these, four specific pedestrians in the interaction scenes were acted by trained actors. It should be noted that the APMV serves as the subject of communication with pedestrians. Therefore, pedestrians (actors) were instructed to face the APMV (i.e., the eyes on the eHMI display of the APMV) to communicate.
Figure 2. The experimental site is a 55 m × 30 m indoor area. In each round, the AVMP’s driving route (red line) encounters with pedestrians (actors) four times at the marked positions.
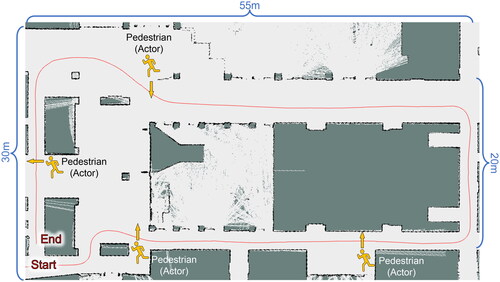
In this site, four similar encounter scenes have been designed along this route (see ). The specific interaction scenario at each scene is set up as an APMV and a pedestrian encounter at an intersection with a blind spot/corner. The APMV stops upon encountering the pedestrian, and likewise, the pedestrian stops synchronously as soon as he/she sees the APMV (see ). Then, the APMV first indicates its intention to yield to the pedestrian, communicated by the eHMI (see ). Afterwards, the pedestrian faces to the eye animation showing on the eHMI display and says “No, after you” to the APMV (see ). Finally, the APMV expresses its thanks to the pedestrian via the eHMI (see ) and then departs (see ). During the whole encounter scenes, passengers (participants) were asked to ride the APMV in a natural and relaxed way and they were not required to perform non-driving related tasks (NDRTs). Furthermore, there were no restrictions on passengers while they were communicating spontaneously with pedestrians.
2.6. Procedure
Firstly, participants were informed about the experiment content, i.e., as a passenger in the APMV to experience the communication between the APMV and pedestrians through different eHMI designs. Meanwhile, the three eHMI designs (see and ) were illustrated in detail through a demonstration. To alleviate any restlessness or nervousness among participants who are unfamiliar with the APMV, we provided an explanation of the principles behind the autonomous driving system and its sensors. Subsequently, we introduced the questionnaire (see Section 3.1) that participants would need to respond during the experiment. After signing the informed consent form, participants were instructed to complete a personality inventory before starting the experiment.
A schematic diagram of the experimental process is shown in . During the experiment, participants (passengers) sat on the APMV, which autonomously drove around the route (see ) for three times with a different eHMI in each round, respectively. It should be noted that the experience order of the three eHMIs and gender of participants were balanced (see ). Namely, there were six different orders to experience the three eHMIs, and each order was experimented with two participants. As there are four encounter scenes per round, each participant experienced a total of 12 encounter scenes while riding the APMV as a passenger. After each encounter scene, participants were required to complete a questionnaire (see Section 3.1) about their subjective feelings of the communication between the APMV (eHMI) and the pedestrians, as perceived from the passenger’s perspective. After each round (including four encounter scenes), participants were asked to complete the UE questionnaire (see Section 3.2) to assess their experience with the eHMI used. After all three rounds of experiencing all eHMIs, participants were asked to answer a final question “Which eHMI is your favorite?” and give a short open interview. In the open interview, passengers were free to share their experiential feelings about these three eHMIs and provide the reasons behind their responses.
Table 3. Order of eHMI experience for the 24 participants in the experiment.
3. Measurement
3.1. Subjective evaluation after each encounter scene
After each encounter scene, passengers were asked to answer five subjective questions about how they felt in the encounter scene using the five-point Likert scale, i.e., 1 = “strongly disagree,” 2 = “disagree,” 3 = “neutral,” 4 = “agree,” and 5 = “strongly agree.” The five questions were asked to be answered from a passenger’s view which are:
Q1: Was the information conveyed by eHMI insufficient for you?
Q2: Was the content of the communication between the eHMI and pedestrians easy to understand?
Q3: Did the APMV excessively communicate with pedestrians via the eHMI?
Q4: Did you feel awkward during the APMV–pedestrian communication?
Q5: Did you worry that the eHMI’s performance might attract too much attention from people around you?
Among them, Q1–Q3 are used to evaluate whether the information conveyed by eHMI can appropriately help passengers achieve situational awareness. Q4 and Q5 are used to evaluate the UE of passengers during the APMV–pedestrian communication.
3.2. User experience
The short version of the UE questionnaire (UEQ-S; Schrepp et al., Citation2017) in JapaneseFootnote4 was used to rate the passengers’ experience with each design of the eHMIs. As shown in , eight items in UEQ-S were analyzed in two UE domains, which were counted by:
3.3. Favorite eHMI
We analyzed the preference of the three eHMIs in relation to the participants’ personality domains. Specifically, the participants were divided into three groups based on their responses to the final question “Which eHMI is your favorite?” The mean of the five personality domains was compared across the participants in each group.
3.4. Big 5 personality domains
To find the relationship between the participants’ choice of the favorite eHMI and their personality inventory, the Japanese version (Oshio et al., Citation2012) of the Ten Item Personality Inventory (TIPI; Gosling et al., Citation2003) was used. As shown in , these ten items were rated by the participants using a seven-point scale.
Table 4. Items of TIPI (Gosling et al., Citation2003).
As outlined by Oshio et al. (Citation2012), the Big 5 personality domains were derived from the scores of TIPI items by aggregating the rating of the corresponding positive item and the residual of the negative item, as following:
Hence, the resulting rating for each personality domain is represented by a discrete value between 1 and 14.
4. Results
4.1. Subjective evaluations after each encounter scene
A summary of the answers to the five subjective evaluations after each encounter scene, i.e., Q1–Q5 described in Section 3.1, reported by the 24 participants is shown in . As shown in , a two-way mixed analysis of variance (ANOVA) revealed that there was no a statistically significant interaction between the effects of gender and eHMI on Q1–Q5, respectively. Additionally, it showed that a main effect of gender was significant on Q5 (p < .05) only, indicating that females were significantly more concerned about eHMI attracting too much attention from people around them than males. Moreover, the two-way mixed ANOVA also reported that main effects of eHMI were significant on Q1–Q5 (p < .001), respectively.
Figure 6. Subjective evaluation results of five questions after each encounter scene, where 1 = “strongly disagree,” 2 = “disagree,” 3 = “neutral,” 4 = “agree,” and 5 = “strongly agree.”.
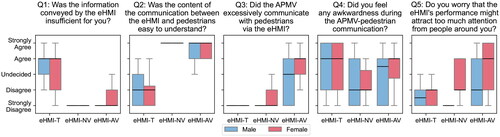
Table 5. Two-way mixed ANOVA for each subjective evaluation question.
Post hoc pairwise comparisons of eHMIs for Q1–Q5 using the Wilcoxon signed-rank test with BH-FDR correction are presented in . For Q1, passengers perceived that eHMI-T conveyed information significantly insufficient compared to eHMI-NV (p < .001) and eHMI-AV (p < .001). Similarly, from the results of Q2, passengers agreed that the communication contents were significantly easier to understand when using eHMI-NV (p < .001) and eHMI-AV (p < .001) compared to using eHMI-T. However, in Q3, passengers also significantly agreed that eHMI-AV exhibited excessive communication compared to eHMI-T (p < .001) and eHMI-NV (p < .001). The comparison of results from Q4 indicated that passengers felt significantly more discomfort when experiencing both eHMI-T (p < .01) and eHMI-AV (p < .01) compared to using eHMI-NV. Regarding Q5, passengers perceived that when experiencing eHMI-AV, they significantly worried about attracting too much attention from people compared to eHMI-T (p < .01) and eHMI-NV (p < .01).
Table 6. Post hoc two-sided pairwise comparisons using the Wilcoxon signed-rank test with Benjamini/Hochberg FDR correction for main effect of eHMI for each subjective evaluation question.
4.2. User experience
The results of the 24 participants answering the UEQ-S for three eHMIs were calculated into two UE domains, i.e., pragmatic quality and hedonic quality. Cronbach’s alpha in pragmatic quality items, i.e., UEQ item 1–4, is 0.78, and Cronbach’s alpha in hedonic quality items, i.e., UEQ item 5–8, is 0.92.
The evaluation results of UE for pragmatic quality and hedonic quality of three eHMIs by male and female participants are shown in . The average values of pragmatic quality and hedonic quality were calculated as the overall UE results, which are shown in . Background colors of each domain in represent the UE benchmarks obtained from a dataset including over 400 studies that used the UEQ to evaluate different products (Hinderks et al., Citation2018).
Figure 7. Results of UEQ-S for three types of eHMIs with the UEQ benchmark. Error bars show their confidence intervals.
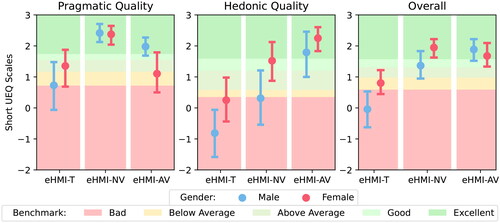
The two-way mixed ANOVA results in indicated significant effects of eHMI on various aspects of UE. Specifically, eHMI significantly influenced the pragmatic quality (p < .001), hedonic quality (p < .001), and the overall UE (p < .001). In terms of gender, the main effect was significant only on the hedonic quality (p < .05), indicating that the influence of eHMI on the hedonic quality of UE may also depending on the gender. The two-way mixed ANOVA further revealed a significant interaction between the effects of gender and eHMI on the pragmatic quality (p < .05) and the overall UE (p < .05). However, this interaction was not significant for the hedonic quality.
Table 7. Two-way mixed ANOVA for UE domains.
presents the simple main effects of gender within eHMIs on the pragmatic quality and overall UE by using two-sided t-tests with Holm correction. For all eHMIs, no significant differences were found in the pragmatic quality and overall UE due to gender differences.
Table 8. Simple main effects of gender within eHMIs by t-tests (two-sided) with Holm correction for UE domains.
presents the simple main effects of eHMIs within gender on the pragmatic quality and overall UE by using by two-sided paired t-tests with Holm correction. For the pragmatic quality, both females and males rated eHMI-NV significantly higher than eHMI-T (female: p < .05, male: p < .01), as well as eHMI-NV significantly higher than eHMI-AV (female: p < .05, male: p < .05). However, a gender difference was observed in the pragmatic quality of eHMI-AV and eHMI-T. Only males rated eHMI-AV significantly higher than eHMI-T (p < .05) in terms of pragmatic quality, while females did not perceive a significant difference between the two. In terms of overall UE, both males and females rated eHMI-T significantly lower than eHMI-NV (female: p < .001, male: p < .001) and eHMI-AV (female: p < .05, male: p < .001). However, the significant difference in overall UE between eHMI-NV and eHMI-AV was only observed in males (p < .05), not in females.
Table 9. Simple main effects of eHMIs within gender by paired t-tests (two-sided) with Holm correction for UE domains.
The post hoc pairwise comparisons for main effect of eHMIs, conducted using two-sided t-tests with Holm correction across different UE domains, are detailed in . In which, eHMI-NV significantly outperforms both eHMI-T (p < .001) and eHMI-AV (p < .001) in the pragmatic quality, but there is no significant difference between eHMI-T and eHMI-AV. For hedonic quality, eHMI-T significantly underperforms both eHMI-NV (p < .001) and eHMI-AV (p < .001), while eHMI-AV significantly outperforms eHMI-NV (p < .001). When considering the overall UE, taking into account both pragmatic quality and hedonic quality, eHMI-T significantly underperforms both eHMI-NV (p < .001) and eHMI-AV (p < .001). However, there is no significant difference between eHMI-NV and eHMI-AV.
Table 10. Post hoc pairwise comparisons by paired t-tests (two-sided) with Holm correction for UE domains.
4.3. Favorite eHMI
After experiencing all eHMIs, participants were asked to select the favorite eHMI. shows the number of female, male as well as all participants who selected each type of favorite eHMI. Fisher’s exact test (two-sided) was used to determine if there was a significant association between two genders and three favorite eHMIs. Its result indicated that there was not a statistically significant association between the favorite eHMIs and genders (p = 0.680).
4.4. Big 5 personality domains
The results of the Big 5 personality domains for 12 male and 12 female participants are shown in . For each personality domain, a t-test (two-sided) was used to test for differences between male and female. shows that there were no significant differences between males and females in all five personality domains.
Figure 9. Distribution of the Big 5 personality domains for male and female participants. Dots indicate mean scores and error bars indicate standard deviations.
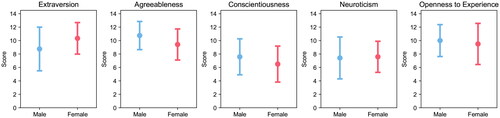
Table 11. The Big 5 personality domains of 12 male and 12 female participants.
4.5. Relation between the favorite eHMI and personality
In Sections 4.3 and 4.4, no significant differences were observed between males and females in their choice of favorite eHMI and their Big 5 personality domains. Therefore, in this section, the analysis of the relationship between participants’ choice of favorite eHMI and their personality domains did not take into account gender differences.
shows the differences of the Big 5 personality domains of the participants based on their favorite eHMI. In which, participants with lower scores in extraversion, openness to experience and conscientiousness tended to favor eHMI-T, while those with higher scores in those domains tended to prefer eHMI-AV.
Figure 10. Big 5 personality domains of the participants based on their favorite eHMI. The solid line represents the mean, while the dashed line represents the standard deviation.
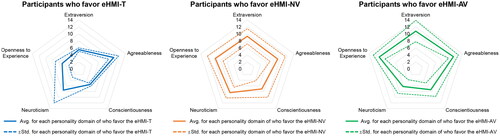
It is worth noting that although the average neuroticism of passengers who chose eHMI-T is between the average neuroticism of people who chose eHMI-AV and eHMI-NV, its standard deviation was large. This is primarily due to the small sample size of passengers who chose eHMI-T, specifically, one male and one female.
Compared to those who favored eHMI-AV, individuals who preferred eHMI-NV exhibited lower levels of extraversion, openness to experience, and agreeableness, while demonstrating a slightly higher degree of neuroticism. This suggests that different eHMIs may appeal to different personality types.
5. Discussion
5.1. UE of APMV passengers on the silent GUI-based eHMI
The subjective evaluation results of Q1 and Q2 in and show that, compared with the MUI-based eHMIs (i.e., eHMI-NV and eHMI-AV), the passengers got insufficient information from eHMI-T, which hindered their situation awareness and made them more difficult to understand the communication between APMV and pedestrians. In post-experiment interviews, participants made the same comments as “without the sound (i.e., eHMI-T), I don’t know what is being displayed. The pedestrians are talking by themselves and I don’t know what kind of communication they are having.”
Moreover, this lack of understand led passengers to feelings of awkwardness, as indicated in Q4. Also, from the interview, almost all passengers reported that the silence time when eHMI-T conveyed information to pedestrians was awkward. For example, “there was a long period of silence in the encounter, which caused a strong sense of awkwardness.” Moreover, some passengers also reported feeling “left out when eHMI-T and pedestrians communicated.”
Compared to other MUI-based eHMIs, the above issues resulted in significantly lower ratings of the eHMI-T’s pragmatic quality and hedonic quality of UE by both male and female passengers (see ). Particularly when compared to the benchmark in Hinderks et al. (Citation2018), the hedonic quality of the eHMI-T was already categorized in the bad range. This suggests that utilizing the voice cues may offer a more engaging and enjoyable experience for passengers compared to relying solely on a silent GUI-based eHMI.
According to the above research results, the answer to RQ 1 is that from the perspective of passengers, the silent GUI-based eHMI may not be suitable for APMV because of insufficient information, difficulties in understanding and the feeling of awkwardness during the APMV–pedestrian communications.
5.2. UE of APMV passengers on the MUI-based eHMIs
The findings from Q1 and Q2, illustrated in and , reveal that communication with pedestrians through two MUI-based eHMIs, specifically eHMI-NV and eHMI-AV, enabled the sharing of information with passengers via voice cues, thereby facilitating passengers’ understanding of the communication.
However, as shown in and , the results of Q3 and Q4 indicated that passengers felt the eHMI-AV communicated excessively compared to the eHMI-NV, leading to significant feeling of awkwardness for passengers experiencing the communication between eHMI-AV and pedestrians. From the interviews, we found that there are differences in the experience of male and female passengers with the eHMI-AV. Specifically, a few male passengers felt that the eHMI-AV gave too much information compared to the eHMI-NV, e. g., “I preferred eHMI-NV over eHMI-AV because it provided the minimum necessary communication.” However, half of the female passengers said that “the eHMI-AV was too casual, especially when the encountering pedestrian who was a stranger.”
The results from Q5, as shown in , indicated that passengers felt significantly more self-conscious about attracting attention from those around them when using the eHMI-AV compared to the eHMI-NV. Interestingly, revealed a gender disparity in the responses to Q5 for eHMI-NV. Specifically, male passengers were not concerned about eHMI-NV drawing too much attention from people around them, whereas female passengers expressed more concern about this issue. This suggests that gender may play a role in shaping passengers’ perceptions and experiences when using these interfaces. This finding can be explained by the conclusion in Turk et al. (Citation1998), which reported that in some public places, females were with significantly greater fear than males being the center of attention. Similarly, Zentner et al. (Citation2023) also found that females have higher levels of social anxiety than males.
The above conclusions can also be reflected in the UEQ results described in Section 4.2. The results presented in and suggest that eHMI-NV was found to have significantly better pragmatic quality than eHMI-AV. This is consistent with the results of Q3–Q5 in and , i.e., the eHMI-NV accomplishes negotiating with pedestrians in the most concise communication possible, without the perceived excessive communication that causes passengers to feel awkward, as is the case with the eHMI-AV. In the interview we got similar results, such as “I felt that they communicated better with voice than with text (eHMI-T), but I preferred eHMI-NV because it communicated the minimum required compared to eHMI-AV.”
However, from the perspective of hedonic quality, eHMI-AV is considered significantly better than eHMI-NV, as shown in and . In the interview, some passengers told that “I like the eHMI-AV better than the eHMI-NV because I feel that the eHMI-AV is more communicative,” as well as “I think the robot character (i.e., APMV’s eHMI) should have its own personality.” Above results are in line with (Lee et al., Citation2019), where the more anthropomorphic voice was favored and rated higher in trust, pleasure, and the sense of control than a voice-command interface. Besides, the speech embodiment of more conversational speech was evaluated as more warm and social presence (Wang et al., Citation2021).
Therefore, the answer to RQ 2 is that MUI-based eHMIs with voice cues, i.e., the eHMI-NV and eHMI-AV, both significantly enhance the passenger’s UE in APMV and pedestrian interactions. Moreover, it should be noted that each of these two MUI-based eHMIs has its own advantages, i.e., eHMI-NV has an advantage in pragmatic quality, while eHMI-AV has an advantage in hedonic quality. This difference in pragmatic and hedonic qualities between eHMI-NV and eHMI-AV aligns with the findings from a study on human-robot interaction by Ullrich (Citation2017). They reported that the neutral robot personality was rated best in a goal-oriented situation, while the positive robot personality was preferred in experience-oriented scenarios. Above results highlight the importance of considering both pragmatic and hedonic quality in the design of eHMIs, and the potential benefits of using voice cues to enhance passengers’ UEs.
5.3 eHMI designs for personality
To answer the RQ 3, the relationship between participants’ personalities and their favorite eHMI was discussed. As results disrupted in Section 4.5, this study found that individuals with different personality traits tend to prefer different types of eHMI. Specifically, those with lower scores in extraversion, openness to experience, and conscientiousness were more inclined to favor eHMI-T, while those with higher scores in these domains tended to prefer eHMI-AV. This result suggests a potential correlation between personality traits and the choice of eHMI, which could have implications for designing interfaces that cater to the preferences and needs of different personality types. This result aligns with the conclusion drawn in (Sarsam & Al-Samarraie, Citation2018; Tapus & Matarić, Citation2008). In interviews, 20 out of 24 passengers thought it was important that the eHMI was designed to fit their personality. A number of representative sentiments were reported, such as “If the APMV can reflect the passenger’s personality, that’s fine. But if it deviates from the passenger’s personality, I will feel strange”, “I think it’s better to match my personality because I feel like it is a part of me” as well as “I think this is very important because if our personalities don’t match well, I might feel it’s overly polite, or like my feelings aren’t being properly responded to.” Moreover, two passengers thought that matching personalities is important, but they have concerns, such as “As a user, I think it’s better if it matches but I don’t like attracting too much attention.” The remaining two passengers made it clear that they did not think the eHMI needed to be designed to fit their personalities, for example “I don’t think so much. I think a machine is a machine. I think APMV is a separate entity from me.” and “I prioritize efficiency, so I prefer not to have unnecessary communication.”
In summary, to answer the RQ 3, it may be beneficial to take into account the personality traits of different user groups to better meet their needs and preferences when designing eHMIs. For instance, simpler and more straightforward interfaces (such as eHMI-T) might be suitable for users who are more introverted and less adventurous, while more complex and diverse interfaces (such as eHMI-AV) could be provided for users who are more extroverted, open, and conscientious.
5.4. Limitations
The preference evaluation on eHMIs under different user scenarios may be different. In this study, we used a simple but common scenario found on campus for testing, which may not fully represent real-life situations, like crowded places with lots of pedestrians. Furthermore, for some quiet scenes, e. g., library and museum, the passengers’ UE of eHMI with voice information cues may be different from the results of this study.
Since one of the objectives of this study was to verify whether the silent eHMI-T could provide a good UE for a wide range of passengers, we did not balance personality differences between participants before the experiment. It led to a small number of people choosing eHMI-T and made the standard deviation of neuroticism for passengers who chose eHMI-T too large. In this regard, we will target introverted passengers in future works to verify if they prefer to use the silent eHMI-T.
Moreover, the participants in this experiment were young Japanese people in their twenties who tend to be more easily receptive to new things than the elderly. The results may not generalize to other age groups, e. g., elderly people, non-student groups or cultural backgrounds.
It should be noted that in this study, the observation of the gender on UE is exploratory, which gives the hints that it is worth further investigation by taking gender differences into consideration for the APMV’s eHMI design. However, in this study, the sample sizes of each gender are limited. To further verify the impact of gender differences on UE, larger sample sizes are needed in future work.
5.5. Future works
In our future work, we will explore specific design guidelines tailored to APMV passengers with different personalities and genders. Additionally, we plan to pre-screen participants using the questionnaire to identify subjects with significantly different trait levels. This approach will enable us to invite individuals with diverse personalities to help validate the proposed eHMI design guidelines. We are also planning to develop an eHMI of APMV which can automatically adjust the content of the information and the tone of voice based on the user’s personality traits and genders. We will also explore the effects of passengers performing NDRTs, such as reading a book, looking at a cell phone, etc., on UEs of the eHMI while riding the APMV.
6. Conclusion
In this article, we discussed three eHMI designs, i.e., GUI-based eHMI with a text message (eHMI-T), MUI-based eHMI-NV, and MUI-based eHMI-AV used for the APMV–pedestrian communication, from the perspective of APMV passengers.
The field study suggested that the silent GUI-based eHMI-T might not be the most suitable option for APMVs. This is because when the APMV communicated with a pedestrian, its passenger might encounter difficulties in comprehending the communication, leading to a sense of awkwardness due to the lack of information. In particular, participants reported the APMV–pedestrian communication was less comprehensive and sufficient, and they felt awkward during the “silent time,” due to the lack of information cues received as passengers.
We also found that the use of voice cues can improve passengers’ understanding of the communication between the APMV and pedestrian. This enhancement contributes to an overall improved UE of eHMIs. Specifically, eHMI-NV has an advantage in pragmatic quality, while eHMI-AV has an advantage in hedonic quality.
This study also highlights that the passengers’ personalities should be considered when designing eHMI for APMVs to enhance their UE. For instance, simpler and more straightforward interfaces, (such as eHMI-T), might be better suited for passengers with lower levels of extraversion and openness to experience. Conversely, interfaces with more personality and emotion, (such as eHMI-AV), could be offered to users with higher levels of extraversion, openness to experience, and agreeableness. In addition, the eHMI-NV is also advantageous for the masses, as the eHMI-NV has also been recognized by the majority of passengers as it balances adequate functionality with acceptable hedonic quality.
We have also observed that gender differences can influence specific aspects of UE for eHMIs, such as drawing excessive attention and hedonic quality. This demonstrates the necessity of considering passenger gender when designing APMV’s eHMI.
CRediT author statement
Hailong Liu: conceptualization, investigation, methodology, software, validation, formal analysis, project administration, funding acquisition, writing—original draft. Yang Li: investigation, methodology, writing—review and editing. Zhe Zeng: methodology, writing—review and editing. Hao Cheng: methodology, writing—review and editing. Chen Peng: methodology, writing—review and editing. Takahiro Wada: methodology, writing—review and editing.
Acknowledgments
The authors would like to thank all participants who took part in the experiment. The authors appreciate MiM Mrs. Yaxi Wu, who is the wife of Hailong Liu, for creating the illustration of , free of charge.
Disclosure statement
The authors declare that the research was conducted in the absence of any commercial or financial relationships that could be construed as a potential conflict of interest.
Additional information
Funding
Notes on contributors
Hailong Liu
Hailong Liu is an assistant professor at Graduate School of Science and Technology, NAIST, Japan. He acquired BS (2013), MS (2015) and PhD (2018) in Engineering from Ritsumeikan University, Japan. He was a Research Fellowship for Young Scientists (DC2) of JSPS (2016–2018), and a researcher at Nagoya University (2018–2021).
Yang Li
Yang Li is a PhD candidate at KIT, Germany, specializes in the human–machine interface for communication between AVs and human traffic partners on ambiguous roads. With a 12-month visiting study at the University of Leeds and participation in the Hi-Drive Program, she also visited NAIST in 2023.
Zhe Zeng
Zhe Zeng is a postdoctoral researcher at the University of Ulm, Germany. She received her MSc degree in 2017 and PhD degree in 2022 from the Technical University of Berlin, Germany. Her research interests include eye tracking, natural gaze interaction, and human–robot interaction.
Hao Cheng
Hao Cheng is a postdoctoral researcher at the University of Twente, The Netherlands. He acquired MSc in 2017 and PhD in 2021 degrees in informatics at Leibniz University Hannover, Germany. His research focuses on exploring deep learning in modeling interactions between vehicles and VRUs to facilitate safer autonomous driving.
Chen Peng
Chen Peng is a research fellow and a PhD candidate at the Institute for Transport Studies, University of Leeds. She received her Master’s degree in Human Technology Interaction from the Eindhoven University of Technology in 2019. Her research interests include user comfort in automated driving, human–computer interaction, and aging.
Takahiro Wada
Takahiro Wada is a Full Professor at NAIST, Japan, since 2021. He obtained his PhD in Robotics from Ritsumeikan University, Japan, in 1999, and served as an Assistant and Associate Professor at Kagawa University for 12 years. He became a Full Professor at Ritsumeikan University in 2012.
Notes
1 WHILL Autonomous developed by WHILL Inc.: https://youtu.be/vJWhwNnUPRs
2 RakuRo developed by ZMP Inc.: https://youtu.be/lWhbJ0rBwjM
3 The voice is generated by Microsoft Nanami Online. The voice pitch was set to −10% for the neutral voice and +15% for the affective voice. The voices of eHMI-NV and eHMI-AV can be found at https://1drv.ms/f/s!AqdIEHyOvvX56E_YDn2VRzSeU1KK
4 The Japanese UEQ-S and the data analysis tool download from https://www.ueq-online.org
References
- Ahn, S., Lim, D., & Kim, B. (2021, July 24–29). Comparative study on differences in user reaction by visual and auditory signals for multimodal eHMI design. In HCI International 2021-Posters: 23rd HCI International Conference, HCII 2021, Virtual Event, Proceedings, Part III 23 (pp. 217–223).
- Ali, S., Lam, A., Fukuda, H., Kobayashi, Y., & Kuno, Y. (2019). Smart wheelchair maneuvering among people. In International Conference on Intelligent Computing (pp. 32–42).
- Bazilinskyy, P., Kooijman, L., Dodou, D., & De Winter, J. (2021). How should external human–machine interfaces behave? Examining the effects of colour, position, message, activation distance, vehicle yielding, and visual distraction among 1,434 participants. Applied Ergonomics, 95, 103450. https://doi.org/10.1016/j.apergo.2021.103450
- Brill, S., Payre, W., Debnath, A., Horan, B., & Birrell, S. (2023). External human–machine interfaces for automated vehicles in shared spaces: A review of the human–computer interaction literature. Sensors, 23(9), 4454. https://doi.org/10.3390/s23094454
- Chang, C.-M. (2020). A gender study of communication interfaces between an autonomous car and a pedestrian [Paper presentation]. 12th International Conference on Automotive User Interfaces and Interactive Vehicular Applications (pp. 42–45). https://doi.org/10.1145/3409251.3411719
- Dey, D., van Vastenhoven, A., Cuijpers, R. H., Martens, M., & Pfleging, B. (2021). Towards scalable ehmis: Designing for AV-VRU communication beyond one pedestrian [Paper presentation]. 13th International Conference on Automotive User Interfaces and Interactive Vehicular Applications (pp. 274–286). https://doi.org/10.1145/3409118.3475129
- Dou, J., Chen, S., Tang, Z., Xu, C., & Xue, C. (2021). Evaluation of multimodal external human–machine interface for driverless vehicles in virtual reality. Symmetry, 13(4), 687. https://doi.org/10.3390/sym13040687
- Gosling, S. D., Rentfrow, P. J., & Swann, W. B. Jr. (2003). A very brief measure of the big-five personality domains. Journal of Research in Personality, 37(6), 504–528. https://doi.org/10.1016/S0092-6566(03)00046-1
- Haimerl, M., Colley, M., & Riener, A. (2022). Evaluation of common external communication concepts of automated vehicles for people with intellectual disabilities. Proceedings of the ACM on Human-Computer Interaction, 6(MHCI), 1–19. https://doi.org/10.1145/3546717
- Hinderks, A., Schrepp, M., & Thomaschewski, J. (2018). A benchmark for the short version of the user experience questionnaire. In WEBIST (pp. 373–377).
- Kobayashi, Y., Suzuki, R., Sato, Y., Arai, M., Kuno, Y., Yamazaki, A., & Yamazaki, K. (2013). Robotic wheelchair easy to move and communicate with companions [Paper presentation]. Chi ’13 Extended Abstracts on Human Factors in Computing Systems, New York, NY, USA (pp. 3079–3082). Association for Computing Machinery. https://doi.org/10.1145/2468356.2479615
- Kreißig, I., Morgenstern, T., & Krems, J. (2023). Blinking, beeping or just driving? Investigating different communication concepts for an autonomously parking e-cargo bike from a user perspective. Human Interaction and Emerging Technologies (IHIET-AI 2023): Artificial Intelligence and Future Applications, 70(70). http://doi.org/10.54941/ahfe1002930
- Lee, S. C., Sanghavi, H., Ko, S., & Jeon, M. (2019). Autonomous driving with an agent: Speech style and embodiment. In Proceedings of the 11th International Conference on Automotive User Interfaces and Interactive Vehicular Applications: Adjunct Proceedings (pp. 209–214).
- Li, Y., Cheng, H., Zeng, Z., Deml, B., & Liu, H. (2023). An AV-MV negotiation method based on synchronous prompt information on a multi-vehicle bottleneck road. Transportation Research Interdisciplinary Perspectives, 20, 100845. https://doi.org/10.1016/j.trip.2023.100845
- Li, Y., Cheng, H., Zeng, Z., Liu, H., & Sester, M. (2021). Autonomous vehicles drive into shared spaces: eHMI design concept focusing on vulnerable road users [Paper presentation]. 2021 IEEE International Intelligent Transportation Systems Conference (ITSC) (pp. 1729–1736). https://doi.org/10.1109/ITSC48978.2021.9564515
- Liu, H., Hirayama, T., Morales, L. Y., & Murase, H. (2020). What timing for an automated vehicle to make pedestrians understand its driving intentions for improving their perception of safety? In 2020 IEEE 23rd International Conference on Intelligent Transportation Systems (ITSC) (pp. 1–6).
- Liu, H., Hirayama, T., Morales, L. Y., & Murase, H. (2023). Implicit interaction with an autonomous personal mobility vehicle: Relations of pedestrians’ gaze behavior with situation awareness and perceived risks. International Journal of Human–Computer Interaction, 39(10), 2016–2032. https://doi.org/10.1080/10447318.2022.2073006
- Liu, H., Hirayama, T., & Watanabe, M. (2021). Importance of instruction for pedestrian-automated driving vehicle interaction with an external human machine interface: Effects on pedestrians’ situation awareness, trust, perceived risks and decision making [Paper presentation]. 2021 IEEE Intelligent Vehicles Symposium (IV) (pp. 748–754). https://doi.org/10.1109/IV48863.2021.9575246
- Oshio, A., Abe, S., & Cutrone, P. (2012). Development, reliability, and validity of the Japanese version of ten item personality inventory (TIPI-J). The Japanese Journal of Personality, 21(1), 40–52. https://doi.org/10.2132/personality.21.40
- Sarsam, S. M., & Al-Samarraie, H. (2018). Towards incorporating personality into the design of an interface: A method for facilitating users’ interaction with the display. User Modeling and User-Adapted Interaction, 28(1), 75–96. https://doi.org/10.1007/s11257-018-9201-1
- Schrepp, M., Hinderks, A., & Thomaschewski, J. (2017). Design and evaluation of a short version of the user experience questionnaire (UEQ-S). International Journal of Interactive Multimedia and Artificial Intelligence, 4(6), 103–108.
- Sodnik, J., Dicke, C., Tomažič, S., & Billinghurst, M. (2008). A user study of auditory versus visual interfaces for use while driving. International Journal of Human-Computer Studies, 66(5), 318–332. https://doi.org/10.1016/j.ijhcs.2007.11.001
- Tapus, A., Matarić, M. J. (2008). User personality matching with a hands-off robot for post-stroke rehabilitation therapy. In Experimental Robotics: The 10th International Symposium on Experimental Robotics (pp. 165–175).
- Turk, C. L., Heimberg, R. G., Orsillo, S. M., Holt, C. S., Gitow, A., Street, L. L., Schneier, F. R., & Liebowitz, M. R. (1998). An investigation of gender differences in social phobia. Journal of Anxiety Disorders, 12(3), 209–223. https://doi.org/10.1016/s0887-6185(98)00010-3
- Ullrich, D. (2017). Robot personality insights. Designing suitable robot personalities for different domains. i-com, 16(1), 57–67. https://doi.org/10.1515/icom-2017-0003
- Wang, M., Lee, S. C., Kamalesh Sanghavi, H., Eskew, M., Zhou, B., & Jeon, M. (2021). In-vehicle intelligent agents in fully autonomous driving: The effects of speech style and embodiment together and separately [Paper presentation]. 13th International Conference on Automotive User Interfaces and Interactive Vehicular Applications (pp. 247–254). https://doi.org/10.1145/3409118.3475142
- Watanabe, A., Ikeda, T., Morales, Y., Shinozawa, K., Miyashita, T., & Hagita, N. (2015). Communicating robotic navigational intentions [Paper presentation]. 2015 IEEE/RSJ International Conference on Intelligent Robots and Systems (IROS) (pp. 5763–5769). https://doi.org/10.1109/IROS.2015.7354195
- Zentner, K. E., Lee, H., Dueck, B. S., & Masuda, T. (2023). Cultural and gender differences in social anxiety: The mediating role of self-construals and gender role identification. Current Psychology, 42(25), 21363–21374. https://doi.org/10.1007/s12144-022-03116-9
- Zhang, B., Barbareschi, G., Ramirez Herrera, R., Carlson, T., & Holloway, C. (2022). Understanding interactions for smart wheelchair navigation in crowds [Paper presentation]. In Proceedings of the 2022 Chi Conference on Human Factors in Computing Systems (pp. 1–16). https://doi.org/10.1145/3491102.3502085