Abstract
Trust is critical in humans’ interactions with artificial intelligence (AI). In this large-scale survey study (N = 2187), we examined the effects of 19 factors on people’s trust in AI. Across the three dimensions of trust (i.e., the trustor, the trustee, and their interacting context), factors related to the trustee (i.e., AI) were found to affect trust in AI the most. Among factors in the trustee category, warmth and competence, two factors also critical to human trust relationships, emerged as the most pivotal ones, and they partially mediated the effects of other factors on trust in AI. We further show that trust in AI influenced participants’ intentions to collaborate and use AI both directly and as the mediator between warmth, competence, and other factors and intentions. These findings indicate that trust in AI shares much common ground with trust in humans, and provide practical suggestions on how to promote people’s trust in AI effectively.
1. Introduction
When operating a vehicle on the road, drivers typically demonstrate a remarkable degree of trust toward other drivers, even though most of them are total strangers. While humans are vulnerable to the devastating consequences of car crashes, trust in the driving ability and intentions of others remains a foundation for road safety. But what if the vehicles on the other side of the road are fully controlled by artificial intelligence (AI)? Do people still trust these “drivers” as much?
Benefiting from developments in computer hardware, network technology, big data, and machine learning algorithms, AI technology has made tremendous progress since the turn of this century. It is now a driving force for socioeconomic development and considered a cornerstone of the Fourth Industrial Revolution. That said, the use of AI also raises serious concerns about safety, ethics, and accountability (Bigman & Gray, Citation2018; Lee et al., Citation2015), and the long-term impact of AI on human society is still a subject of much debate (Ozmen Garibay et al., Citation2023). Moreover, as AI technology grows in complexity and scale, the issue of trust has also become more prominent.
Trust is the foundation for collaboration. The target of trust can be individuals, groups, organizations, or technologies. When people are uncertain about the reliability and capability of a new technology, they tend to trust the technology less and are less interested in using it than well-established technologies (Jian et al., Citation2000). Theories such as the unified theory of acceptance and use of technology have been proposed to explain the determinants and process of technology acceptance (Venkatesh et al., Citation2012), and the role of trust has been increasingly emphasized in this line of research (Zhang et al., Citation2022).
The key difference between AI and other types of technology lies in the high levels of autonomy of AI; that is, AI can produce optimized solutions as the application scenarios change, such as making moves in a chess game and turns in an autonomous driving vehicle, with little instruction and intervention from humans (Shneiderman, Citation2020). This property gives AI a humanlike characteristic and leads to a particularly intricate relationship between AI and humans. In dealing and collaborating with such a unique and sophisticated technology, users must establish appropriate levels of trust in AI, not only to fully harness AI’s power but also to avoid potential harms it may cause. Therefore, it is crucial to understand the key factors that influence the formation and maintenance of people’s trust in AI, the possible interactions among these factors, and their downstream effects on the acceptance of AI.
1.1. A three-dimension view of trust in AI
In a comprehensive review of trust in automation, Lee and See (Citation2004) defined trust as “the attitude that an agent will help achieve an individual’s goals in a situation characterized by uncertainty and vulnerability” (p. 51). The agent can be human or an automated machine and system, such as AI. Kaplan et al. (Citation2021) later proposed a conceptual framework to organize and distinguish factors that may affect people’s trust in AI. The framework classifies the factors into three categories or dimensions: those related to the trustor (i.e., the person or entity placing trust in AI), to the trustee (i.e., the AI technology or system being trusted), and to the context in which the trustor and the trustee interact (i.e., the task environment under which the trust relationship takes place). Factors in these dimensions interact with each other and together determine the degree of trust.
We adopted this framework in our search for and organization of potentially relevant factors. Besides its fit to the three-dimension framework, each factor had to be measurable by a scale with good psychometric properties. After an extensive search, we ended up with 19 factors. shows the factors and the source study in which the underlying construct of each was defined. There are six, eight, and five factors in the trustor, trustee, and context dimensions, respectively.
Table 1. Factors potentially related to trust in artificial intelligence (AI).
In previous studies, these factors were examined either in isolation or in small groups, and their relationship with trust in AI was not always the focus of investigation. Thus, the relative impact of each factor, as well as each dimension, on trust in AI and the possible interactions among these factors are unclear. One goal of the present study was to fill this gap. Among the three dimensions, factors related to the trustee—in our case, AI—have attracted particular attention, partly because the technology of AI had been developing rapidly, constantly changing people’s perceptions of what it is and its trustworthiness.
1.2. Trustworthiness of AI: The prominent roles of warmth and competence
Trust and trustworthiness are related but distinct concepts. Trust is a trustor’s attitude toward a trustee in terms of the trustee’s ability and intention to help. Trustworthiness, on the other hand, is a characteristic of the trustee and represents the degree to which the trustee is perceived as reliable and capable of being trusted. Individuals generally trust a trustworthy agent, and an agent’s trustworthiness can inspire trust (Flores & Solomon, Citation1998). Thus, trustworthiness plays a crucial role in evaluating and predicting trust levels (Colquitt et al., Citation2007). As with many psychological constructs, the assessment of trustworthiness is a cognitive process that involves the consideration of multiple factors. What, then, are the factors that contribute the most to making an agent trustworthy?
Because trustworthiness is typically understood as a social perception (Kong, Citation2018; Ye et al., Citation2020), research on trust in AI often looks at trust from a sociocognitive perspective, with the stereotype content model (SCM) being a key theory. According to the SCM (Fiske et al., Citation2002), individuals’ perceptions of other individuals can be organized along two main dimensions: warmth and competence. Warmth reflects the perceived (good or bad) intentions of others, and competence represents the judgment of how capable others will be in carrying out those intentions. These two dimensions have been demonstrated to play a significant role in how individuals evaluate and behave toward members of different groups, and this extends to the evaluation of and behavior toward AI systems as well (Frischknecht, Citation2021).
For example, Kulms and Kopp (Citation2018) showed that when playing with computer agents in a collaborative game, participants first tried to infer these agents’ warmth and competence and then decided how much trust they would place in these agents. In a study examining consumers’ responses to human and robotic service workers, Frank and Otterbring (Citation2023) found that consumers’ loyalty level (e.g., making a future purchase) increased after receiving an acknowledgement, and the effect was mediated by their perceived warmth and competence levels of the workers. These results suggest that warmth and competence might be two key factors determining humans’ perceptions of AI and its trustworthiness.
Mayer et al. (Citation1995) also proposed an integrative model that considers ability, benevolence, and integrity as three key trustworthiness factors. Ability pertains to the trustee’s competence and skill in carrying out specific tasks; benevolence is the extent to which the trustee is believed to have the trustor’s best interests at heart; and integrity is the trustor’s perception that the trustee adheres to a set of principles acceptable to the trustor. Ability and competence correspond well to competence and warmth in the SCM, making them central aspects to consider in judging the trustworthiness of AI. Integrity, on the other hand, covers some important ethical issues in the applications of AI.
Ethical principles are often established and regulated by national or international bodies, which provide guidelines and standards that help ensure AI systems are designed, deployed, and used in a manner that respects privacy and promotes fairness, transparency, and accountability (AI HLEG, Citation2019). When AI developers adhere to these principles, their values can be aligned with ethical frameworks and societal values, fostering trust among users and stakeholders. Although ethical principles have been widely discussed, there is limited research on people’s perception of how well these principles are implemented, as well as their impacts on trust in AI. Specifically, among the trustee factors that also include warmth and competence, it is unclear just how important the factors pertaining to ethics and integrity—such as accountability, fairness, privacy protection, and transparency examined in the present study—are in determining people’s trust in AI. The same question applies to the two other trustee factors we examined, anthropomorphism and robustness, as well.
1.3. Factor interactions
There are a large number of possible interactions among the 19 factors included in the present study. To narrow down the scope, we focused on those in the trustee dimension that lead to the judgment of AI’s trustworthiness. Kulms and Kopp (Citation2018) suggested that the perception of warmth could moderate the effect of competence on trust in a computer agent. Specifically, even if an agent is highly competent, a lower perceived warmth can diminish participants’ trust in it; and conversely, an agent perceived as warm may be entrusted more compared to a less warm one with the same competence. Whether such an interaction also holds for AI technology needs to be examined.
Moreover, data and algorithms serve as the foundations for AI, but they also give rise to two major issues: privacy infringement and the “black box” problem (Li et al., Citation2022; Santoni de Sio & Mecacci, Citation2021). The latter is a metaphor for the lack of transparency of AI’s operational process and the accountability for its outcomes (Shin & Park, Citation2019). To develop trustworthy AI, various laws and regulations have been introduced. For instance, the General Data Protection Regulation enforced by the European Union in 2018 established stringent privacy protection policies and outlined clear principles of algorithm transparency and accountability (Felzmann et al., Citation2019).
The three factors investigated in our study, namely, privacy protection, transparency, and accountability, relate directly to these concerns. As discussed above, they appear to tap into the integrity aspect of AI’s trustworthiness and can therefore affect trust in AI directly. Their effects may additionally take place through the other two trustee factors: warmth and competence. Warmth, according to its definition (e.g., Fiske et al., Citation2002; Harris-Watson et al., Citation2023), is the perceived intention of an agent to behave in a way that promotes the trustor’s interests. Being transparent, accountable, and protective of privacy should correlate positively with such an intention, and high levels of these characteristics could thus increase trust in AI via their effects on enhanced perception of warmth. Following the same line of reasoning, competence may mediate the effects of these three factors as well.
Previous studies that examined the joint effects of multiple trustee factors support the possibility of these mediation effects (e.g., Christoforakos et al., Citation2021; Gefen & Straub, Citation2004; Ye et al., Citation2020). One possible reason is that the effects of warmth and competence on trust might be so dominant that other factors’ effects on trust are likely to go through them. We sought to determine if this is indeed the case for trust in AI.
1.4. From trust to behavioral intention
Behavioral intention plays a pivotal role in shaping actual behavior toward specific targets, and a positive perception of quality can predict favorable behavioral intentions, such as adopting technology (Choung et al., Citation2023), purchasing products (Rheu et al., Citation2021), and engaging in collaboration (Leeman & Reynolds, Citation2012). According to the theory of reasoned action (Ajzen & Fishbein, Citation2000), behavior is primarily influenced by behavioral intention, which is in turn mainly determined by attitudes and subjective norms (i.e., permissions and expectations of others). In the context of AI, because trust reflects one’s attitude toward AI (Schepman & Rodway, Citation2023), it is reasonable to expect a positive relationship between trust in AI and one’s intention to use it. Previous research has demonstrated supportive evidence for this relationship (Chao, Citation2019; Khalilzadeh et al., Citation2017; Zhang et al., Citation2022).
Previous research has also shown that perceptions of warmth and competence, two key factors in the judgment of an agent’s trustworthiness, can affect behavioral intentions as well (Cuddy et al., Citation2008; Simon et al., Citation2020). Therefore, there may be two ways by which trust influences behavioral intention in the context of AI: one direct and the other with warmth and competence as the antecedents; that is, trust mediates the effects of warmth, competence, and possibly other factors on behavioral intention.
Among the many behaviors directed toward AI, we focused on two that have generated much interest recently. The first is users’ intention to share private data with AI systems. Many AI products work by gathering and making use of users’ personal information. How product providers should handle and protect such data had been a major issue of discussion (Santoni de Sio & Mecacci, Citation2021). Similarly to when they converse with a confidant, users need to have enough trust in AI to agree to share their data with it. The second relates to the use of AI in the workplace, especially for human resources management (HRM). With the increasing application of AI by organizations, there has been much debate on issues such as bias and accountability in management research (Canals & Heukamp, Citation2020; Hofeditz et al., Citation2023). HRM decisions have widespread effects on employees, and employees’ trust in AI should be important in determining how much they intend to support the use of AI in HRM.
1.5. The present study
Conducting a cross-sectional survey of a representative adult sample in China, the present study was centered on three main research questions (RQ) aimed at identifying what factors are crucial in determining people’s trust in AI, the dynamics among these factors, and the joint effects of these factors and trust on people’s intention to use AI.
RQ1: What is the relative importance of each dimension of trust (i.e., trustor, trustee, and context), as well as the associated factors within a dimension, in determining people’s trust in AI?
RQ2: How do factors influencing trust in AI, such as warmth, competence, accountability, and privacy protection interact with each other in the formation of trust in AI?
RQ3: What is the role of trust in people’s intention to use AI besides the possible positive direct association between the two?
2. Methods
We conducted a cross-sectional survey of a representative sample of the Chinese adult population age 18–60 years. In the survey, participants were asked to complete 22 scales that measured their trust in AI, the 19 factors potentially related to trust in AI (), their intention to share private data with AI, and their support for using AI in HRM. Survey studies on trust or other issues of AI seldom use representative samples, and to our knowledge, our study covers the largest number of factors related to trust in AI so far.
2.1. Participants
Participants were recruited with the assistance of a professional survey company that had contact information of a large pool of Chinese participants. Probability sampling across four major economic regions of Mainland China—Northeastern, Eastern, Central, and Western—was used in the recruitment process. The sample size for each region was determined according to the proportion of its population size in Mainland China for adults. Within each region, we further applied stratified sampling based on age, which was divided into three groups: 18–29, 30–39, and 40–60 years, and sex to obtain a subsample with distributions of these two attributes matching those of the population in the region.
We aimed to collect data from 2000 participants in total. In the end, 2456 participants completed the survey through an Internet platform between September 9 and October 13 2023. Three exclusion criteria were applied for data quality control: duplicate IP addresses, mismatches between reported age or sex with information verified by the survey company, and incorrect responses on the attention check items in the survey. After these controls, 2187 participants were retained in the final sample (Mage = 36.5 years, SD = 10.3; 1011 women). shows key demographic statistics of the final sample, and supplemental Figure S1 reports the numbers of actual and anticipated participants in each stratified subsample. In general, although the proportion of men in the 40–60 years group was higher than anticipated in some regions, the overall age and sex distributions in the entire sample aligned closely with population statistics.
Table 2. Key demographic characteristics of participants.
2.2. Measures and procedure
Guided by the three-dimension framework, we conducted an extensive search of the literature in multiple databases, including Web of Science, PubMed, and PsycInfo, for factors potentially related to trust in AI and identified 19 factors (see ). Scales with good psychometric properties could be found for the measurement of most factors. However, there were some scales with items not designed with AI as the target, and some with too many items or items not suitable for the current development of AI in the Chinese context. For these scales, we either modified the items for the purpose of the present study or came up with new items based on relevant existing scales. A pilot study (N = 193) was run to pre-examine the qualities of all scales and to refine the items used. Supplemental Table S1 shows the items included in the final scale for the measurement of each factor.
Trust in AI is the central construct examined in the present study. It was measured with an eight-item scale that included items such as “I am willing to allow AI to make all decisions” and “I think AI is more trustworthy than humans.” The scale was designed to measure participants’ general trust in AI instead of trust in a specific AI system, function, or product, and aimed to provide a broad understanding of trust in AI across various contexts and a general depiction of the relationships between trust in AI and factors of the three trust dimensions. The items were adapted from relevant previous studies (Calhoun et al., Citation2019; Merritt, Citation2011; Sundar & Kim, Citation2019). Intention to share private data with AI systems and support for using AI in HRM were each measured with a four-item scale adapted from relevant studies (e.g., AI HLEG, Citation2019; Lockey et al., Citation2020). The former included questions such as “How willing are you to share sensitive information with an application that uses AI?” and the latter asked about degrees of support for using AI to make hiring, performance evaluation, and firing decisions, as well as to monitor employees. The specific items in these three scales can be found in Supplemental Table S1.
In addition to completing the scales, participants were asked to report their age, sex, education level, employment status (i.e., currently employed or not), and subjective social status. Subjective social status has been shown to correlate with a host of important indicators and constructs, such as health, well-being, and risk attitude (Zell et al., Citation2018), and consistent with previous research, we measured it with a 10-rung ladder in which the top rung represents the highest level of social status perceived by participants.
After signing up for the study, participants were directed to an online platform where they gave their informed consent and then were given a brief introduction to the concept of AI and application examples of AI, before answering the questions in the survey. It took on average around 20 min to complete the survey, and each participant received 15 RMB (approximately 2 U.S. dollars) for their work. The study was approved by the Ethics Committee of the Institute of Psychology, Chinese Academy of Sciences.
3. Results
3.1. Measurement scores, reliability, and validity
shows the means and standard deviations of all 22 key measures examined in the present study. Scores of each measure ranged between 1 (the lowest) and 5 (the highest), and the score distributions of all measures can be found in Supplemental Figure S2. Among the measures, the perceived degree of privacy protection, the rated anthropomorphism—that is, the degree to which AI resembles humans—and support for using AI in HRM were particularly low, with an average score of 2.117, 2.582, and 2.899, respectively. On the other end, the perceived benefits and uncertainties of AI had the highest scores of 4.214 and 4.155, respectively. The (general) trust in AI, meanwhile, had an average score of 3.153, suggesting that participants in our sample were generally ambivalent about whether they should trust AI.
Table 3. Descriptive statistics and reliability and validity indices of 22 key measures.
It was necessary to check the possible common method bias among the measures, because all were based on self-report scales. A Harman single-factor test shows that the first factor extracted from an exploratory factor analysis accounted for 28.9% of the total variance, which is below the critical threshold of 50% (Podsakoff et al., Citation2003) and suggests that our results were unlikely subject to common method bias. For each measure, we also assessed the composite reliability and Cronbach’s alpha to determine its reliability, and both exceeded the acceptable levels of 0.70 (). We further calculated the average variance extracted (AVE) of each measure to evaluate its convergent and discriminant validities. Specifically, the AVE of each measure was above 0.50, indicating an acceptable level of convergent validity. Discriminant validity was evaluated with the Fornell-Larcker criterion, which stipulates that the square root of the AVE of a measure should exceed its correlations with all other administered measures to demonstrate discriminant validity; this criterion was met for each measure (see Supplemental Table S2). In general, results of these analyses indicate that our scales were reliable and valid measures of the intended underlying constructs.
3.2. Trust in AI by participant group
There was a sizeable variance in trust in AI across participants (SD = 0.687). shows the average levels of trust in different participant groups. First, with respect to age, trust levels were higher for participants in older age groups, F(2, 2184) = 84.554, p < 0.001, partial η2 = 0.072. Post hoc comparisons show significant differences between each pair of age groups, ps < .001 in all three comparisons. Second, for subjective social status, we reduced the originally reported 10 levels to two: relatively high (> 5) and relatively low (≤ 5). The high-status group had a significantly higher level of trust in AI than the low-status group, t(2185) = 6.036, p < 0.001, Cohen’s d = 0.261.
Figure 1. Trust in AI by participant group. (A) by three age groups, (B) by two levels of subjective social status, (C) by two levels of education, (D) by sex, and (E) by four economic regions in China. Error bars indicate one standard deviation.
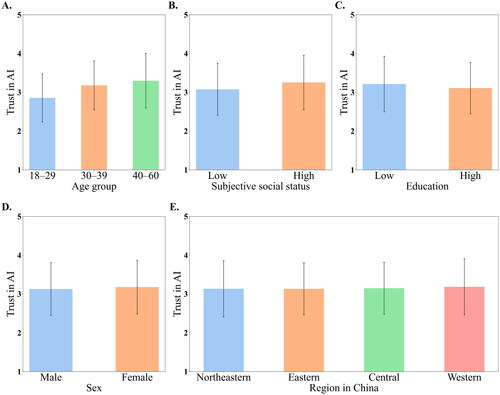
Third, similar to subjective social status, participants’ education levels were reduced to two broad categories: high (those with a bachelor’s degree or higher) and low (all others). The trust level of the more highly educated participants was significantly lower, t(2185) = 3.468, p = .001, Cohen’s d = 0.151. Last, there was no significant difference between female and male participants, t(2,185) = 1.680, p = .093, Cohen’s d = 0.072, or across the four economic regions, F(3, 2,183) = 0.680, p = .564, partial η2 = 0.001. In general, our results show that compared to other participants, those who were older, who perceived themselves as relatively high in social status, and who did not have a postsecondary degree tended to have a higher level of trust in AI.
3.3. The determinants of trust in AI
One main question we aimed to address in this study is which factor or factors—as well as which dimension in the three-dimension framework of trust—have the most impact on trust in AI. To reduce the possible confounding effects of irrelevant variables, we followed a method applied in previous research (Frey et al., Citation2017), first running a linear regression model with age, sex, region, subjective social status, and education as the predictors and trust in AI as the predicted variable and then using the residuals as the input data for the ensuing correlation and other analyses. In essence, results out of these analyses were the results after controlling for the five demographic and individual variables. We also conducted the analyses with the original data. The results aligned well with the ones using the residuals and led to the same conclusions.
shows the correlation matrix of the 22 key variables measured in the present study. All 19 factors were significantly correlated with trust in AI, ps < 0.001, but the strength of association varied considerably across the factors. Warmth (r = 0.63) and competence (r = 0.60), two trustee-related factors, emerged as the factors most highly correlated with trust in AI, whereas uncertainties about AI (r = −0.16), a context-related factor, and trust propensity (r = 0.28), a trustor-related factor, had the lowest correlations. The pairwise correlations among the 19 factors also varied substantially. Among them, the correlation between warmth and competence was relatively high (r = 0.58), consistent with findings from a large-scale study on stereotypes of social groups conducted in Mainland China (Ji et al., Citation2021).
Table 4. Correlation coefficients among the 22 key variables.
Next, we ran a series of regression models to examine how well all factors together and by each trust dimension could explain trust in AI. Because there were a large number of factors and many of them were correlated with other factors, we calculated the variance inflation factor (VIF) of each factor to test degree of collinearity. The results are reported in Supplemental Table S2, which shows that the VIF values ranged between 1.18 (anthropomorphism) and 3.04 (emotional experience), indicating that collinearity was not an issue among the factor variables (James et al., Citation2023).
For the regressions, the model with all 19 factors could account for 56.6% of the variance in trust in AI. Factors in the trustor, trustee, and context dimensions could account for 40.3%, 53.6%, and 41.2% of the variance, respectively. These results show that the 19 factors explained trust in AI well, and factors in the trustee dimension (i.e., those related to the judgment of the trustworthiness of AI) appeared to have the highest explanatory power.
To pin down the relative contributions of the factors to trust in AI, we further ran a stepwise regression analysis with all 19 factors as predictors, employing a forward selection method with the Bayesian information criterion as the selection metric. The main results of this analysis are summarized in . First, nine factors were entered into the regression model, and collectively, they explained 56.6% of the variance in trust in AI. This is the same amount of variance explained by the 19 factors together, suggesting that the other 10 factors did not provide any additional explanatory power after these nine.
Figure 2. Cumulative adjusted R2 in a stepwise regression analysis of trust in artificial intelligence. Each bar represents the cumulative R2 contribution of a factor that was entered into the regression model. Nine factors were selected, and they are ordered by their selection sequence. The bars are color-coded to indicate the trust dimension to which each selected factor belongs.
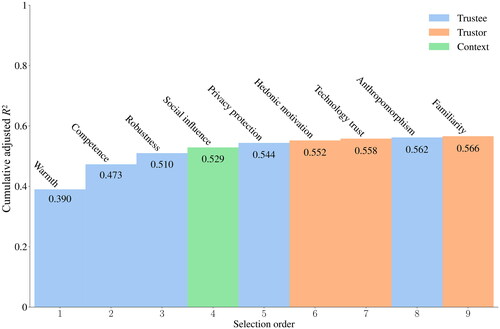
Second, among the nine factors, five were from the trustee dimension, including the first three selected: warmth, competence, and robustness. Warmth and competence alone could explain 47.3% of the variance in trust in AI. For the remaining four factors, social influence was the only one from the context dimension; the other three were all from the trustor dimension, although their added contributions were limited.
Overall, our analyses show that warmth and competence were the two most influential factors affecting participants’ trust in AI. Coincidentally, they were also identified as the two main factors affecting human trust relationships (Fiske et al., Citation2002). This suggests that trust in AI and trust in humans may be built on similar foundations with warmth and competence as the two main pillars. Given the relative importance of warmth and competence, we next explored the possible interaction between these two factors, as well as the interactions between them and other factors in determining trust in AI.
3.4. Interactions among trust-relevant factors
Previous research (e.g., Kulms & Kopp, Citation2018) suggested that warmth might moderate the effect of competence, and vice versa, on trust level. A moderation analysis shows that the interaction between these two factors was indeed significant in our study, β = 0.096, 95% confidence interval (CI) [0.063, 0.129]. visualizes this effect by plotting the average scores of trust in AI under low and high levels of warmth and competence. Slope analysis shows that the difference in the two lines’ slopes was statistically significant with p < 0.001. In general, these results indicate that the positive effect of competence on trust in AI was significantly enhanced when the perceived warmth was high, and a particularly high level of trust was obtained under the condition of high competence and high warmth.
Figure 3. Interactions among warmth, competence, and three other factors related to trust in artificial intelligence (AI). (A) The interaction between warmth and competence. High and low levels of warmth and competence correspond to one standard deviation above and below the mean, respectively. Values of trust in AI were the mean residual values after controlling for demographic and individual variables. (B), (C), and (D) The standardized direct and indirect effects of three factors on trust in AI with warmth and competence as the mediators. Δb is the mediation effect of warmth minus that of competence. Path coefficients in each model were significant with ps < 0.001. CI: confidence interval.
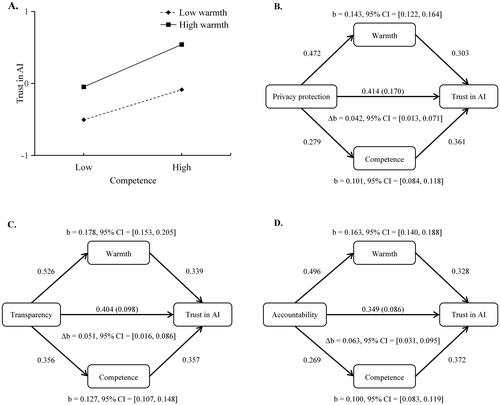
As explained in the Introduction, the effects of some factors on trust in AI might take two separate routes: directly and indirectly through warmth and competence. To test this, we ran a series of mediation models. In each model, we assumed both a direct path from one of the 17 factors to trust in AI and two parallel indirect paths via warmth and competence. Detailed results of these models can be found in Supplemental Table S3, and Panels B, C, and D of summarize the results for three factors: privacy protection, transparency, and accountability. These factors were singled out because they relate to issues that have generated much concern and discussion with the development of AI (AI HLEG, Citation2019).
The patterns of results for these three factors are very similar, so here we describe just those for privacy protection in detail. First, privacy protection could affect trust directly. However, this effect was greatly reduced after taking the mediation effects of warmth and competence into consideration, as each of these effects was sizable and statistically significant. Second, with two parallel mediation paths, we could compare the sizes of the two mediation effects. The effect of warmth turned out to be significantly larger than that of competence, as the 95% CI of their difference (i.e., Δb) did not include 0. Both results held for transparency and accountability as well.
As for the 14 other factors, our analyses show that the mediation effects of both warmth and competence were significant at the level of p < 0.001 for each, although the sizes of the effects in some cases were relatively small (e.g., safeguards and uncertainties). Moreover, for each of these factors, the absolute size of warmth’s mediation effect was larger than that of competence’s. However, their difference was significant at the level of p < 0.001 only for seven of them: agency, anthropomorphism, fairness, hedonic motivation, safeguard, trust propensity, and uncertainties, and not for the other seven: emotional experience, facilitating conditions, familiarity, perceived benefits, robustness, social influence, and technology trust.
Overall, results of the mediation analyses support our speculation that the effect of a factor on trust in AI usually takes place both directly and indirectly through warmth and competence. This was the case for all 17 factors examined in the present study. Between warmth and competence, the mediation effect of warmth was significantly larger than that of competence for most factors, whereas the reverse was not the case for a single one. Warmth, therefore, appears to play an especially important role in people’s trust in AI.
3.5. The role of trust in behavioral intentions
One important consequence of trust is the trustor’s intention to collaborate with and rely on the trustee to complete tasks and accomplish goals. We measured two behavioral intention variables in this study: intention to share private data with AI and support for using AI in HRM. The correlations between trust in AI and these two measures were 0.60 and 0.47, respectively, ps < 0.001 (see ), indicating that trust was indeed positively associated with behavioral intentions.
As previously discussed and shown, trust in AI was affected by a host of factors. If one treats these factors as antecedents of trust, the results of our study support a three-construct model in which influence starts with these factors influencing trust, then flows from trust to behavioral intention. Additionally, trust could also be an intervening variable between these factors and behavioral intention, mediating their effects. To test this, we constructed a series of mediation models, in each of which there was a direct path from one of the 19 factors to an intention measure and an indirect path through trust in AI. Detailed results of these models can be found in Supplemental Tables S4 and S5. shows the results pertaining to warmth and competence, the two factors with the greatest impact on trust in AI.
Figure 4. The direct and indirect effects of warmth and competence on two behavioral intention measures with trust in artificial intelligence (AI) as the mediator. (A) and (B) Intention to share private data with AI as the intention measure. (C) and (D) Support for using AI in HRM as the intention measure. The path coefficients in each model were significant with ps < 0.001. The results for support for using AI in HRM were based on data from participants who reported being currently employed (N = 1701). CI: confidence interval; HRM: human resources management.
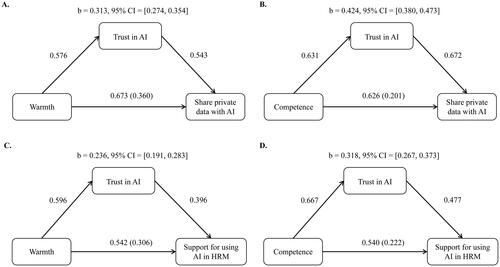
In each of the four models shown in , the mediation effect of trust in AI not only was statistically significant but also could account for a large proportion of the total effect, supporting the additional influence path from each factor to the behavioral intentions. The total effects of warmth and competence were similar, but their direct effects did differ, with those of warmth substantially higher than those of competence. This means that the impact of competence on behavioral intention depended more heavily on its indirect influence through trust in AI than that of warmth.
The mediation effects of trust in AI were also significant for the other 17 factors. Over the 19 factors, mediation could on average account for 59.2% (SD = 10.8%) and 50.0% (SD = 7.8%) of the total effect for intention to share private data with AI and support for using AI in HRM, respectively. With regard to the two intention measures, intention to share private data with AI was generally better explained by a mediation model than support for using AI in HRM, with the average size of the total effect for the former at 0.475 (SD = 0.139) and the latter at 0.410 (SD = 0.107) over the 19 factors.
Overall, the results indicate that trust in AI played two roles in its influence on the two behavioral intention measures: It affected the intentions directly and also served as an intervening variable between its antecedents and the intentions. A complex model could be constructed to describe the intricate relationships among the antecedents of trust, trust, and behavioral intention, and we did do so (see Supplemental Figures S4 and S5). The results confirm the dual roles of trust but provide limited insight beyond that. Therefore, we opted not to show and discuss them in detail here.
4. Discussion
It can be challenging for most people to understand a newly emerged technology, what it can do, how to use it, and how reliable it will be. With experience and knowledge, people gradually form their own perceptions of and attitudes toward the new technology, using it to varying degrees. Trust plays a critical role in this process. It is both a product of learning and understanding and a catalyst for the further development of the technology or its demise. With fast-growing AI technology as the target, we examined factors that affect people’s trust in AI and how these factors and trust together influence people’s intention to collaborate with AI (i.e., share private data with AI systems) and support for its use in the workplace (i.e., for HRM-related tasks).
Conducting a large-scale cross-sectional survey on a representative adult sample in China, we centered our investigation on addressing three main questions. We found that first, among the three dimensions of a trust relationship, factors related to the trustee (i.e., AI) affected participants’ trust in AI the most; and among these factors, warmth and competence were the two most pivotal ones. Second, warmth moderated the effect of competence on trust in AI, and these two factors mediated other factors’ influences on trust, with the mediation effect of warmth being particularly strong. And third, trust in AI not only correlated positively with behavioral intentions but also mediated the effects of factors affecting it (i.e., the antecedents of trust) on intentions, providing an integrated view of the relationships among antecedents of trust, trust, and behavioral intention. Next, we discuss the connections of our results with those of previous studies and the theoretical contributions and practical implications of our findings.
4.1. Levels of trust in AI
Participants in our study in general did not demonstrate a high level of trust in AI (M = 3.153 out of 5), and younger participants in the 18- to 29-year-old age range were particularly wary of AI (M = 2.854). In a recent worldwide study, Gillespie et al. (Citation2023) collected data in 17 countries, including China, asking participants a series of questions regarding trust in AI. Despite the differences in the primary goals of and the corresponding analyses used in their study and ours, the two studies share much common ground.
They found that the level of trust in AI differed substantially across countries: Whereas participants in Western countries, such as France and Australia, were quite skeptical of AI (with mean scores below 4 on a 7-point scale), participants in China and India showed the highest levels of trust (with mean scores slightly above 5, equivalent to roughly 3.6 out of 5). Their China finding, therefore, appears different from ours. They also found contrasting patterns of age differences in trust: Whereas younger people trusted AI more than older people in most countries, this pattern was reversed in China and South Korea. This finding in China was consistent with ours and offers a clue to why the overall trust level of their Chinese sample was higher than that of our study.
It turns out that their study included participants in the 55- to 91-year-old age range, which they referred to as “Baby Boomers plus,” whereas we did not recruit participants above 60 years old for concerns that participants in this age group would have limited experience with and knowledge about AI. This made the average age of their Chinese participants much older than ours (43.0 vs. 36.5). Given that older people were found to be more trusting in AI in both studies for China, having more such participants would inflate the average level of trust in the entire sample. The different scales used to measure trust in the two studies and other factors might contribute to the differing results as well, but we think that the difference in age composition is likely the primary cause.
The Gillespie et al. (Citation2023) study also asked about participants’ willingness to rely on AI in four different domains: recommender systems, healthcare, security, and human resources (HR). They found that over all the countries, as well as in China, participants were least willing to rely on AI in HR. This low level of intention is consistent with the finding of our study with regard to support for using AI in HRM. Moreover, they treated trust in AI as a different construct from the perceived trustworthiness of AI (i.e., the overall impression of AI as the trustee) and argued that trustworthiness of AI has three main components: ability, humanity, and integrity, a treatment similar to ours. That said, besides reporting some descriptive statistics about these three factors, they did not describe the relationships between these factors and trust in AI or the relative importance of these factors.
4.2. Warmth and competence
One key finding of our study is the ultra-important roles of warmth and competence in determining people’s trust in AI. Warmth and competence are two factors first identified in the SCM, which was originally proposed by psychologists to explain how people form impressions and stereotypes of out-group members (Fiske et al., Citation2002). Over the years, SCM and its two-factor structure have been adopted to understand the formation and evolution of trust in various contexts, including trust between humans and computers, robots, and AI (Xue et al., Citation2020). Apparently, people’s trust of AI can be understood as a generalization of their trust of other people and has a strong component of sociality. Why?
According to the computers are social actors paradigm, individuals commonly assign social roles to computers and other automated agents, instead of treating them as emotionless and asocial machines, and apply social rules to them (Nass et al., Citation1994). This is likely caused by people lacking the experience, ability, or cognitive effort to distinguish representations of intelligence from real intelligence. With respect to AI, research has found that people extend their psychological expectations of humans to AI and exhibit prosocial behavior toward AI in economic games, such as the ultimatum game and the public goods game (Nielsen et al., Citation2022; Russo et al., Citation2021). Moreover, people tend to attribute social qualities to AI, such as gender and personality, and even develop stereotypes about it (Ahn et al., Citation2022; Eyssel & Hegel, Citation2012).
As discussed previously, AI differs from other automation technologies in its heightened autonomy. In recent years, the increasing anthropomorphization of AI, including its appearance, voice, and emotional responses, and the portrayal of AI in popular media as powerful, intentional thinking machines make AI even more humanlike and further strengthen people’s social expectations in their interactions with AI (Troshani et al., Citation2021). Therefore, it is perhaps not surprising that warmth and competence, the two characteristics valued in human trust relationships, are also the two most important ones in people’s trust relationship with AI.
Between the two, warmth was found in our study to play an overall more prominent role than competence. In addition to having a higher correlation with trust in AI, the mediation effects of warmth on other factors’ influences on trust were also generally stronger than those of competence. Warmth captures the intention component of trust, and an ill-intentioned AI should not be trusted, despite (and especially because of) being highly competent. On the other hand, an AI of relatively low competence can still earn people’s trust if it is perceived to care for humans’ interests (i.e., judged as warm). The joint effect of being highly warm and highly competent simultaneously, as found in our study, could be more than the simple addition of the two, giving trust in AI an extra boost. The implication of the findings on warmth is clear: AI developers and regulators should gear toward making AI behave more in alignment with humans’ interests and values. Solving this “alignment problem” might be the key to humans’ trust in and acceptance of AI (see also Christian, Citation2020).
4.3. Other influencing factors of trust in AI
Of the 19 factors we identified that might influence trust in AI, all were found to be correlated with trust at statistically significant levels. Given the large sample size of our study, this is perhaps not entirely unexpected. However, with a median correlation of 0.47, the associations were fairly strong, suggesting the relevance of these factors and validating our inclusion of them in the study. A stepwise regression analysis further shows that nine factors were more important than the others. Caution should be taken in interpreting this result, though, because these factors were selected on the basis of their relative contributions to explaining variance in trust, and it is possible that the other factors were not selected because of their shared variances with the nine selected factors. Each factor should by itself play a role in determining participants’ trust in AI.
Among the nine relatively important factors, five were from the trustee dimension, which represents participants’ perceived characteristics of AI. Of these factors, we have discussed warmth, competence, and privacy protection, and the role of anthropomorphism is intuitive to understand. Robustness, on the other hand, is a factor that has often been neglected in research on trust in AI. Although it has been thoroughly discussed and emphasized by AI developers, regulators, and tech-savvy users (AI HLEG, Citation2019), it was uncertain how laypeople would value it. Robustness turned out to be the third most important factor, after warmth and competence, suggesting that a robust AI was generally appreciated by our participants and could enhance their trust level. In their communications with users, AI providers may note this finding and put more emphasis on the reliability of their products and the repeatability of the outcomes.
Of the three factors in the trustor dimension, hedonic motivation was the relatively more important one. Previous research has shown that individuals with a strong hedonic motivation tend to exhibit greater engagement and more sustained use of a product (Gursoy et al., Citation2019). To enhance their experiences with AI products, however, individuals are often required to compromise their privacy (Guo et al., Citation2016), such as by sharing their preferences and personal history with an automatous driving vehicle or a short-video app. This presents a dilemma to both the users and the developers of AI: To have a higher level of trust in an AI product, experience-seeking users want the product to be more enjoyable, but that may come at a cost of being more intrusive to privacy. How to resolve this dilemma is an issue that can have profound impacts on the future development of AI.
Last, social influence was the sole factor from the context dimension. This finding highlights the effects of social networks and influential figures on people’s trust in AI. Under conditions of uncertainty, people often seek and try to learn from social information—that is, opinions, decisions, and behaviors of others—to guide their own decisions and actions (Gigerenzer et al., Citation2022; Sykes et al., Citation2009). With a perceived high level of uncertainty about AI by our participants (M = 4.155 out of 5), it is thus reasonable that they might form their trust attitudes toward AI under the influence of social information. Developers and companies promoting user trust in their AI products might consider having campaigns that make good use of social media and persuasive figures.
4.4. Practical implications
Understanding and navigating the complexity of human interactions with emerging technologies such as AI necessitates a nuanced appreciation of trust. Our study shows that warmth and competence stood out as the most critical determinants of trust in AI. This discovery underscores the importance of embedding social qualities and aligning AI algorithms with human interests and values in AI design, in addition to the pursuit of high performance capacity and reliability. By prioritizing the development of AI systems that are perceived as both warm (caring about human interests) and competent (effective and reliable), developers can help facilitate a more trusting relationship between users and AI technologies. This will not only enhance user acceptance but also pave ways for more integrative and cooperative human-AI interactions.
Trust is often dynamic, starting with a propensity and evolving with experience and the changing context of a trust relationship (Li et al., Citation2024). With regard to trust in AI, the three-dimension framework of trust can facilitate the understanding of this dynamic process and offer insights on possible interventions when necessary. For example, besides hedonic motivation, two factors in the trustor dimension, technology trust and familiarity, were also found as factors that could positively influence trust in AI. This suggests that educational and training programs designed to improve users’ understanding of how AI applications work and allow them to interact with AI applications deeply, such as riding in an autonomously driving vehicle than simply watching videos of it, should help enhance users’ trust in AI technology, even though they may be dubious about the technology at the first place. In their suggestions to promote a trustworthy AI, the AI HLEG (Citation2019) also proposed communication, education, and training as key strategies. The underlying rationale is that trust is a dyadic relationship between a trustor and a trustee, and building a strong relationship of trust requires active contributions from both parties.
AI has been increasingly applied in workplace, but has also faced much resistance from employees and managers (Canals & Heukamp, Citation2020). Besides the fear of losing their jobs to AI, concerns on fairness, transparency, and accountability of AI are also major reasons for this reluctance. Findings of our study imply that the issue at heart might be trust; thus, it is essential for organizations to find ways to enhance the trust of their employees in AI first in order to increase their support for the use of AI (see also Glikson & Woolley, Citation2020). For example, when introducing a new AI algorithm, explanations on how its design is aligned, or at least aims to align, with the interests of most employees in the organization should be supplemented on top of its functionalities. Also, it is advisable to establish a feedback mechanism that allows employees to express their concerns on AI. This feedback could facilitate employees’ perceptions of AI’s warmth and competence levels, help refine AI to better meet their needs, and thereby boost trust in and acceptance of AI applications.
5. Conclusion
Built on the three-dimension framework of trust, our study examined the influences of a large collection of factors on trust in AI, as well as their downstream effects on behavioral intentions toward AI. One key finding is that similar to their roles in interpersonal trust, warmth and competence were the two most important factors determining participants’ trust in AI, not only explaining a significant proportion of the variance in trust directly but also mediating the relationships between other factors and trust in AI. Although we measured a relatively large sample of participants, the measurement took place in only a single slice of time. With the fast development of AI, future research could employ a longitudinal approach to explore the evolution of trust in AI and its determinants over time. Moreover, expanding the focus from intentions to actual behaviors should deepen our understanding of how trust affects AI adoption, providing more insights and more direct evidence on how to enhance user engagement and trust in the applications of AI.
On October 30 2023, President Biden of the United States signed an executive order on the “safe, secure, and trustworthy development and use of artificial intelligence” (The White House, Citation2023). This and similar regulations and legal recommendations by government bodies around the world (e.g., AI HLEG, Citation2019) signify the urgent need for stakeholders to come up with frameworks that ensure the trustworthiness of AI. Input from behavioral research with the perspective of end users on this and related issues should be taken seriously in shaping such frameworks. Our study examined what people consider important factors in determining their trust in AI and the influences of these factors and trust on their intentions to collaborate with AI and use AI in the workplace. Our findings contribute to a better understanding of trust in AI from the user’s perspective, and provide practical suggestions for how AI developers and regulators could improve users’ trust in AI.
Trust in AI_Supplemental Material.pdf
Download PDF (1.2 MB)Acknowledgement
We thank members of the RAUM Lab for their comments and suggestions during the study, and Anita Todd for editing the article.
Disclosure statement
No potential conflict of interest was reported by the author(s).
Data availability statement
Data pertaining to the analyses and results of this study can be accessed without restrictions at https://osf.io/z4qr2.
Additional information
Funding
Notes on contributors
Yugang Li
Yugang Li is a PhD candidate at the Institute of Psychology, Chinese Academy of Sciences. His research focuses on human-AI interactions, especially on humans’ trust attitudes and behaviors toward AI.
Baizhou Wu
Baizhou Wu is a PhD candidate at the Institute of Psychology, Chinese Academy of Sciences. His research interests include intergroup behaviors, wisdom of the crowds, and the influences of social network and AI algorithms on individual and group behaviors.
Yuqi Huang
Yuqi Huang is a PhD candidate at the Institute of Psychology, Chinese Academy of Sciences. Her research focuses on impulsivity, impulsive and risky behaviors, and decision making under uncertainty.
Jun Liu
Liu Jun is a PhD candidate at the Institute of Psychology, Chinese Academy of Sciences. Her research interests include decision making under uncertainty, behavioral nudges, and the influences of misinformation and disinformation on human communications.
Junhui Wu
Junhui Wu is an associate professor at the Institute of Psychology, Chinese Academy of Sciences. Her research interests include trust and cooperation within and between groups, norm enforcement, pro-environmental behavior, and moral decisions by AI.
Shenghua Luan
Shenghua Luan is a professor and director of the Risk and Uncertainty Management (RAUM) lab at the Institute of Psychology, Chinese Academy of Sciences. His research covers various topics in judgment and decision making, including decision models, managerial decision making, group decisions, and collaborative decisions by humans and AI.
References
- Ahn, J., Kim, J., & Sung, Y. (2022). The effect of gender stereotypes on artificial intelligence recommendations. Journal of Business Research, 141, 50–59. https://doi.org/10.1016/j.jbusres.2021.12.007
- High-Level Expert Group on Artificial Intelligence [AI HLEG]. (2019, April). Ethics guidelines for trustworthy AI [European Commission report]. https://op.europa.eu/en/publication-detail/-/publication/d3988569-0434-11ea-8c1f-01aa 75ed71a1
- Ajzen, I., & Fishbein, M. (2000). Attitudes and the attitude-behavior relation: Reasoned and automatic processes. European Review of Social Psychology, 11(1), 1–33. https://doi.org/10.1080/14792779943000116
- Bigman, Y. E., & Gray, K. (2018). People are averse to machines making moral decisions. Cognition, 181, 21–34. https://doi.org/10.1016/j.cognition.2018.08.003
- Calhoun, C. S., Bobko, P., Gallimore, J. J., & Lyons, J. B. (2019). Linking precursors of interpersonal trust to human-automation trust: An expanded typology and exploratory experiment. Journal of Trust Research, 9(1), 28–46. https://doi.org/10.1080/21515581.2019.1579730
- Canals, J., & Heukamp, F. (2020). The future of management in an AI world. Palgrave Macmillan.
- Chao, C. M. (2019). Factors determining the behavioral intention to use mobile learning: An application and extension of the UTAUT Model. Frontiers in Psychology, 10, 1652. https://doi.org/10.3389/fpsyg.2019.01652
- Choung, H., David, P., & Ross, A. (2023). Trust in AI and its role in the acceptance of AI technologies. International Journal of Human–Computer Interaction, 39(9), 1727–1739. https://doi.org/10.1080/10447318.2022.2050543
- Christian, B. (2020). The alignment problem: Machine learning and human values. WW Norton & Company.
- Christoforakos, L., Gallucci, A., Surmava-Große, T., Ullrich, D., & Diefenbach, S. (2021). Can robots earn our trust the same way humans do? A systematic exploration of competence, warmth, and anthropomorphism as determinants of trust development in HRI. Frontiers in Robotics and AI, 8, 640444. https://doi.org/10.3389/frobt.2021.640444
- Colquitt, J. A., Scott, B. A., & LePine, J. A. (2007). Trust, trustworthiness, and trust propensity: A meta-analytic test of their unique relationships with risk taking and job performance. The Journal of Applied Psychology, 92(4), 909–927. https://doi.org/10.1037/0021-9010.92.4.909
- Cuddy, A. J., Fiske, S. T., & Glick, P. (2008). Warmth and competence as universal dimensions of social perception: The stereotype content model and the BIAS map. Advances in Experimental Social Psychology, 40, 61–149. https://doi.org/10.1016/S0065-2601(07)00002-0
- Delgosha, M. S., & Hajiheydari, N. (2021). How human users engage with consumer robots? A dual model of psychological ownership and trust to explain post-adoption behaviours. Computers in Human Behavior, 117, 106660. https://doi.org/10.1016/j.chb.2020.106660
- Eyssel, F., & Hegel, F. (2012). (S)he’s got the look: Gender stereotyping of robots. Journal of Applied Social Psychology, 42(9), 2213–2230. https://doi.org/10.1111/j.1559-1816.2012.00937.x
- Felzmann, H., Villaronga, E. F., Lutz, C., & Tamò-Larrieux, A. (2019). Transparency you can trust: Transparency requirements for artificial intelligence between legal norms and contextual concerns. Big Data & Society, 6(1), 205395171986054. https://doi.org/10.1177/2053951719860542
- Fiske, S. T., Cuddy, A. J. C., Glick, P., & Xu, J. (2002). A model of (often mixed) stereotype content: Competence and warmth respectively follow from perceived status and competition. Journal of Personality and Social Psychology, 82(6), 878–902. https://doi.org/10.1037/0022-3514.82.6.878
- Flores, F., & Solomon, R. C. (1998). Creating trust. Business Ethics Quarterly, 8(2), 205–232. https://doi.org/10.2307/3857326
- Frank, D. A., & Otterbring, T. (2023). Being seen… by human or machine? Acknowledgment effects on customer responses differ between human and robotic service workers. Technological Forecasting and Social Change, 189, 122345. https://doi.org/10.1016/j.techfore.2023.122345
- Frey, R., Pedroni, A., Mata, R., Rieskamp, J., & Hertwig, R. (2017). Risk preference shares the psychometric structure of major psychological traits. Science Advances, 3(10), e1701381. https://doi.org/10.1126/sciadv.1701381
- Frischknecht, R. (2021). A social cognition perspective on autonomous technology. Computers in Human Behavior, 122, 106815. https://doi.org/10.1016/j.chb.2021.106815
- Gefen, D., Karahanna, E., & Straub, D. W. (2003). Trust and TAM in online shopping: An integrated model. MIS Quarterly, 27(1), 51–90. https://doi.org/10.2307/30036519
- Gefen, D., & Straub, D. W. (2004). Consumer trust in B2C e-Commerce and the importance of social presence: Experiments in e-Products and e-Services. Omega, 32(6), 407–424. https://doi.org/10.1016/j.omega.2004.01.006
- Gigerenzer, G., Reb, J., & Luan, S. (2022). Smart heuristics for individuals, teams, and organizations. Annual Review of Organizational Psychology and Organizational Behavior, 9(1), 171–198. https://doi.org/10.1146/annurev-orgpsych-012420-090506
- Gillespie, N., Lockey, S., Curtis, C., Pool, J., & Akbari, A. (2023). Trust in artificial intelligence: A global study. The University of Queensland and KPMG Australia. https://doi.org/10.14264/00d3c94
- Glikson, E., & Woolley, A. W. (2020). Human trust in artificial intelligence: Review of empirical research. Academy of Management Annals, 14(2), 627–660. https://doi.org/10.5465/annals.2018.0057
- Guo, X., Zhang, X., & Sun, Y. (2016). The privacy–personalization paradox in mHealth services acceptance of different age groups. Electronic Commerce Research and Applications, 16, 55–65. https://doi.org/10.1016/j.elerap.2015.11.001
- Gursoy, D., Chi, O. H., Lu, L., & Nunkoo, R. (2019). Consumers acceptance of artificially intelligent (AI) device use in service delivery. International Journal of Information Management, 49, 157–169. https://doi.org/10.1016/j.ijinfomgt.2019.03.008
- Harris-Watson, A. M., Larson, L. E., Lauharatanahirun, N., DeChurch, L. A., & Contractor, N. S. (2023). Social perception in human-AI teams: Warmth and competence predict receptivity to AI teammates. Computers in Human Behavior, 145, 107765. https://doi.org/10.1016/j.chb.2023.107765
- Hofeditz, L., Mirbabaie, M., & Ortmann, M. (2023). Ethical challenges for human–agent interaction in virtual collaboration at work. International Journal of Human–Computer Interaction, 40, 1–17. https://doi.org/10.1080/10447318.2023.2279400
- James, G., Witten, D., Hastie, T., Tibshirani, R., & Taylor, J. (2023). An introduction to statistical learning: With applications in python. Springer Nature.
- Ji, Z., Yang, Y., Fan, X., Wang, Y., Xu, Q., & Chen, Q. W. (2021). Stereotypes of social groups in mainland China in terms of warmth and competence: Evidence from a large undergraduate sample. International Journal of Environmental Research and Public Health, 18(7), 3559. https://doi.org/10.3390/ijerph18073559
- Jian, J. Y., Bisantz, A. M., & Drury, C. G. (2000). Foundations for an empirically determined scale of trust in automated systems. International Journal of Cognitive Ergonomics, 4(1), 53–71. https://doi.org/10.1207/S15327566IJCE0401_04
- Kaplan, A. D., Kessler, T. T., Brill, J. C., & Hancock, P. A. (2021). Trust in artificial intelligence: Meta-analytic findings. Human Factors, 65(2), 337–359. https://doi.org/10.1177/00187208211013988
- Khalilzadeh, J., Ozturk, A. B., & Bilgihan, A. (2017). Security-related factors in extended UTAUT model for NFC based mobile payment in the restaurant industry. Computers in Human Behavior, 70, 460–474. https://doi.org/10.1016/j.chb.2017.01.001
- Khan, G. F., Swar, B., & Lee, S. K. (2014). Social media risks and benefits: A public sector perspective. Social Science Computer Review, 32(5), 606–627. https://doi.org/10.1177/0894439314524701
- Kim, D. J., Ferrin, D. L., & Rao, H. R. (2008). A trust-based consumer decision-making model in electronic commerce: The role of trust, perceived risk, and their antecedents. Decision Support Systems, 44(2), 544–564. https://doi.org/10.1016/j.dss.2007.07.001
- Komiak, S. X., & Benbasat, I. (2004). Understanding customer trust in agent-mediated electronic commerce, web-mediated electronic commerce, and traditional commerce. Information Technology and Management, 5(1/2), 181–207. https://doi.org/10.1023/B:ITEM.0000008081.55563.d4
- Kong, D. T. (2018). Trust toward a group of strangers as a function of stereotype-based social identification. Personality and Individual Differences, 120, 265–270. https://doi.org/10.1016/j.paid.2017.03.031
- Kulms, P., & Kopp, S. (2018). A social cognition perspective on human–computer trust: The effect of perceived warmth and competence on trust in decision-making with computers. Frontiers in Digital Humanities, 5. https://www.frontiersin.org/article/10.3389/fdigh.2018.00014 https://doi.org/10.3389/fdigh.2018.00014
- Lee, J. D., & See, K. A. (2004). Trust in automation: Designing for appropriate reliance. Human Factors, 46(1), 50–80. https://doi.org/10.1518/hfes.46.1.50_30392
- Lee, J. G., Kim, K. J., Lee, S., & Shin, D. H. (2015). Can autonomous vehicles be safe and trustworthy? Effects of appearance and autonomy of unmanned driving systems. International Journal of Human-Computer Interaction, 31(10), 682–691. https://doi.org/10.1080/10447318.2015.1070547
- Leeman, D., & Reynolds, D. (2012). Trust and outsourcing: Do perceptions of trust influence the retention of outsourcing providers in the hospitality industry? International Journal of Hospitality Management, 31(2), 601–608. https://doi.org/10.1016/j.ijhm.2011.08.006
- Li, C., Wang, C., & Chau, P. Y. K. (2022). Revealing the black box: Understanding how prior self-disclosure affects privacy concern in the on-demand services. International Journal of Information Management, 67, 102547. https://doi.org/10.1016/j.ijinfomgt.2022.102547
- Li, Y., Wu, B., Huang, Y., & Luan, S. (2024). Developing trustworthy artificial intelligence: Insights from research on interpersonal, human-automation, and human-AI Trust. Frontiers in Psychology, 15, 1382693. https://doi.org/10.3389/fpsyg.2024.1382693
- Lockey, S., Gillespie, N., & Curtis, C. (2020). Trust in artificial intelligence: Australian insights. The University of Queensland and KPMG. https://doi.org/10.14264/b32f129
- Mayer, R. C., Davis, J. H., & Schoorman, F. D. (1995). An integrative model of organizational trust. Academy of Management Review, 20(3), 709–734. https://doi.org/10.5465/amr.1995.9508080335
- McKnight, D. H., Cummings, L. L., & Chervany, N. L. (1998). Initial trust formation in new organizational relationships. Academy of Management Review, 23(3), 473–490. https://doi.org/10.5465/amr.1998.926622
- Merritt, S. M. (2011). Affective processes in human–automation interactions. Human Factors, 53(4), 356–370. https://doi.org/10.1177/0018720811411912
- Nass, C., Steuer, J., & Tauber, E. R. (1994). Computers are social actors. In R. Grinter, T. Rodden, P. Aoki, E. Cutrell, R. Jeffries, & G. Olson (Eds.), Proceedings of the SIGCHI conference on human factors in computing systems (pp. 72–78). Association for Computing Machinery.
- Nielsen, Y. A., Thielmann, I., Zettler, I., & Pfattheicher, S. (2022). Sharing money with humans versus computers: On the role of honesty-humility and (non-) social preferences. Social Psychological and Personality Science, 13(6), 1058–1068. https://doi.org/10.1177/19485506211055622
- Ozmen Garibay, O., Winslow, B., Andolina, S., Antona, M., Bodenschatz, A., Coursaris, C., Falco, G., Fiore, S. M., Garibay, I., Grieman, K., Havens, J. C., Jirotka, M., Kacorri, H., Karwowski, W., Kider, J., Konstan, J., Koon, S., Lopez-Gonzalez, M., Maifeld-Carucci, I., … Xu, W. (2023). Six human-centered artificial intelligence grand challenges. International Journal of Human–Computer Interaction, 39(3), 391–437. https://doi.org/10.1080/10447318.2022.2153320
- Podsakoff, P. M., MacKenzie, S. B., Lee, J.-Y., & Podsakoff, N. P. (2003). Common method biases in behavioral research: A critical review of the literature and recommended remedies. The Journal of Applied Psychology, 88(5), 879–903. https://doi.org/10.1037/0021-9010.88.5.879
- Rheu, M., Shin, J. Y., Peng, W., & Huh-Yoo, J. (2021). Systematic review: Trust-building factors and implications for conversational agent design. International Journal of Human–Computer Interaction, 37(1), 81–96. https://doi.org/10.1080/10447318.2020.1807710
- Russo, P. A., Duradoni, M., & Guazzini, A. (2021). How self-perceived reputation affects fairness towards humans and artificial intelligence. Computers in Human Behavior, 124, 106920. https://doi.org/10.1016/j.chb.2021.106920
- Salisbury, W. D., Pearson, R. A., Pearson, A. W., & Miller, D. W. (2001). Perceived security and World Wide Web purchase intention. Industrial Management & Data Systems, 101(4), 165–177. https://doi.org/10.1108/02635570110390071
- Santoni de Sio, F., & Mecacci, G. (2021). Four responsibility gaps with artificial intelligence: Why they matter and how to address them. Philosophy & Technology, 34(4), 1057–1084. https://doi.org/10.1007/s13347-021-00450-x
- Schepman, A., & Rodway, P. (2023). The General Attitudes towards Artificial Intelligence Scale (GAAIS): Confirmatory validation and associations with personality, corporate distrust, and teneral trust. International Journal of Human–Computer Interaction, 39(13), 2724–2741. https://doi.org/10.1080/10447318.2022.2085400
- Shin, D., & Park, Y. J. (2019). Role of fairness, accountability, and transparency in algorithmic affordance. Computers in Human Behavior, 98, 277–284. https://doi.org/10.1016/j.chb.2019.04.019
- Shneiderman, B. (2020). Human-centered artificial intelligence: Reliable, safe & trustworthy. International Journal of Human–Computer Interaction, 36(6), 495–504. https://doi.org/10.1080/10447318.2020.1741118
- Simon, J. C., Styczynski, N., & Gutsell, J. N. (2020). Social perceptions of warmth and competence influence behavioral intentions and neural processing. Cognitive, Affective & Behavioral Neuroscience, 20(2), 265–275. https://doi.org/10.3758/s13415-019-00767-3
- Sundar, S. S., & Kim, J. (2019). Machine heuristic: When we trust computers more than humans with our personal information. In Proceedings of the 2019 CHI Conference on Human Factors in Computing Systems (Article 538). Association for Computing Machinery. https://doi.org/10.1145/3290605.3300768
- Sykes, T. A., Venkatesh, V., & Gosain, S. (2009). Model of acceptance with peer support: A social network perspective to understand employees’ system use. MIS Quarterly, 2, 33, 371–393. https://doi.org/10.2307/20650296
- Troshani, I., Hill, S. R., Sherman, C., & Arthur, D. (2021). Do we trust in AI? Role of anthropomorphism and intelligence. Journal of Computer Information Systems, 61(5), 481–491. https://doi.org/10.1080/08874417.2020.1788473
- Venkatesh, V., Morris, M. G., Davis, G. B., & Davis, F. D. (2003). User acceptance of information technology: Toward a unified view. MIS Quarterly, 3, 27, 425–478. https://doi.org/10.2307/30036540
- Venkatesh, V., Thong, J. Y. L., & Xu, X. (2012). Consumer acceptance and use of information technology: Extending the unified theory of acceptance and use of technology. MIS Quarterly, 1, 36, 157–178. https://doi.org/10.2307/41410412
- The White House (2023, October 30). Fact sheet: President Biden issues executive order on safe, secure, and trustworthy artificial intelligence. The White House. https://www.whitehouse.gov/briefing-room/statements-releases/2023/10/30/fact-sheet-president-biden-issues-executive-order-on-safe-secure-and-trustworthy-artificial-intelligence/
- Xue, J., Zhou, Z., Zhang, L., & Majeed, S. (2020). Do brand competence and warmth always influence purchase intention? The moderating role of gender. Frontiers in Psychology, 11, 248. https://doi.org/10.3389/fpsyg.2020.00248
- Ye, C., Hofacker, C. F., Peloza, J., & Allen, A. (2020). How online trust evolves over time: The role of social perception. Psychology & Marketing, 37(11), 1539–1553. https://doi.org/10.1002/mar.21400
- Zell, E., Strickhouser, J. E., & Krizan, Z. (2018). Subjective social status and health: A meta-analysis of community and society ladders. Health Psychology: official Journal of the Division of Health Psychology, American Psychological Association, 37(10), 979–987. https://doi.org/10.1037/hea0000667
- Zhang, J., Luximon, Y., & Li, Q. (2022). Seeking medical advice in mobile applications: How social cue design and privacy concerns influence trust and behavioral intention in impersonal patient–physician interactions. Computers in Human Behavior, 130, 107178. https://doi.org/10.1016/j.chb.2021.107178