Abstract
Datafication, the process where users’ actions online are pervasively recorded, tracked, aggregated, analysed, and exploited by online services in multiple ways, is becoming increasingly common today. However, we know little about how children, especially non-Western children, perceive such practices. Through one-to-one semi-structured interviews with 36 children aged 11–14 from Chinese middle schools, we examined how Chinese children perceive datafication practices. We identified three knowledge gaps in children’s current perceptions of datafication practices online, including their lack of recognition of (i) their data ownership, (ii) data being transmitted across platforms, and (iii) datafication could go beyond video recommendation and include inferences and profiling of their personal aspects. Through contextualising these observations within the Chinese context and its unique online ecosystem, we identified cultural traits in Chinese children’s perceptions of datafication. We drew on education theories to discuss how to support the future digital literacy development and design online platforms for Chinese children.
1. Introduction
In today’s digital age, more and more children globally are engaging with online platforms with increasing frequency. As online technologies – internet-enabled platforms, applications, and digital tools – continue to grow, they are becoming increasingly crucial for children’s access to essential resources such as education, socialisation, participation, wellness, and entertainment (Livingstone et al., Citation2019). In the UK, 96% of children aged 5–15 are online, and more than half of the ten-year-olds go online using their own devices (Ofcom, Citation2020). In the US, 97% of children between 3 to 18 have regular access to the internet (National Center for Education Statistics, Citation2023). Meanwhile, China is home to more than 20% of the online population worldwide (DataReportal, Citation2022; Statista Research Department, Citation2022). 183 million Chinese children are online, 46.2% them already on social media platforms (China Internet Network Information Center, Citation2022), 62.5% using the Internet for gaming, and 89.9% using the Internet for online learning.
This growing dependency on digital platforms has raised increasing concerns about the long-term implications of datafication. This process involves the pervasive recording, tracking, and analysis of children’s actions by online services, often exploited for behavioural engineering and monetisation (Mascheroni, Citation2020; Mejias & Couldry, Citation2019; Zuboff, Citation2019). Central to this datafication phenomenon is the ability of these online services to conduct data inference, utilising algorithms to evaluate personal attributes associated with a user (Livingstone et al., Citation2019). These attributes may include aspects such as performance at work, economic status, health, preferences, interests, reliability, behaviour, location, or movement patterns (Information Commissioner’s Office, Citation2020). This practice of datafication is nearly inescapable and irreversible, even with attempts at data deletion (Livingstone et al., Citation2019). Both children’s and adult’s online experience is being systematically quantified, analysed, and used to create profiles, which could consequently have immediate or long-term implications for them (Couldry & Mejias, Citation2019; Lupton & Williamson, Citation2017; Zuboff, Citation2019). On the other hand, these processes, which often occur behind the scenes of mobile apps and online services, are not as widely recognised or discussed as potential risks, in comparison to other, more readily identifiable harms like the collection or disclosure of particular kinds of sensitive data, or exposure to inappropriate content and stranger danger online. The phenomena of datafication aren’t exclusively focused on children. However, it’s crucial to note that the current generation of children is the inaugural one growing up amid this increasingly ubiquitous data-centric environment. This unique circumstance has the potential to profoundly shape their long-term development (Alneyadi et al., Citation2023; Benvenuti et al., Citation2023). While it is recognised that there are both positive and negative sides of datafication, such as providing a more personalised experience to users, it is worth noting that children can be a vulnerable group and may often be overlooked in the designs sociotechnical systems, particularly by online service providers. Considering these factors, we feel a strong need to investigate children’s ongoing experiences and understand their perceptions of these pervasive datafication practices.
While some recent studies have found evidence that children possess some rudimentary understanding of online datafication and related implications, indicating the various levels of understanding and perceptions of children (Mioduser & Levy, Citation2010; Wang et al., Citation2022, Citation2023), limited research has focused on non-western countries and more specifically the Chinese context. China is an upper-middle-income developing country, an emerging market, and a developing economy (International Monetary Fund, Citation2023; The World Bank, Citation2023; Ye et al., Citation2023). It is home to 1.05 billion internet users, accounting for more than 20% of the online population worldwide. Understanding how Chinese children perceive the practice of datafication is crucial to extending the current research landscape. However, the self-contained nature of the Internet ecosystem in China (explained further in Section Datafication on Online Platforms, including Chinese Platforms) presents unique challenges to achieving this understanding. This research provides timely insights to fill this knowledge gap.
In this paper, we focused on investigating how children in China understand and perceive datafication on social media platforms that are unique to the Chinese market and address the following research questions:
RQ1: What are the current perceptions among children in China regarding datafication?
RQ2: How, if at all, may children in China demonstrate any unique cultural traits regarding their perceptions of datafication?
RQ3: What kinds of designs and supports may be needed by Chinese children to help them navigate datafication?
We report our findings from semi-structured interviews with 36 children from Chinese middle schools, aged 11–14, undertaken between October and November 2022. We identified three key knowledge gaps in children’s current awareness and perceptions of datafication practices online, including their lack of recognition of (i) their data ownership, (ii) data being transmitted across platforms, and (iii) the potential for datafication to extend beyond simple video recommendations and make inference of their personal aspects. Through contextualising these observations within Chinese culture and philosophy, we examine specific cultural traits that may be associated with Chinese children’s perceptions of datafication. Drawing on critical education theories, we discuss what future digital literacy support and the design of online platforms for Chinese children should entail, considering China’s unique online ecosystem and cultural influences.
2. Background and related work
2.1. Datafication on online platforms, including Chinese platforms
To set the boundaries for our exploration, we initially seek to clarify our use of the term datafication in the online context. This term refers to the extensive recording, tracking, collating, analysis, and exploitation of user activities by online services for various purposes, including behavioural engineering and monetisation (Mascheroni, Citation2020; Mejias & Couldry, Citation2019; Zuboff, Citation2019). Central to datafication is the ability of online services to undertake data inference, where they analyze user data, guided by algorithms, with the intention of assessing specific personal facets related to an individual (Livingstone et al., Citation2019). This can particularly encompass predicting elements concerning an individual’s work performance, financial status, health, personal preferences, interests, reliability, conduct, location, or movements (Information Commissioner’s Office, Citation2020). While, datafication has been proven effective in certain use cases, such as providing users with the services they prefer and improving their digital experiences (Agrawal et al., Citation2011; Khan et al., Citation2014), such practices can pose significant challenges to people’s privacy, and potentially undermine users’ autonomy due to advanced dataveillance techniques (Sax, Citation2016; Wachter, Citation2020).
To this end, datafication in the Chinese online context and related app ecosystem is specialised by its “self-sustained” nature due to the restrictions of China’s Golden Shield project on the internet of mainland China, also known as “Great Firewall of China” (Kaye et al., Citation2021). Most Western/International tech giants, including Google and Meta, were banned from the country’s digital sphere. Their absence led to the Chinese market being shared by multiple domestic tech companies (and their apps), including WeChat, DouYin (with TikTok being its international version owned by the same company), TaoBao and more, while with no clear market domination. More than 2.32 million mobile apps were available on the domestic market in China (Statista, Citation2022). For online video platforms only, there exist more than ten apps that are known as “popular” (with more than hundreds of millions of active users), ranging from Bilibili, iQIYI, Youku Video, Tencent Video, DouYin, KuaiShou and more. Meanwhile, it has been known that these platforms regularly collect and process users’ data. Research on DouYin showed that there have been profiling practices on its users, nudging users towards certain content such as idealised images which could have negative impacts on the body satisfaction of young girls (Maes & Vandenbosch, Citation2022). WeChat has been found to introduce personalised adverts based on user profiles, in particular their estimated social status and income (Liu et al., Citation2019). A market analysis of prevalent social media apps on the Chinese market showed that many of them (such as Taobao) have been introducing price discrimination on their users based on interest estimation (Han et al., Citation2018).
Meanwhile, the Chinese government have attempted to protect children from such practices. Similar to GDPR, the newly released DSL (Data Security Law of China) and PIPL (Personal Information Protection Law) lay out rules on web service providers, restricting them from collecting and processing personal information of children under 14 unless they received legal consent from their guardians (Pernot-Leplay, Citation2020), and strictly banned children under 16 to access livestream shopping services unless receiving parental consents (Cunningham et al., Citation2019). That said, unlike most Western social media platforms, which mandate a minimum age requirement of 13 for their users, most Chinese social media platforms do not have any compulsory age requirements for setting up an account (apart from the use of their livestream services). And while some tech companies have introduced parental control options and teen modes, such as how DouYin tried to limit the use of the platform for children under 14 to 40 minutes a day (BBC News, Citation2021), most children could easily access most social media platforms simply through the visitor mode. According to the latest report from the China Internet Network Information Center, 183 million Chinese minors are now online whereas 61% of children use the Internet for gaming and 46.2% are on social media platforms such as DouYin and Kuaishou (China Internet Network Information Center, Citation2022).
2.2. Children’s perceptions of datafication online
Datafication practices are becoming increasingly common in the online world today, and can be found on almost any online platform around the world (Büchi et al., Citation2020; Kazai et al., Citation2016; Rao et al., Citation2015). There has been growing concern relating to the datafication of children, especially as children may lack the awareness, knowledge, or mental capacities to understand or be aware of such practices. Kumar et al. (Citation2017) reported that U.S. children between the ages of 8 and 11 begin to understand that data collection on online platforms could create some risks for them, but tend to associate such risks mainly with ‘stranger-danger’, which limits their association with the algorithmic operations underneath. Zhao et al. (Citation2019) found that UK children aged 6–10 could identify and articulate certain privacy risks well, such as information oversharing or revealing real identities online, but had less awareness of other risks, such as online tracking or personalised promotions. Another study shows that children between 12–17 demonstrated some awareness of the ‘data traces’ they left online (done with Belgium children) (Zarouali et al., Citation2017) and of device tracking (done with U.S. children) (Malvini Redden & Way, Citation2017), but found it hard to make a personal connection or apply such knowledge to themselves (done with U.S. children) (Acker & Bowler, Citation2017).
Apart from the privacy implications of the datafication of children, some previous literature has also looked at how children interpret the algorithmic nature of data processing practices. It has been shown that when given sufficient support, children were capable of understanding the role of data in determining machine behaviours (Mioduser & Levy, Citation2010). Meanwhile, practices of teaching digital literacy based on learning science theory have been proposed to help children understand the implications of data processing, thus promoting their ability to engage in the critique of algorithmic practices (Ali et al., Citation2019; Gibson, Citation2006; Rachayu et al., Citation2022; Tempornsin et al., Citation2019), although not specifically focusing on online datafication practices.
On the other hand, some more recent research has specifically looked at how children interpreted online datafication practices, and found that children were capable of grasping essential concepts related to datafication, such as that their personal data (such as activity history) could be processed and used to sell products to users such as themselves (Livingstone et al., Citation2019), and they already posed rudimentary understanding of online datafication such that platforms could exploit their data and algorithms to “make assumptions” about them (Wang et al., Citation2022).
The existing research on children’s perception of online privacy provided us with a useful starting point. Meanwhile, very few of these previous research with children were done in developing countries including China. Previous research has typically investigated how Chinese children perceive topics around data security and online safety in general. Yu et al. (Citation2021), for instance, looked at children’s knowledge of information security and found that children care significantly about the subject. Zheng (Citation2015) also found that Chinese children were to some extent aware of online risks, including potential personal privacy risks. However, evidence from the latest report on Children’s Media Literacy in China (China National Children’s Center & Tencent, Citation2022) suggested that many Chinese children might not be ready for recognising broader online risks, especially those related to data and privacy, due to the lack of information and digital literacy. On the other hand, through a survey with 500 teens (between 13 and 18) users of DouYin, it has been found that parental influence is significant in children’s privacy behaviours on short video platforms and active mediation from parents can help teens develop privacy strategies and reduce risk-seeking behaviours (Kang et al., Citation2021).
2.3. Cultural considerations of privacy in China
Several studies have highlighted the role of parents, family, and culture in shaping children’s privacy behaviours. While privacy is a universal concern, its conceptualisation may vary among different cultural groups (Altman, Citation1977), emphasizing the importance of considering the cultural contexts when studying privacy. In the Chinese culture, privacy may be perceived differently from the Western culture, often facing conflicts with other societal values (Ma, Citation2023). The influence of Confucianism, a prominent philosophy in China, and collectivism, significantly impacts the perceptions of privacy among Chinese children and their families Naftali (Citation2010). For instance, in the Confucian ethos, Chinese children are first seen as part of the family or a collective (‘’) before being considered as individuals, which may affect have children’s privacy is perceived by adults or the society. Other studies have also shown that collectivism is deeply rooted in the Chinese society, particularly in discussions related to privacy (Cao, Citation2009; Yao-Huai, Citation2005). This contributes to a paradoxical situation where Chinese children’s privacy is being increasingly debated in public policy while parents continue to retain strong control of children’s freedom of movement.
In fact, while individual privacy has been long recognised as a fundamental human right in many Western societies (Warren & Brandeis, Citation1890; Diggelmann & Cleis, Citation2014), privacy is a relatively recent concept in China. Historically, discussions on privacy in China have been often framed in terms of the relationship between the “realm of si () [personal, self, selfish, private] and gong () [public, public space, open, communal].” (McDougall et al., Citation2002). Under the collectivism Chinese culture, the concept of si () often carries a negative connotation of being suspicious and selfish.
This statement has been further validated by empirical evidence. For example, Wang and Metzger (Citation2021) investigated how collectivism and individualism influence how Chinese college-age students manage their privacy boundaries on social media. Students from collectivist backgrounds tend to disclose more personal information to their parents on social media, as a way to promote family harmony. They perceived their parents as almost co-owners of their personal information and reported less perceived parental privacy invasion. Parents in collectivist cultures also tended to set smaller privacy boundaries for their children compared to those from individualistic cultures (Wang & Metzger, Citation2021). The collectivist culture also links the interests of the children to those of the nation, which influences how Chinese children perceive their online data privacy (Naftali, Citation2009). Thus, an understanding of how Chinese children perceive datafication will contribute fresh and timely inputs to the global research agenda and aid practitioners in designing for the Chinese online ecosystem.
3. Study design
The focus of our study is on investigating children’s perceptions of datafication practices online in China. For this, we chose to use one-to-one semi-structured interviews with children to gain deep, qualitative data for our research. The study was conducted in person between October and November 2022. Our interview protocol was a reproduction of the one published in the prior research by Wang et al. (Citation2022), which conducted interviews with children aged 7–13 from the UK to examine their perspectives on datafication. Our intention in replicating their methodology is twofold: firstly, to set a benchmark and to provide evidence for children in developing countries such as China. This is particularly important given that the majority of existing research centers on Western children, and experiences and understandings can vary significantly across different cultural contexts. Additionally, replication is invaluable in HCI to analyze quickly evolving phenomena, particularly the phenomenon of datafication (Oulasvirta & Hornbæk, Citation2016).
In the meantime, we made several distinctive adjustments to the original method of Wang et al. (Citation2022). First of all, we carefully considered the scenarios that would resonate with the experience of Chinese children and incorporated localised adjustments suitable for a Chinese context (elaborated later). Furthermore, we adapted discussions about data and associated privacy implications to better suit the local language context. For example, the expression of digitalisation (and datafication () can closely resemble each other in Chinese, posing a challenge for middle school children to distinguish. To deal with this challenge, before children watched videos, we introduced the general concept of datafication to them. Then during the interview, whenever appropriate, we encouraged children to clarify and verify they were referring to the correct concept, i.e., dataficaiton, before proceeding with the activities. Finally, in our study protocol, we did not limit our discussions with children to a specific video platform (such as YouTube). Unlike many other markets that often are dominated by a few major online platforms (e.g., YouTube, Netflix), the Chinese digital landscape is characterized by a multitude of competing social media platforms, e.g., iQIYI, Youku Video, Tencent Video, Bilibili, DouYin, KuaiShou. Chinese Internet users are often less committed to a single platform, emphasising the necessity of maintaining open discussions in our study protocol.
Our study involves two steps: (1) to begin with, children were invited to engage in hands-on tasks to recreate their everyday experiences with online social media platforms and share their thoughts about these tasks, and (2) children were exposed to brief videos, featuring fictional scenarios that depict various datafication practices commonly used by online social media platforms to process and make use of users’ data; and subsequently children were invited to express their thoughts about the datafication practices shown in the videos.
3.1. Part 1: Video platform tasks
In part 1, we began the interview by walking through a series of tasks with participant children on their familiar devices provided by us (). The purpose was partially to recreate and remind children of their everyday experience on social media platforms (especially in the forms of online video platforms and short video platforms) and for us to observe how children perceive data during several critical interaction moments. For this purpose, we started with initiating conversations by discussing the most frequently used video platforms by the participants. We then encouraged children to complete several core interaction tasks on the platform, including finding a video, identifying how videos are recommended and deciding which video to watch. We observed that core interactions are very similar across all Chinese online video platforms. In view of the emphasis on the datafication process, rather than content, the tasks fit well in the context of different video platforms.
Figure 1. Children were invited to complete three tasks on video platforms they used most frequently.
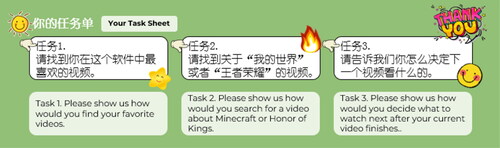
Figure 2. Screenshots of video 1 and 2. The metaphor is about a chef may learn about your personal taste based on what you eat frequently and what kind of food you may like more.
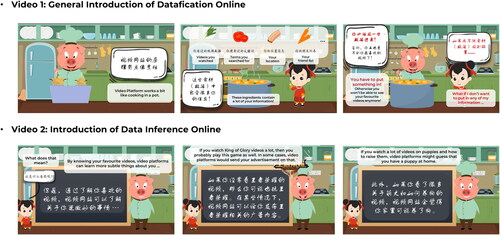
Our problem-solving tasks were carefully designed based on the ‘critical interaction points’ proposed in Wang et al. (Citation2022). These critical interaction points refer to: 1). Entering the online platform - where children were asked to show the researchers how they would normally find their favorite videos on the video platforms (Task 1); 2). Searching for a video - the search function was considered a ‘critical interaction point’ as it’s the most important functionality on the platform in order to find a video to watch. We asked the children to search for popular themes (Minecraft, King of Glory) or any terms that were most familiar to them. Here we observed how children made use of the search function and how they would choose and decide which video to click on from the search list (Task 2); 3). Selecting the next video to watch - here children were asked to wait for a current video to finish, and then show the researchers how they would decide what to watch next. Task 3 aimed to observe how children would normally react to such choices and examine their perceptions of such recommendation practices. For all these tasks, instead of focusing on discussing what was returned, we particularly focused on using these tasks to encourage children to recall their everyday experiences with the online video platforms and their feelings and motivations that drive their practices as much as possible. All apps were set in the default mode and we asked children about their setting preferences when they interacted with the app.
3.2. Part 2: Video scenarios
In the second part of the interview, we presented the children with two videos about the datafication practices on video platforms, capturing the data collection and data inference practices respectively (as shown in ).
The videos introduced a fictional character named Luoluo, a 12-year-old girl who likes to watch videos on a video platform, and how she learns about data collection and processing on this platform. Considering cooking is an essential part of the Chinese culture (Ma, Citation2015; Newman, Citation2004), we used the cooking process as a metaphor and compared the collection and processing of children’s data. Specifically, we compare the data collection process to combining various ingredients into a cooking pot. Similarly, we symbolize the data processing and analysis process by comparing it to the act of following different recipes and cooking processes. Finally, we compare the data referencing process to the act of cooking up various dishes.
Previous research showed that children as young as 5 can start to comprehend metaphors (Rowe et al., Citation2008), and that metaphors and stories are effective ways of building children’s understanding of abstract concepts (Billow, Citation1981; Cameron, Citation1996). Thus, we use the metaphor as a beginning point of our discussion. We also checked with several teachers beforehand to confirm if the videos were easy to interpret for children. Teachers confirmed that children are familiar with cooking and some of them use various platforms to search for cooking tutorials and watch related videos. When children watched videos during the interview, they were attracted to this metaphor. Some even immediately imagined the potential relatedness between cooking and AI before the video finished. They also used this metaphor to help them express their understanding of datafication in the interview.
Each video lasts about 1.5 minutes. After watching each video, children were invited to comment on specific plots presented in the video. A screen capture as well as the questions were presented to the children by the researchers so that children can recall the content, and we took care to express the questions in a language appropriate for the participant’s age and development.
3.2.1. Video 1 - General perception of datafication online
This video pictures the video platform (used most frequently by the participant) as ‘a cooking pot’ that requires access to a range of data, including videos we watched, terms we searched for, websites we visited, our friend lists, and our location (as ingredients for making dishes). After having watched the video, children were prompted to articulate on how they perceive the general datafication practices on the video platform. Specifically, whether they were surprised by or happy about the data being collected by the platform, and what do they think will happen to their data.
3.2.2. Video 2 - Perception of data inference online
This video provides more details about what happens inside the cooking pot of the platform. In this video, we used the metaphor of how a chef could infer on different people’s preferences and provide personalised cooking based on the ingredients threw into the pot. In this way, we focused on the data inference part of the datafication process - online platforms could learn more subtle things about users (apart from interests) based on their data. This refers to the likelihood that video platforms could infer about a child on a more personal level, such as inferring their age, socio-economic status, and more. Considering Chinese app ecosystem as mentioned above, we also described the cross-platform datafication as most children would be multi-app users (e.g., data inference could be based on not only data collected by video platforms but also from other platforms children have been using, such as DouYin etc). We then invited children to think about and articulate on the data inference practices: specifically, whether they were surprised by or happy about how the video platform use different types of data about them to recommend new videos, learn more about their personal life, or send them adverts more personalised to their interests.
4. Study method
Participants were recruited from local schools in Hangzhou, South East of China. Inviting emails were sent to local schools after we have received institutional ethical approval. Schools interested in our research offered us the opportunity to recruit volunteers for our interviews in their IT classes. 36 children were interviewed in person between October and November 2022. At each of these sessions, teachers helped children to set up their devices and then left the children with the researchers until the interview was completed. Each study was facilitated by at least two researchers.
4.1. Participant information
We made a careful selection on the age range of the children participants, setting it as from 11 to 14. We chose to work with this age group as various previous reports have shown that 11 to 14 is the key transition stage in China for children to become more heavily involved in online activities, especially gaming and social media platforms (Youth Rights and Interests Protection Department and China Internet Network Information Center, Citation2022). Among the 36 participant children (), 18 were boys and 18 were girls, with an average age of 12.5 (range = 11–14, s.d. = 0.98).
Table 1. Summary of participants’ ages and genders.
Apart from the age of participant children, we also made a careful selection to ensure the diversity of the demographic background of our participants. Children were recruited from diverse schools, locations (both urban and rural), socioeconomic statuses, and academic achievements. For individual participants, we also noted down their usage behaviours on social media platforms (online video and short video platforms), family background and education related to datafication (if mentioned), as well as school education on topics related to datafication (if mentioned). See and in Appendix for details on individual participant.
4.2. Study process
Each interview consisted of four parts, including an introduction session, Part 1 - a walk-through of tasks on video platforms they used most frequently, Part 2 - a walk-through of videos on datafication scenarios, and finally an open-ended session about children’s thoughts and needs as well as any issues not so far discussed. The whole study was planned to last approximately 1 hour. As the majority of study is based on interactions and tasks, it mitigated the power imbalance between adult researchers and children and gives children sufficient time to build rapport with the researchers.
Children were asked to choose their most used device when accessing video platforms to maximise the recreation of their everyday experience. In Part 1 of the study, children were given some time to read through the task sheet. One of the researchers read with them, and supported them with any technical questions throughout the process. As children were completing the tasks, researchers observed their activities and encouraged children to ‘think-aloud’ their interactions. Children were told there are no “right answers” to any tasks or questions. Due to the lack of a clear market dominance of Chinese online video platforms, we found that children were more familiar with platforms such as Bilibili, DouYin, Youku, Tencent Video, or KuaiShou. We adjusted the tasks according to the platforms children chose to interact with during the study. We made sure that such adjustments did not influence children’s responses as the core interactions (e.g., how to find a video, how videos are recommended) are very similar across all Chinese online video platforms.
Screen and audio recordings were taken during the studies. Screen recordings of children completing tasks were played back during the transcription and data analysis phases to highlight any notable patterns and then deleted immediately.
5. Data analysis method
All interviews were conducted in Mandarin. The interviews were transcribed and analysed through an open coding approach (Corbin & Strauss, Citation1990) by the first two authors (who are native Chinese speakers) to develop codes and themes reported and discussed by the children. All codes, themes and relevant quotes were then translated into English.
Results from part 1 of the study contained both children’s experiential descriptions of their own experiences of using video platforms (e.g., Bilibili, iQIYI, Youku Video, Tencent Video, DouYin, Kuaishou), and their perceptions of these tasks. We identified the experiential reflections mentioned by the children, and then carefully coded the specific synchronic elements mentioned (such as their emotions, sequence of actions, and information used to inform their actions) by the children during their completion of each specific task. In this way, we could gain a more in-depth understanding about how participant children currently managed critical interaction points on the platforms and what elements of knowledge were used by them. This gave us a set of codes about children’s usage patterns, their existing knowledge points, and their general expression of experiences about video platforms. Additionally, throughout our study, we noticed that children’s online experiencese have been heavily influenced by their families. This also gave us a set of codes about children’s online interaction with their families and friends.
With respect to the data from the video-guided interviews, we tried to calibrate how children perceived video platforms’ data processing practices, from the general datafication to the more specific data inference practices, by carefully examining their use of language, for example, how they described data and those who collected their data, what data and how the data was processed, as well as how they knew about such information(e.g., from parents, from siblings, from schools, from news, or came up with themselves).
We transcribed the interviews and analysed the data using a grounded, thematic approach Alhojailan (Citation2012), to develop codes and themes related to data processing practices reported and discussed by the children. The coding process started by dividing the transcriptions into three (roughly) equal-sized sets. The first two authors independently analysed the first set of transcriptions to derive an initial set of codes. Then they met to consolidate and reconcile codes. These codes were then applied to the second (yet unseen) set of transcriptions by the same set of researchers, they then met again to consolidate newly emerged codes, with a Cohen’s kappa of 0.79. This final set of codes is then used to code the remaining third set of the transcriptions.
6. Results
We present our results by first outlining children’s overall experience and usage of video platforms in general. We then present children’s general understanding of datafication online in terms of the collection and sharing of their data. We then present an in-depth analysis regarding children’s perceptions of the more specific data inference practices. While our participant children demonstrated different perceptions and varied level of understanding, we found no significant differences between children of different age or gender. We present individual children’s quotes with their participant id.
6.1. Children’s overall experience and usage
Most children (28/36) in our study did not own their own devices (phone, tablet, computer), and the majority of them (31/36) shared devices with their family members (parents, siblings, grandparents). Only a few children (7/36) would sign in to an account of their own in order to access the online platform, including those who paid for a membership (for videos of better image quality and membership-only video resources). A large proportion of children (14/36) reported sharing accounts with their family members (parents, siblings, grandparents). The remaining (15/36) accessed the platforms through visitor mode.
In terms of usage, many (21/36) children reported spending more than 8 hours per week on online video or short video platforms. Interestingly, unlike previous research which found children typically used video platforms (e.g., YouTube, TikTok) for entertainment (Common Sense Media and Rideout, Citation2011; Ofcom, Citation2022), children in our study demonstrated more varied platform usage. A child would use multiple online platforms for various aspects in their life, ranging from the use for watching cute and funny videos, use of YouKu Video and iQIYI (online video platforms) for their favourite TV series, use of RED (similar to Instagram) for finding the most trendy online “how to dos,” use of Tencent video (an online video platform) for learning purposes, to use of DouYin and Kuaishou (short video platforms) to learn about news and exciting stuffs around them.
Meanwhile, children demonstrated quite sophisticated online interactions. Children in our study would intentionally purchase memberships and some even tried to earn money in apps through uploading videos or completing tasks on apps. Many children also brought up how they formed online communities such as fan clubs and hobby groups through functions offered by platforms; some even reported they would continue to chat with people they met from these communities by adding them to their WeChat (similar to WhatsApp).
However, it is interesting to see that peer pressure was not as relevant as suggested by some previous literature about Western children (O'Keeffe et al., Citation2011; Sherman et al., Citation2016) – very few children in our study talked about how their friends influenced their social media use. Instead, we noticed that children’s social media use was heavily influenced by their families – their parents, siblings, and even their grandparents and cousins in a lot of cases. Many children reported sharing devices and accounts with their family members, and they also reported watching videos together with their family members (‘My granny and I share interests’ - P29) and being recommended or taught how to navigate social media platforms by their families (‘My brother showed me how to follow this channel and I think it’s pretty good’ - P15). Family members also shared their online experiences with each other (‘We chat a lot about what’s interesting on RED’ - P23). Likewise, we also observed controls being commonly imposed by family members, for instance, almost all children (31/36) mentioned how they were not “allowed to” either view content from a specific channel or stay too long on the platforms. Some children also mentioned how their decisions online would be influenced by family members (‘My sister uses DouYin all the time so I figured it should be quite good’ - P7).
6.2. Children’s general perceptions of datafication online
We present our findings on children’s general perceptions of datafication practices on video platforms mentioned, specifically, how children perceive what data is collected and what happens to these data.
6.2.1. Data collection: What is secret and owned by me
All children knew that information about them would be collected as they use the platforms. They exhibited different perceptions about what is private depending on the type of data. To start with, all children demonstrated some understanding of what data is and what means to be private, many coming from school and family education:
We learnt about what private information is in our IT class, your real name, your number, your home address etc. (P37)
Additionally, children were eager to share their data with their families.
My dad told me never to tell them (people online) my name and my bank PIN number, cause they could go to my bank and steal all my money. (P18)
I do not think sharing my data with my parents or families is data leakage. In fact, I should share my detailed location data with my parents to guarantee my safety. If my parents want to share my data with others, I may feel a little bit uncomfortable… But I think they will not. (P19)
On the other hand, children were less certain when it came to online behavioural data such as their watching history and searching history. In fact, many of them believed these data was not private to them, and a surprising portion of the children (23/36) thought it was either the platforms or even the content uploaders that owned their data. One possible explanation to this, as we observed from the way children expressed themselves, is that they seemed to struggle with recognising that records of their online activities were also a form of data that was being collected; they therefore had difficulty distinguishing between the content of a video itself and the behavioural data that contained information about which videos they had watched:
The uploaders worked hard on creating these videos, therefore they should own them? The fact that you watched these videos doesn’t make them yours? (P9)
To be honest I don’t think any of these are private info, even my location. I don’t really mind them knowing where I am cause it’s not a secret anyway. (P8)
6.2.2. Data as part of a process: (only) used to provide better services
Almost all children (32/36) in our study believed the only purpose for online platforms to collect their data was to offer better services for them. And they reported having quite positive experience when being recommended content they liked:
My sister and I share our device, they (platforms) would know what we liked and give us more of such contents. It’s really amazing! (P5)
I think it’s (datafication) for gaining more traffic. We (the whole family) sometimes watch streaming sessions together, and there’s tens of thousands of people in there! (P2)
They give you better videos, they have more audience on their platforms, and they get more money from their boss. It’s a win-win. (P35)
6.2.3. Data flow: Data won’t flow but stored somewhere
Most children (29/36) in our study believed data collected by a certain platform would only be used by that platform. And they generally found it confusing why different platforms would trade and share data with each other, thinking it’s technically infeasible, nor legally allowed:
I don’t think DouYin and Bilibili will share our data. My dad told me they’re enemies. (P26)
My data would not be collected as long as I’m not signed in. That’s why my grandpa didn’t allow me to sign up for one. (P24)
It’s gotta be stored somewhere, and that place will go old or become not big enough, and get abandoned after some time. (P1)
That means my data is in safe hands. (P10)
Maybe they (government) could merge all these data from different apps into one large big database and protect it. (P23)
6.3. Children’s perceptions of data inference online
Following children’s perceptions of the general datafication practices online, we further investigated their understanding and perceptions of the data inference practices online.
6.3.1. What is data inference: Video recommendations
To start with, most children described data inference as the process in which online social media platforms were trying to make predictions about them, some described this process as “making predictions about people” (P35). However, almost all children in our study believed such inference was made only to predict their interests, specifically on what type of videos they like to watch, without realising some more subtle things about them (e.g., their personal characteristics) could be inferred. In some cases, we noticed from their languages that they seemed to equate datafication to pure video recommendation:
Datafication is trying to predict what you might like, and recommend videos to you. (P2)
I just don’t want to see the same videos every day. It’s like how your mom cooks for you, tell her nothing you would most definitely only get chicken soup every day. (P20)
6.4. Personal data and big data
While most children thought datafication on social media platforms was just recommendation, and data inference was only about their interests in videos, interestingly, many children mentioned the term “Big Data” as they explained what datafication was. When children tried to articulate this concept, they demonstrated more awareness in terms of how data could be used to infer people’s characteristics in other contexts outside social media platforms. For instance, they described Big Data as doing “data analysis on a collection of a lot of people’s data” (P18), which could be used to learn about people’s personal details:
Big Data would get to know your age, what school you go to, where your parents go to work and many other things. (P7)
The textbook, the news… They all said Big Data and AI a national policy and should be greatly encouraged. It’s good for our country. (P11)
My grandma told me the Covid-tracing app is using big data, so that the government can know where everyone is, who’s sick and who needs help. (P32)
I’m willing to give out my data to help more people. It’s good for social development. (P36)
It brings convenience to our lives, and I think I can sacrifice some little things. One for all, all for one. (P30)
6.4.1. How is data inference conducted
In general, all children showed awareness that some kind of automated process was conducted for data inference, describing it as “an automated process,” “some kind of machine,” “a robot.” On the other hand, only a minority of children (9/36) specifically mentioned the term algorithm:
My dad once mentioned, they are using some kind of algorithm or something. (P22)
They (the developers who wrote the algorithms) can decide whatever each people get to see. (P10)
Only very few children (3/36) described the algorithms as using models, and showed an awareness that the output of the algorithm was not decided by human, but by the input data itself.
I don’t think they (developers) have any power over what ads you get, it only depends on what you watch every day. (P21)
6.4.2. How children perceive data inference practices
All children in our study commented on data inference in quite positive ways, partly because they often equated data inference to content recommendation, and therefore happy to receive what interests them. During the interviews, we also touched on topics around how stereotypical contents might be presented to different people purely based on information about inferred gender or economic status, such as how different products (e.g., dolls v.s. robotic toys) might target girls against boys (Zotos & Tsichla, Citation2014), and how adverts targeting at female consumers would use different colour tones (e.g., pink) (Grau & Zotos, Citation2016). Interestingly, children in our study seemed to be generally less concerned about such implications around data inference:
I think it’s pretty fair. They predict that girls might like dolls so they give them that. (P24)
My sister told me that the platform would offer some gifts cards for food if it had inferred that you wanted to order a takeaway. That’s great, isn’t it? (P29)
I like pink anyway. As long as they give you good stuff then it’s pretty fair. (P7)
One possible explanation for this and a key theme we observed is that children in our study often described datafication as making “predictions,” and thus associated fairness of data inference with the factual accuracy of the predictions, believing data inference is fair as long as it generated what people might like.
I would say this inference thing is fair as long as their predictions are accurate. And it is true that most boys prefer sports to girls, it’s just big data. (P36)
Meanwhile, children demonstrated more care for the actual content they receive and their actual experience online, and developed their perceptions of data inference accordingly. For instance, the only 2 children in our study who thought data inference could be unfair mentioned that they would feel being “looked down upon’ or “being discriminated” if they were not considered equal to others by the algorithms.
Yes, my mom once said WeChat would show different adverts to different ‘target users’. Like they would show BMW ads to some but not others. It’s like looking down upon people. (P3)
6.5. Desire for regulations and being informed
Many children demonstrated strong desires for improved data regulations and to be more informed on topics around datafication.
To start with, although at the start of the study, children were unaware of the more sophisticated datafication practices such as cross-platform data sharing/trading and how subtle things about them could be inferred. Children in our study demonstrated a strong desire to defend their own data rights online when being prompted to think about such topics, and how they would feel if these practices do exist. For instance, many of them believed data trading should be made illegal, and they became furious if any data crossing was conducted without their consent:
I never thought it’s (selling data to make money) possible before today? I would call 110 (the police number in China) if they do it behind my back. (P4)
My grandfather was almost scammed online when the person impersonated me and asked my grandfather to transfer money. As a result, my grandfather and I made a pact not to comment or reveal real information, post, pop-ups or chat on social media later on. I wonder what we could do further. (P33)
Some children (7/36) explicitly expressed their desire to defend for their data rights online through legislation efforts. They envisioned a series of regulations including requiring online social media platforms to gain users’ approval before any data practices, prohibiting platforms from inferring on people’s sensitive information or about vulnerable groups such as children:
There should be new laws on these companies, like you shouldn’t try to learn about whether that people is sick or not. I heard from my grandma that’s what Baidu was doing, sending fake hospital adverts to people with illnesses. (P36)
I would be really keen to have a list from them, like all the things they collect and how they use them. It’s all just very confusing now. (P5)
If they do learn more than just my interests, I would be creeped out. Does that also mean they can learn about my family as well? I would really want to see what they’re actually doing. (P11)
At school they just give a bunch of definitions, saying Big Data is used by the government and it’s good for social development and stuff, you fill that in for exams and that’s it. Would be good to know how that actually helps with our everyday lives. (P17)
7. Discussion
7.1. Key findings and contributions
In our study, children demonstrated some basic understanding of data collection, data processed through “automated process,” and the idea that datafication could be used to “make predictions on people.” On the other hand, our results align with previous findings on Western children, indicating that children may not always fully grasp online datafication practices (Livingstone et al., Citation2019; Pangrazio & Cardozo-Gaibisso, Citation2021; Stoilova et al., Citation2020). We identified three key knowledge gaps in children’s perception of datafication practices, including their lack of recognition of their data ownership, the transmission of data across platforms and the broader implications of datafication beyond video recommendation, such as inferences about their personal aspects. These findings provide significant contributions to our understanding of how Chinese children aged 11–14 perceive datafication in their daily interactions with digital platforms. Our research shed light on the challenges Chinese children face in fully comprehending the implications of data processing, many of which are influenced by the unique aspects of Chinese culture and digital landscape. These findings provided important future design directions for supporting the development of children’s digital literacy and digital autonomy in China.
7.2. Interpreting children’s perceptions under the Chinese context
Before delving into children’s digital literacy development and future design implications, we contextualise our findings within the Chinese context, considering both the cultural and institutional aspects. It’s important to note that our intention is not to conduct cross-cultural comparisons by referencing previous studies on children from different countries. Instead, we utilise existing research to demonstrate how our work contributes to the current understanding of how Chinese children perceive datafication in their context.
To begin with, many children’s responses reflected a strong element of pragmatism, as defined by Putnam (Citation1994) as “focus on the practical effectiveness and usefulness of ideas or actions, rather than theories or ideologies.” When comparing our findings with those of children in the same age group from other countries (Skinner et al., Citation2020; Solyst et al., Citation2023; Wang et al., Citation2022), distinct patterns emerged. For example, children in our study mostly described datafication as making “predictions.” They often equated fairness with the accuracy of recommendations and generally believed data inference was fair as long as it generated content people might like. As a result, they were generally less likely to be concerned about stereotypical recommendations. This perspective could partly be attributed to Chinese children’s general limited understanding of the implications of datafication; however, our observations indicated further nuances.
Chinese children often talked positively about the use of datafication and big data for achieving “collective good for the whole society” and were generally less sensitive to how datafication practices could have impacts on them as individuals. Such a perception was further reinforced by external influences. For instance, many children mentioned how datafication was usually portrayed positively at school and in the news, emphasising its benefits for “greater social development,” which echoes the overall positive framing of privacy, datafication, and related AI technologies in China. Digital technologies, such as Alpha-Go or COVID contact tracing, are often depicted positively and as non-threatening in the Chinese media, in contrast to the Western press (Curran et al., Citation2020). While technological advancements are highlighted for their positive contributions to society, concerns of their potential drawbacks for individuals are often downplayed or overlooked. These positive attitudes towards datafication may imply a form of dataism within both individuals and Chinese society – a concept encompassing the trust and belief that human activities can and should be subject to datafication (Adamczyk, Citation2023; Uribe, Citation2023). However, this study has not explored extensively how our participants perceive the role of data in shaping society and the world around us. A previous study comparing the privacy and AI laws in China with those of Europe also found that Chinese laws and regulations focused more on promoting the “collective good” (e.g., “upgrade of all industries and prevention of data monopoly”), whereas Western laws emphasises fairness and diversity for individuals from specific demographic groups (Fung & Etienne, Citation2023).
These differences may stem from the emphasis on pragmatism in Chinese culture, which prioritises “practical application operation and rapid result feedback” (Li and Wu, 1608). In fact, “Chinese practicality” or “practical pragmatism,” is a cultural and philosophical approach deeply rooted in Chinese society. It emphasises practicality, usefulness, and tangible results over abstract theories or ideologies. Chinese pragmatism is closely linked to traditional cultural values, including Confucianism and Daoism, which emphasize practical virtues, harmony, and balance. Pragmatism may influence how data privacy is regulated in China, how technologies may be adopted for rapid outcomes, and how users perceive the boundaries of privacy differently (e.g., limited family privacy boundary). Future design and privacy initiatives must consider these unique cultural traits to support Chinese children’s understanding of privacy implications.
Pragmatism also influences the role of families in Chinese children’s datafication experience, including the shared use of devices within the family, joint media engagement among family members (e.g., co-watching and discussing related topics), parental and family mediation (e.g., setting rules for the use of online platforms), and the share of knowledge between family members (e.g., “My mom/my brother told me…”). It is worth noting that, such influence extends beyond just parents to include siblings, and even grandparents.
Research with Western children has shown that while parental influence remains significant for children in the age group of 11–14, peer influence begins to play a more prominent role, making children more sensitive to interpersonal privacy risks (Stoilova et al., Citation2020). One potential explanation for this, supported by previous research on privacy perception in Chinese society (Chang & Chen, Citation2017; Chen & Cheung, Citation2018; Tang & Dong, Citation2006; Zhu et al., Citation2020), is that the perceptions of privacy among Chinese children and their families can be influenced by Confucian ethos, which places a high value on family and hierarchy. According to the Confucian philosophy, individuals are first considered members of a family or community within a hierarchical structure, rather than as independent individuals (Tang & Dong, Citation2006). This may explain why, in our study, children’s perceptions of datafication are more likely to be influenced by their families, as opposed to the more common observation among their Western peers (Wang & Ollendick, Citation2001).
7.3. Implications for digital literacy development
Children in our study demonstrated a rudimentary understanding of the datafication process (e.g., data collection, data inference, data transmission, etc.), and expressed their willingness to learn more. However, while children reported substantial learning-related experiences in families, schools and society, they lack understanding in key areas such as data ownership, cross-platform data transmission and the broader implications of datafication. This emphasises the necessity to design effective learning experiences for children aged 11 to 14 concerning datafication issues.
The constructivist theory emphasises the importance of constructing a learning experience for the learners and encouraging their independent knowledge development abilities, which provides a promising direction for addressing the knowledge gaps that we have identified in our study. This theory encourages a holistic approach of considering the facilitation of children’s learning experiences from a combination of three key aspects (Wilson, Citation2017): (1) the learning materials, for engaging the learning and teaching; (2) the learning strategies, for carrying out the education and knowledge transfer; and (3), the learning environment, which is crucial for achieving effective learning outcomes.
From our observations, existing approaches for supporting children’s digital literacy have rarely approached the design of learning experiences from all three aspects. For instance, Ali et al. (Citation2019) presented the ‘Popbots’ toolkit, a programmable social robot for kids, coupled with a curriculum on AI concepts, to enhance children’s algorithmic understanding; ‘DataMove’ (Brazauskas et al., Citation2021) lets kids explore data through physical movement and dance; and Hitron et al. (Citation2019) developed a platform where children can train ML systems with hand-held input gestures. While these tools provide effective support for children’s learning, they fell short of children’s needs to learn about datafication in different environments (e.g., family, school, etc.), from different materials (e.g., social media, real-life experiences, etc.), and by different strategies (e.g., collaborative learning, informal learning, etc.), emphasising the multi-perspective consideration of children’s learning experiences. Previous research emphasised the importance of multi-perspective consideration of children’s learning experiences (Bada & Olusegun, Citation2015; Hendry et al., Citation1999; Perkins, Citation2013), and we encourage educators and designers to consider enhancing the design of learning experiences related to datafication by drawing on the constructivism theory as a foundation.
Another crucial challenge we identified is that our children participants often struggled to recognise risk factors in their everyday scenarios even though they demonstrated a good understanding of ‘private’ data or ‘what recommendations mean’ in our study. Children exhibited limitations in applying and transferring their knowledge about datafication. Future designs for children’s digital literacy development should focus on either situational relevance or experiences that naturally engage children. Indeed, existing approaches such as Interland (Google, Citation2022) and strategies like Project-based learning (PBL) (Kokotsaki et al., Citation2016) have successfully applied these learning theories to enhance children’s understanding of online safety. Our research underscores the need for further investigations into supporting children’s active knowledge construction, particularly by situating these in contexts that resonate with them. Future research should explore strategies for enhancing digital literacy to boost children’s resilience against harmful online content and experiences.
Finally, our findings indicate the potential benefits of designing learning experiences that emphasise the role of families in the context of children’s education on datafication and their development of digital literacy skills. Children in our research described their school-based learning about datafication as being ‘exam-oriented’; on the other hand, their experiences and understanding of datafication were deeply woven into their families’ daily activities. This highlights the potential to incorporate learning experiences into home environment. Prior studies on familial learning experiences have shown that parents and caregivers can play a critical role in enhancing children’s understanding of digital technologies and literacy. However, these parents require additional support from educators and researchers to grasp the effective parenting strategies to assist their children. (Kumpulainen et al., Citation2020; McDougall et al., Citation2018; Öztürk, Citation2021). Family involvement in children’s learning extends beyond the home setting (Van Voorhis et al., Citation2013). Other initiatives, such as family workshops at schools and school-led family outreach, also contribute to children’s literacy development. For example, a 10-week digital workshop by Hébert et al. (Citation2022) helped parents and their children, aged 10 to 13, enhance their digital competency and storytelling skills. Both constructivist and social-constructivist theories have been successfully applied to family learning situations, underscoring the importance of social interactions and collaboration (Muhideen et al.; Cook-Cottone, Citation2004; Sterian & Mocanu, Citation2016).
7.4. Design future digital experiences for children in China
Our understanding of Chinese children’s current experience and perceptions indicates an urgent need to reconsider how future data-driven digital experiences should be designed for children, especially in terms of their need for more legibility and being informed of online datafication platforms.
To start with, children’s difficulty in recognising data transitions across platforms and the subsequent data inference and monetisation indicated how the lack of transparency of the current datafication approach on online platforms was damaging children’s development of identity (Mascheroni, Citation2020; Zhao et al., Citation2019), and their ability to effectively link such practices with data processing and inferences. As a result, we had the majority of the children believing that datafication was only for generating better videos for them and viewing it as a localised phenomenon. This pragmatic view may explain why children in our study tended to be pragmatic regarding the impact of datafication. They had seldom thought about the subtle and sensitive implications that could arise from their data. Our results show that once children were made aware of such implications, they reacted with confusion, frustration, and a strong desire for more information about datafication, especially how datafication practices were applied beyond video recommendations. Meanwhile, while children in our study demonstrated strong collectivist tendencies, it does not imply that they care less about their individual rights than their adult counterparts in China (Chen & Cheung, Citation2018; Nemati et al., Citation2014). Rather, our study showed that the Chinese children of this generation placed a higher value on their privacy and the potential sensitivity of their data. Once hinted about how datafication extends beyond video recommendations, children became quite sensitive to their digital/data rights online and came up with a variety of different ideas to defend themselves against the platform exploitation of their data.
How shall future online datafication platforms in China adapt to accommodate such needs and desires from children then? While some efforts have been made by online platforms in China for children such as introducing “child-friendly mode” (Xiaohongshu, Citation2021; Youku, Citation2019), such attempts were primarily oriented around children’s online safety issues, such as stranger danger and screen time control. Almost no efforts have been made by the Chinese service providers to support children to become more informed of their privacy rights and datafication practices.
To fill this essential gap we recommend that future designers in China pay closer attention to the unique challenges mentioned above while localising existing efforts from the Western online platforms in China. Such mechanisms include transparency mechanisms that empower children with a better understanding of key computational concepts through child-friendly ways, such as Lego’s Caption Safety (Lego, Citation2021) and Google’s Be Internet Legends (Google, Citation2021). There has also been an increased number of tools and technologies supporting children’s algorithmic thinking, such as the UNESCO Algorithm & Data Literacy Project (Digtal Moment et al., Citation2022) and Track This Platform (Hull, Citation2019). While these initiatives were typically designed for Western children, they could be great resources for designers in China. Meanwhile, our identification of the unique cultural traits of Chinese children also suggested that these Western effort would need to be adapted and localised in a Chinese context in order for them to become more effective. For instance, Chinese children’s emphasis on the practicality and factual accuracy of datafication, as well as the central role of families in their digital lives, suggest opportunities for designers to incorporate practical and real-world datafication examples and enhance family involvement in educating children about datafication.
Finally, while our study did not initially intend to look into how cultures might impact children’s perception of datafication, our research revealed that children from distinct cultural backgrounds, such as China’s pragmatic, collective, and family-centric culture, exhibit unique characteristics in their understanding and perception of privacy and datafication concepts. Such observations echo many previous cultural-specific studies (although not specifically for children and datafication), which suggest that populations from Arab, Africa, East Asia, South Asia and other regions would all have different and unique perceptions of online privacy (Kaya & Weber, Citation2003; Krasnova et al., Citation2012; Mohammed & Tejay, Citation2017). Much research on human behaviour and psychology assumes that everyone shares the fundamental cognitive and affective processes, and that findings from one population apply across the board. However, growing evidence has suggested that this is not the case (Henrich et al., Citation2010). Our findings provide a great example of how children in China may face unique challenges of when dealing with datafication practices online. We therefore call for attention to more cultural-specific studies, recognising the full extent of human diversity when building towards a more ethical and informed data society for all of us.
8. Limitations and future work
There are several important limitations of this work; the first pertains to the sample size of the study population. Firstly, the schools whose children participated may already be more interested and cautious about datafication practices than the average population, which is likely to significantly impact children’s understanding and perceptions. While we did not collect information about participants’ family income, the families’ areas of residence were centred around Hangzhou, a city that is home to some of China’s biggest technology companies. This might mean our findings reflect higher digital literacy or a more positive attitude towards technology compared to the broader population.
Meanwhile, we based our analysis on children’s self-report data, and children may have moderated their responses according to how the questions were asked. We attempted to mitigate this through several ways, such as ensuring that children knew there were no “right answers” to whichever task or question they were presented with, using language appropriate for the participant’s age and development, and carefully choosing our wording to avoid nudging children towards particular responses or understandings.
Finally, datafication is a broad topic related to a variety of different issues - ranging from monetisation, behavioral manipulation, belief shaping, and more. In this study, we were only able to focus on social media platforms specifically in the form of online video platforms. Although we chose these platforms because of their significant role in children’s online experiences, our choice is likely to have shaped our results relating to datafication, focusing primarily on issues most relevant to these platforms. Studies using different apps (e.g., games or online shopping) may yield additional perspectives and address issues complementary to those we identified.
Future work aims to explore how we may design approaches to enable children to expand their knowledge about datafication practices and develop ways to support children’s algorithmic literacy development. We intend to run co-design workshops (Kumar et al., Citation2018; McNally et al., Citation2018) with children as follow-up studies, which may investigate design options to stimulate critical thinking on data-related issues among children.
9. Conclusion
As children are growing up in an age of datafication, their data are now being routinely used to profile, analyse and make predictions about them. Children’s actions online are not only recorded, tracked, and aggregated, but also analysed and monetised. Such practices are difficult to understand, even for adult users, let alone children. This paper is the first to contribute to an understanding of how Chinese children perceive datafication and the more specific data inference practices that dominate their online information consumption. By contextualising these observations within the framework of Chinese culture and philosophy, we identified specific cultural traits in Chinese children’s perceptions of datafication. Drawing on critical education theories, we discuss what future digital literacy support for Chinese children should look like. Our findings provide fresh insights into Chinese children’s perception of datafication within their unique online ecosystem and offer crucial inputs regarding the need for more attention to cultural-specific considerations in future child-computer interaction research.
Disclosure statement
No potential conflict of interest was reported by the author(s).
Additional information
Notes on contributors
Yumeng Zhu
Yumeng Zhu is a Ph.D. student majored in educational technology at the College of Education, Zhejiang University, PR China. She is also a recognized student of the University of Oxford, Computer Science Department, Human-Centered Computing Group. Her research interests include AI in education, human-centered computing, etc.
Ge Wang
Ge Wang is a recent DPhil graduate from the Department of Computer Science at Oxford University. Her research investigates the algorithmic impact on families and children, and what that means for their long-term development. She is a recipient of multiple best paper awards at the premier HCI academic venues.
Yan Li
Yan Li is a professor at Zhejiang University, PR China, and Vice Dean of the College of Education. She directs the Research Centre for AI in Education and the Department of Curriculum and Learning Sciences. Her research interests include distance education, ICT education, AI in education, and educational innovations.
Jun Zhao
Jun Zhao is a senior research fellow at the Department of Computer Science of Oxford University. She is the founder and director of the Oxford Child-Centred AI Design Lab. Her research focuses on investigating the impact of AI on our everyday lives, with a particular emphasis on young children.
Notes
1 “流量” in Chinese.
2 Also known as “Health Code”, the Covid-19 tracing app was introduced in China in 2020. Without Health Code, citizens in China cannot access any public transport or public areas over the COVID pandemic. The app collects the real name, ID number, COVID test results, and a tracking record of users’ locations in the past 15 or up to 30 days (Liang, Citation2020).
References
- Acker, A., & Bowler, L. (2017). What is your data silhouette? Raising teen awareness of their data traces in social media [Paper presentation]. Proceedings of the 8th International Conference on Social Media & Society (pp. 1–5). Association for Computing Machinery. https://doi.org/10.1145/3097286.3097312
- Adamczyk, C. L. (2023). Communicating dataism. Review of Communication, 23(1), 4–20. https://doi.org/10.1080/15358593.2022.2099230
- Agrawal, D., Bernstein, P., Bertino, E., Davidson, S., Dayal, U., Franklin, M., Gehrke, J., Haas, L., Halevy, A., Han, J., et al. (2011). Challenges and opportunities with big data 2011-1 (Cyber Center Technical Reports. Paper 1). http://docs.lib.purdue.edu/cctech/1
- Alhojailan, M. I. (2012). Thematic analysis: A critical review of its process and evaluation. In WEI International European Academic Conference Proceedings, Zagreb, Croatia. https://citeseerx.ist.psu.edu/document?repid=rep1&type=pdf&doi=0c66700a0f4b4a0626f87a3692d4f34e599c4d0e
- Ali, S., Payne, B. H., Williams, R., Park, H. W., & Breazeal, C. (2019). Constructionism, ethics, and creativity: Developing primary and middle school artificial intelligence education. In International Workshop on Education in Artificial Intelligence k-12 (eduai’19) (pp. 1–4).
- Alneyadi, S., Abulibdeh, E., & Wardat, Y. (2023). The impact of digital environment vs. traditional method on literacy skills; reading and writing of emirati fourth graders. Sustainability, 15(4), 3418. https://doi.org/10.3390/su15043418
- Altman, I. (1977). Privacy regulation: Culturally universal or culturally specific? Journal of Social Issues, 33(3), 66–84. https://doi.org/10.1111/j.1540-4560.1977.tb01883.x
- Bada, S. O., & Olusegun, S. (2015). Constructivism learning theory: A paradigm for teaching and learning. Journal of Research & Method in Education, 5(6), 66–70. https://doi.org/10.9790/7388-05616670
- BBC News. (2021). China: Children given daily time limit on douyin - its version of tiktok.
- Benvenuti, M., Wright, M., Naslund, J., & Miers, A. C. (2023). How technology use is changing adolescents’ behaviors and their social, physical, and cognitive development. Current Psychology, 42(19), 16466–16469. https://doi.org/10.1007/s12144-023-04254-4
- Billow, R. M. (1981). Observing spontaneous metaphor in children. Journal of Experimental Child Psychology, 31(3), 430–445. https://doi.org/10.1016/0022-0965(81)90028-X
- Brazauskas, J., Lechelt, S., Wood, E., Evans, R., Adams, S., McFarland, E., Marquardt, N., & Rogers, Y. (2021). Datamoves: Entangling data and movement to support computer science education [Paper presentation]. Designing Interactive Systems Conference 2021 (pp. 2068–2082). https://doi.org/10.1145/3461778.3462039
- Büchi, M., Fosch-Villaronga, E., Lutz, C., Tamò-Larrieux, A., Velidi, S., & Viljoen, S. (2020). The chilling effects of algorithmic profiling: Mapping the issues. Computer Law & Security Review, 36, 105367. https://doi.org/10.1016/j.clsr.2019.105367
- Cameron, L. (1996). Discourse context and the development of metaphor in children. Current Issues in Language and Society, 3(1), 49–64. https://doi.org/10.1080/13520529609615452
- Cao, J. (2009). The analysis of tendency of transition from collectivism to individualism in china. Cross-Cultural Communication, 5(4), 42–50. http://dx.doi.org/10.3968/j.ccc.1923670020090504.005
- Chang, C. L-h., & Chen, J. Q. (2017). The information ethics perception gaps between Chinese and American students a Chinese guanxi perspective. Information Technology & People, 30(2), 473–502. https://doi.org/10.1108/ITP-08-2014-0181
- Chen, Z. T., & Cheung, M. (2018). Privacy perception and protection on Chinese social media: A case study of wechat. Ethics and Information Technology, 20(4), 279–289. https://doi.org/10.1007/s10676-018-9480-6
- China Internet Network Information Center. (2022). The 49th report about the development of the internet in china.
- China National Children’s Center and Tencent. (2022). Minors’ network media literacy behavior analysis report.
- Common Sense Media and Rideout, V. (2011). Zero to eight: Children’s media use in America. Common Sense Media.
- Cong, L. W., & Li, S. (2021). A model of influencer economy. Available at SSRN 3974661.
- Cook-Cottone, C. (2004). Constructivism in family literacy practices: Pararents as mentors. Reading Improvement, 41(4), 208–217. https://link.gale.com/apps/doc/A127158660/AONE?u=anon∼f64ffb20&sid=googleScholar&xid=c9a9bfde
- Corbin, J. M., & Strauss, A. (1990). Grounded theory research: Procedures, canons, and evaluative criteria. Qualitative Sociology, 13(1), 3–21. https://doi.org/10.1007/BF00988593
- Couldry, N., & Mejias, U. A. (2019). The costs of connection. Stanford University Press.
- Cunningham, S., Craig, D., & Lv, J. (2019). China’s livestreaming industry: Platforms, politics, and precarity. International Journal of Cultural Studies, 22(6), 719–736. https://doi.org/10.1177/1367877919834942
- Curran, N. M., Sun, J., & Hong, J.-W. (2020). Anthropomorphizing alphago: A content analysis of the framing of google deepmind’s alphago in the chinese and american press. AI & Society, 35(3), 727–735. https://doi.org/10.1007/s00146-019-00908-9
- DataReportal. (2022). Digital around the world.
- Diggelmann, O., & Cleis, M. N. (2014). How the right to privacy became a human right. Human Rights Law Review, 14(3), 441–458. https://doi.org/10.1093/hrlr/ngu014
- Digtal Moment, CCUNESCO, and UNESCO. (2022). The algorithmic and data literacy project.
- Fung, P., & Etienne, H. (2023). Confucius, cyberpunk and Mr. science: Comparing ai ethics principles between china and the EU. AI and Ethics, 3(2), 505–511. https://doi.org/10.1007/s43681-022-00180-6
- Gibson, S. E. (2006). Using webquests to support the development of digital literacy and other essential skills at the k-12 level. In Handbook of research on literacy in technology at the K-12 Level (pp. 322–336). IGI Global.
- Google. (2021). Be internet legends.
- Google. (2022). Interland.
- Grau, S. L., & Zotos, Y. C. (2016). Gender stereotypes in advertising: A review of current research. International Journal of Advertising, 35(5), 761–770. https://doi.org/10.1080/02650487.2016.1203556
- Han, W., Gao, Y., & Deng, A. (2018). Algorithmic price discrimination on online platforms and antitrust enforcement in china’s digital economy. Antitrust Source. https://ssrn.com/abstract=3250075
- Hébert, C., Thumlert, K., & Jenson, J. (2022). # digital parents: Intergenerational learning through a digital literacy workshop. Journal of Research on Technology in Education, 54(1), 34–91. https://doi.org/10.1080/15391523.2020.1809034
- Hendry, G. D., Frommer, M., & Walker, R. A. (1999). Constructivism and problem-based learning. Journal of Further and Higher Education, 23(3), 369–371. https://doi.org/10.1080/0309877990230306
- Henrich, J., Heine, S. J., & Norenzayan, A. (2010). Most people are not weird. Nature, 466(7302), 29–29. https://doi.org/10.1038/466029a
- Hitron, T., Orlev, Y., Wald, I., Shamir, A., Erel, H., & Zuckerman, O. (2019). Can children understand machine learning concepts? The effect of uncovering black boxes. In Proceedings of the 2019 CHI Conference on Human Factors in Computing Systems (pp. 1–11).
- Hull, L. (2019). Hey advertisers, track this.
- Hung, K. (2020). Celebrity and influencer in a fan economy: Unfolding the fans’ roles in enhancing endorsement effects. In Multidisciplinary perspectives on media fandom (pp. 323–340). IGI Global.
- Information Commissioner’s Office. (2020). What is automated individual decision-making and profiling?
- International Monetary Fund. (2023). Regional economic outlook. Asia and Pacific: challenges to sustaining growth and disinflation.
- Kang, H., Shin, W., & Huang, J. (2021). Teens’ privacy management on video-sharing social media: The roles of perceived privacy risk and parental mediation. Internet Research, 32(1), 312–334., https://doi.org/10.1108/INTR-01-2021-0005
- Kaya, N., & Weber, M. J. (2003). Cross-cultural differences in the perception of crowding and privacy regulation: American and turkish students. Journal of Environmental Psychology, 23(3), 301–309. https://doi.org/10.1016/S0272-4944(02)00087-7
- Kaye, D. B. V., Chen, X., & Zeng, J. (2021). The co-evolution of two Chinese mobile short video apps: Parallel platformization of douyin and tiktok. Mobile Media & Communication, 9(2), 229–253. https://doi.org/10.1177/2050157920952120
- Kazai, G., Yusof, I., & Clarke, D. (2016). Personalised news and blog recommendations based on user location, facebook and twitter user profiling [Paper presentation]. Proceedings of the 39th International ACM SIGIR Conference on Research and Development in Information Retrieval (pp. 1129–1132). ACM. https://doi.org/10.1145/2911451.2911464
- Khan, N., Yaqoob, I., Hashem, I. A. T., Inayat, Z., Ali, W. K. M., Alam, M., Shiraz, M., & Gani, A. (2014). Big data: Survey, technologies, opportunities, and challenges. The Scientific World Journal, 2014(1), 712826. https://doi.org/10.1155/2014/712826
- Kokotsaki, D., Menzies, V., & Wiggins, A. (2016). Project-based learning: A review of the literature. Improving Schools, 19(3), 267–277. https://doi.org/10.1177/1365480216659733
- Krasnova, H., Veltri, N. F., & Günther, O. (2012). Self-disclosure and privacy calculus on social networking sites: The role of culture. Business & Information Systems Engineering, 4(3), 127–135. https://doi.org/10.1007/s12599-012-0216-6
- Kumar, P., Naik, S. M., Devkar, U. R., Chetty, M., Clegg, T. L., & Vitak, J. (2017). ’no telling passcodes out because they’re private’: Understanding children’s mental models of privacy and security online. Proceedings of the ACM on Human-Computer Interaction, 1(CSCW), 1–21. https://doi.org/10.1145/3134699
- Kumar, P., Vitak, J., Chetty, M., Clegg, T. L., Yang, J., McNally, B., & Bonsignore, E. (2018). Co-designing online privacy-related games and stories with children [Paper presentation]. Proceedings of the 17th ACM Conference on Interaction Design and Children (pp. 67–79). ACM. https://doi.org/10.1145/3202185.3202735
- Kumpulainen, K., Sairanen, H., & Nordström, A. (2020). Young children’s digital literacy practices in the sociocultural contexts of their homes. Journal of Early Childhood Literacy, 20(3), 472–499. https://doi.org/10.1177/1468798420925116
- Lego. (2021). Captain safety.
- Li, T., & Wu, Y. (2016/08). Pragmatism in China - Chinese pragmatism [Paper presentation]. Proceedings of the 2016 International Conference on Humanity, Education and Social Science (pp. 40–43). Atlantis Press. https://doi.org/10.2991/ichess-16.2016.9
- Liang, F. (2020). COVID-19 and health code: How digital platforms tackle the pandemic in china. Social Media + Society, 6(3). https://doi.org/10.1177/2056305120947657
- Liu, S., Perry, P., & Gadzinski, G. (2019). The implications of digital marketing on wechat for luxury fashion brands in china. Journal of Brand Management, 26(4), 395–409. https://doi.org/10.1057/s41262-018-0140-2
- Livingstone, S., Stoilova, M., & Nandagiri, R. (2019). Children’s data and privacy online: Growing up in a digital age: An evidence review. London School of Economics and Political Science, Department of Media and Communications. https://eprints.lse.ac.uk/101283/
- Lupton, D., & Williamson, B. (2017). The datafied child: The dataveillance of children and implications for their rights. New Media & Society, 19(5), 780–794. https://doi.org/10.1177/1461444816686328
- Ma, G. (2015). Food, eating behavior, and culture in Chinese society. Journal of Ethnic Foods, 2(4), 195–199. https://doi.org/10.1016/j.jef.2015.11.004
- Ma, Y. (2023). Relatedness and compatibility: The concept of privacy in mandarin Chinese and American English Corpora. Journal of the Association for Information Science and Technology, 74(2), 249–272. https://doi.org/10.1002/asi.24728
- Maes, C., & Vandenbosch, L. (2022). Adolescent girls’ instagram and tiktok use: Examining relations with body image-related constructs over time using random intercept cross-lagged panel models. Body Image, 41(2022), 453–459. https://doi.org/10.1016/j.bodyim.2022.04.015
- Malvini Redden, S., & Way, A. K. (2017). ‘Adults don’t understand’: Exploring how teens use dialectical frameworks to navigate webs of tensions in online life. Journal of Applied Communication Research, 45(1), 21–41. https://doi.org/10.1080/00909882.2016.1248465
- Mascheroni, G. (2020). Datafied childhoods: Contextualising datafication in everyday life. Current Sociology, 68(6), 798–813. https://doi.org/10.1177/0011392118807534
- McDougall, B. S., McDougall, B. S., & Hansson, A. (2002). Chinese concepts of privacy (Vol. 55). Brill Academic Pub.
- McDougall, J., Readman, M., & Wilkinson, P. (2018). The uses of (digital) literacy. Learning, Media and Technology, 43(3), 263–279. https://doi.org/10.1080/17439884.2018.1462206
- McNally, B., Kumar, P., Hordatt, C., Mauriello, M. L., Naik, S., Norooz, L., Shorter, A., Golub, E., & Druin, A. (2018). Co-designing mobile online safety applications with children [Paper presentation]. Proceedings of the 2018 CHI Conference on Human Factors in Computing Systems (pp. 523). ACM. https://doi.org/10.1145/3173574.3174097
- Mejias, U. A., & Couldry, N. (2019). Datafication. Internet Policy Review, 8(4), 1–10. https://doi.org/10.14763/2019.4.1428
- Mioduser, D., & Levy, S. T. (2010). Making sense by building sense: Kindergarten children’s construction and understanding of adaptive robot behaviors. International Journal of Computers for Mathematical Learning, 15(2), 99–127. https://doi.org/10.1007/s10758-010-9163-9
- Mohammed, Z. A., & Tejay, G. P. (2017). Examining privacy concerns and ecommerce adoption in developing countries: The impact of culture in shaping individuals’ perceptions toward technology. Computers & Security, 67(2017), 254–265. https://doi.org/10.1016/j.cose.2017.03.001
- Muhideen, S., Iddrisu, I., Mohammed, J., Okagbue, E. F., Iddrisu, S., & Akintunde, T. Y. The family use of mobile applications at home: A constructivism theory approach. https://ssrn.com/abstract=4421662
- Naftali, O. (2009). Empowering the child: Children’s rights, citizenship and the state in contemporary china. The China Journal, 61(61), 79–103. https://doi.org/10.1086/tcj.61.20648046
- Naftali, O. (2010). Caged golden canaries: Childhood, privacy and subjectivity in contemporary urban china. Childhood, 17(3), 297–311. https://doi.org/10.1177/0907568209345612
- National Center for Education Statistics. (2023). Children’s internet access at home. Condition of education.
- Nemati, H., Wall, J. D., & Chow, A. (2014). Privacy coping and information-sharing behaviors in social media: A comparison of Chinese and us users. Journal of Global Information Technology Management, 17(4), 228–249. https://doi.org/10.1080/1097198X.2014.978622
- Newman, J. M. (2004). Food culture in China. Greenwood Publishing Group.
- Ofcom. (2020). Ofcom children’s media lives: Life in lockdown.
- Ofcom. (2022). Children and parents: media use and attitudes report 2022.
- O'Keeffe, G. S., & Clarke-Pearson, K, on Communications, C., and Media (2011). The impact of social media on children, adolescents, and families. Pediatrics, 127(4), 800–804. https://doi.org/10.1542/peds.2011-0054
- Oulasvirta, A., & Hornbæk, K. (2016). Hci research as problem-solving [Paper presentation]. Proceedings of the 2016 CHI Conference on Human Factors in Computing Systems (pp. 4956–4967). https://doi.org/10.1145/2858036.2858283
- Öztürk, M. (2021). An embedded mixed method study on teaching algebraic expressions using metacognition-based training. Thinking Skills and Creativity, 39(2021), 100787. https://doi.org/10.1016/j.tsc.2021.100787
- Pangrazio, L., & Cardozo-Gaibisso, L. (2021). Chapter 2 “Your data can go to anyone”: The challenges of developing critical data literacies in children. In Critical digital literacies (pp. 35–51). Brill. https://doi.org/10.1163/9789004467040_003
- Perkins, D. N. (2013). What constructivism demands of the learner. In Constructivism and the technology of instruction (pp. 161–165). Routledge.
- Pernot-Leplay, E. (2020). China’s approach on data privacy law: third way between the US and the Eu? Penn State Journal of Law and International Affairs, 8(1), 49–117. https://elibrary.law.psu.edu/jlia/vol8/iss1/6
- Putnam, H. (1994). Pragmatism. Blackwell Publishers.
- Rachayu, I., Masitoh, S., & Nursalim, M. (2022). The digital literacy on constructivist philosophical aspect. Cendikia: Media Jurnal Ilmiah Pendidikan, 13(2), 211–216. https://iocscience.org/ejournal/index.php/Cendikia/article/view/3009
- Rao, A., Schaub, F., & Sadeh, N. (2015). What do they know about me? Contents and concerns of online behavioral profiles. arXiv preprint arXiv:1506.01675.
- Rowe, M. L., Ozçalişkan, S., & Goldin-Meadow, S. (2008). Learning words by hand: Gesture’s role in predicting vocabulary development. First Language, 28(2), 182–199. https://doi.org/10.1177/0142723707088310
- Sax, M. (2016). Big data: Finders keepers, losers weepers? Ethics and Information Technology, 18(1), 25–31. https://doi.org/10.1007/s10676-016-9394-0
- Sherman, L. E., Payton, A. A., Hernandez, L. M., Greenfield, P. M., & Dapretto, M. (2016). The power of the like in adolescence: Effects of peer influence on neural and behavioral responses to social media. Psychological Science, 27(7), 1027–1035. https://doi.org/10.1177/0956797616645673
- Skinner, Z., Brown, S., & Walsh, G. (2020). Children of color’s perceptions of fairness in ai: An exploration of equitable and inclusive co-design [Paper presentation]. Extended Abstracts of the 2020 CHI Conference on Human Factors in Computing Systems (pp. 1–8).
- Solyst, J., Xie, S., Yang, E., Stewart, A. E., Eslami, M., Hammer, J., & Ogan, A. (2023). “I would like to design”: Black girls analyzing and ideating fair and accountable ai [Paper presentation]. Proceedings of the 2023 CHI Conference on Human Factors in Computing Systems, CHI ’23. Association for Computing Machinery. https://doi.org/10.1145/3544548.3581378
- Statista. (2022). Number of mobile apps available for download on the app stores in China from 2017 to June 2022.
- Statista Research Department. (2022). Number of internet and social media users worldwide as of July 2022(in billions).
- Sterian, M., & Mocanu, M. (2016). Family, education and social constructivism. Euromentor Journal, 7(3), 99. https://www.ceeol.com/search/article-detail?id=531116
- Stoilova, M., Livingstone, S., & Nandagiri, R. (2020). Digital by default: Children’s capacity to understand and manage online data and privacy. Media and Communication, 8(4), 197–207. https://doi.org/10.17645/mac.v8i4.3407
- Tang, S., & Dong, X. (2006). Parents’ and children’s perceptions of privacy rights in china: A cohort comparison. Journal of Family Issues, 27(3), 285–300. https://doi.org/10.1177/0192513X05283095
- Tempornsin, J., Chaijaroen, S., & Somabut, A. (2019). The design and development of constructivist web-based learning environment framework to enhance digital literacy for higher education. In International conference on innovative technologies and learning (pp. 469–476). Springer.
- The World Bank. (2023). The World Bank in China.
- Uribe, D. A. (2023). A new religion for the 21st century: An interpretation of ‘dataism’ from the sociology of pierre bourdieu. The Journal of Psychology & Sociology, 85(2), 122–133. https://doi.org/10.26577/JPsS.2023.v85.i2.012
- Van Voorhis, F. L., Maier, M. F., Epstein, J. L., & Lloyd, C. M. (2013). The impact of family involvement on the education of children ages 3 to 8: A focus on literacy and math achievement outcomes and social-emotional skills. MDRC.
- Wachter, S. (2020). Affinity profiling and discrimination by association in online behavioral advertising. Berkeley Technology Law Journal, 35(2), 367–430. https://www.jstor.org/stable/27121752
- Wang, G., Zhao, J., Van Kleek, M., & Shadbolt, N. (2022). ‘Don’t make assumptions about me!’: Understanding children’s perception of datafication online. Proceedings of the ACM on Human-Computer Interaction, 6(CSCW2), 1–24. https://doi.org/10.1145/3555144
- Wang, G., Zhao, J., Van Kleek, M., & Shadbolt, N. (2023). ‘Treat me as your friend, not a number in your database’: Co-designing with children to cope with datafication online [Paper presentation]. Proceedings of the 2023 CHI Conference on Human Factors in Computing Systems, CHI ’23. Association for Computing Machinery. https://doi.org/10.1145/3544548.3580933
- Wang, L. H., & Metzger, M. J. (2021). The role of culture in privacy management on social media among emerging adult children and their parents. Journal of International and Intercultural Communication, Pages, 16(2), 162–190. https://doi.org/10.1080/17513057.2021.1993966
- Wang, Y., & Ollendick, T. H. (2001). A cross-cultural and developmental analysis of self-esteem in Chinese and western children. Clinical Child and Family Psychology Review, 4(3), 253–271. https://doi.org/10.1023/a:1017551215413
- Warren, S. D., & Brandeis, L. D. (1890). The right to privacy. Harvard Law Review, 4(5), 193–220. http://links.jstor.org/sici?sici=0017-811X%2818901215%294%3A5%3C193%3ATRTP%3E2.0.CO%3B2-C
- Wilson, B. G. (2017). Constructivism for active, authentic learning. In B. Reiser & J. Dempsey (Eds.), Current trends in instructional design and technology (4th ed., pp. 61-Draft chapter). Pearson Prentice Hall.
- Xiaohongshu. (2021). Xiaohongshu children/youth personal information protection rules.
- Yao-Huai, L. (2005). Privacy and data privacy issues in contemporary china. Ethics and Information Technology, 7(1), 7–15. https://doi.org/10.1007/s10676-005-0456-y
- Ye, J., He, L., & Beestrum, M. (2023). Implications for implementation and adoption of telehealth in developing countries: A systematic review of china’s practices and experiences. NPJ Digital Medicine, 6(1), 174. https://doi.org/10.1038/s41746-023-00908-6
- Youku. (2019). Youku children’s personal information protection rules.
- Youth Rights and Interests Protection Department and China Internet Network Information Center. (2022). 2021 national research report on internet use by minors.
- Yu, L., Suo, F., Zhu, S., Lu, C., & Wu, D. (2021). Research on the construction and application of information literacy evaluation model for middle and high school students-taking the fourth and fifth grade students as an example (in Chinese). China Audio-Visual Education, 2021(5), 63–69. https://kns.cnki.net/kcms2/article/abstract?v=m22VhQxdXyctNck2O7OIpJsPyzlGOyf7cr7LqfcAz_oqtGRrvoBg2pXqjHQkC5viljsbnxqdRZSm8eQrK9E1YHS6ehZIEnRBTtZKvPRLnnQ92_m_6A_UamgW8e3SToki8RXy_RFxtb7aA_Mq6WRs6A==&uniplatform=NZKPT&language=CHS
- Zarouali, B., Ponnet, K., Walrave, M., & Poels, K. (2017). “Do you like cookies?” adolescents’ skeptical processing of retargeted facebook-ads and the moderating role of privacy concern and a textual debriefing. Computers in Human Behavior, 69(2017), 157–165. https://doi.org/10.1016/j.chb.2016.11.050
- Zhao, J., Wang, G., Dally, C., Slovak, P., Edbrooke-Childs, J., Van Kleek, M., & Shadbolt, N. (2019). ‘I make up a silly name’: Understanding children’s perception of privacy risks online. In Proceedings of the 2019 CHI conference on human factors in computing systems, CHI ’19 (pp. 1–13). Association for Computing Machinery.
- Zheng, W. (2015). Investigation and analysis of information needs and utilization of teenagers-based on the investigation of 7 middle schools in Maoming, Guangdong Province (in Chinese). Library and Information Service, 59(19), 68–74+99. http://doi.org/10.13266/j.issn.0252-3116.2015.19.009
- Zhu, R., Srivastava, A., & Sutanto, J. (2020). Privacy-deprived e-commerce: The efficacy of consumer privacy policies on china’s e-commerce websites from a legal perspective. Information Technology & People, 33(6), 1601–1626. https://doi.org/10.1108/ITP-03-2019-0117
- Zotos, Y. C., & Tsichla, E. (2014). Female stereotypes in print advertising: A retrospective analysis. Procedia-Social and Behavioral Sciences, 148(2014), 446–454. https://doi.org/10.1016/j.sbspro.2014.07.064
- Zuboff, S. (2019). The age of surveillance capitalism: The fight for a human future at the new frontier of power: Barack Obama’s books of 2019. Profile books.