ABSTRACT
Particulate matter ≤10 μm (PM10) emissions due to wind erosion can vary dramatically with changing surface conditions. Crust formation, mechanical disturbance, soil texture, moisture, and chemical content of the soil can affect the amount of dust emitted during a wind event. A refined method of quantifying windblown dust emissions was applied at Mono Lake, CA, to account for changing surface conditions. This method used a combination of real-time sand flux monitoring, ambient PM10 monitoring, and dispersion modeling to estimate dust emissions and their downwind impact. The method identified periods with high emissions and periods when the surface was stable (no sand flux), even though winds may have been high. A network of 25 Cox sand catchers (CSCs) was used to measure the mass of saltating particles to estimate sand flux rates across a 2-km2 area. Two electronic sensors (Sensits) were used to time-resolve the CSC sand mass to estimate hourly sand flux rates, and a perimeter tapered element oscillating microbalance (TEOM) monitor measured hourly PM10 concentrations. Hourly sand flux rates were related by dispersion modeling to hourly PM10 concentrations to back-calculate the ratio of vertical PM10 flux to horizontal sand flux (K-factors). Geometric mean K-factor values (K f) were found to change seasonally, ranging from 1.3 × 10−5 to 5.1 × 10−5 for sand flux measured at 15 cm above the surface (q 15). Hourly PM10 emissions, F, were calculated by applying seasonal K-factors to sand flux measurements (F = K f × q 15). The maximum hourly PM10 emission rate from the study area was 76 g/m2·hr (10-m wind speed = 23.5 m/sec). Maximum daily PM10 emissions were estimated at 450 g/m2·day, and annual emissions at 1095 g/m2·yr. Hourly PM10 emissions were used by the U.S. Environmental Protection Agency (EPA) guideline AERMOD dispersion model to estimate downwind ambient impacts. Model predictions compared well with monitor concentrations, with hourly PM10 ranging from 16 to over 60,000 μg/m3 (slope = 0.89, R 2 = 0.77).
Under a U.S. Environmental Protection Agency (EPA)-approved plan, the method described in this paper has been used since 2000 at Owens Lake, CA, to identify and successfully mitigate dust from over 100 km2 of the dry lakebed. It continues to be used to monitor dust control compliance at Owens Lake. Scaled-down versions of the Owens Lake network can be implemented in other areas in a manner similar to the Mono Lake study. Once K-factors are established, low-cost CSC samplers (about $35 U.S.) may be used for periodic monitoring (e.g., daily, weekly, or monthly) to estimate PM10 emissions or to evaluate dust control compliance.
INTRODUCTION
The exposed shoreline at Mono Lake, CA, is a large source of windblown dust. The highest 24-hr average particulate matter ≤10 μm (PM10) concentration in the United States in 2008 was measured at Mono Lake (2769 μg/m3).Citation1 The highest 24-hr average PM10 concentration measured at Mono Lake was 14,147 μg/m3 on November 20, 2009. This is almost 100 times higher than the federal PM10 standard of 150 μg/m3. The highest hourly PM10 concentration on that day was 65,112 μg/m3 with 10-m wind speeds over 23 m/sec (50 miles per hour). More recently in 2010, windblown dust caused 22 monitored violations of the federal PM10 standard on the north shore of Mono Lake. The photo in shows the solar-powered PM10 TEOM monitor site on the north shore at Mono Lake. A dust plume typical of windblown dust generated from the exposed playa can be seen in the background.
Exposure of the lakebed at Mono Lake to wind erosion was primarily caused by the diversion of water from the Mono Basin by the City of Los Angeles from 1941 through 1989. This resulted in lowering the lake level and exposing over 24 km2 of the lakebed to wind erosion. An air quality model analysis in 1994 showed that a lake level of 6391 feet above mean sea level would submerge enough of the exposed shoreline that windblown dust would be sufficiently reduced to attain the federal PM10 standard.Citation2 In 1995, the California State Water Resources Control Board used the air quality model results and other environmental analyses related to aquatic impacts to set a lake level elevation of 6391 feet as a management target and limited the City of Los Angeles' water diversions until that lake level is attained.Citation3 The lake level rose after the Water Board decision, but never reached the 6391 foot target, which likely explains why the exposed lakebed continued to cause federal PM10 standard violations. The highest lake level since the management target was set was 6385 feet in 1999 and 2006.Citation4
The 1994 air quality model used PM10 emissions from portable wind tunnel tests taken at Mono Lake in 1993.Citation5 The model gave good predictions for locations near the PM10 monitor sites that existed in 1993. In 2000, a new PM10 monitor was placed at the highest impact location at Mono Lake, which indicated significant model underprediction. Monitoring data from the new site at Mono Shore showed that actual PM10 concentrations for the largest events were about 7 times higher than model predictions at this new site.Citation6
To improve the air quality model for Mono Lake, a refined method of estimating PM10 emissions and air quality impacts was implemented. This refined method was patterned after the Owens Lake Dust Identification Program (Dust ID) that has been used at Owens Lake, CA, since 2000.Citation7 The Dust ID method uses a combination of continuous monitoring of saltation (or sand) flux, ambient PM10 monitoring, and dispersion modeling to estimate hourly PM10 emissions. It has been used at Owens Lake to identify and mitigate windblown dust from over 100 km2 of dry lakebed. This federally approved methodCitation8 continues to be used at Owens Lake to identify new areas for control and to monitor compliance in areas that are mitigated by Best Available Control Measures for windblown dust.
The primary advantage of the Dust ID method over the wind tunnel method used in the 1994 Mono Lake model is that emissions vary hourly in response to changes in erosion rates detected by electronic devices that measure sand flux and seasonally by changes in the ratio of vertical PM10 flux to horizontal sand flux. Since sand flux and PM10 measurements are taken continuously after instruments are placed in the field, all of the windblown dust events during the sampling period are sampled. The method identifies periods with high emissions and periods when the surface is stable (no sand flux), even though winds may be high. Sand flux measurements taken from different areas can identify spatial variations in erosion, and areas with zero sand flux provide evidence that the areas did not contribute PM during an event. This latter point may be useful to help settle disputes regarding the PM contribution from suspected dust source areas, such as agricultural fields or natural desert areas. In contrast to the Dust ID method, wind-tunnel-based PM10 emissions, as well as traditional emission estimation methods recommended by the U.S. Environmental Protection Agency (EPA) in AP-42, are primarily a function of wind speed as opposed to sand flux. They usually use a single emission algorithm for a given soil texture, and rely on the investigator to know when a surface is in a state that might be stable and not susceptible to wind erosion.Citation9 The actual surface soil texture may be nonuniform and the PM emission potential can vary temporally with surface changes that may be caused by mechanical disturbance, precipitation, or surface crust formation. The Dust ID method provides information on the spatial and temporal changes in surface conditions and dust emissions that is usually not available to investigators who use wind tunnel emission estimation methods.
THEORY
During a wind erosion event sand-sized particles creep and saltate across the surface, and finer particles, such as PM10, are lofted and can be carried many kilometers downwind. Because saltating particles move relatively short distances during a wind event, measurements of horizontal sand flux indicate the amount of wind erosion taking place near measurement sites. The Dust ID method is based on theoretical and experimental evidence that the vertical flux of PM10 is proportional to the horizontal flux of sand-sized particles. Shao et al.Citation10 theorized that the ratio of PM10 to sand flux tends to be constant for soils with the same binding energy. However, the binding energy of soils with similar texture and chemistry will change if surface moisture and temperature cause the soil to become more erodible or to form a crust and become stable. Gillette et al.Citation11 applied this relationship between PM10 and sand flux to estimate PM10 emissions at Owens Lake. They related horizontal sand flux to the downwind PM10 concentration using the EPA nonsteady-state puff model CALPUFF dispersion model to calculate PM10 emissions, and seasonal and spatial K-factors to account for changes in the proportionality constant related to changes in the binding energy of soil particles. The vertical flux of hourly PM10 emissions generated from the dust source, F (g/cm2·s), were calculated using Equationeq 1:
K-factors were calculated for every hour with active sand flux in areas upwind of a PM10 monitor. Hourly K-factors were screened to remove hours that did not have strong source–receptor relationships between the active source area and the downwind PM10 monitor. Screening criteria excluded hours when the edge of a dust plume may have impacted a PM10 monitor site. There was less confidence in the source–receptor relationship in this case because the edge of a dust plume has a very high concentration gradient, a few degrees error in the plume direction could greatly affect the model-predicted impact and therefore, the calculated K-factor. K-factor screening criteria included (1) hourly modeled and monitored PM10 both greater than 150 μg/m3, and (2) sand flux greater than 2 g/cm2·hr in at least one cell that was located within ±15° upwind from a monitor site.
METHODS
A monitoring network including 25 Cox sand catchers (CSCs), two Sensits, one meteorological tower, and one TEOM PM10 monitor was operated on the north shore of Mono Lake as shown in The meteorological tower and PM10 TEOM monitor were located in cell 14, and two Sensits were collocated with CSCs in cells 1 and 17. The CSCs measured sand flux for 25 subareas within the 2-km2 study area. The subarea boundaries shown in were based on the proximity to adjacent sand catchers, soil texture, and topographical features on the playa. Some of the boundaries were based on the location of terraced banks that were formed by wave action at a time when the lake level was higher and others on differences in the sand grain size, recognizing that coarser sands were at higher elevations on the playa. Sampling took place from July 1, 2009, through June 30, 2010. Hourly sand flux, wind speed, wind direction, and PM10 concentrations were measured during the study period.
Hourly Sand Flux
Mass measurements from CSCs were time-resolved with electronic Sensit readings to calculate the hourly sand flux. CSCs are passive instruments that are used to collect sand-sized particles that blow across the surface during dust events (). Sensits electronically measure the particle count and kinetic energy of sand-sized particles as they saltate across the surface. Previous studies have shown that Sensit readings as particle count or kinetic energy are linearly proportional to the CSC sand catch for the same period.Citation11 EquationEquation 3 was used to time-resolve the sand catch from each of the 25 CSC sites to calculate the hourly sand flux at each site:
pcqi;c = sand flux (at 15 cm height) for hour i at CSC site c (g/cm2hr)
CSC p;c = sand catch mass for period p at CSC site c(g)
PC i;s = Sensit particle counts for hour i, with n number of hours during period p at Sensit site s (closest Sensit Sensit to CSC site c) (counts)
1.2 = effective inlet area of CSC (cm2)
CSCs were provided by the Great Basin Unified Air Pollution Control District and cost about $35 (U.S.) to produce. CSCs have no moving parts, are durable, and can collect sand for months without overloading the sampling tube. Sampling tubes can be made in longer lengths (usually 60 to 120 cm) to increase the sample collection volume in areas with high sand flux rates. CSCs and Sensits were placed with the midpoint of their inlet or sensor positioned at 15 cm above the surface, as shown in the photo in Because of sand deposition and deflation around the sand flux sites, the CSC inlets and Sensit sensor heights were checked and adjusted if necessary during site visits to maintain the inlets at 15 cm (±1 cm) above the surface. CSC sampling tubes were collected and replaced monthly and taken to the laboratory for accurate mass measurements. Note for other investigators: more frequent site visits may be necessary to maintain the sampling height in areas with significant soil inflation or deflation, such as in sand dune areas. The battery-powered Sensits were augmented with solar charging systems. A data logger recorded hourly Sensit data during inactive periods and switched to 5-min data during active erosion periods. Each Sensit site costs about $3,500 (U.S.); $1,900 for the Sensit and $1,600 for the data logger, power supply and other materials.
A previous study comparing CSCs to BSNE (Big Spring Number Eight) samplers used by the United States Department of Agriculture for wind erosion research showed that CSCs had better measurement precision: ±3% for CSCs as opposed to ±8% for BSNEs.Citation11 This comparison was also used to establish the effective area of the inlet opening of CSCs to calculate sand flux. The CSCs circular design does not have an obvious inlet area size for a flux calculation (see and ), whereas BSNEs have rectangular inlet dimensions that can be used to calculate sand flux (mass/area). By comparing the CSC sand catch mass to the BSNE flux, the CSC was found to have an effective inlet area of 1.2 cm2.Citation7 This inlet opening area is incorporated in the flux calculation in Equationeq 3.
PM10 and Meteorological Data
Hourly PM10 concentration, wind speed, and wind direction data were collected from a site near the northern boundary of the sand flux monitoring network. The TEOM PM10 monitor is a federal equivalent method sampler for measuring PM10.Citation12 Operating procedures for the PM10 monitor and meteorological data collection followed EPA guidelines (40 CFR, Part 58).
Dispersion Modeling
The AERMOD dispersion modeling system was applied following EPA modeling guidance (40 CFR, Part 51, Appendix W). AERMOD is a steady-state plume dispersion model that is intended to be used to support air quality analyses for new sources and State Implementation Plans in the United States. The source area configuration for each dust source area was applied as shown in with the boundaries of the source areas represented by each CSC configured to account for surface features and different soil textures. The 25 area sources were modeled using the AREAPOLY area source algorithm/option in AERMOD. Unlike the gridded source areas used in the CALPUFF model for the Owens Lake Dust ID Program, AREAPOLY is a precise area source algorithm for modeling emissions from irregular shaped area sources. PM10 emissions from each dust source area were first estimated by applying the hourly sand flux in Equationeq 3 to estimate PM10 emissions in Equationeq 1 with an initial K-factor, K i, of 5 × 10−5. These hourly emissions were applied to the model source areas along with hourly wind speed, direction, and sigma-theta. Receptor locations for model predictions included the PM10 monitor site.
Hourly model predictions were compared to PM10 monitor concentrations to calculate hourly K-factors as shown in Equationeq 2. Composite K-factors for the study area were calculated for each hour with erosion activity in one or more monitored cells. The hourly background PM10 concentration was estimated at 16 μg/m3. This was based on PM10 concentrations measured on the southwest side of the lake in Lee Vining, CA, when hourly average winds were greater than 7.5 m/sec and from the south. Because there were no significant PM10 sources between the Lee Vining monitor and the study area, this is a good estimate of the average background concentration and is well below the model and monitor concentrations (>150 μg/m3) that were used to estimate K-factors in Equationeq 2.
K-factor Screening and Seasonal K-factors
Hourly K-factors derived from Equationeq 2 were screened to remove hours that did not have strong source–receptor relationships between the monitored dust source areas and the downwind PM10 monitor based on the following criteria:
• | Wind speed greater than 5 m/sec at 10-m anemometer height. | ||||
• | Hourly modeled and monitored PM10 concentrations both greater than 150 μg/m3. | ||||
• | Hourly wind direction between 120° and 300°. | ||||
• | Hourly average sand flux at all sites greater than 0.5 g/cm2·hr. |
Screened hourly K-factors were used to generate seasonal K-factors by looking for shifts in K-factor values. The use of seasonal K-factors provides a longer-term stable value that helps to compensate for uncertainty in hourly K-factors associated with sand flux estimates, dispersion model assumptions, and PM10 monitor measurements, and it provides K-factor values for events when hourly K-factors do not pass the screening criteria. For this study, seasonal K-factors were based on the geometric mean value of K-factors during each period. The accumulated hourly K-factors are fit with a geometric mean because they tend to have a log-normal distribution, in part because they are calculated from a ratio of the monitored and modeled concentrations (see Equationeq 2). As discussed later in this paper, the geometric mean provided a good balance of underprediction and overprediction of hourly PM10 concentrations, with most hourly model predictions being within a factor of 2 of the observed monitor concentrations. K-factors based on 1- to 2-day wind events can also be applied as opposed to seasonal K-factors. Event-specific K-factors are less stable than seasonal K-factors. They varied by a factor of 2 or more from the seasonal K-factors in this study. Although event-specific K-factors weren't used for this study, they tend to improve model performance for those events.
PM10 Emissions and Revised Dispersion Model Concentrations
Hourly PM10 vertical flux emissions from each source area can be calculated by applying seasonal K-factors to Equationeq 1:
It is not necessary to rerun the dispersion model to incorporate emissions based on the seasonal K-factors into the revised model predictions. Model results can be recalculated using Equationeq 2 to relate the initial and seasonal K-factor to the initial and revised model results:
RESULTS
Ambient PM10 Monitor Concentrations
There were 25 violations of the 24-hr federal PM10 standard of 150 μg/m3 during the 1-yr study period. The highest 24-hr average concentration was 14,147 μg/m3 on November 20, 2009. The highest hourly PM10 concentration was 65,113 μg/m3 on the same day. There were 259 hr with monitored hourly average PM10 concentrations greater than 150 μg/m3, which is one of the screening criteria used to calculate hourly K-factors.
Sand Flux
Hourly sand flux rates were calculated from the Sensit and CSC data using Equationeq 3. A plot of daily average sand flux at the 25 CSC sites versus time is shown in The log plot of the daily sand flux data shows the high variability of wind erosion that spans over a 4 order of magnitude range. The plot shows a notable gap in sand flux from December 2009 to March 2010. During this period the measured sand flux was zero (a value that cannot be plotted on a log graph). Although there were high winds during this period, the study area had no wind erosion because the surface was either covered with snow or the soil had enough moisture that it was stable.
A comparison of daily PM10 concentrations to the daily average sand flux at the 25 CSC sites is shown in the log-log plot in The observed linear relationships between sand flux and PM10 supports the theory that PM10 emissions are proportional to sand flux. Although this relationship between sand flux and PM10 does not consider the K-factor value or variations in sand flux across the study area, it provides an estimate of the amount of sand flux that could cause a monitored PM10 violation even before the dispersion modeling analysis is completed. In terms of potential PM10 impacts, daily sand flux of around 25 g/cm2 corresponded to daily average PM10 monitor concentrations that could violate the 24-hr federal PM10 standard of 150 μg/m3.
K-factors
Hourly K-factors were calculated using Equationeq 2 and screened using the previously described criteria to ensure strong source–receptor relationships. The hourly K-factors are plotted versus time in Several seasonal K-factor cutpoints were selected based on apparent shifts in K-factor values. The geometric mean K-factor values ranged from 1.3 × 10−5 to 5.1 × 10−5. The highest seasonal K-factors were in the fall and spring periods. This is a time when cold temperatures and moist soil conditions cause an efflorescent salt to form on the surface of the playa. Efflorescent salt formation occurs seasonally at Owens and Mono Lakes and is associated with the largest PM10 dust events. The efflorescent salts turn the surface white, and as the salts dry they form a fragile crust with an underlying dry powdery dust. These surfaces are easily eroded by saltating sand and agglomerated crustal material.Citation7,Citation13 The lack of K-factors from December 2009 through March 2010 is primarily due to the fact that the surface was stable and sand flux was zero during much of this period. The geometric mean K-factor is carried through this entire period and is applied to any hour with non-zero sand flux to calculate PM10 emissions using Equationeq 4.
PM10 Emissions
Seasonal K-factors were applied to the hourly sand flux to calculate hourly PM10 emissions using Equationeq 4. The maximum hourly PM10 vertical flux was 76 g/m2·hr. During this peak-emission hour, the hourly average wind speed was 23.5 m/sec. Maximum daily PM10 emissions were 450 g/m2·day on November 20, 2009. It is interesting to note that emissions for this one day represented 40% of the annual emissions, which were estimated at 1095 g/m2·yr.
To visualize how much PM10 is emitted each year, one can look at the depth of soil loss represented by the PM10 emissions. The depth of soil loss can be estimated by dividing the PM10 vertical flux by the bulk soil density. Assuming PM10-sized particles were not redeposited in the study area and the bulk soil density was 1.5 g/cm3 (based on sandy soils at Owens Lake, CACitation14), the PM10 emissions would represent an average depth of soil loss of 0.7 mm per year across the study area.
Hourly average PM10 emissions from the study area are plotted as a function of wind speed in Emissions were calculated from hourly sand flux and seasonal K-factors using the geometric mean values shown in The Mono Lake wind tunnel PM10 emissions algorithmCitation5 that was originally used to model PM10 at Mono Lake is plotted on the same graph to show the contrast between assuming windblown dust emissions as a simple function of wind speed and the scatter in actual emissions versus wind speed. It should be noted that wind tunnel emission algorithms are normally derived from a limited number of tests. In this case, there were only 6 data points to derive the wind tunnel algorithm, as compared to 355 data points for the Dust ID method as shown in this plot. This semilog plot does not show hours with zero emissions for which there were 8020 hr during the 1-yr study period.
Figure 9. Hourly average PM10 emissions versus wind speed using the Dust ID method and the portable wind tunnel emissions algorithm.
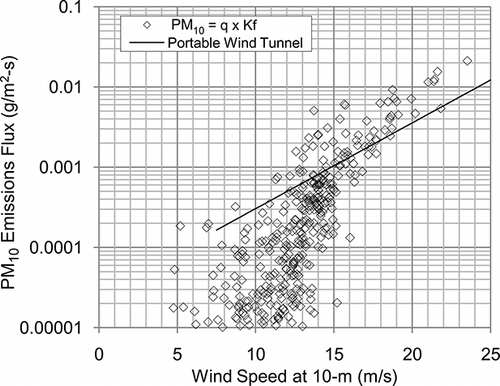
PM10 emissions from each of the 25 source areas in can be determined for each hour, and therefore, for any period using Equationeq 1 and the appropriate sand flux, K-factor and area size for each source area. Although it was not done for this analysis, the ambient PM10 impact from individual source areas can be extracted from the dispersion model output. Estimates of the PM10 contribution from each source area to the ambient PM10 concentration at model receptor locations can be used to determine the highest contributions from each area for control strategy evaluation purposes. This source contribution analysis is done annually at Owens Lake to determine if there are new dust source areas that should be controlled.Citation7
Comparison of PM10 Model and Monitor Concentrations
There is no standardized measurement method to ascertain the accuracy of PM10 emission estimates for windblown dust, but one way to evaluate the validity of emission estimates from this study is to utilize the emissions in a dispersion model and see how well they predict monitored concentrations. Revised hourly PM10 model predictions using Equationeq 5 were compared to PM10 TEOM monitor concentrations. Hourly PM10 model predictions for the monitor site location were paired with monitored concentrations for the same hours, so that model predictions and monitored observations were paired in both space and time. Because the study area was the only PM10 emissions source used in the model, data pairs were screened to evaluate only hours when winds were from the study area toward the monitor site (120° to 300°). To balance under- and overprediction statistics for lower concentrations, data pairs were excluded if the sum of the model predicted (P) and observed (O) monitor concentrations were less than 150 μg/m3. The 150 μg/m3 level was selected as an hourly screen for the statistics to focus on the higher predictions and observations. Using the sum P + O > 150 as opposed to just P > 150 or just O > 150 trims the data pairs symmetrically about the P = O line. Both severe over- and underprediction can be examined when the data pairs are trimmed in this fashion. For example, if the analysis only required O > 150, then it would leave out all the cases where the model over predicts when observed concentrations are small. In the x-y scatter plot shown in , 60% of the hourly model concentrations were predicted within a factor of 2 (dashed lines) higher or lower than the monitored concentrations. includes a statistical analysis of the hourly results displayed in Model predictions compared well with monitor concentrations, with hourly PM10 ranging from 16 to over 60,000 μg/m3 (slope = 0.89, R 2 = 0.77). An example of model predictions and monitor concentrations for the November 20, 2009, event is shown in PM10 model predictions tracked favorably with hourly monitor concentrations over a 4 order of magnitude range for this event. The model-predicted 24-hr average concentration was 16,062 μg/m3 as compared to the monitored concentration of 14,147 μg/m3.
Table 1. Hourly model performance statistics July 2009 to June 2010
DISCUSSION
The use of sand flux measurements to detect when wind erosion is taking place provides measurable evidence of erosion activity. By relating sand flux measurements to PM10 emissions through the use of K-factors, a realistic estimate of the amount of dust produced during wind events can be made. Alternative methods of estimating dust emissions are based on wind tunnel tests or other field studies that rely on the user to know when the surface is in an erodible condition. These alternative methods estimate emissions using wind speed, and other parameters that may be difficult to determine, such as threshold wind speed, which may vary with seasonal surface conditions. Even when wind tunnel tests are performed in the same area, the assumptions in the emissions model may cause significant errors, such as estimating emissions when there are none. Sand flux measurements can provide direct evidence of the magnitude of erosion and when surfaces are susceptible to wind erosion as shown in The Mono Lake portable wind tunnel PM10 emissions algorithm that was originally used to model PM10 at Mono Lake underestimated monitored impacts for the large events at this site by about a factor of 7.Citation6 The use of the sand flux-based hourly emission rates from this study significantly improved model predictions.
PM10 emissions using Equationeq 1 are mostly affected by changes in the sand flux rate. Daily sand flux measurements typically ranged from 0.1 to over 1000 g/cm2 (i.e., more than 4 order of magnitude difference in the daily sand flux). In contrast, there was relatively little change in the seasonal K-factor, which ranged from 1.3 × 10−5 to 5.1 × 10−5 (i.e., a factor of 4 difference). K-factors measured during this study were in the same range as K-factors measured in the Keeler dunes and other sandy areas at Owens Lake, which range from 1.5 × 10−5 to 7.4 × 10−5.Citation7 Soil texture appears to be an important variable that affects K-factor values. Measurements at Owens Lake found that K-factors for sandy areas are normally lower than for silt and clay soils and may be about 10% of that for surfaces that form efflorescent salts.Citation11 The similarity in K-factor values for sandy soils measured at Owens Lake and Mono Lake provides evidence that this K-factor range could also be appropriate for other locations with sandy soils. If so, PM10 emissions could be estimated using Equationeq 1 with sand flux data collected from the area of interest and K-factors defaulting to values measured at Owens Lake and Mono Lake.
For readers interested in comparing sand flux measurements with other studies, it should be noted that sand flux can be measured at 15 cm above the surface, as it was in this study, or as total sand flux. Total sand flux is the integrated saltation particle mass that passes through a vertical plane of infinite height and defined width (mass/length). Total sand flux can be calculated by measuring sand flux at multiple heights, fitting the data to a mathematical curveCitation11,Citation15 and then integrating from 0 to 1 m, which is the region where most of the saltation flux occurs. For relatively flat terrain, the flux at a given height is proportional to the total sand flux. The proportion of sand flux at 15 cm can be determined by integrating the sand flux from 14.5 to 15.5 cm and comparing it to the total sand flux. Long-term measurements using multiheight BSNE samplers at Owens LakeCitation11 found that the relationship of the total sand flux, Q, to the sand flux at 15 cm was
This same relationship was confirmed by another study in a coastal dune area in California.Citation16
The method described in this study can be applied in varying degrees of complexity. The most complex application is at Owens Lake, which has over 180 Sensit/CSC sites, 11 PM10 TEOM sites, and 14 meteorological sites, covering a 300-km2 area. The Owens Lake network is used to determine seasonal and spatial K-factors for different areas of the lakebed and to determine dust control compliance under the state implementation plan. The CALPUFF dispersion model for Owens Lake is run daily and sand flux and PM10 model results can be viewed on the Internet.Citation17 This method has worked successfully at Owens Lake for a variety of soil textures that included silt and clay dominated soils and soils that formed efflorescent salts; therefore this method is not restricted to sandy soils such as those at Mono Lake. The Mono Lake study was a modest application of the methodology, both in terms of the 2-km2 size of the area covered and the number of measurement sites. Simpler applications of the method could be done using only CSCs to provide evidence of erosion in mechanically disturbed areas or natural areas. CSCs could also be used to determine compliance with dust control regulations if an emissions threshold is set based on sand flux measurements. If K-factors are available for similar soil textures, then sand flux measurements could be used to estimate PM10 emissions without going through the more rigorous PM10 monitoring and modeling steps.
CONCLUSION
The results of this study showed that PM10 emission estimates based on sand flux and K-factors provided better dispersion model results and downwind predictions than wind tunnel-based emissions that were measured for the same surface. Hourly sand flux measurements showed that wind erosion is not a simple function of wind speed, as presumed by wind tunnel-based AP-42 emissions algorithms, but it can be affected by changes in the surface condition that are not easily predicted and make erosion rates highly variable. The results also showed that the ratio of PM10 to sand flux, known as the K-factor, changed seasonally at Mono Lake, with higher K-factors occurring during periods when efflorescent salts formed on the surface.
ACKNOWLEDGMENTS
The authors gratefully acknowledge Christopher Lanane, Nik Barbieri, and Bill Cox at the Great Basin Unified Air Pollution Control District for lending their vast experience to set up the monitoring network at Mono Lake, and Kyle Heitkamp and Benjing Sun of ENVIRON International Corp. for performing portions of the dispersion modeling analysis. Without their help this project would not have been possible. The authors are also grateful to the Center for the Study of Open Source Emissions, led by Dr. Chatten Cowherd, for the valuable feedback and support from its members to push this measurement method forward to a wider audience.
REFERENCES
- U.S. Environmental Protection Agency. AirData: Access to Air Pollution Data http://www.epa.gov/air/data/ (http://www.epa.gov/air/data/) (Accessed: 6 May 2011 ).
- McCulley , Frick and Gilman , Inc . 1994 . Final Mono Lake Air Quality Modeling Study , Lynnwood , WA : McCulley, Frick, and Gilman, Inc. .
- State of California Water Resources Control Board . 1994 . Mono Lake Basin Water Right Decision 1631 , Sacramento , CA : State of California Water Resources Control Board .
- Mono Lake Committee. Mono Lake Levels 1979 to Present http://www.monolake.org/today/water (http://www.monolake.org/today/water) (Accessed: 6 May 2011 ).
- Ono , D. March 23 1993 . Mono Lake Modeling Emission Algorithm , March 23 , Bishop , CA : memo to Ken Richmond, Great Basin Unified Air Pollution Control District .
- Great Basin Unified Air Pollution Control District . 2010 . Reasonable Further Progress Report for the Mono Basin PM-10 State Implementation Plan , 6 Bishop , CA : Great Basin Unified Air Pollution Control District .
- Great Basin Unified Air Pollution Control District . 2008 . 2008 Owens Valley PM10 Planning Area Demonstration of Attainment State Implementation Plan , Bishop , CA : Great Basin Unified Air Pollution Control District .
- U.S. Environmental Protection Agency . 2010 . Approval and Promulgation of Implementation Plans; Designation of Areas for Air Quality Planning Purposes; State of California PM-10 Redesignation of the Coso Junction Planning Area to Attainment; Approval of PM-10 Maintenance Plan for the Coso Junction Planning Area . Fed. Regist. , 75 : 54031 – 54033 .
- U.S. Environmental Protection Agency. Miscellaneous Sources; Emissions Factors & AP42 Compilation of Air Pollutant Emission Factors; Chapter 13 http://www.epa.gov/ttn/chief/ap42/ (http://www.epa.gov/ttn/chief/ap42/) (Accessed: 25 February 2011 ).
- Shao , Y. , Raupach , M.R. and Findlater , P.A. 1993 . Effect of Saltation Bombardment on the Entrainment of Dust by Wind . J. Geophys. Res. , 98 : 12719 – 12726 .
- Gillette , D. , Ono , D. and Richmond , K. 2004 . A Combined Modeling and Measurement Technique for Estimating Windblown Dust Emissions at Owens (Dry) Lake, California . J. Geophys. Res. , 109 : F01003 doi: 10.1029/2003JF000025
- U.S. Environmental Protection Agency. List of Designated Reference and Equivalent Methods; EQPM-1090-079; U.S. EPA: Research Triangle Park, NC http://www.epa.gov/ttnamti1/criteria.html (http://www.epa.gov/ttnamti1/criteria.html) (Accessed: 25 February 2011 ).
- 1995 . Mono Basin Planning Area PM-10 State Implementation Plan , Bishop , CA : Great Basin Unified Air Pollution Control District .
- Soil and Water West, Inc . May 2000 . Owens Lake Bed Soil Survey , May , Bishop , CA : Report Prepared for the Great Basin Unified Air Pollution Control District .
- Shao , Y. and Raupach , M.R. 1992 . The Overshoot and Equilibrium of Saltation . J. Geophys. Res. , 97 : 20559 – 20564 .
- Craig , J. , Cahill , T. and Ono , D. February 2010 . “ Appendix B: San Luis Obispo County Air Pollution Control District ” . In South County Phase 2 Particulate Study , February , San Luis Obispo , CA : San Luis Obispo County Air Pollution Control District .
- Owens Lake Yesterday; Great Basin Unified Air Pollution Control District: Bishop, CA http://www.gbuapcd.org/owenslake/yesterday/index.htm (http://www.gbuapcd.org/owenslake/yesterday/index.htm) (Accessed: 6 May 2011 ).