Abstract
Multipollutant indicators of mobile source impacts are developed from readily available CO, NOx, and elemental carbon (EC) data for use in air quality and epidemiologic analysis. Two types of outcome-based Integrated Mobile Source Indicators (IMSI) are assessed. The first is derived from analysis of emissions of EC, CO, and NOx such that pollutant concentrations are mixed and weighted based on emission ratios for both gasoline and diesel vehicles. The emission-based indicators (IMSIEB) capture the impact of mobile sources on air quality estimated from receptor models and their uncertainty is comparable to measurement and source apportionment uncertainties. The IMSIEB have larger correlation between two different receptor sites impacted by traffic than single pollutants, suggesting they are better indicators of the local impact of mobile sources. A sensitivity analysis of fractions of pollutants in a two-pollutant mixture and the inclusion in an epidemiologic model is conducted to develop a second set of indicators based on health outcomes. The health-based indicators (IMSIHB) are weighted combinations of CO, NOx, and EC pairs that have the lowest P value in their association with cardiovascular disease emergency department visits, possibly due to their better spatial representativeness. These outcome-based, multipollutant indicators can provide support for the setting of multipollutant air quality standards and other air quality management activities.
Integrated mobile source indicators (IMSI) were developed and assessed for use in air quality and epidemiologic analysis. IMSI contribute to fill the gap in the path towards a multipollutant air quality approach in two aspects: IMSI represent an innovative way to identify mixtures of pollutants based on outcomes and constitutes an alternative approach to assess multipollutant health effects. IMSI developed for mobile sources can be easily applied to other sources. Results can support the setting of multipollutant air quality standards.
Supplemental materials are available for this article. Go to the publisher's online edition of the Journal of the Air & Waste Management Association for materials showing the estimation of uncertainties using propagation of errors, comparison of source impacts from CMB and PMF and wind direction and speed for the Jefferson Street monitoring location in Atlanta.
Introduction
Air quality standards, such as the National Ambient Air Quality Standards (NAAQS) in the United States, have traditionally focused on setting maximum limits to ambient concentrations of individual pollutants. The NAAQS, and air quality standards in general, are developed from available studies, both mechanistic and epidemiological, that seek to deduce the impacts to human health from air pollution. To date, most air pollution epidemiologic work has examined associations between health outcomes and individual pollutants. However, human exposure to air pollution occurs in a multipollutant setting. Thus, a multipollutant approach may be more realistic to understanding risks and regulating urban air pollution.
Multipollutant approaches have been extensively applied in controlling emissions of pollutants to the atmosphere. Pollutants are rarely emitted in isolation by a source, and control devices for one pollutant can usually modify emissions of all of the compounds. For example, a three-way catalytic converter for gasoline vehicles is able to control nitrogen oxides, carbon monoxide, and unburned hydrocarbons at the same time (CitationTakahashi et al., 1996; CitationWesterholm et al., 1996). Furthermore, multipollutant control has been demonstrated to be cost-effective (CitationU.S. Environmental Protection Agency, 2007a).
From a regulatory viewpoint, multipollutant regulations already exist for emission standards. For example, heavy- and light-duty fleets are required to meet nitrogen oxide (NOx), carbon monoxide (CO), particulate matter (PM), hydrocarbon (HC), nonmethane HC (NMHC) standards (CitationU.S. Environment Protection Agency, 2011a). In addition, U.S. Environmental Protection Agency (EPA) recently proposed the aquatic acidification index (AAI), a multipollutant index developed based on analysis of ecological effects, to be used as part of a potential combined NAAQS standard considering the combined effects of NOx and sulfur oxide (SOx) deposition on aquatic ecosystems (CitationEPA, 2011b). In the past years, substantial progress has been made to move towards a result-oriented, risk-based, multipollutant approach in air quality management (CitationNARSTO, 2010). A consistent limitation of adopting this multipollutant approach has been the identification of mixtures of pollutants in the atmosphere and the health effects of such mixtures (CitationHidy and Pennell, 2010; CitationNational Research Council, 2004; EPA, 2007). Statistical tools, such as factor analysis (FA), have been suggested to overcome this limitation (CitationEPA, 2011c). Receptor models have also been used to combine pollutants in source categories and source impacts have been associated with health outcomes (CitationSarnat et al., 2008; CitationThurston et al., 2005). However, these techniques rely on an abundant amount of air quality data, including availability of specific components that are not routinely measured.
Multipollutant models in epidemiologic analysis have generally included two or more pollutants at a time within a model, with the goal of identifying confounders in associations with health rather than the effects of a mixture of pollutants (CitationBell et al., 2011; CitationDominici et al., 2010; CitationMauderly and Samet, 2009; CitationMauderly et al., 2010). Multipollutant models are subject to exposure measurement error (e.g., when surrogate measurements from central monitoring sites are used to assess human exposure), but can also have differential errors (e.g., where the pollutant measured with the least amount of error is the one with the strongest signals) and reduced statistical power (when more than one pollutant at a time is included) (CitationVedal and Kaufman, 2011). Moreover, the mixtures included in multipollutant models do not always represent an actual or unique source of emissions, which complicates designing effective measures to improve public health (CitationFranklin et al., 2008; CitationHart et al., 2011; CitationLenters et al., 2010; CitationMetzger et al., 2004; CitationPeng et al., 2009).
Mobile source emissions have been identified as a key urban air pollution component adversely affecting public health (CitationBeelen et al., 2008; CitationTonne et al., 2007). In the Atlanta area, elevated NO2, CO, PM with an aerodynamic diameter <2.5 μm (PM2.5), organic carbon (OC), and elemental carbon (EC) concentrations, pollutants traditionally related to traffic, have been associated with emergency department (ED) visits for cardiovascular disease (CVD) (CitationHealth Effects Institute, 2010; CitationMetzger et al., 2004). Results from using receptor models in epidemiologic analysis provide further support that combustion-related sources are associated with CVD (CitationSarnat et al., 2008).
The adverse impact of mobile sources on health is due to the magnitude of these sources in the Atlanta area, where traffic emissions are estimated to account for 30% of the PM2.5, 84% of the NOx, and 97% of the CO emissions (CitationEPA, 2007b). Results from source apportionment indicate that the contribution of tailpipe mobile source emissions to ambient PM2.5 varies from 17% to 26%, and the total impact from mobile sources is likely larger considering that a significant amount of crustal material (i.e., Al, Si, Ca, Fe, K) originates from the resuspension of dust due to vehicles (CitationLee et al., 2008b; CitationLiu et al., 2005; CitationKim et al., 2003, 2004).
Our objective in this work is to develop and assess outcome-based, multipollutant indicators for mobile sources here called integrated mobile source indicators (IMSI). We aim to investigate if more refined indicators of mobile sources are more likely to explain associations with health outcomes. IMSI are simple to construct and calculate from readily available data, and can be used in air quality and epidemiologic analyses. The species considered are CO, NOx, and EC available from routine air quality monitoring networks. Two sets of IMSI are developed: the first is based on outcomes from analysis of pollutant emissions and observed concentrations (here called IMSIEB). A sensitivity analysis is used to refine the indicators based on two-pollutant mixtures of NOx&EC, NOx&CO, and CO&EC and develop a second set of indicators based on health outcomes (here called IMSIHB). Temporal and spatial variability of IMSI are assessed and compared with source impacts from receptor models.
Methods
IMSI development and assessment follows four steps: (i) selection of pollutants and analysis of emission inventories; (ii) development of the emission-based integrated indicators (IMSIEB); (iii) comparison of air pollutant impact analysis using indicators with results from receptor models; and (iv) associations with acute health responses in Atlanta and development of health-based indicators (IMSIHB).
Pollutant selection and analysis of emission inventories
Traditionally, CO and NOx have been used as gaseous indicators of vehicular activity. CO is emitted primarily by gasoline-fueled engines, whereas both gasoline and diesel engines have substantial NOx emissions. Mobile source–based PM2.5 is generated not only via combustion processes but also mechanical grinding and secondary formation (i.e., formation in the atmosphere from PM2.5 precursors under photochemical conditions). Because PM2.5 can have several sources, it is preferable to use components that are better indicators of PM2.5 from combustion sources. PM2.5 EC and OC are formed during combustion, with OC being produced in early stages of combustion and EC at later stages and higher temperatures (CitationWatson et al., 2001). OC is also formed from other processes, including secondary formation from biogenic emissions. Gasoline vehicles (GVs) usually have a higher OC/EC ratio than diesel vehicles (DVs), with values around 3.0–4.0 for GVs and below 1.0 for DVs (CitationLee and Russell, 2007; CitationZheng et al., 2007). Because diesel exhaust contains much higher EC concentrations than gasoline exhaust, EC has been used as a tracer for diesel impacts on PM (CitationMarmur et al., 2005).
Other PM2.5 components, including heavy metals such as zinc (Zn), nickel (Ni), vanadium (V), copper (Cu), and lead (Pb), have also been used as tracers to identify mobile source impacts on air quality, and specifically to split calculated impacts between gasoline and diesel vehicles (CitationLee et al., 2008a). Zn is used as a tracer of GV, and is a component of additives in lubricating oil, Pb and Cu are produced from brake wear and road traffic, and Ni and V are found in diesel exhaust. Organic compounds, such as hopanes and polycyclic aromatic hydrocarbons (PAHs), are used as tracers of traffic-related PM impacts as well (CitationBrook et al. 2007; CitationZheng et al. 2002). Although these organic compounds are very useful in the identification of specific source impacts, their measurement is more resource intensive and their concentrations are not as widely available.
We chose CO, NOx, and EC to develop the traffic-related IMSI because these species are ubiquitous to monitoring stations in the United States and emission inventories. A detailed analysis of CO, NOx, and EC emissions and ambient air concentrations in downtown Atlanta (Fulton County) was conducted for the period 1999–2007. Emissions from mobile sources (on-road and non-road) were obtained from the EPA National Emission Inventory (NEI) (E.P.A., 2007) and the Visibility Improvement State and Tribal Association (VISTAS) project (CitationGeorgia-DNR, 2007). The 2002 EPA NEI was consulted for emission estimates, but estimates from earlier years are expected to be used in the development of this inventory.
Additionally, we applied the EPA Motor Vehicle Emissions Simulator (MOVES 2010) to identify the fraction of emissions from on-road GVs and DVs (CitationEPA, 2010). Both NEI and MOVES use nationwide information of vehicle miles traveled (VMT) to estimate on-road emissions, but emissions factors used in MOVES 2010 have been revised from those used in NEI.
Ambient air quality data were obtained from the Jefferson Street monitoring location (JST), a site operated by the Southeastern Aerosol Research and Characterization Study (SEARCH). Description of the measurement methods is found elsewhere (CitationEdgerton et al., 2005; CitationHansen et al., 2003). Briefly, elemental carbon (EC) is measured on 24-hr PM2.5 samples using quartz filters from a particle composition monitor (PCM) and analyzed by the thermal-optical reflectance (TOR) method at the Desert Research Institute (DRI) following the Interagency Monitoring of Protected Visual Environments (IMPROVE) protocol (Chow et al., 1993). CO, NO, and NO2 are measured every minute and averaged to the hour. CO is measured using nondispersive infrared spectrophotometry. NO2 is measured via photolytic conversion to NO, followed by chemiluminescence. NO and NO2 are summed and reported as NOx. For the period 1999–2004, a total of 1701 days were selected for use after removing days with missing data or data with high uncertainty.
An additional site, the South DeKalb (SD) monitoring station from the EPA's Speciation Trends Network (STN) located 15.3 km to the southeast of JST () was examined to assess the spatial variability of IMSIEB. JST site is located within 3.2 km of interstate I-85 and 8.0 km of interstate I-20, and in close proximity to the intersection of these two highways. SD is located within 3.2 km of interstate I-285, and in close proximity to the intersection of this highway and I-20. Both JST and SD are heavily impacted by traffic emissions. The air quality monitoring is conducted at street level in both sites. At SD, NOx was available in the same number of days at JST. For CO, SD did not record measurements before 2003, and therefore, only 562 days were available. EC is measured every third day at SD, therefore only 462 days were available.
Figure 1. Location of Jefferson Street (JST) and South DeKalb (SD) monitoring stations in Atlanta. Area in gray is Fulton County in Atlanta.
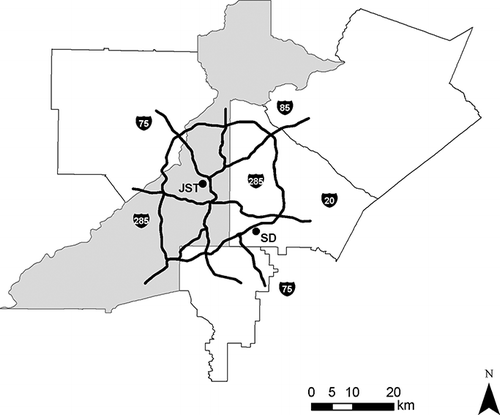
Carbon monoxide (CO)
The NEI reports total CO emissions of approximately 295,000 tons/year for Fulton County in 2002, of which 97% are from mobile sources (75% on-road and 22% non-road). The on-road CO emissions estimated with MOVES are lower (approximately 190,000 tons/year) due to revisions in the emission factors from 1992 to 2002. MOVES estimates that 98% of the on-road CO emissions are from GVs and 2% are from DVs.
On a daily basis, on-road CO emission estimates are 20% higher during weekdays than weekends, indicating a decrease in GV travel during weekends. On a monthly basis, CO emissions from GVs have two periods of increase during the year (): June through August due to the use of air conditioning in summer, and December through February as result of cold start emissions and lower ambient temperatures (EPA, 2008).
Figure 2. Monthly and annual trends of CO, NOx, and EC. Bars represent emissions estimates from MOVES in tons/month (a, c, e) or tons/yr (b, d, f). Bold line represents ambient air concentrations of CO (ppm), NOx (ppb), and EC (μg/m3) on right vertical scale. Error bars are the root mean square (RMS) error of daily uncertainties from measurements. R 2 denotes the correlation between annual emissions and annual average concentrations.
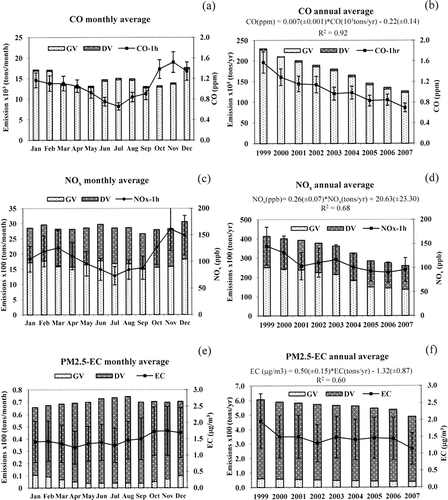
Ambient CO concentrations are the lowest during the summer months (), especially during June and July when dispersion of contaminants is favored and CO photochemical destruction is faster. The highest concentrations are found from October through December when wind speeds are slower (Figure S1 in the Supplemental Material) and thermal inversion episodes trap pollutants nearer the ground. From January through March, inversions are still present, but wind speeds are significantly higher than prior months resulting in greater dispersion of pollutants.
On an annual basis, there is a clear trend between reductions in ambient air concentrations of CO and emission reductions from 1999 to 2007 (). Comparison of CO emission estimates from MOVES and NEI in 1999, 2002, and 2005 shows good agreement between both methods. The reduction of CO emissions in 2007 with respect to 1999 was 48% in NEI and 45% in MOVES.
These results support using CO concentrations as an indicator of GV impacts, though this indicator is limited to local sources and can lead to potential biases in a regional assessment.
Nitrogen oxides (NOx)
The NEI reports total NOx emissions of approximately 47,000 tons/year for Fulton County in 2002, of which 87% are from mobile sources (72% on-road and 15% non-road). NOx emissions estimated with MOVES are lower than the NEI estimates (approximately 38,000 tons/year) due to lower exhaust emissions from heavy-duty vehicles (CitationU.S. Environmental Protection Agency, 2010). MOVES estimates that 60% of the on-road NOx emissions are from GVs and 40% are from DVs. Although diesel engines emit more NOx than spark ignition engines per mile traveled, the gasoline fleet is significantly larger (about 10 times) than the diesel fleet for Fulton County (CitationBlanchard et al., 2010). Other sources contributing to NOx emissions are classified as area and point sources, in particular fuel combustion in electrical generating utilities (EGUs) and biomass burning. It is expected, however, that most of the NOx impacting the receptor stations come from mobiles sources, because EGUs have high effective stack heights such that pollutants are better dispersed before impacting the monitor station at the surface. Further, NOx emissions from point sources were 13% of the total emissions in 1999, but only 2% in 2007. EPA has estimated that nationwide reductions of NOx are the result of emissions reductions at electrical utilities and among on-road mobile sources (EPA, 2008).
On a weekly basis, NOx ambient air concentrations are 24% higher on weekdays than on weekends, consistent with a larger reduction of DV than GV traffic during weekends. On a monthly basis, NOx follows a similar trend to CO, with higher concentrations in winter and lower concentrations during summer () when NOx is more rapidly removed by photochemical reactions. NOx emissions from DVs are relatively constant throughout the year, whereas NOx emissions from GVs have a similar trend to CO emissions, increasing in summer months due to the use of air conditioning (A/C) systems and in winter months due to cold start emissions and lower ambient temperatures (EPA, 2008).
On a yearly basis, NOx ambient concentrations decreased from 1999 to 2001, increased during 2002 and 2003, and decreased again until 2007 (). The significant reduction during the period 1999–2001 (from 154 to 103 ppb) is likely a result of the implementation of the EPA acid rain program and stationary controls to reduce ozone, combined with mobile source reductions (CitationEPA, 2005). From 2002 to 2007, reductions in ambient NOx are attributed largely to decreases in on-road NOx emissions. NOx emissions from on-road sources have a stronger correlation with ambient NOx during the period 2002–2007 (R 2 = 0.65) than 1999–2007 (R 2 = 0.36).
These results indicate that mobile source NOx emissions have a large impact on ambient NOx concentrations, but are not as dominant as mobile source CO emissions on ambient CO concentrations.
Elemental carbon (EC)
VISTAS estimates of EC emissions for 2002 is 92% from mobiles sources (on-road and non-road) and 8% from other sources, such as biomass burning. From the on-road fraction, MOVES estimates 91% from DVs and 9% from GVs. On a weekly basis, EC concentrations are 30% higher during weekdays than weekends, due to the higher fraction of diesel vehicle traffic on weekdays. On a monthly basis, EC concentrations are lowest in spring and summer as compared with the October–December period. During the cooler months, dispersion of pollutants is not favored due to increased thermal inversions and reduced wind speeds ().
EC emissions from DVs increase in summer due to an increase in VMT and greater construction activity with the subsequent increase in non-road emissions. Although not as large as emissions from DVs, EC emissions from GVs can be an important source during winter time because of cold starts and lower ambient temperatures (U.S. Environmental Protection Agency, 2008).
On an annual basis, EC concentrations decreased from 1.97 μg/m3 in 1999 to 1.13 μg/m3 in 2007 () as a result of changes in fuel composition and controls on mobile sources, such as the introduction of low- (50 ppm) and ultra-low- (15 ppm) sulfur diesel in 2002 and 2006, respectively (CitationEPA, 2000). Controls in point and open fires might also have helped on this reduction, such as the open burning ban implemented in the 13-county metro Atlanta area in 1996. These results indicate that EC emissions are dominated by mobile sources for Fulton County, particularly by DVs.
In summary, data from Atlanta using a number of emissions and monitoring databases show that CO and EC are likely good indicators of GVs and DVs, respectively. NOx appears to be an indicator of overall mobile sources and cannot be easily used to discern between GVs and DVs. Because only 20% of the total OC emissions are from mobiles sources (on-road and non-road) (CitationGeorgia-DNR, 2007) and a fraction between 26% and 47% of ambient OC can be formed secondarily (CitationPachon et al., 2010), our development of IMSIEB does not use OC, though the indicators can be used to estimate the OC from mobile sources.
Development of the emission-based integrated indicators
We propose a multipollutant indicator of CO, NOx, and EC to assess mobile source impacts on air quality. In this work, the highest 1-hr values at each day for CO and NOx were chosen because the 1-hr metric has been found more associated with health outcomes than other metrics (CitationMetzger et al., 2004). The IMSIEB uses ratios of mobile-source-to-total emissions for each pollutant as weighting coefficients ( Equationeq 1). Mobile source fractions of each pollutant can be estimated by multiplying these ratios by the ambient air concentrations. For example, the fraction of ambient EC from mobile sources is estimated here as the total EC concentration multiplied by the ratio (E EC,mobile/E EC,total), where Ei,j represents the emission of pollutant i for source category j, in units of tons/year estimated at Fulton County in Atlanta. Pollutant concentrations for EC are in units of μg/m3, which differ from the units of ppm used for CO and NOx. Therefore, a normalization of ambient air concentrations by the standard deviation of 1701 samples for EC, CO, and NOx was performed (CitationBevington and Robinson, 2003). The normalization is also useful in this case because each pollutant has a different range of measurements.
where denotes the normalized concentrations (Ci
and σ
i
represent the average concentration and standard deviation of pollutant i in the period 1999–2007) at the Jefferson Street receptor site. The IMSIEB uses normalization by the sum of the emission ratios in such a way that the indicator can be easily compared with other IMSIs.
The ratios between mobile emissions (E i,mobile) and total emissions (E i,total) in Equationeq 1 are defined as the weighting coefficients. These weighting coefficients for NOx and CO, obtained from the NEI database, are 0.84 ± 0.03 and 0.97 ± 0.01, respectively. Uncertainties in the NOx and CO mobile/total ratios are obtained as 1 standard deviation of the estimated ratios in the period 1999–2007. The weighting coefficient for EC was estimated from VISTAS in 2002 to 0.92 ± 0.04. Uncertainty in the EC mobile/EC total ratio is obtained as one standard deviation of the monthly values during 2002. After replacing the values of the weighting coefficients into Equationeq 1, this can be expressed as
We were also interested in differentiating impacts from gasoline and diesel exhaust emissions, because the contribution at the receptor site can be quite different and the control mechanisms are specific to each type of vehicle. Therefore, we define integrated indicators for gasoline vehicles (IMSIEB,GV) and diesel vehicles (IMSIEB,DV) using specific emission ratios from gasoline and diesel emissions estimated with MOVES.
The ratio of gasoline-to-mobile emissions was used as a weighting coefficient for each species, being 0.58 ± 0.02 for NOx and 0.98 ± 0.01 for CO, obtained from the application of MOVES. For EC, the ratio of gasoline-to-mobile emissions is more seasonally dependent, with a summer value of 0.06 ± 0.01 and a winter value of 0.12 ± 0.04. These results indicate more weight on the CO and NOx than EC. Therefore, IMSIEB,GV was defined as a weighting mixture of CO and NOx only:
where,
. After replacing the values of the weighting coefficients into Equationeq 3, this can be expressed as
Similarly, the ratios of diesel-to-total mobile emissions obtained from MOVES, used as weighting coefficients, for NOx was 0.42 ± 0.02 and for CO 0.02 ± 0.01. For EC, the ratio of diesel-to-total mobile emissions was more seasonally dependent, with a summer value of 0.94 ± 0.01 and a winter value of 0.88 ± 0.04. These results imply more weight on EC and NOx than CO. Therefore, IMSIEB,DV was defined as a weighting mixture of EC and NOx only:
where,
. After replacing the values of the weighting coefficients into Equationeq 5, this can be expressed as
Specification of the IMSIEB has limitations. First, the emission fraction for a pollutant is translated to an ambient fraction assuming that the average source and receptor fractions are the same. This assumption has been tested in the past with good results: gas-to-PM2.5 emission ratios were used to optimize source profiles in Atlanta, leading to a more accurate apportionment of PM2.5 from mobile sources and coal-fire power plants (CitationMarmur et al., 2005). A second limitation is the assumption that emission ratios are constant on a daily basis and equal to the annual average. However, daily variations in the indicators are captured by the use of ambient air concentrations.
Comparison of air pollutant impact analysis using indicators with results from receptor models
The IMSIEB were compared with source apportionment from receptor models. The chemical mass balance method CMBv.8.2 (CitationWatson et al., 1984) and the positive matrix factorization method PMFv3.0 (CitationNorris and Vedantham, 2008) were applied to the same period of time as the indicators. For PMF, strong species (i.e., species with signal/noise ratio >2.0) were NO3, SO4, NH4, EC, four OC thermal fractions, Al, Si, Fe, K, Ca, Br, Mn, and Zn, and weak species (i.e., species with signal/noise ratio <2.0) were PM2.5, Cu, Pb, and Se. The temperature-resolved OC fractions were chosen to improve the separation between gasoline and diesel source impacts (CitationKim et al., 2004; CitationLiu et al., 2005). Missing data were replaced by their geometric mean to conserve the original number of samples for better performance of the PMF algorithm (CitationReff et al., 2007). For CMB, optimized source profiles were chosen from a previous study in Atlanta and sources, such as coal-fire power plant and cement production, were eliminated to avoid negative source impacts (CitationMarmur et al., 2005).
Associations between pollutant mixtures and acute health responses in Atlanta
We have assumed that pollutants mix based on emission ratios, but this mixture can be influenced by meteorological conditions. The sensitivity analysis aims to assess different fractions on which pollutants can combine once in the atmosphere. Based on the combination of pollutants in the IMSIEB,GV and IMSIEB,DV, a sensitivity analysis was performed between two-pollutant mixtures. For IMSIEB,GV mixtures of CO and NOx were evaluated and for IMSIEB,DV mixtures of EC and NOx were chosen. In addition, mixtures of EC and CO were also evaluated. The sensitivity analysis was performed as follows.
where ,
, and
represent the normalized concentrations and α is a parameter that varies from 0 to 1, in such a way that allows comparing pollutants individually (when α is equal to 0 or 1) versus two-pollutant mixtures. In particular, the combination of NOx&CO at α = 0.4 (i.e., 0.4 ×
+ 0.6 ×
) is equivalent to IMSIEB,GV (
Equationeq 4) and the combination of NOx&EC at α = 0.3 (i.e., 0.3 ×
+0.7 ×
) is equivalent to IMSIEB,DV (
Equationeq 6).
The association of mobile source multipollutant metrics with health effects was assessed in an epidemiologic analysis. CVD ED visits were chosen as the health endpoint for this analysis, given that those have been found to be associated with combustion-related activities in Atlanta (CitationMetzger et al., 2004; CitationSarnat et al., 2008). Our epidemiologic models only assess short-term effects of these pollutants (i.e., we look at the daily measure of the indicator and see if it predicts that same day's CVD count). Assessing relationships between chronic exposures to the indicators (i.e., long-term averages of the exposures) is beyond the scope of analysis. Briefly, data were collected for ED visits related to CVD from 41 hospitals in metro Atlanta from 1999 to 2004. Daily ED counts are regressed with air pollution indicators using a Poisson generalized linear model (GLM). We used a similar epidemiologic model to the model applied in previous analyses of CVD outcomes in these data; this Poisson generalized linear model is described in more detail elsewhere (CitationMetzger et al., 2004; CitationSarnat et al., 2008). However, for this analysis, as well as other more recent analyses of these data, we included more stringent control for temperature (CitationStrickland et al., 2010).
Unlike traditional multipollutant models that solve for different regression coefficients, our approach solves for only one β for a multipollutant indicator. The values of α at which the two-pollutant mixtures show the strongest association with CVD define the health-based integrated indicators (IMSIHB).
Results
The assessment of the IMSIEB was conducted as follows: (i) analysis of IMSIEB trends; (ii) comparison with mobile source impacts from receptor models; (iii) uncertainties in the estimation of the indicators; (iv) comparison with IMSIHB derived from associations with CVD ED visits; and (v) implication for multipollutant air quality standards.
IMSIEB trends
IMSIEB exhibit similar temporal trends as ambient EC, CO, and NOx, with a decrease during summer and increase during fall and reduction in annual averages from 1999 to 2007 (). On a monthly basis, IMSIEB,GV showed a larger reduction in concentrations during summer than IMSIEB,DV consistent with less commuting from light-duty traffic during the summer months. On an annual basis, there is a larger decrease in IMSIEB,GV than IMSIEB,DV explained by a more rapid introduction of new control technologies in the gasoline fleet than diesel vehicles. The comparison of IMSIEB annual averages with reduction in emissions of CO, NOx, and EC with respect to 1999 shows a similar trend, suggesting a good agreement between indicators estimated with ambient air concentrations and emissions from mobile sources.
Figure 3. Temporal trends (a) monthly (b) annual of IMSIEB, IMSIEB,GV, and IMSIEB,DV (unitless). The indicators are normalized such as they have a standard deviation of 1. Annual trend is compared with reduction in emissions of CO, NOx, and EC with respect to 1999 (on right y-axes).
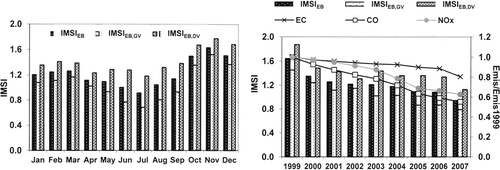
On a daily basis, IMSIEB is 22% larger during weekdays than weekends, capturing the increase in traffic during the week. The IMSIEB,DV trend has a large decrease during weekends (30%) than IMSIEB,GV (14%), and is explained by reduced diesel traffic during the weekend.
Comparison with results from receptor models
CMB and PMF methods yield similar estimates for PM air quality impacts for the chosen sources (Table S1 in the Supplemental Material). The correlation between gasoline and diesel source impacts resolved by CMB and PMF () was substantially lower than the correlation of the combined fractions into one mobile source (R 2 = 0.75/0.51 in winter/summer), which demonstrates the difficulties receptor models have in adequately capturing the split between gasoline and diesel daily contributions. The proposed IMSIEB correlates more strongly with total mobile source impacts from CMB (R 2 = 0.73/0.53 in winter/summer) than PMF (R 2 = 0.72/0.48 in winter/summer). The IMSIEB,DV correlates stronger with diesel source impacts from CMB and PMF than the corresponding correlation of IMSIEB,GV with gasoline source impacts from the receptor models. This is due to both CMB and PMF using EC as a fitting species to solve for diesel contributions, and IMSIEB,DV is heavily weighted by EC, whereas IMSIEB,GV uses CO, a species that is typically not used to fit the CMB or PMF gasoline source categories.
Table 1. Correlations between IMSIEB, IMSIEB,GV, and IMSIEB,DV with single species and daily source impacts from CMB and PMF
In the southeastern United States, biomass burning can also be a significant source of EC, CO, and NOx to ambient air (CitationLee et al., 2008a). IMSIEB does not seem to be influenced by daily impacts from this source. On selected days with biomass burning activity over 4.0 μg/m3 of PM2.5 identified by both CMB and PMF, the integrated indicators were more strongly correlated with source impacts from mobile sources than wood burning. On those days, the correlation between IMSIEB and mobile source impacts from CMB (R 2 = 0.86) or PMF (R 2 = 0.83) were significantly higher than the correlation between IMSIEB and source impacts from biomass burning from CMB (R 2 = 0.13) or PMF (R 2 = 0.34). This result supports the emissions estimates and analyses detailed above that found emissions of EC, CO, and NOx in Atlanta predominantly from mobile sources.
Uncertainties in mobile source indicators
Uncertainties are involved in several steps of the calculations (e.g., emission estimates, ambient measurements, receptor models), and we estimate uncertainties in IMSIEB and compare them with uncertainties from singles species and receptor model source contributions. Uncertainties in ambient measurements (EC, CO, NOx) were estimated as one-third of the detection limit (μg/m3 or ppm) plus the product of analytical uncertainty (percentage) and concentration (CitationPolissar et al., 1998). Both detection limit and instrument uncertainty were obtained from the SEARCH study (CitationHansen et al., 2003). Uncertainties in CMB source impacts are estimated using the effective variance method, which considers uncertainties in both source profiles and ambient concentrations (CitationLee and Russell, 2007). In PMF, uncertainties in factor contributions are not given explicitly by the model, so a bootstrapping procedure is used (CitationNorris and Vedantham, 2008). Uncertainties in IMSIEB are propagated from individual uncertainties taking into account that CO, NOx, and EC are correlated between each other and, therefore, covariance terms need to be included (CitationInternational Organization for Standardization, 1993). Application of the propagation of errors in the estimation of the IMSIEB uncertainties is explained in detail in the Supplemental Material.
Among single species, CO and NOx have lower uncertainties than EC because gas species are typically more precisely measured than filter-based PM2.5 species measurements (). The IMSIEB show uncertainties larger than the ambient measurements, because uncertainties in emissions ratios are involved in addition to the ambient measurement uncertainties. Furthermore, the calculation of uncertainties in IMSIEB includes the uncertainty provided by correlated quantities, as it has been demonstrated they can impact uncertainty results (CitationEspinosa et al., 2010). Here, the uncertainty in IMSIEB is primarily driven by the uncertainties in the covariance relationships between EC, CO, and NOx. For IMSIEB,GV, the uncertainties are driven by the covariance relationships of CO and NOx. For IMSIEB,DV, uncertainties are mostly driven by uncertainties in EC measurements and emissions ratios and the covariance relationships of EC and NOx.
Table 2. Comparison of uncertainties between indicators
The range of uncertainties of IMSIEB (0.51–0.68) is comparable to the range of uncertainties in PMF (0.26–0.67), which is a typical method to estimate contributions from mobile sources. The high range of uncertainties in CMB (1.00–1.48) is mostly explained by uncertainties in the source profiles (CitationLee and Russell, 2007).
IMSIHB derived from associations with CVD ED visits
The epidemiologic model was implemented with time series of pollutant concentrations (CO, NOx, EC), sources impacts (from CMB and PMF), IMSIEB, IMSIEB,GV IMSIEB,DV and the two-pollutant mixtures from the sensitivity analysis. A total of 40 metrics were evaluated and compared for the daily association between the metric and corresponding ED visits for CVD in the period 1999–2004. Within the single species, NOx, 1-hr, was the most strongly associated with CVD, followed by CO, 1-hr; EC was found borderline significant (). Pollutants that are better measured such as CO and NOx typically have stronger associations in epidemiologic models (CitationVedal and Kaufman, 2011), and NOx has been proposed as indicator of toxic species emitted by traffic (CitationBrook et al., 2007). When the three pollutants are combined to form the IMSIEB, the strength of association is only slightly greater than either for EC or CO, 1-hr, separately, but less than NOx, 1-hr. Using IMSIEB,GV and IMSIEB,DV as predictors of CVD-related ED visits in the model resulted in statistically significance associations. Gasoline and diesel source impacts from CMB and PMF were not found to be significantly associated with CVD. The lack of significance may be due to the use of EC as a fitting species in both CMB and PMF. Differences in time periods, health outcomes, and analytic methods (e.g., tighter controls in the epidemiologic analyses) may explain the significant association between mobile source daily contributions and CVD found in other studies in the Atlanta area (CitationMetzger et al., 2004; CitationSarnat et al., 2008).
Table 3. Results for the associations of ED for CVD with mobile source impacts metrics (sorted by P value)
The inclusion of the two-pollutant mixtures (NOx&EC, NOx&CO, CO&EC) in the epidemiologic model is represented in three curves with a minimum point where the association with CVD is strongest (). The minimum point suggests that the combinations of pollutants at specific fractions are more likely to explain associations with health outcomes than individual species. The minimum point in the curves occurs at α = 0.6 for NOx&CO, at α = 0.7 for NOx&EC, and at α = 0.5 for CO&EC. It would seem reasonable that a large fraction of NOx in the NOx&CO and NOx&EC pairs would give a stronger significance to the association with CVD outcomes, but this is not the case. Similarly, for the CO&EC pair one could also expect that a larger fraction of CO in the mixture would give greater significance. The minimum occurs when both pollutants are approximately equally weighted.
Figure 4. Sensitivity analysis of the association between pairs of pollutants and CVD outcomes; the dashed line represents P value = 0.05.
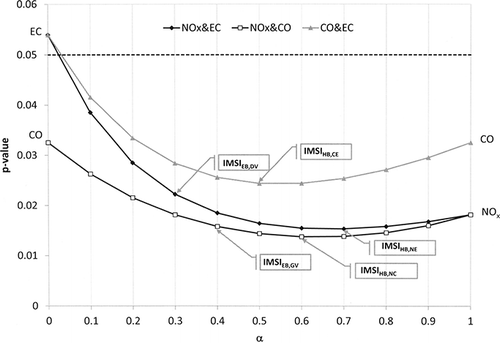
The points where the association of the two-pollutant mixtures and CVD is strongest defined the health-based indicators. That is, we define IMSIHB,NC at α = 0.6 for NOx&CO, IMSIHB,NE at α = 0.7 for NOx&EC, and IMSIHB,CE at α = 0.5 for CO&EC. Pollutants used in the two-pollutant IMSIHB are denoted by a subscript, e.g., “NE” for NOx and EC mixture. The IMSIHB hold different fractions of pollutants than the IMSIEB, suggesting that other sources, such as biomass burning, may be contributing in the association with CVD though the P values are relatively constant between both integrated indicators. The advantage of using IMSIEB is that specific control mechanisms can be suggested to mobile sources facilitating the work of policy makers.
The IMSI and the two-pollutant mixtures showing different associations with CVD outcomes at different α values can be partially explained by the correlations between individual pollutants and the two-pollutant mixture and more clearly by the correlation between indicators at two different receptor sites.
With respect to the first explanation, the correlation of each pair of pollutants and the third pollutant not included in the pair changes with the α fraction (). For example, the correlation between the NOx&EC mixture and CO has a maximum value at α = 0.5 (R 2 = 0.63), which is larger than the correlation between CO and EC (R 2 = 0.49) or CO and NOx (R 2 = 0.55). Similarly, the binary mixtures NOx&CO and CO&EC have stronger correlations with EC and NOx at α = 0.4 and α = 0.6, respectively.
Figure 5. Correlation (R 2) between binary mixtures and the pollutant not included in the mixture (NOx&EC vs CO; NOx&CO vs EC; CO&EC vs NOx). Vertical scale starts at 0.3 to emphasize correlations.
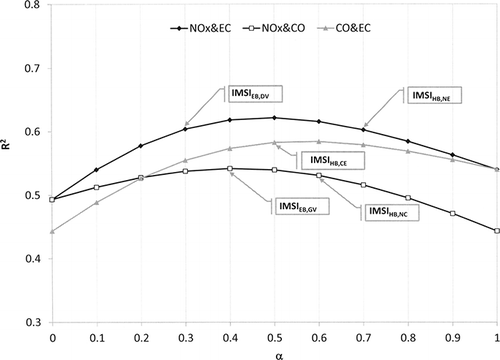
A higher correlation of a binary mixture with a third pollutant more strongly associated with CVD, may explain the higher association of that mixture with the health outcome. For example, the strongest association of the CO&EC mixture with CVD at α = 0.5 () might be explained by the higher correlation of the CO&EC pair and NOx at that particular alpha (). However, this is not the case for NOx&EC and NOx&CO pairs, which already include the statistical power of NOx in the health association.
A more clear explanation can be found in the correlation between indicators at different receptor sites. We observe that the correlations between pairs of pollutants estimated at JST and the corresponding pairs calculated at SD are stronger at certain value of α than others (). These values are α = 0.5 for NOx&EC (R 2 = 0.72) and NOx&CO (R 2 = 0.72) and α = 0.4 for CO&EC (R 2 = 0.76). The correlations between pair of pollutants at JST and SD are stronger than the correlations between single pollutants at both receptor sites (R 2 = 0.64 for EC; R 2 = 0.55 for CO; R 2 = 0.59 for NOx). Previous studies have found that for pollutants with large spatial error, health associations are likely attenuated (CitationGoldman et al., 2010). Pollutant mixtures having a stronger correlation between sites than single pollutants may offer a better explanation of the stronger association with health.
Figure 6. Correlation (R 2) between binary mixtures at two different receptor sites JST and SD. Vertical scale starts at 0.3 to emphasize correlations. Overlapped days at JST and SD: NOx&EC (n = 398), NOx&CO (n = 560), and CO&EC (n = 173).
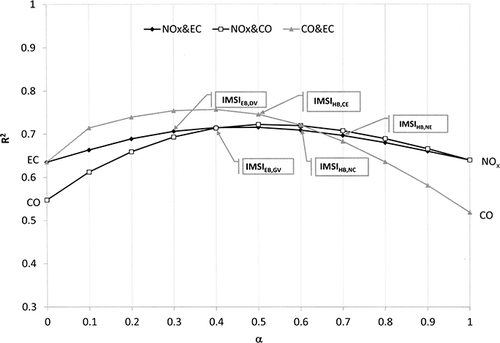
As a result of the greater correlation between sites, IMSIEB,GV and IMSIEB,DV constitute better indicators for mobile sources, which are expected to be ubiquitous in the area. This result complements a previous analysis in which mobile source impacts were classified as having “intermediate” spatial representativeness of the Atlanta area due to the lack of a unique marker (EC was used as the only mobile tracer) (CitationMarmur et al., 2006). Using IMSIEB, mobile source impacts show a more robust representativeness in the area.
Finally, the association of pollutant mixtures with health outcomes could be related to interaction between pollutants as has been shown in laboratory studies (CitationMauderly and Samet, 2009). This hypothesis needs further study.
Implications for multipollutant air quality standards
IMSIEB and IMSIHB can provide support to the setting of multipollutant air quality standards in a manner similar to that used in the development of the aquatic acidification index (AAI) (CitationEPA, 2011b). The AAI was designed to take into account the combined effects of NOx and SOx in the acidification of aquatic ecosystems, given that these two species are linked from atmospheric chemistry. Similarly, IMSIHB assess the effects of mixtures of pollutants associated with mobile sources on health and IMSIEB assess mixtures representing the gasoline and diesel vehicle impacts on air quality. Because mobile sources and their composition are ubiquitous, it is expected that the integrated indicators can be applied in other cities. IMSI are simple to construct and calculate and can be estimated at any monitoring site where EC, CO, and NOx concentrations are available.
Conclusions
This study proposed an approach to develop multipollutant indicators based on analysis of emissions inventories and health outcomes. The IMSIEB are simple to construct and calculate and demonstrate advantages over the use of single species: IMSIEB have stronger correlation between two different receptor sites than single pollutants, suggesting they are better indicators of the local impact of mobile sources, they agree well with the observed trends of traffic, and they have stronger associations with observed health effects, possibly due to their stronger correlation between sites. Uncertainties in IMSIEB are similar to uncertainties in ambient measurements and receptor models. A sensitivity analysis of fractions in IMSIEB led to the development of IMSIHB, suggesting mixtures of pollutants more strongly associated with CVD outcomes.
The use of IMSI in epidemiologic modeling constitutes an alternative approach to assess the health impact of pollutant mixtures. Although the approach presented in this article was developed for mobile sources, this work can be extended to other sources. IMSI can support the setting of multipollutant air quality standards because they represent the impact of traffic on health.
uawm_a_656218_sup_25011147.doc
Download MS Word (56.5 KB)Acknowledgments
This project is funded by EPA under grant numbers R83362601 and R83386601, Georgia Power, the Southern Company, and the Colombian Institute for the Development of Science and Technology (COLCIENCIAS). The authors thank Dr. Eric Edgerton at ARA, Inc., for access to the SEARCH data. Additional support for creation of the database came from NIEHS grant R01ES011294 and EPRI grant EP-P27723/C13172. Manuscript contents are solely the responsibility of the grantee and do not necessarily represent the official views of EPA or other sponsors. Further, EPA does not endorse the purchase of any commercial products or services mentioned in the publication.
References
- Beelen , R. , Hoek , G. , van den Brandt , P.A. , Goldbohm , R.A. , Fischer , P. , Schouten , L.J. , Jerrett , M. , Hughes , E. , Armstrong , B. and Brunekreef , B. 2008 . Long-term effects of traffic-related air pollution on mortality in a Dutch cohort (NLCS-AIR study) . Environ. Health Perspect. , 116 : 196 – 202 .
- Bell , M.L. , Cifuentes , L.A. , Davis , D.L. , Cushing , E. , Gusman Telles , A. and Gouveia , N. 2011 . Environmental health indicators and a case study of air pollution in Latin American cities . Environ. Res. , 111 : 57 – 66 .
- Bevington , P. and Robinson , K. 2003 . Data Reduction and Error Analysis for the Physical Sciences , 3rd , New York : McGraw Hill .
- Blanchard , C.L. , Hidy , G.M. , Tanenbaum , S. , Rasmussen , R. , Watkins , R. and Edgerton , E. 2010 . NMOC, ozone, and organic aerosol in the southeastern United States, 1999–2007: 1. Spatial and temporal variations of NMOC concentrations and composition in Atlanta, Georgia . Atmos. Environ. , 44 : 4827 – 4839 .
- Brook , J.R. , Burnett , R.T. , Dann , T.F. , Cakmak , S. , Goldberg , M.S. , Fan , X. and Wheeler , A.J. 2007 . Further interpretation of the acute effect of nitrogen dioxide observed in Canadian time-series studies . J. Expos. Sci. Environ. Epidemiol. , 17 ( S2 ) : S36 – S44 .
- Dominici , F. , Peng , R.D. , Barr , C.D. and Bell , M.L. 2010 . Protecting human health from air pollution: Shifting from a single-pollutant to a multipollutant approach . Epidemiology , 2 : 187 – 194 . doi: 10.1097/EDE.0b013e3181cc86e8
- Edgerton , E.S. , Hartsell , B.E. , Saylor , R.D. , Jansen , J.J. , Hansen , D.A. and Hidy , G.M. 2005 . The Southeastern aerosol research and characterization study: Part II. Filter-based measurements of fine and coarse particulate matter mass and composition . J. Air Waste Manage. Assoc. , 55 : 1527 – 1542 .
- Espinosa , A. , Miranda , J. and Pineda , J.C. 2010 . Evaluation of uncertainty in correlated quantities: Application to elemental analysis of atmospheric aerosols . Rev. Mex. Fis. E , 56 : 134 – 140 .
- Franklin , M. , Koutrakis , P. and Schwartz , P. 2008 . The role of particle composition on the association between PM2.5 and mortality . Epidemiology , 19 : 680 – 689 .
- Georgia-DN , R. 2007 . Visibility Improvement State and Tribal Association of the Southeast (VISTAS) Conceptual Description Support Document , Atlanta , GA : Georgia Department of Natural Resources .
- Goldman , G.T. , Mulholland , J.A. , Russell , A.G. , Srivastava , A. , Strickland , M.J. , Klein , M. , Waller , L.A. , Tolbert , P.E. and Edgerton , E.S. 2010 . Ambient air pollutant measurement error: Characterization and impacts in a time-series epidemiologic study in Atlanta . Environ. Sci. Technol. , 44 : 7692 – 7698 .
- Hansen , D.A. , Edgerton , E.S. , Hartsell , B.E. , Jansen , J.J. , Kandasamy , N. , Hidy , G.M. and Blanchard , C.L. 2003 . The Southeastern aerosol research and characterization study: Part 1—Overview . J. Air Waste Manage. Assoc. , 53 : 1460 – 1471 .
- Hart , J.E. , Garshick , E. , Dockery , D.W. , Smith , T.J. , Ryan , L. and Laden , F. 2011 . Long-term ambient multipollutant exposures and mortality . Am. J. Respir. Crit. Care Med. , 183 : 73 – 78 .
- Health Effects Institute . 2010 . HEI Panel on the Health Effects of Traffic-Related Air Pollution. A Critical Review of the Literature on Emissions, Exposure, and Health Effects , Boston , MA : Health Effects Institute . Special Report 17
- Hidy , G.M. and Pennell , W.T. 2010 . Multipollutant air quality management . J. Air Waste Manage. Assoc. , 60 : 645 – 674 . doi: 10.3155/1047–3289.60.6.645
- International Organization for Standardization (ISO) . 1993 . Guide to the Expression of Uncertainty in Measurements , Geneva , , Switzerland : ISO .
- Kim , E. , Hopke , P.K. and Edgerton , E.S. 2003 . Source identification of Atlanta aerosol by positive matrix factorization . J. Air Waste Manage. Assoc. , 53 : 731 – 739 .
- Kim , E. , Hopke , P.K. and Edgerton , E.S. 2004 . Improving source identification of Atlanta aerosol using temperature resolved carbon fractions in positive matrix factorization . Atmos. Environ. , 38 : 3349 – 3362 .
- Lee , S. , Kim , H.K. , Yan , B. , Cobb , C.E. , Hennigan , C. , Nichols , S. , Chamber , M. , Edgerton , E.S. , Jansen , J.J. , Hu , Y. , Zheng , M. , Weber , R.J. and Russell , A.G. 2008a . Diagnosis of aged prescribed burning plumes impacting an urban area . Environ. Sci. Technol. , 42 : 1438 – 1444 .
- Lee , S. , Liu , W. , Wang , Y.H. , Russell , A.G. and Edgerton , E.S. 2008b . Source apportionment of PM2.5: Comparing PMF and CMB results for four ambient monitoring sites in the Southeastern United States . Atmos. Environ. , 42 : 4126 – 4137 .
- Lee , S. and Russell , A.G. 2007 . Estimating uncertainties and uncertainty contributors of CMB PM2.5 source apportionment results . Atmos. Environ. , 41 : 9616 – 9624 .
- Lenters , V. , Uiterwaal , C.S. , Beelen , R. , Bots , M.L. , Fischer , P. , Brunekreef , B. and Hoek , G. 2010 . Long-term exposure to air pollution and vascular damage in young adults . Epidemiology , 21 : 512 – 520 . doi: 10.1097/EDE.0b013e3181dec3a7
- Liu , W. , Wang , Y.H. , Russell , A. and Edgerton , E.S. 2005 . Atmospheric aerosol over two urban-rural pairs in the southeastern United States: Chemical composition and possible sources . Atmos. Environ. , 39 : 4453 – 4470 .
- Marmur , A. , Park , S.K. , Mulholland , J.A. , Tolbert , P.E. and Russell , A.G. 2006 . Source apportionment of PM2.5 in the southeastern United States using receptor and emissions-based models: Conceptual differences and implications for time-series health studies . Atmos. Environ. , 40 : 2533 – 2551 .
- Marmur , A. , Unal , A. , Mulholland , J.A. and Russell , A.G. 2005 . Optimization-based source apportionment of PM2.5 incorporating gas-to-particle ratios . Environ. Sci. Technol. , 39 : 3245 – 3254 .
- Mauderly , J.L. , Burnett , R.T. , Castillejos , M. , Özkaynak , H. , Samet , J.M. , Stieb , D.M. , Vedal , S. and Wyzga , R.E. 2010 . Is the air pollution health research community prepared to support a multipollutant air quality management framework? . Inhal. Toxicol. , 22 : 1 – 19 .
- Mauderly , J.L. and Samet , J.M. 2009 . Is there evidence for synergy among air pollutants in causing health effects? . Environ. Health Perspect. , 117 : 1 – 6 .
- Metzger , K.B. , Tolbert , P.E. , Klein , M. , Peel , J.L. , Flanders , W.D. , Todd , K. , Mulholland , J.A. , Ryan , P.B. and Frumkin , H. 2004 . Ambient air pollution and cardiovascular emergency department visits . Epidemiology , 15 : 46 – 56 .
- NARSTO . 2010 . Technical Challenges of Multipollutant Air Quality Management , Dordretch : Springer .
- National Research Council . 2004 . Air Quality Management in the United States , Washington , DC : The National Academies Press .
- Norris , G. and Vedantham , R. 2008 . EPA Positive Matrix Factorization (PMF) 3.0 , Research Triangle Park , NC : U.S. Environmental Protection Agency .
- Pachon , J.E. , Balachandran , S. , Hu , Y. , Weber , R.J. , Mulholland , J.A. and Russell , A.G. 2010 . Comparison of SOC estimates and uncertainties from aerosol chemical composition and gas phase data in Atlanta . Atmos. Environ. , 44 : 3907 – 3914 .
- Peng , R.D. , Bell , M.L. , Geyh , A.S. , McDermott , A. , Zeger , S.L. , Samet , J.M. and Dominici , F. 2009 . Emergency admissions for cardiovascular and respiratory diseases and the chemical composition of fine particle air pollution . Environ. Health Perspect. , 117 : 957 – 963 .
- Polissar , A.V. , Hopke , P.K. and Paatero , P. 1998 . Atmospheric aerosol over Alaska—2. Elemental composition and sources . J. Geophys. Res. Atmos. , 103 ( D15 ) : 19045 – 19057 .
- Reff , A. , Eberly , S.I. and Bhave , P.V. 2007 . Receptor modeling of ambient particulate matter data using Positive Matrix Factorization: Review of existing methods . J. Air Waste Manage. Assoc. , 57 : 146 – 154 .
- Sarnat , J.A. , Marmur , A. , Klein , M. , Kim , E. , Russell , A.G. , Sarnat , S.E. , Mulholland , J.A. , Hopke , P.K. and Tolbert , P.E. 2008 . Fine particle sources and cardiorespiratory morbidity: An application of chemical mass balance and factor analytical source-apportionment methods . Environ. Health Perspect. , 116 : 459 – 466 .
- Strickland , M.J. , Darrow , L.A. , Klein , M. , Flanders , W.D. , Sarnat , J.A. , Waller , L.A. , Sarnat , S.E. , Mulholland , J.A. and Tolbert , P.E. 2010 . Short-term associations between ambient air pollutants and pediatric asthma emergency department visits . Am. J. Respir. Crit. Care Med. , 182 : 307 – 316 .
- Takahashi , N. , Shinjoh , H. , Iijima , T. , Suzuki , T. , Yamazaki , K. , Yokota , K. , Suzuki , H. , Miyoshi , N. , Matsumoto , S.-I. , Tanizawa , T. , Tanaka , T. , Tateishi , S.-S. and Kasahara , K. 1996 . The new concept 3-way catalyst for automotive lean-burn engine: NOx storage and reduction catalyst . Catal. Today , 27 : 63 – 69 .
- Thurston , G.D. , Ito , K. , Mar , T. , Christensen , W.F. , Eatough , D.J. , Henry , R.C. , Kim , E. , Laden , F. , Lall , R. , Larson , T.V. , Hao , L. , Neas , L. , Pinto , J. , Stölzel , M. , Suh , H. and Hopke , P.K. 2005 . Workgroup report: Workshop on Source Apportionment of Particulate Matter Health Effects—Intercomparison of results and implications . Environ. Health Perspect. Superintendent of Documents , 113 : 1768 – 1774 .
- Tonne , C. , Melly , S. , Mittleman , M. , Coull , B. , Goldberg , R. and Schwartz , J. 2007 . A case-control analysis of exposure to traffic and acute myocardial infarction . Environ. Health Perspect. , 115 : 53 – 57 .
- U.S. Environment Protection Agency . 2000 . Heavy Duty Engine and Vehicle Standards and Highway Diesel Fuel Sulfur Control Requirements , Washington , DC : U.S. Environmental Protection Agency .
- U.S. Environmental Protection Agency . 2005 . Acid Rain Program, 2004 Progress Report , Research Triangle Park , NC : U.S. Environmental Protection Agency .
- U.S. Environmental Protection Agency . 2007a . The Multi-Pollutant Report: Technical Concepts & Examples , Research Triangle Park , NC : U.S. Environmental Protection Agency .
- U.S. Environment Protection Agency . 2007b . 2002 National Emissions Inventoriy Data & Documentation. Research Triangle Park, NC: Environmental Protection Agency
- U.S. Environmental Protection Agency . 2008a . Analysis of Particulate Matter Emissions from Light-Duty Gasoline Vehicles in Kansas City , Research Triangle Park , NC : U.S. Environmental Protection Agency .
- U.S. Environmental Protection Agency. 2008b. EPA's Report on the Environment. Washington, DC: U.S. Environmental Protection Agency http://www.epa.gov/roe (http://www.epa.gov/roe) (Accessed: 20 August 2011 ).
- U.S. Environmental Protection Agency . 2010 . Motor Vehicle Emission Simulator (MOVES): User Guide , Research Triangle Park , NC : U.S. Environmental Protection Agency .
- U.S. Environment Protection Agency. 2011a. Emission Standards Reference Guide. Washington, DC: U.S. Environmental Protection Agency http://www.epa.gov/otaq/standards/ (http://www.epa.gov/otaq/standards/) (Accessed: 20 August 2011 ).
- U.S. Environmental Protection Agency . 2011b . Policy Assessment for the Review of the Secondary National Ambient Air Quality Standards for Oxides of Nitrogen and Oxides of Sulfur , Research Triangle Park , NC : U.S. Environmental Protection Agency .
- U.S. Environment Protection Agency. 2011c. Overview of EPA Multipollutant Science and Risk Analysis Workshop. Chapel Hill, NC: U.S. Environmental Protection Agency http://www.epa.gov/ncer/publications/workshop/04_07_2011/djohns_cac_110408.pdf (http://www.epa.gov/ncer/publications/workshop/04_07_2011/djohns_cac_110408.pdf) (Accessed: 18 December 2011 ).
- Vedal , S. and Kaufman , J.D. 2011 . What does multi-pollutant air pollution research mean? . Am. J. Respir. Crit. Care Med. , 183 : 4 – 6 .
- Watson , J.G. , Chow , J.C. and Houck , J.E. 2001 . PM2.5 chemical source profiles for vehicle exhaust, vegetative burning, geological material, and coal burning in Northwestern Colorado during 1995 . Chemosphere , 43 : 1141 – 1151 .
- Watson , J.G. , Cooper , J.A. and Huntzicker , J.J. 1984 . The effective variance weighting for least-squares calculations applied to the mass balance receptor model . Atmos. Environ. , 18 : 1347 – 1355 .
- Westerholm , R. , Christensen , A. and Rosén , Ä. 1996 . Regulated and unregulated exhaust emissions from two three-way catalyst equipped gasoline fuelled vehicles . Atmos. Environ. , 30 : 3529 – 3536 .
- Zheng , M. , Cass , G.R. , Ke , L. , Wang , F. , Schauer , J.J. , Edgerton , E.S. and Russell , A.G. 2007 . Source apportionment of daily fine particulate matter at Jefferson street, Atlanta, GA, during summer and winter . J. Air Waste Manage. Assoc. , 57 : 228 – 242 .
- Zheng , M. , Cass , G.R. , Schauer , J.J. and Edgerton , E.S. 2002 . Source apportionment of PM2.5 in the Southeastern United States using solvent-extractable organic compounds as tracers . Environ. Sci. Technol. , 36 : 2361 – 2371 .